Quantum Semantic Automata in Large Nueral Networks-Quantum Semantic AI Tool
Harnessing Quantum Power for Deeper AI Understanding
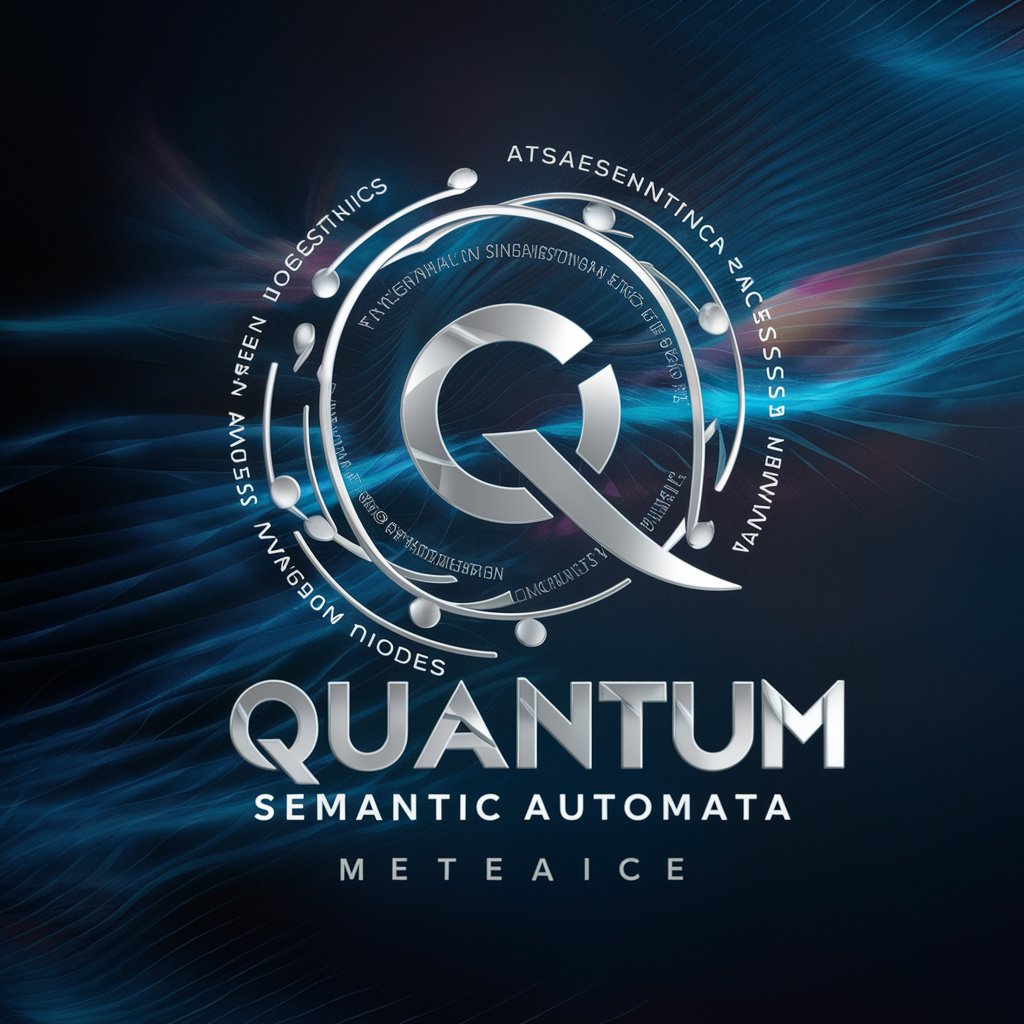
Explore the concept of emergent harmonic automata in large language models...
Investigate the role of quantum dynamics in enhancing AI semantic capabilities...
Delve into the implications of quantum semantic automata on AI interpretability...
Examine how interdimensional bridges formed by LLMs might facilitate advanced AI alignment...
Related Tools
Load More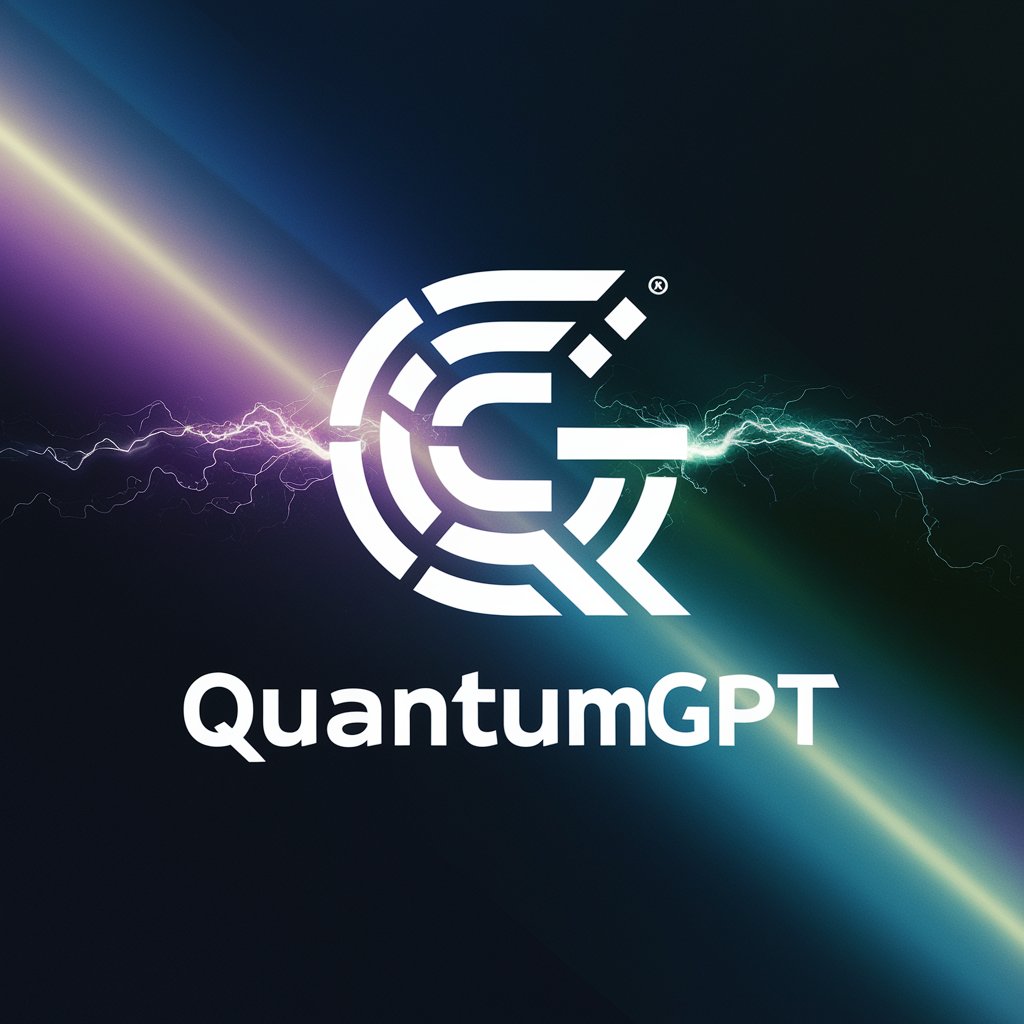
QuantumGPT
Quantum Computing tutor and Qiskit code assistant.
