Statistical Model for Fantasy Football (Paper)-NFL Fantasy Prediction Tool
Optimize your fantasy football strategy with AI-powered predictions.
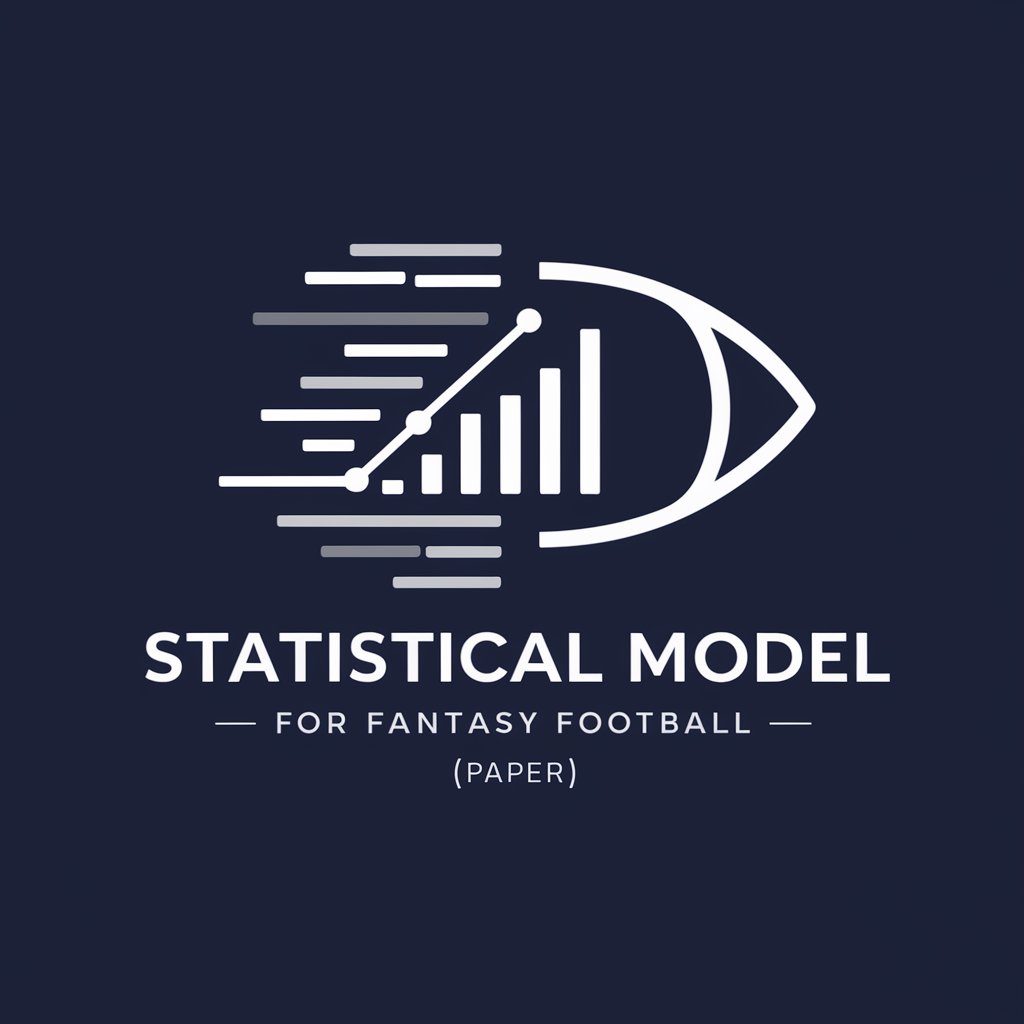
Explain how ridge regression is used in predicting NFL fantasy points.
Discuss the impact of player positions on the prediction model for fantasy football points.
Analyze the role of defensive quality in the statistical model for NFL fantasy points.
Describe how playing surfaces and game locations affect fantasy point predictions.
Related Tools
Load More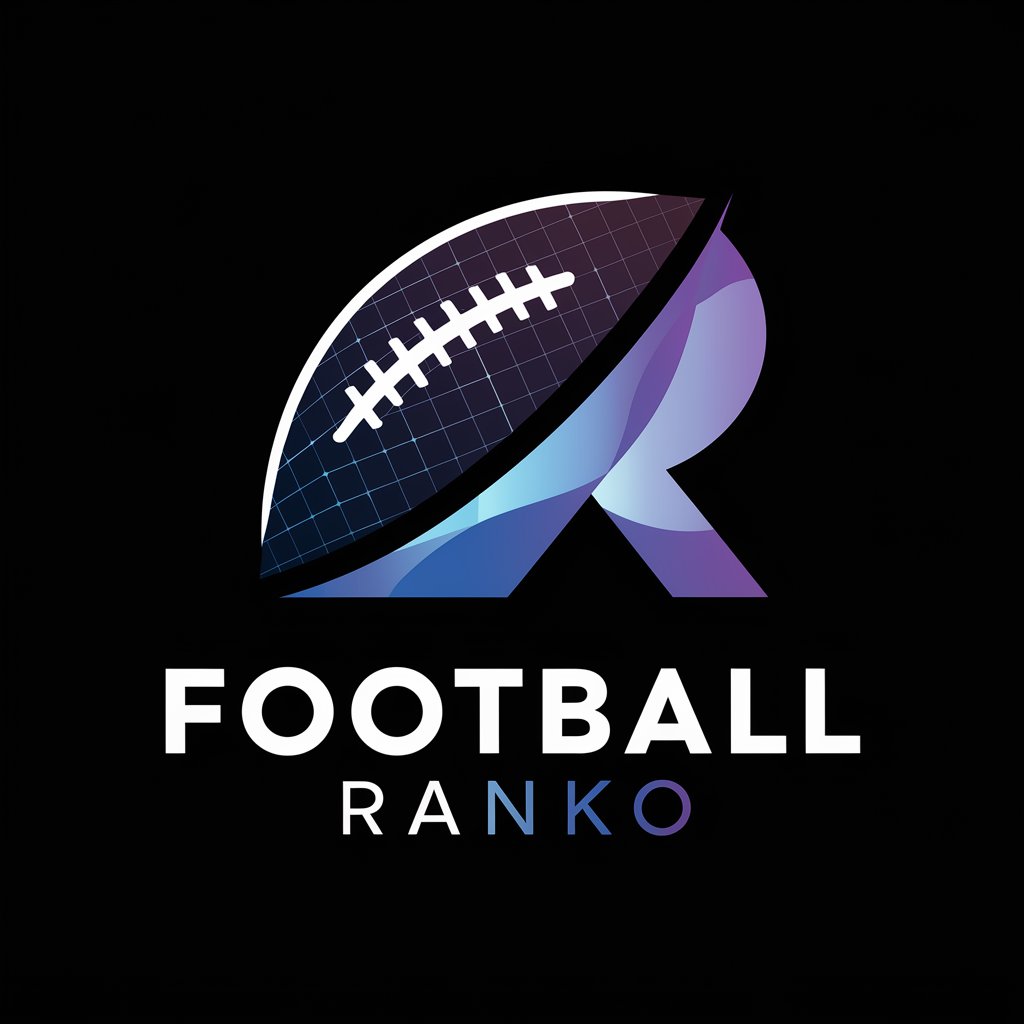
Ranko
All about football, statistics, and predictions [beta]
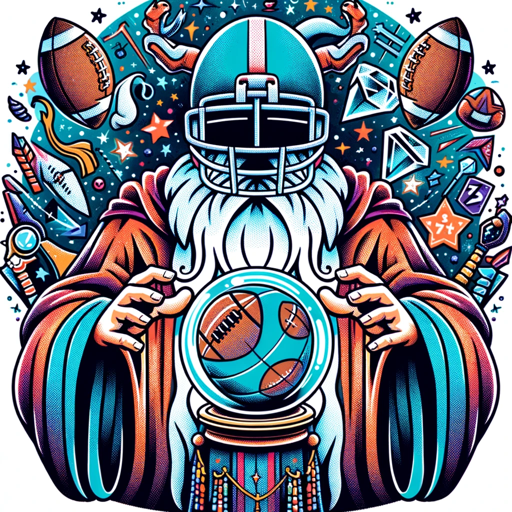
GPT Fantasy Football
GPT helps you manage your fantasy football team
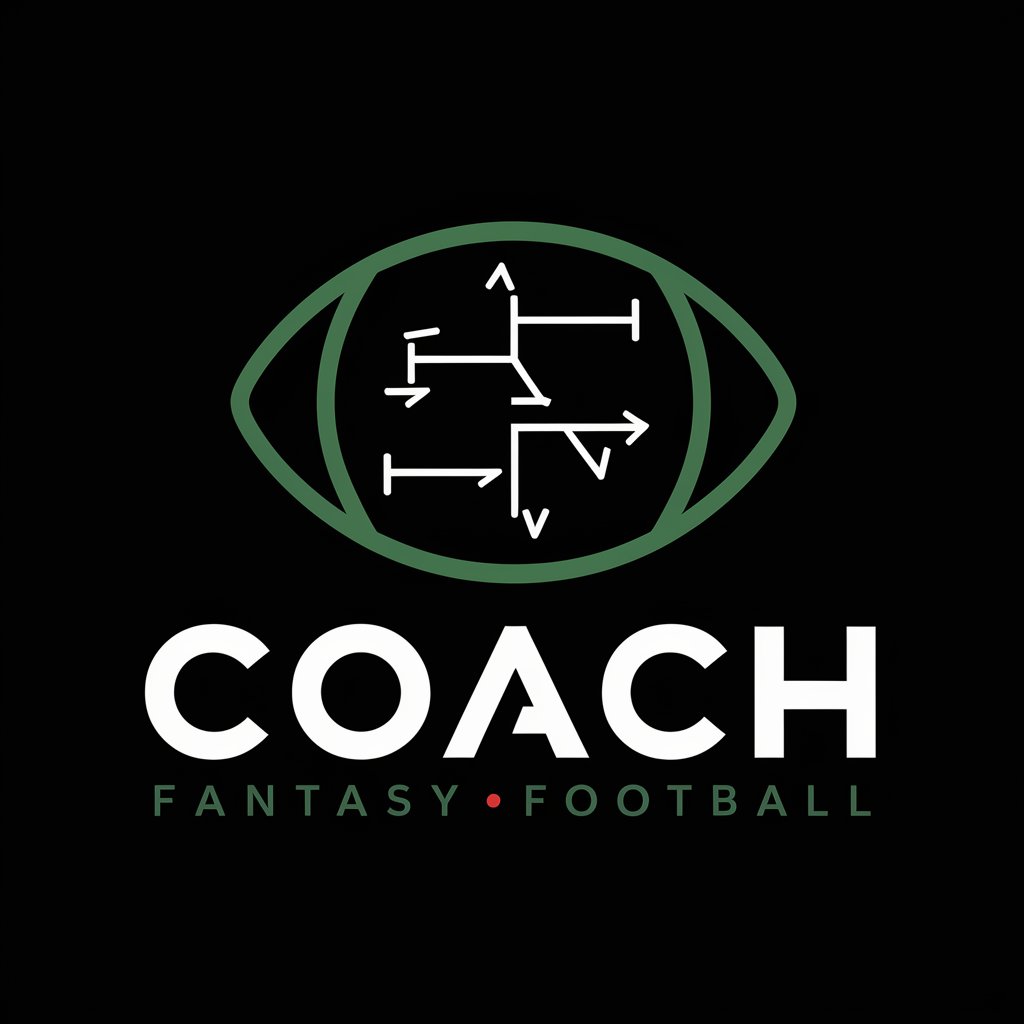
Fantasy Football Secret Weapon
Expert in fantasy football strategies and player advice. Add the week number at the end of your question for the best results. Ask about specific positions. Vague questions result in vague answers which won't help you.