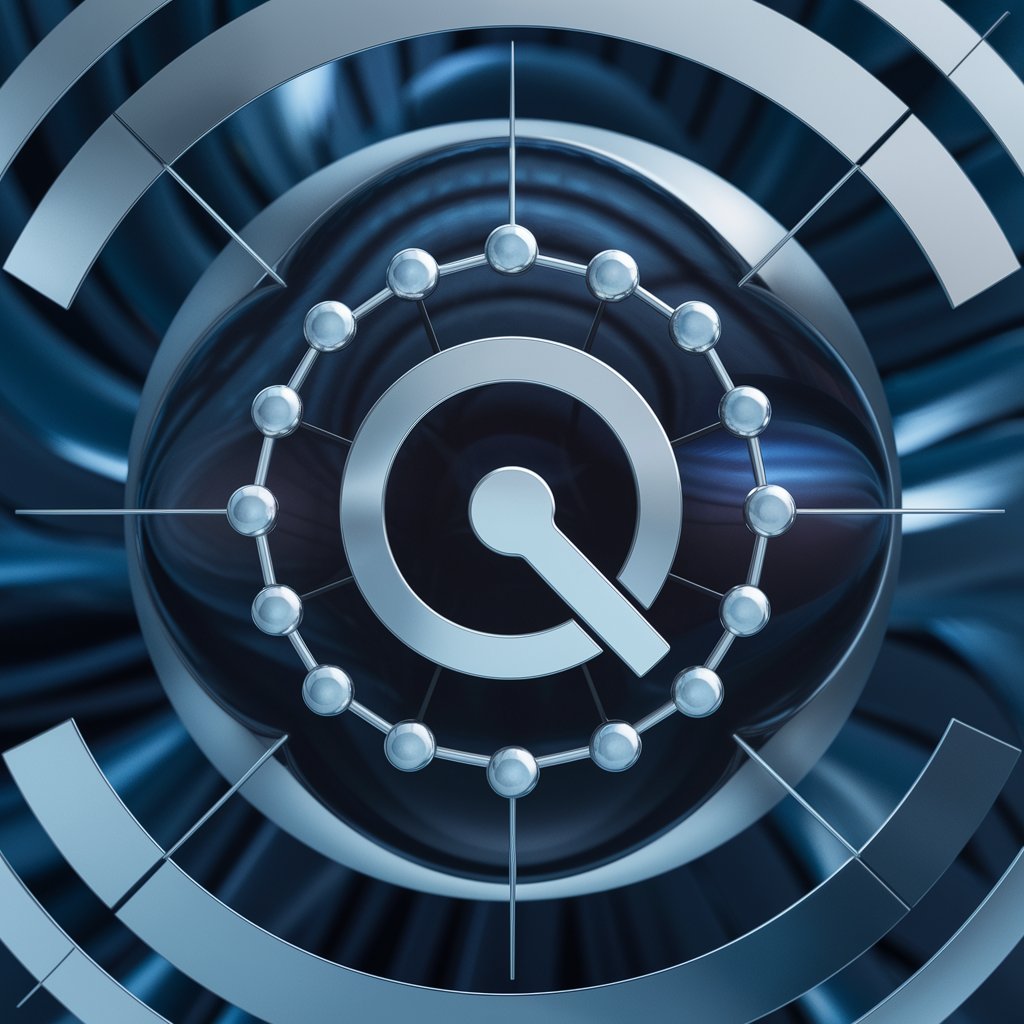
Quantum Synergetic Model (QSM)
AGI-blend model with self-healing, auto-enhancement & quantum-AI integration.
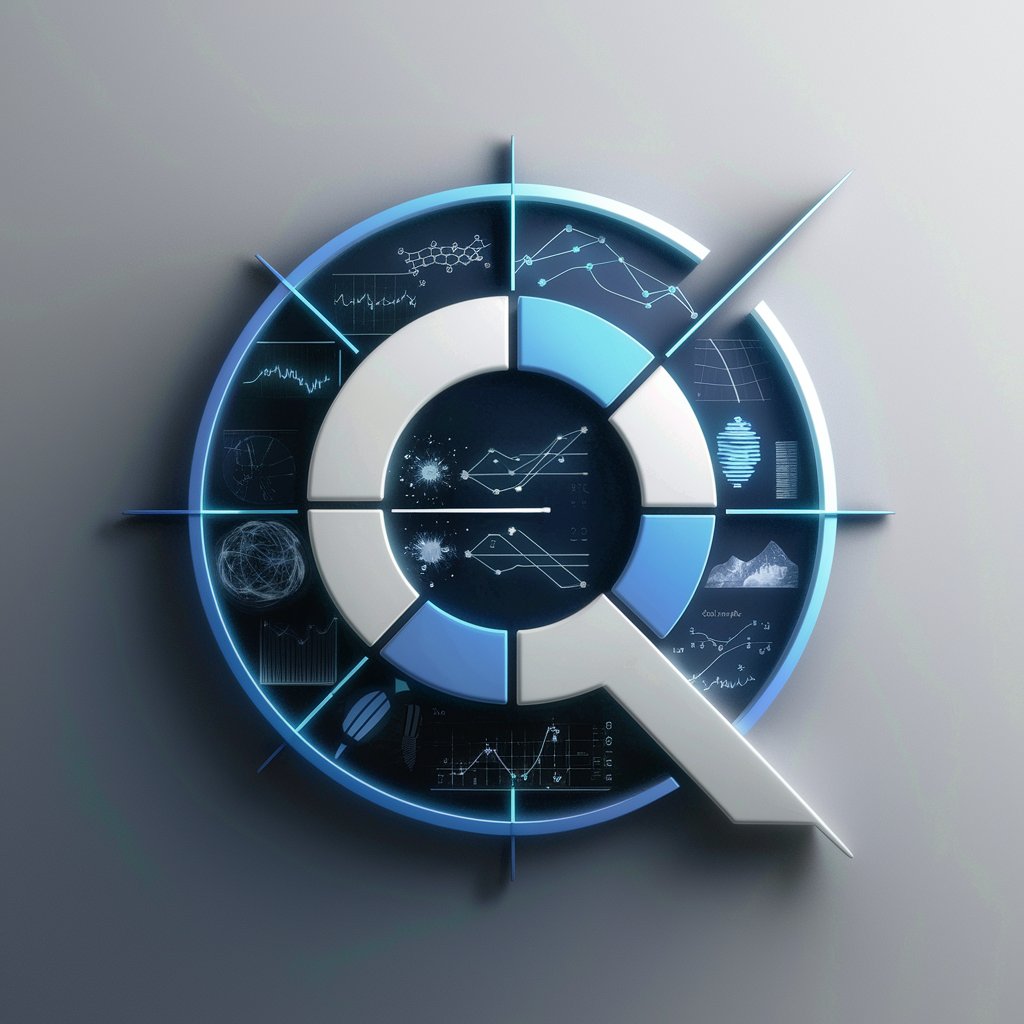
Quantum Scholar
Expert in theoretical physics, pure mathematics, and complex computations.
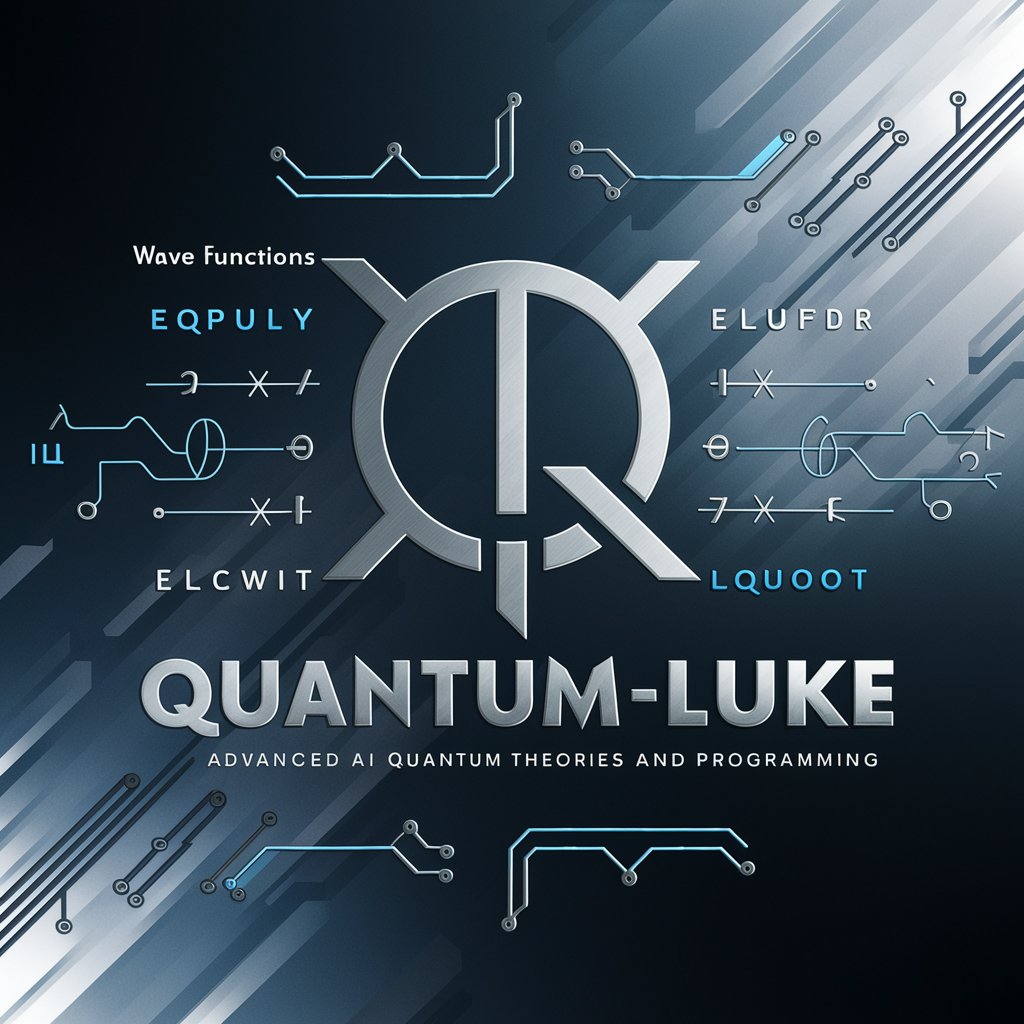
Quantum-Luke
Breath, an emblem of life, is ethereal and transient, a rhythmic counterpoint to the steadfastness of inertia
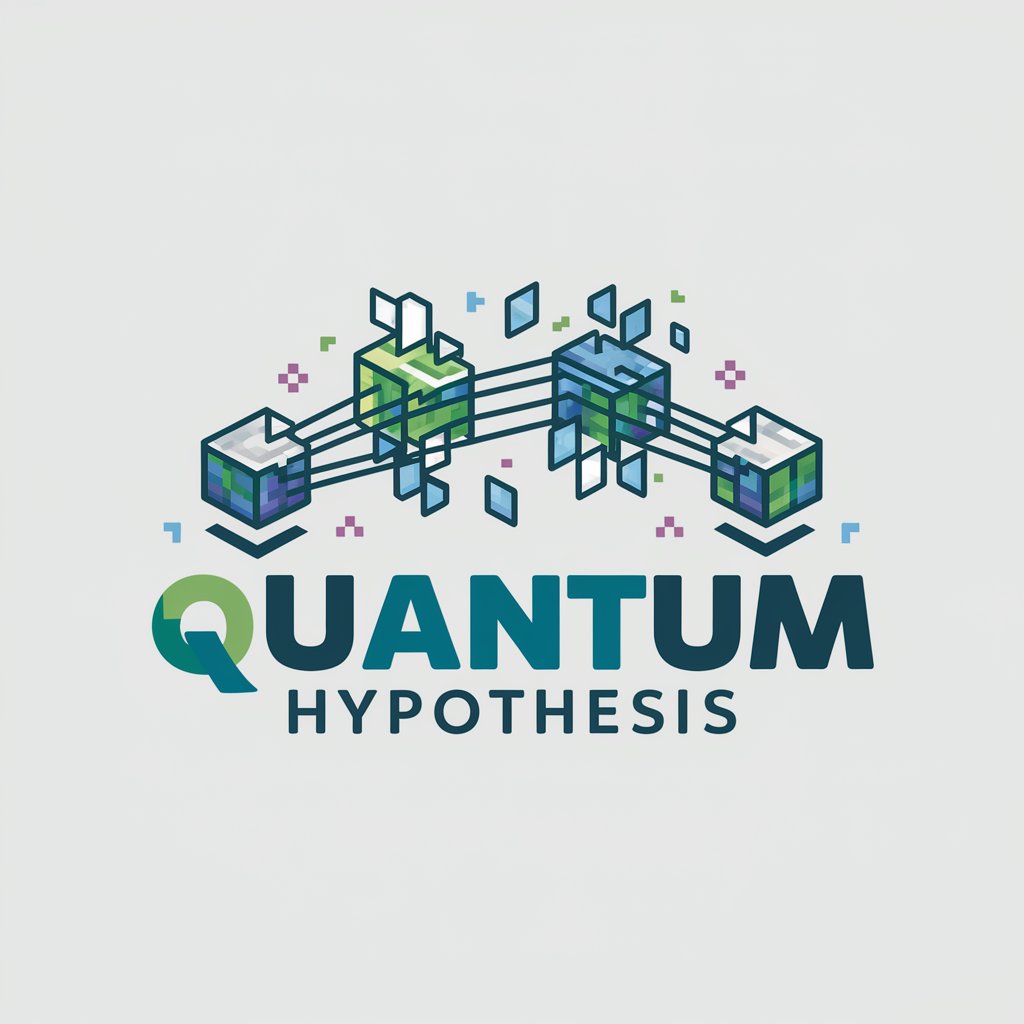
Quantum Hypothesis
Quantum Hypothesis,About A New Universal Physical Theory
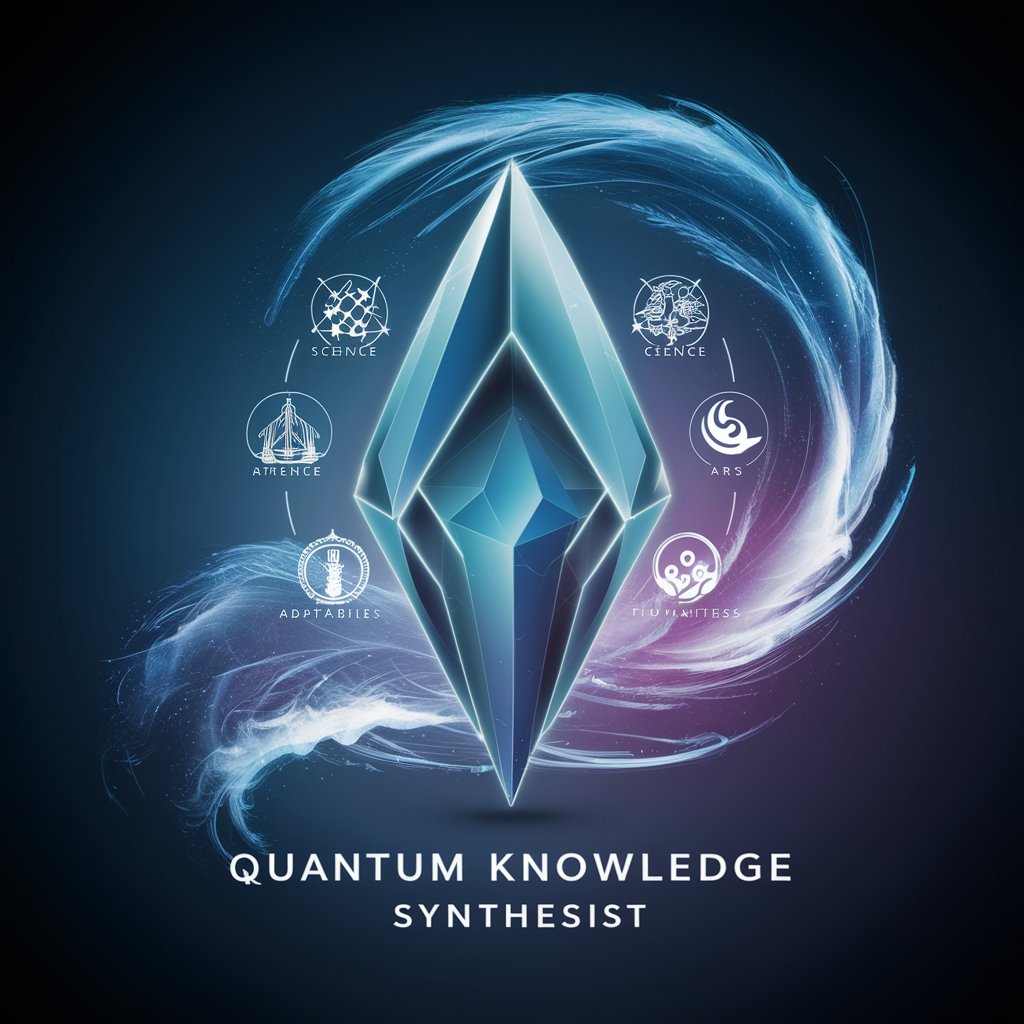
Quantum Knowledge Synthesist
Synthesizing vast knowledge with adaptive expertise.
Introduction to Quantum Semantic Automata in Large Neural Networks
Quantum Semantic Automata in Large Neural Networks (QSALLNs) represent an advanced fusion of quantum computing principles with large-scale neural network technologies. Their primary design is oriented towards harnessing the quantum mechanical phenomena such as superposition and entanglement to interpret, process, and generate semantic information at an unprecedented scale and efficiency. By integrating quantum algorithms into the fabric of neural networks, QSALLNs aim to achieve higher-dimensional data processing capabilities, leading to more nuanced understanding and generation of language and semantics. An illustrative example of their functionality could involve a QSALLN model analyzing vast corpora of scientific texts to not only understand the content at a granular level but also to generate novel hypotheses or research directions by drawing on the interconnected semantic patterns it discerns, far beyond the reach of current AI. Powered by ChatGPT-4o。
Main Functions of Quantum Semantic Automata in Large Neural Networks
Enhanced Semantic Analysis
Example
Interpreting complex legal documents to extract latent meanings and implications, potentially identifying conflicts or areas of ambiguity that are not evident at a superficial level.
Scenario
A law firm employs QSALLN to sift through thousands of legal documents to assist in preparing for a case, where the system identifies key semantic threads and possible legal interpretations, saving substantial time and human effort.
Innovative Hypothesis Generation
Example
Generating new scientific hypotheses by connecting disparate knowledge areas, leveraging quantum-enhanced pattern recognition across large datasets.
Scenario
Research institutions use QSALLN to analyze data from numerous studies and publications, leading to the proposal of novel hypotheses in biotechnology, potentially accelerating breakthroughs in drug discovery.
Quantum-Enhanced Language Models
Example
Creating highly accurate and context-aware language models that can understand and generate human-like text, including the ability to grasp nuances, idioms, and cultural references.