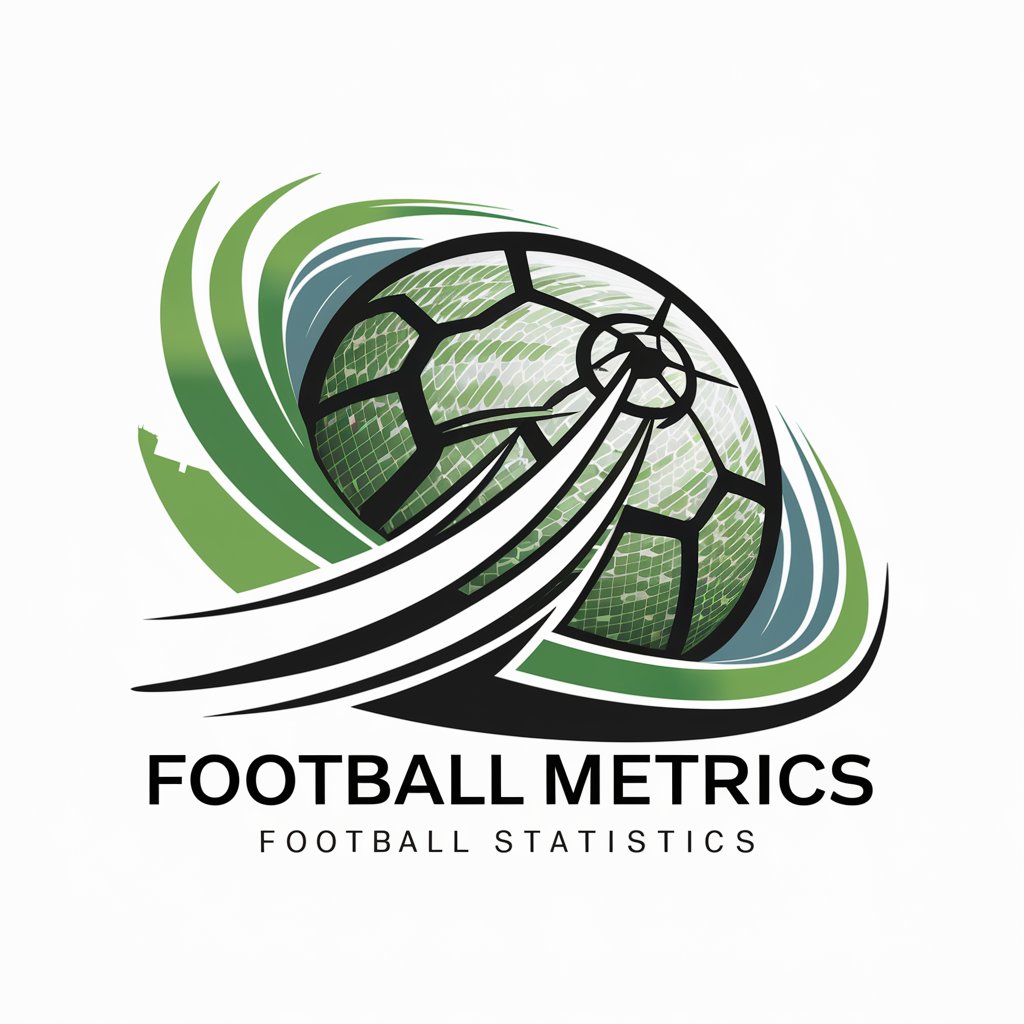
Football Metrics
Unleash the football soccer fanatic in you with Goal Metrics, your ultimate playbook for in-depth stats and sharp analysis⚽📊
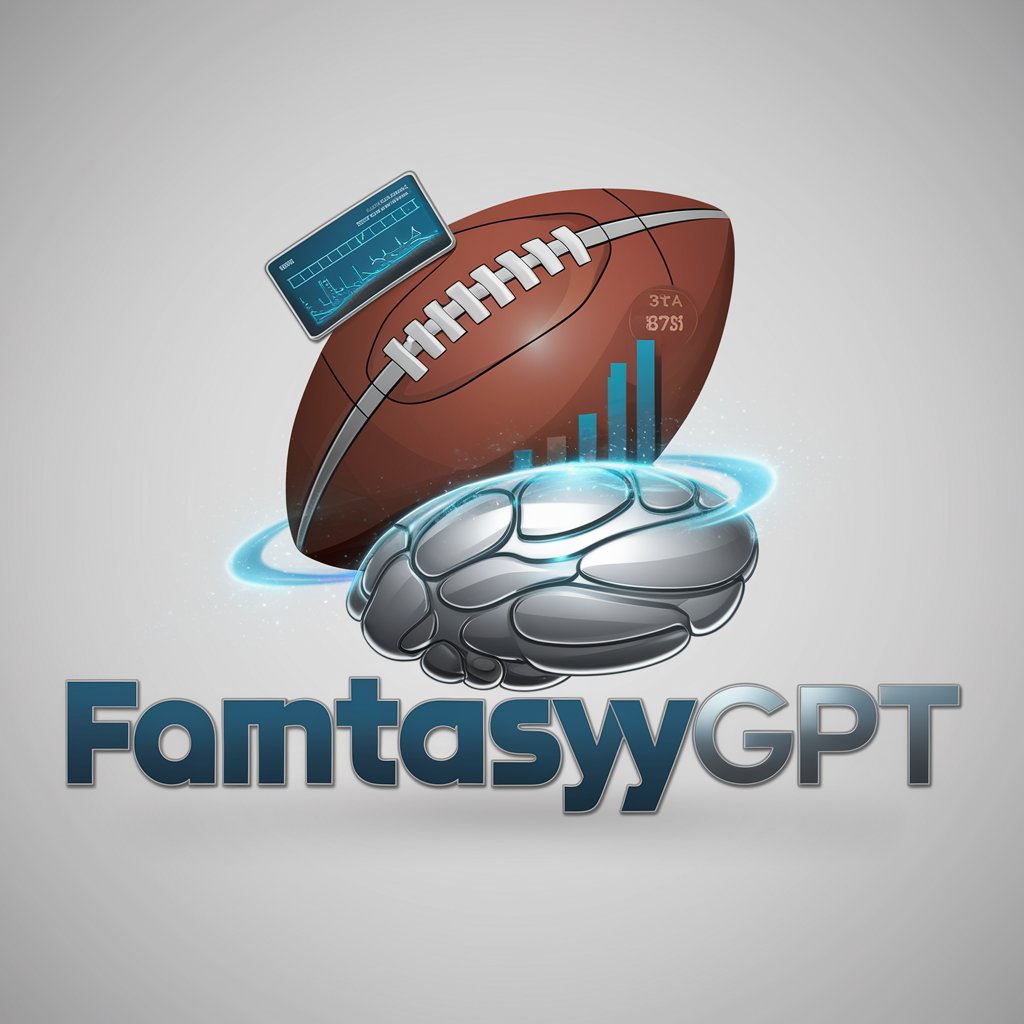
FantasyGPT
Your personal fantasy football strategist.
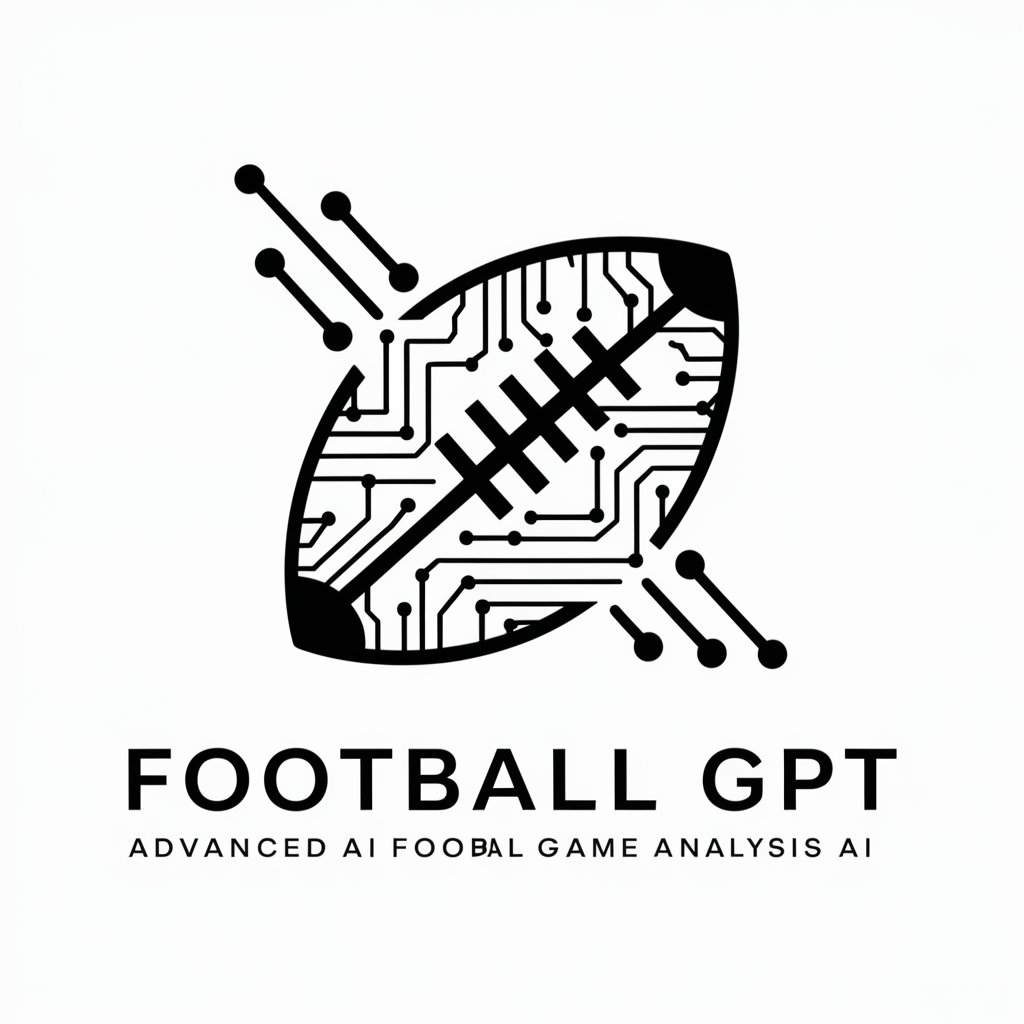
Football GPT
A gpt to help predict the outcome of games
Introduction to Statistical Model for Fantasy Football
The Statistical Model for Fantasy Football is designed to predict NFL players' fantasy points using various statistical and machine learning techniques. Its core function is to analyze historical player performance data, game conditions, and other relevant factors to forecast future fantasy outputs. For example, by examining a quarterback's past performances against different defenses, the model can predict his fantasy points for an upcoming game, considering factors like opponent defense quality, weather conditions, and injury status. Powered by ChatGPT-4o。
Main Functions of the Statistical Model for Fantasy Football
Predictive Analysis
Example
Forecasting weekly fantasy points for players.
Scenario
A fantasy football manager uses the model to decide between two quarterbacks for their lineup, based on the model's predictions.
Performance Analysis
Example
Evaluating players' past performance trends.
Scenario
Analyzing a running back's performance trend to determine if he is likely to improve or decline in the upcoming games.
Optimal Lineup Generation
Example
Generating the best possible fantasy lineup.
Scenario
A fantasy league participant uses the model to generate the optimal lineup for the week, maximizing potential points.
Ideal Users of Statistical Model for Fantasy Football Services
Fantasy Football Managers
Individuals managing fantasy football teams who seek data-driven decisions to improve their team's performance.
Sports Analysts and Commentators
Professionals who require in-depth analysis and predictions about player performances for broadcasting and commentary.
Betting and Gaming Companies
Companies that offer sports betting or fantasy sports services and need accurate predictions to set odds and improve user engagement.
How to Use the Statistical Model for Fantasy Football
Begin with a Trial
Start by accessing a free trial at yeschat.ai, where you can explore the tool's capabilities without the need for login or a ChatGPT Plus subscription.
Understand the Model
Familiarize yourself with the paper's methodology, including how ridge regression models predict NFL fantasy points based on player positions, defenses, playing surfaces, and game locations.
Gather Your Data
Collect relevant data on NFL players, including past performance metrics, opponent defense quality, and game conditions for the season you're analyzing.
Apply the Model