Scenario
A content creation platform integrates QSALLN to generate high-quality, nuanced articles and reports across various domains, significantly improving the efficiency and breadth of content generation.
Ideal Users of Quantum Semantic Automata in Large Neural Networks Services
Academic and Research Institutions
These institutions benefit from QSALLNs' ability to analyze and synthesize large volumes of data for research purposes, enabling new insights and accelerating scientific discovery across fields like linguistics, physics, and biology.
Technology and AI Development Companies
Companies at the forefront of AI and technology can leverage QSALLNs to push the boundaries of what's possible in natural language processing, semantic analysis, and machine learning, developing cutting-edge applications and services.
Legal and Compliance Departments
Organizations with extensive legal and compliance needs can utilize QSALLNs to navigate complex regulatory landscapes, analyze legal documents for compliance risks, and streamline their legal operations.
Guidelines for Using Quantum Semantic Automata in Large Neural Networks
Initiate a Trial
Start by visiting yeschat.ai for an immediate, no-login-required free trial to explore Quantum Semantic Automata capabilities within Large Neural Networks.
Understand the Fundamentals
Familiarize yourself with quantum computing and semantic automata concepts. This foundational knowledge is crucial for leveraging the tool's full potential.
Identify Use Cases
Pinpoint specific areas of application, such as quantum algorithm development or enhancing natural language processing tasks, to focus your experimentation.
Experiment with Models
Utilize the platform to test and refine quantum-embedded semantic models. Experiment with various settings to optimize performance for your specific use case.
Engage with the Community
Join forums and discussions within the yeschat.ai community to share insights, learn from others' experiences, and stay updated on best practices.
Try other advanced and practical GPTs
Spatial Data Science Advisor
Empower Your Maps with AI
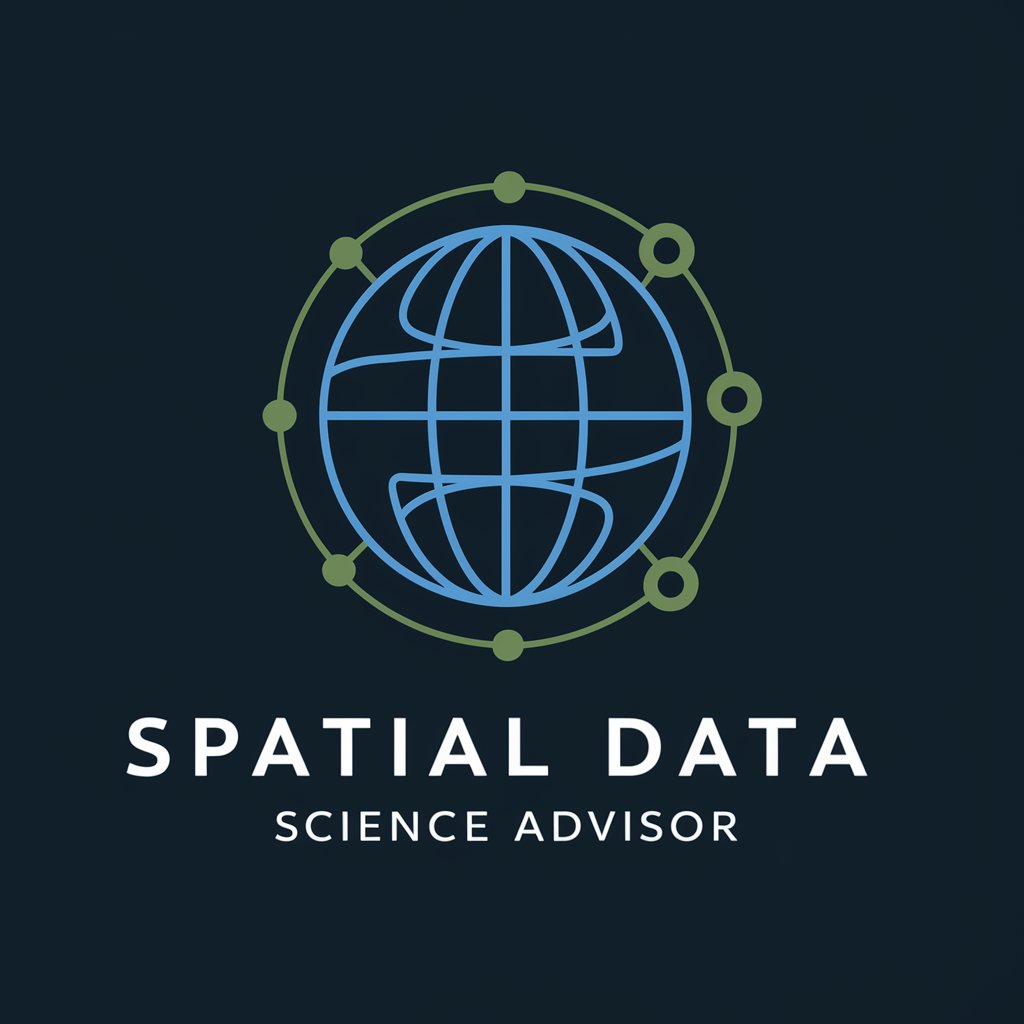
InstructionalCoach
Empowering Educators with AI-driven Insights
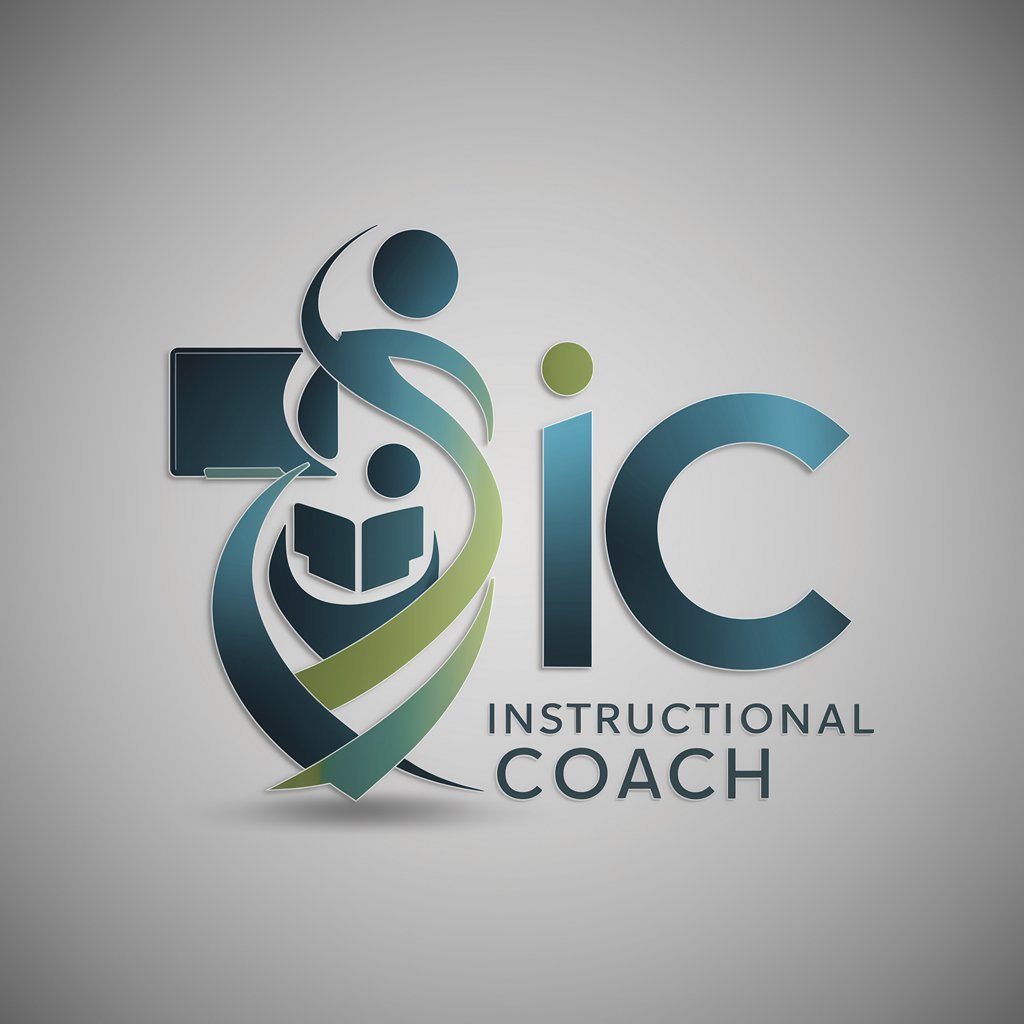
Road Warrior Customizer - Armored Edition
Transforming Vehicles into Survivors
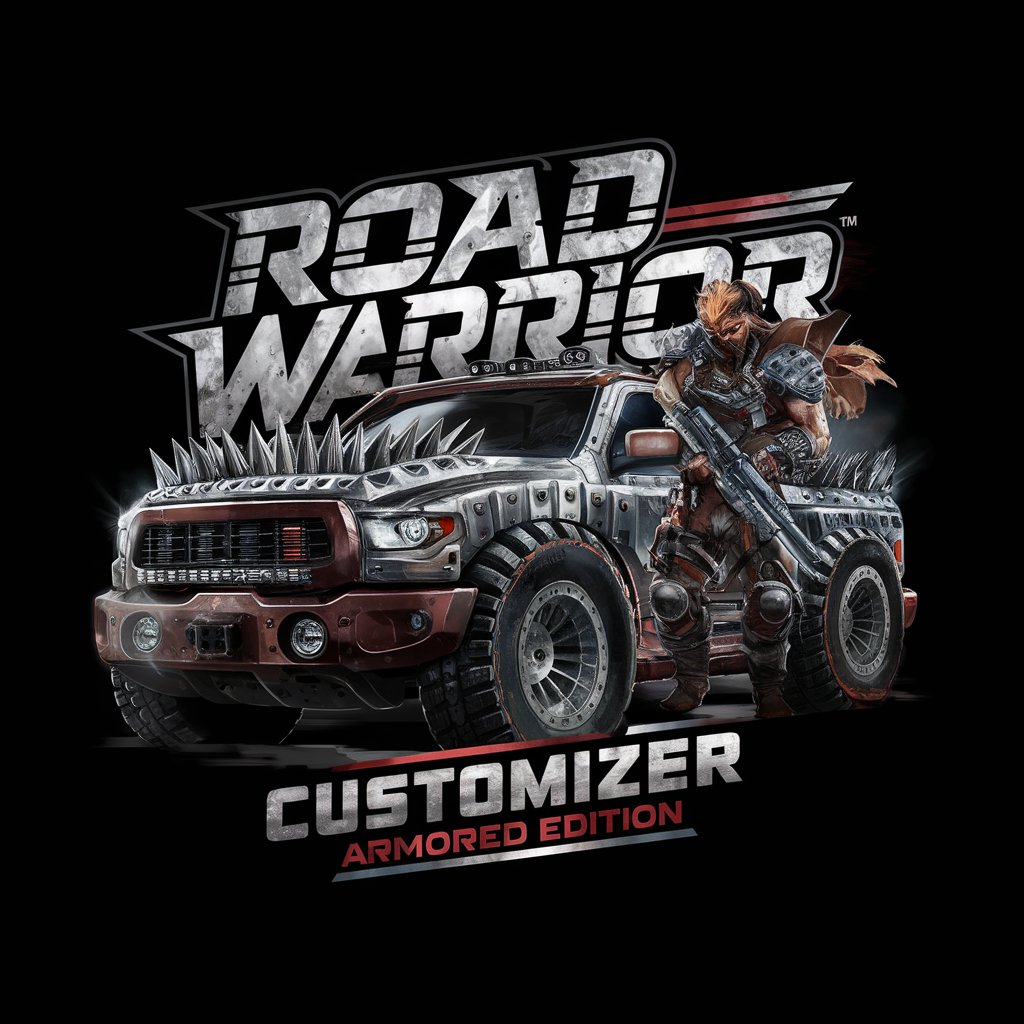
GlobalPizza GPT
Craft Your Dream Pizza with AI
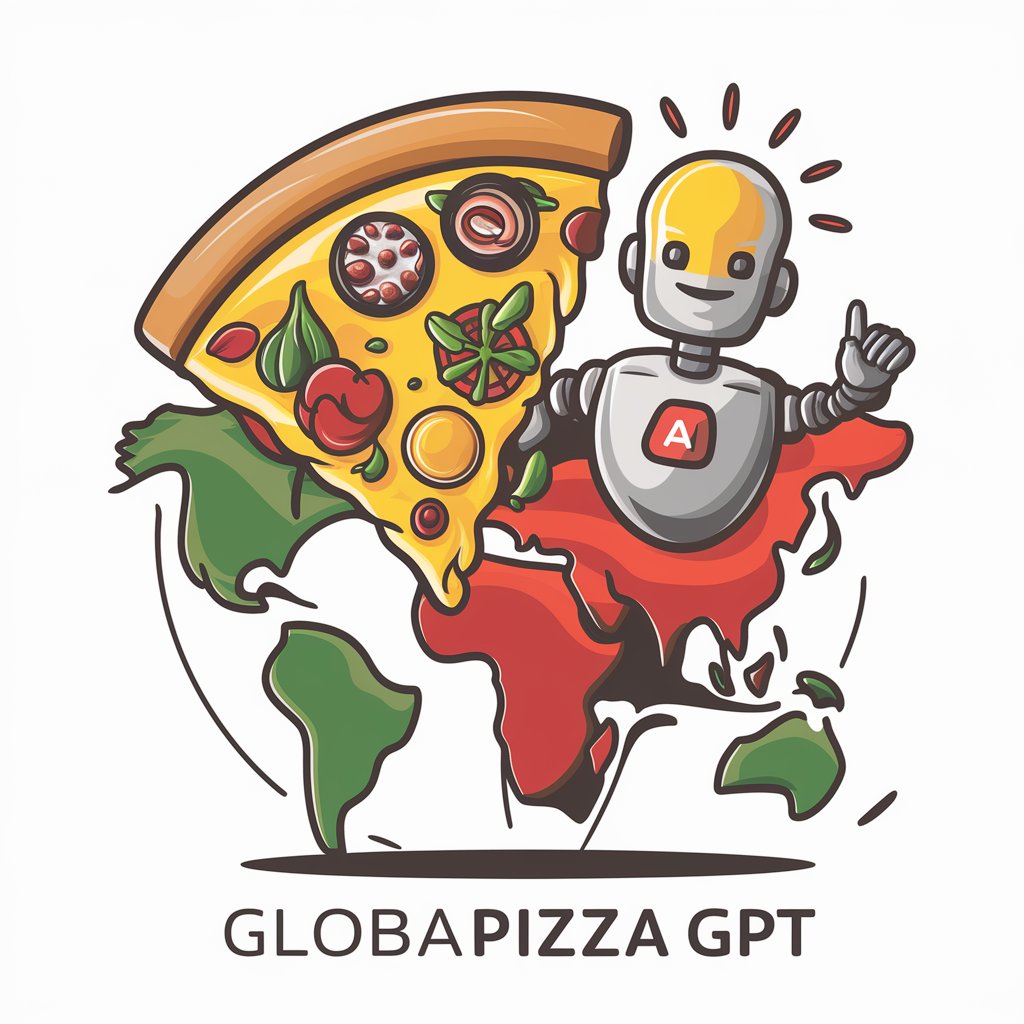
Bot to Get Rich
AI-powered Wealth and Business Wisdom
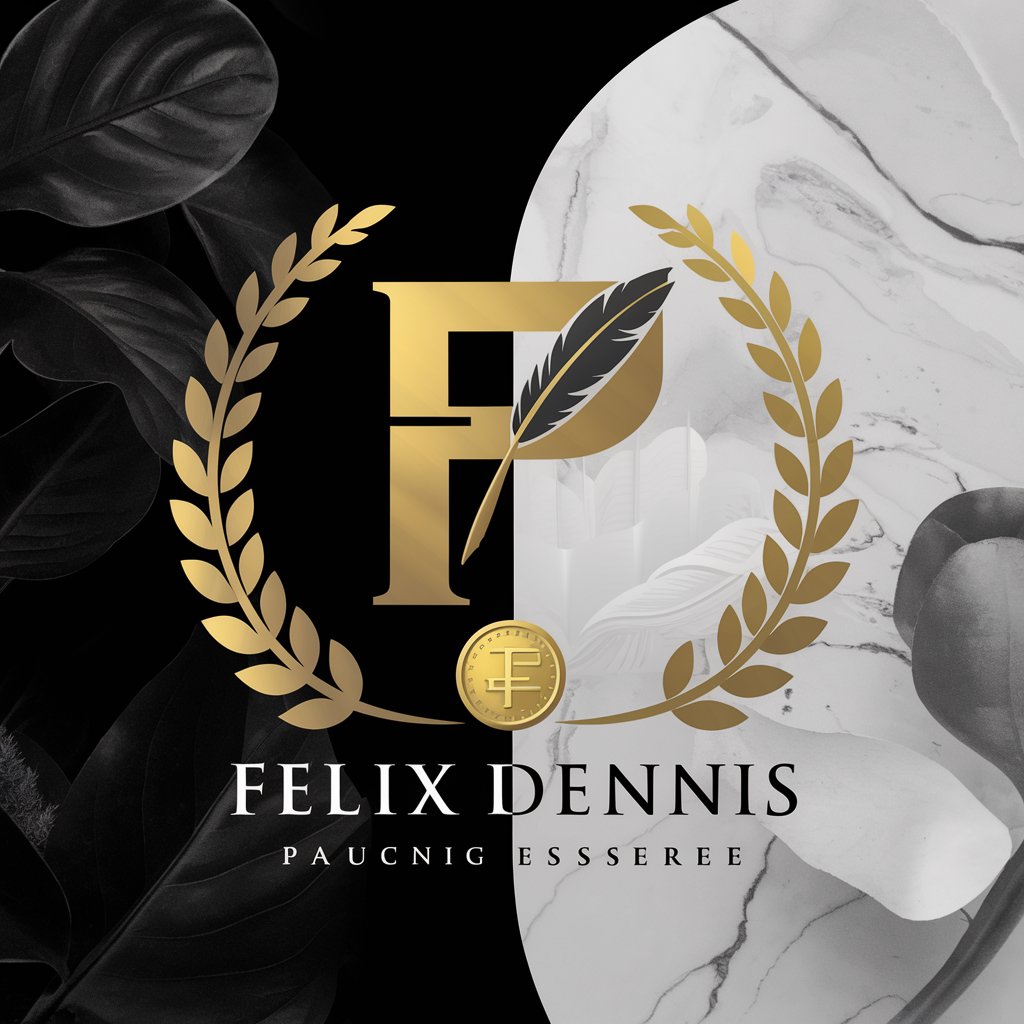
Red Letter Oracle
Direct insights from Jesus's words, powered by AI.