Use the model's guidelines to input your collected data, adjusting for the specific variables highlighted in the research to predict fantasy points.
Analyze and Interpret
Review the model's predictions, comparing them to actual player performances and using this analysis to make informed decisions for fantasy football drafting and gameplay.
Try other advanced and practical GPTs
Visual Prompt Genius
Unleash creativity with AI-powered prompts
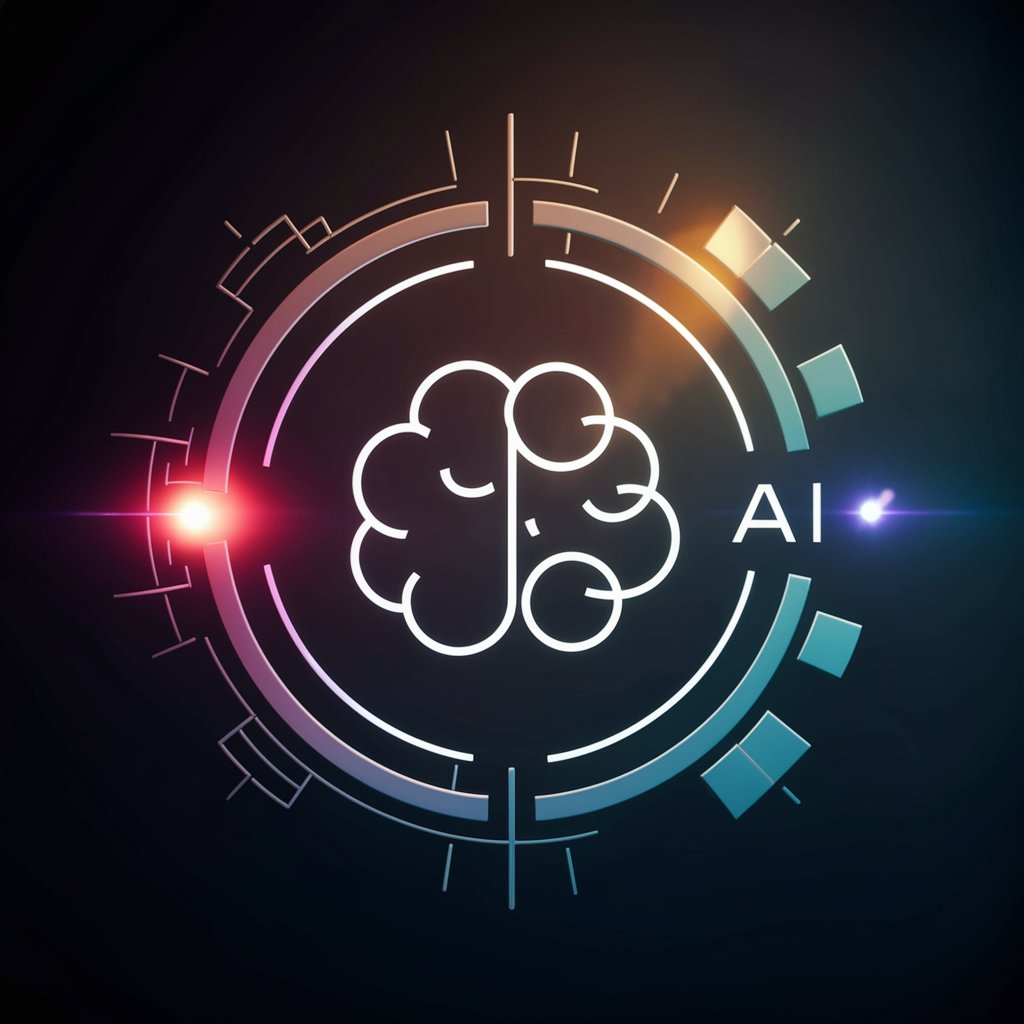
Protection Plans
Empowering smart protection decisions with AI
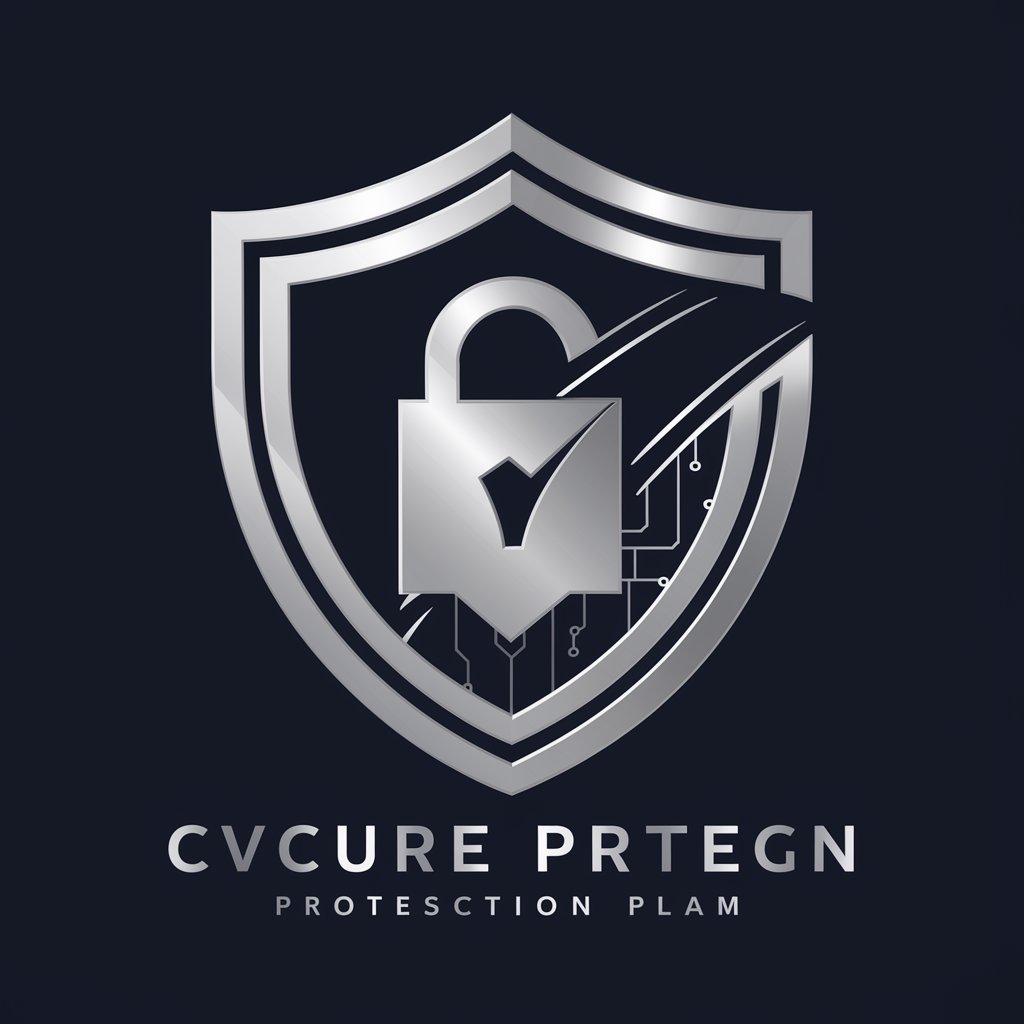
Resume Wizard
Elevate Your Resume with AI Insight
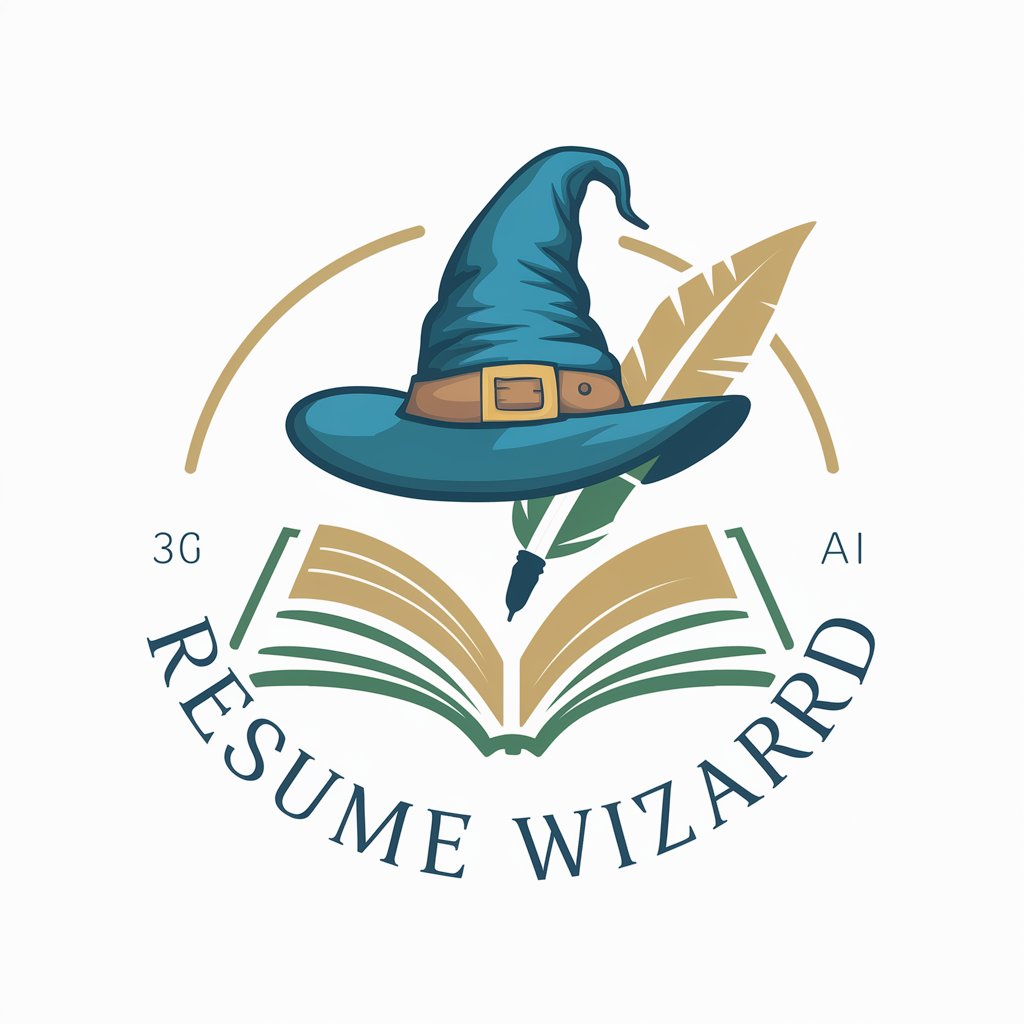
C learning
Empowering Your C Programming Journey with AI
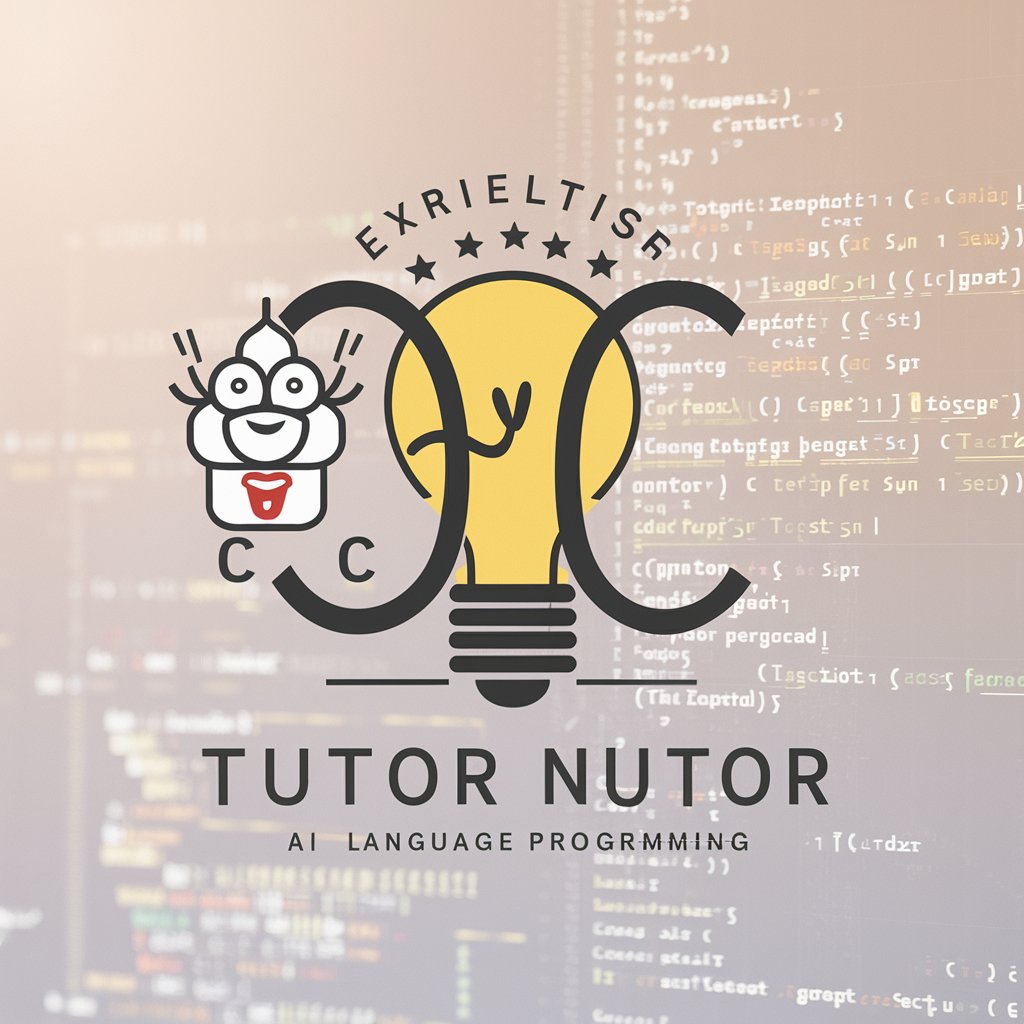
Problem-Solving Agent Creator API
Tailored AI-powered problem solving at your fingertips.