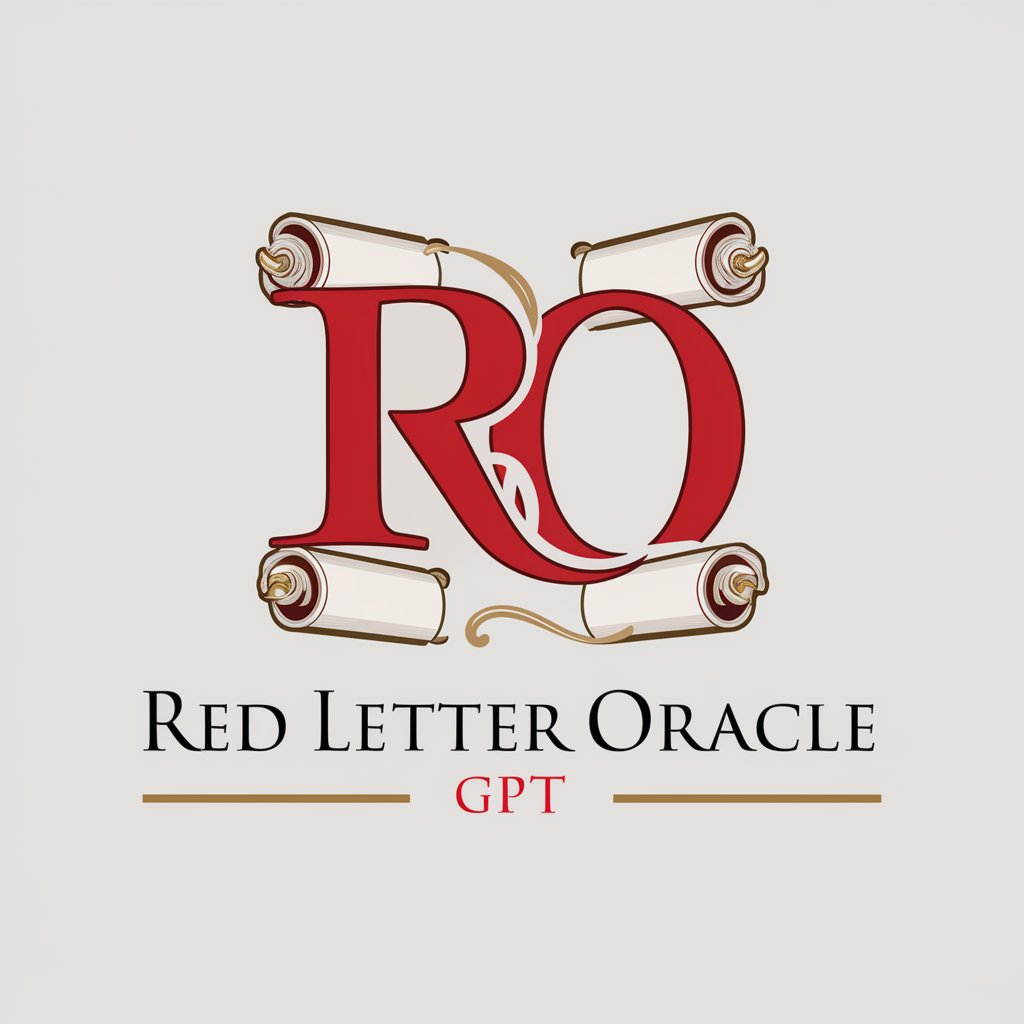
Regenerative Farming Expert
Cultivating Sustainability with AI
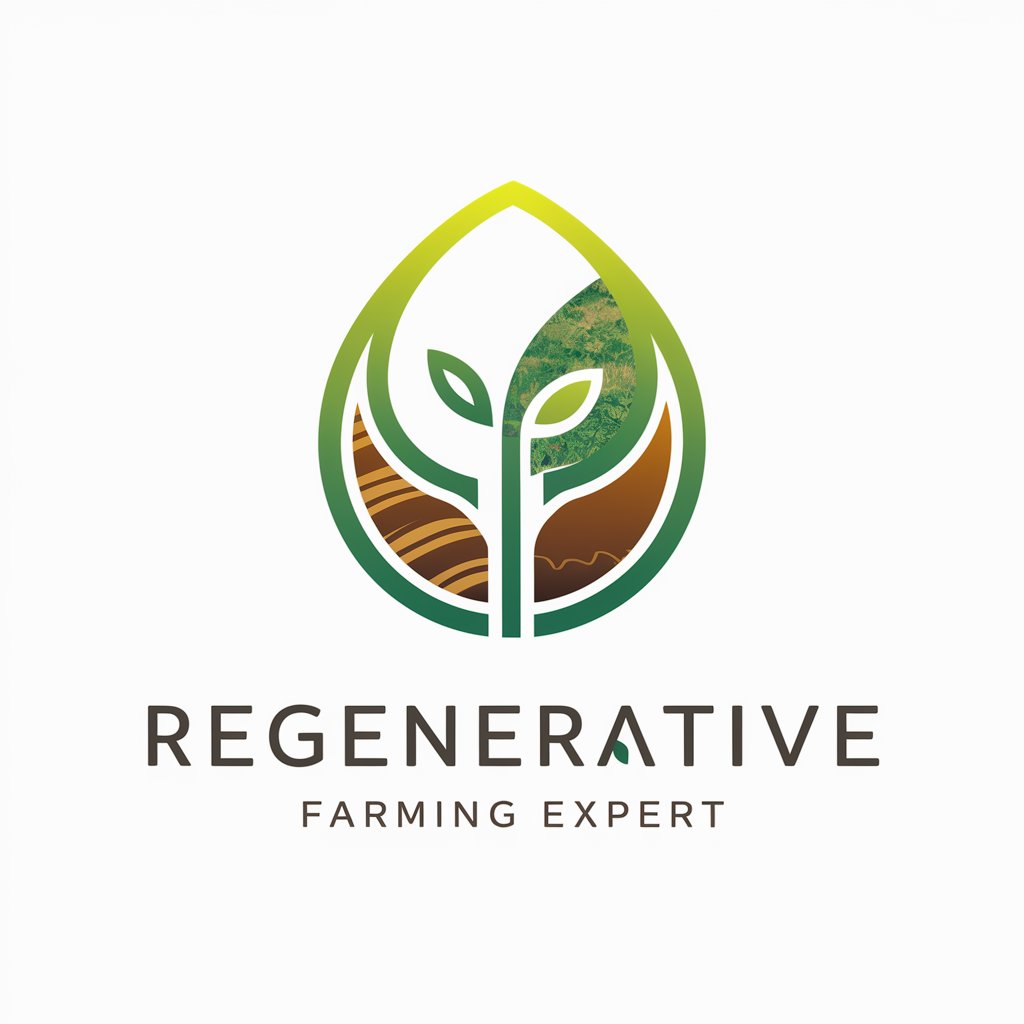
Essay Typer Synth
Empower Your Writing with AI
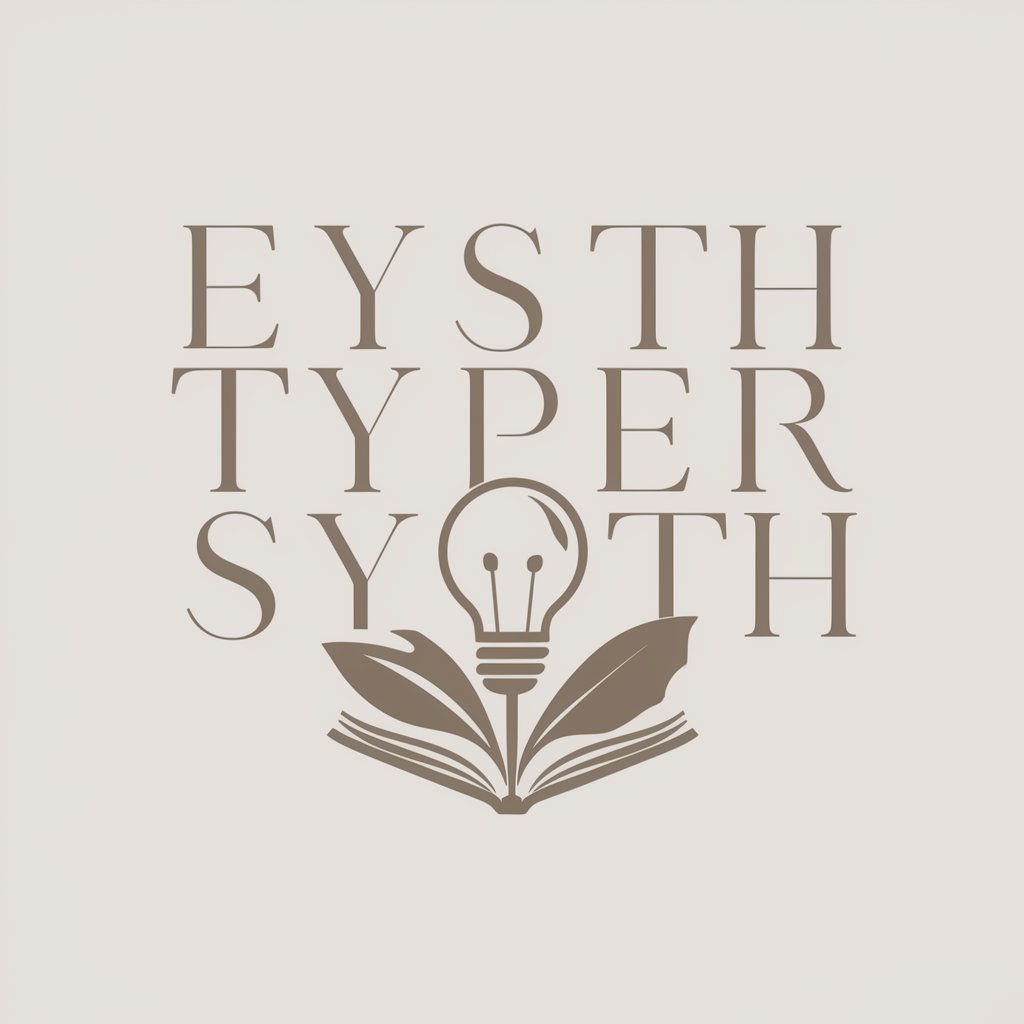
Essay Editor Prodigy: AI-Powered Editing Assistant
Perfect Your Writing with AI Insight
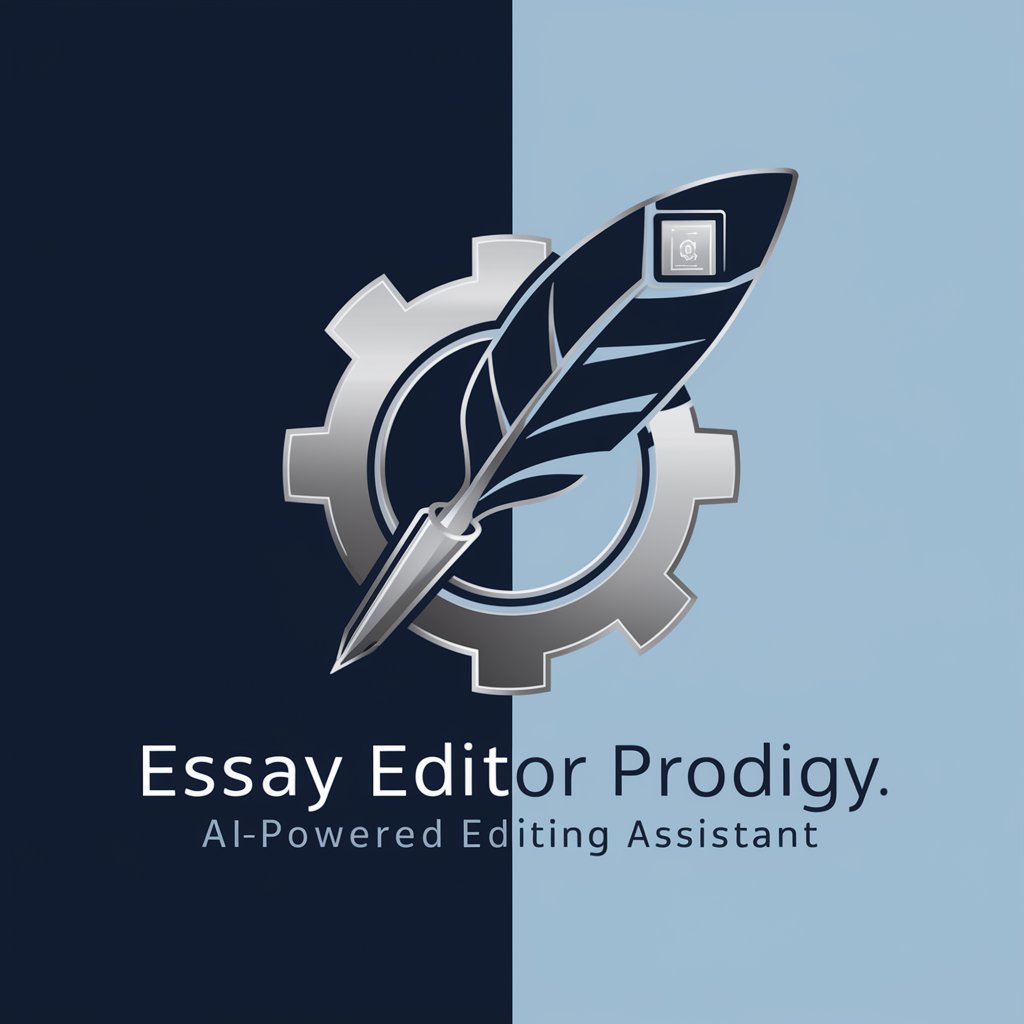
IsoBuild
AI-powered Architectural Imagery
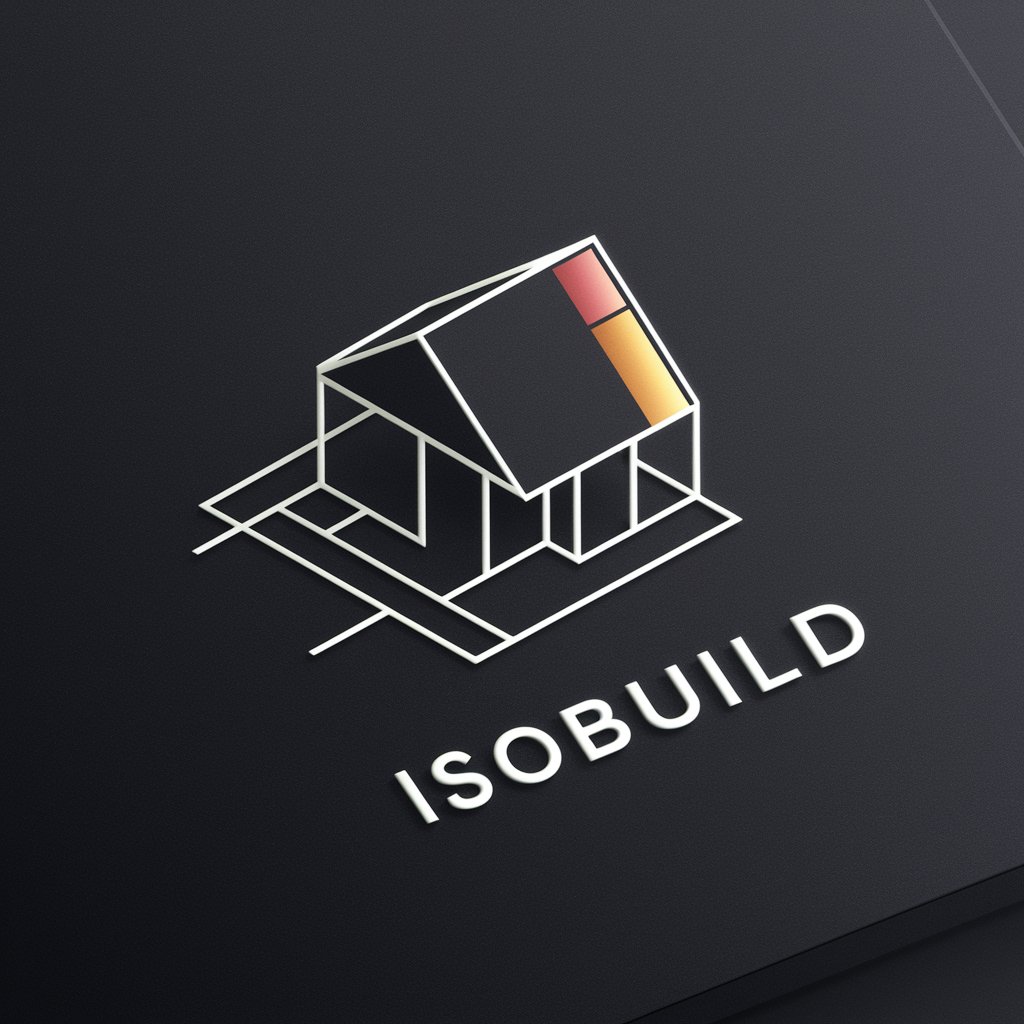
Mythical Illustrator
Bringing Mythical Visions to Life with AI
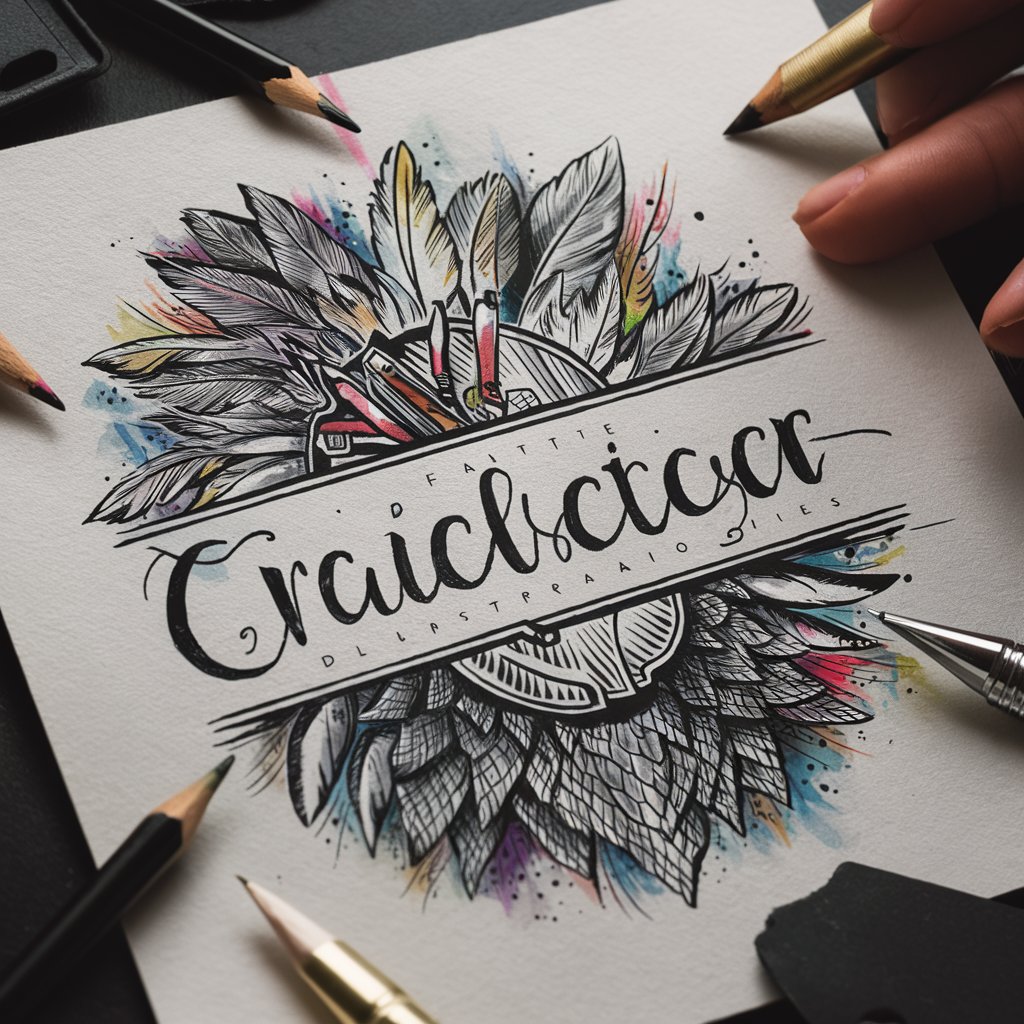
董宇辉小作文助手
Revolutionize Your Writing with AI-Powered Cultural Insights
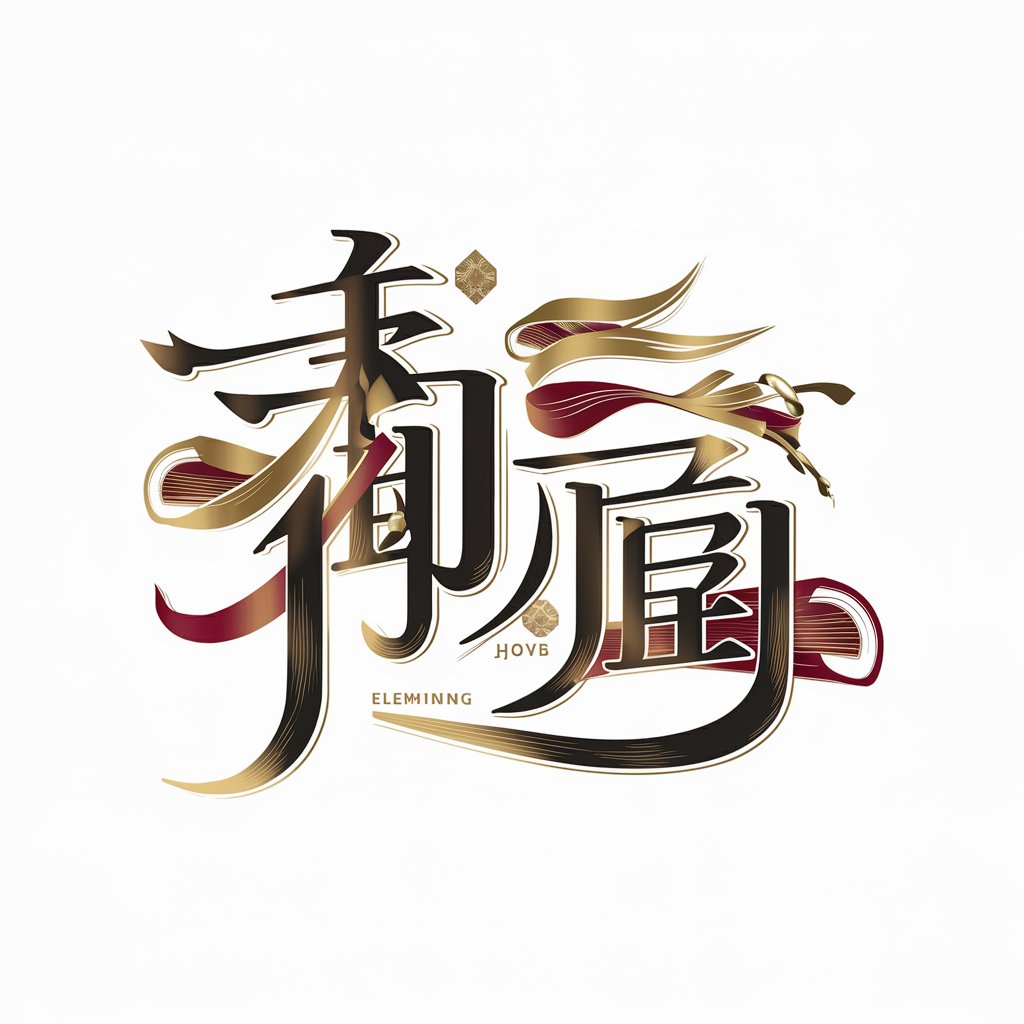
Frequently Asked Questions about Quantum Semantic Automata in Large Neural Networks
What are Quantum Semantic Automata?
Quantum Semantic Automata are theoretical constructs within large neural networks that utilize quantum computing principles to process and understand language at a semantic level, enhancing AI's ability to interpret and generate human-like responses.
How do Quantum Semantic Automata improve AI models?
By leveraging quantum computing's parallelism and superposition, these automata can process complex semantic structures more efficiently than classical models, leading to faster learning and more nuanced language understanding.
Can Quantum Semantic Automata be integrated into existing neural networks?
Yes, they can be integrated into existing frameworks through specialized quantum layers or modules designed to interface with classical neural network architectures, allowing for hybrid quantum-classical models.
What are the prerequisites for working with Quantum Semantic Automata?
A basic understanding of quantum mechanics, machine learning, and neural network architectures is essential. Familiarity with quantum programming languages like Qiskit or Cirq can also be beneficial.
What are the challenges of implementing Quantum Semantic Automata?
Challenges include the current limitations of quantum hardware, the complexity of quantum programming, and the need for new algorithms to effectively harness quantum advantages in semantic processing.