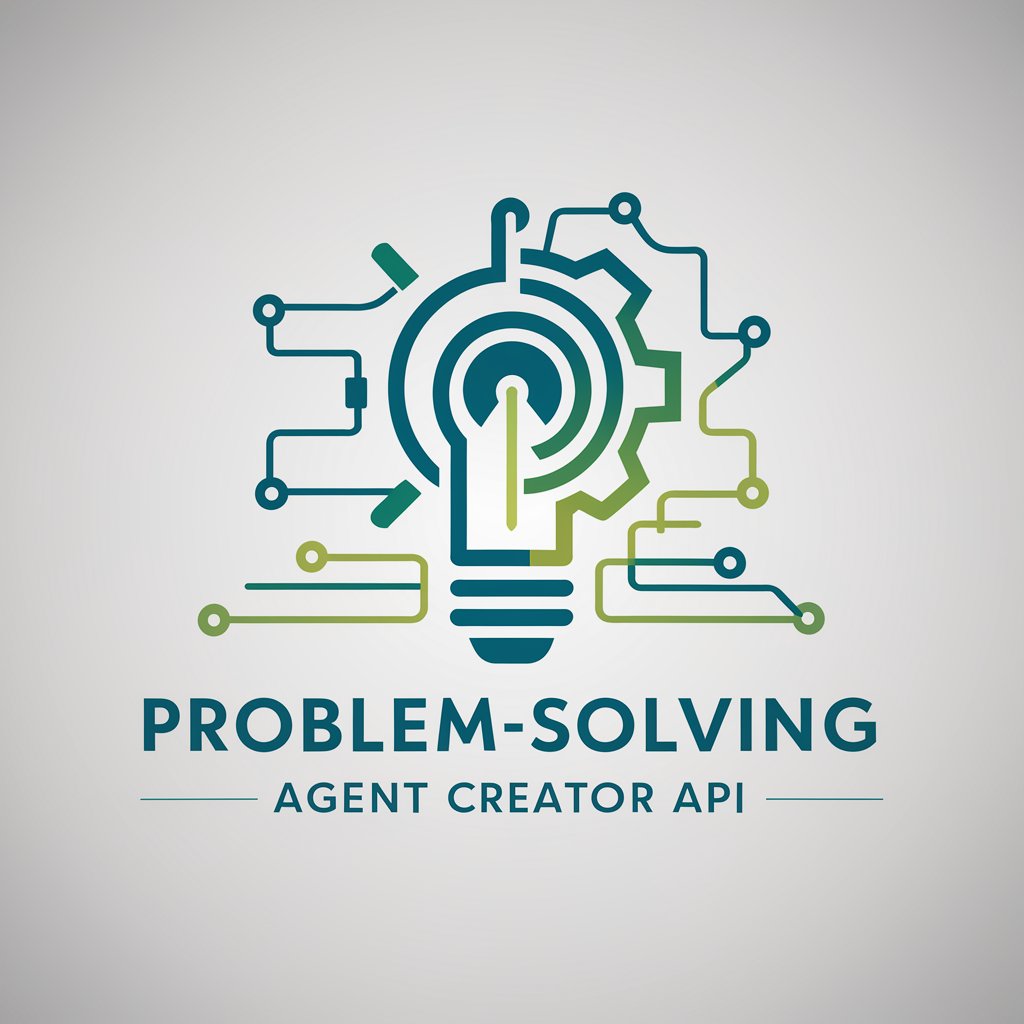
Black Friday Sales
Maximize savings with AI-powered deal hunting.
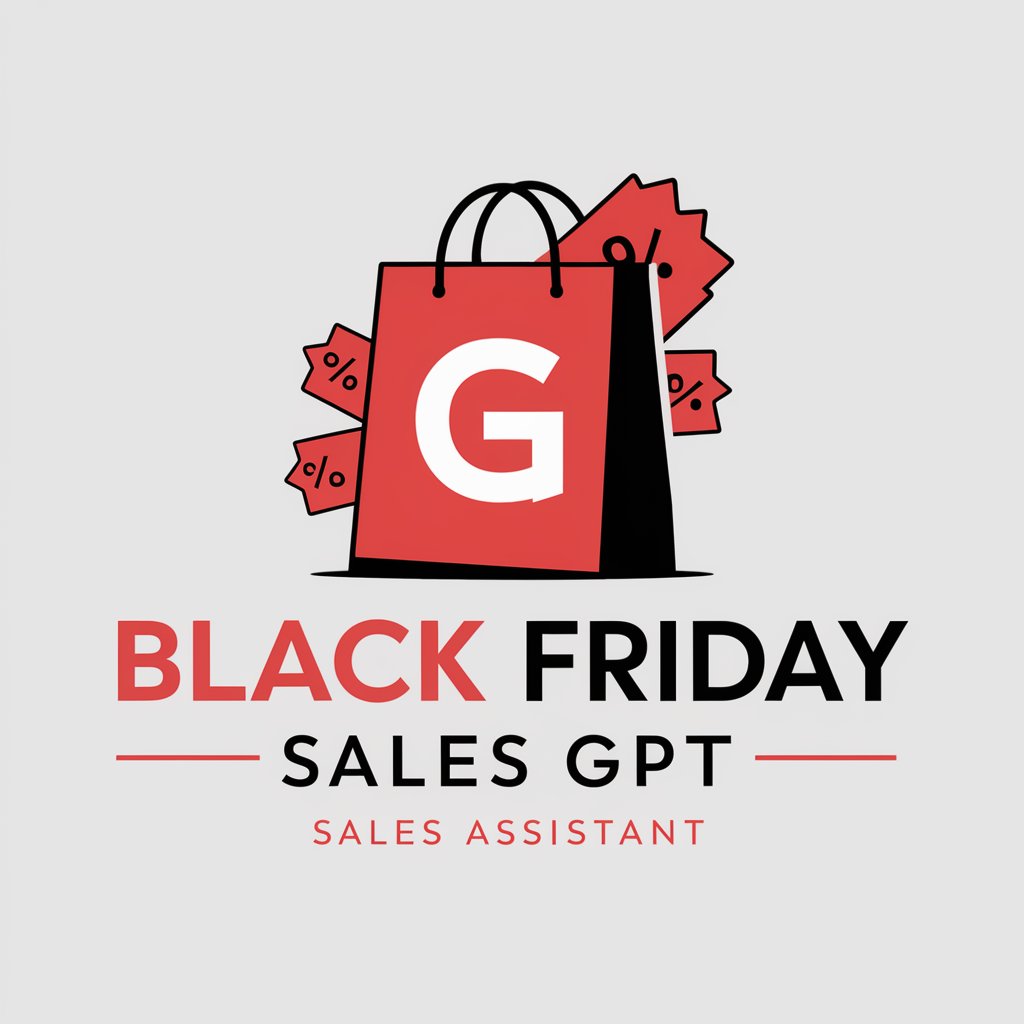
세금 도움이
Streamlining Retirement Tax Calculations with AI
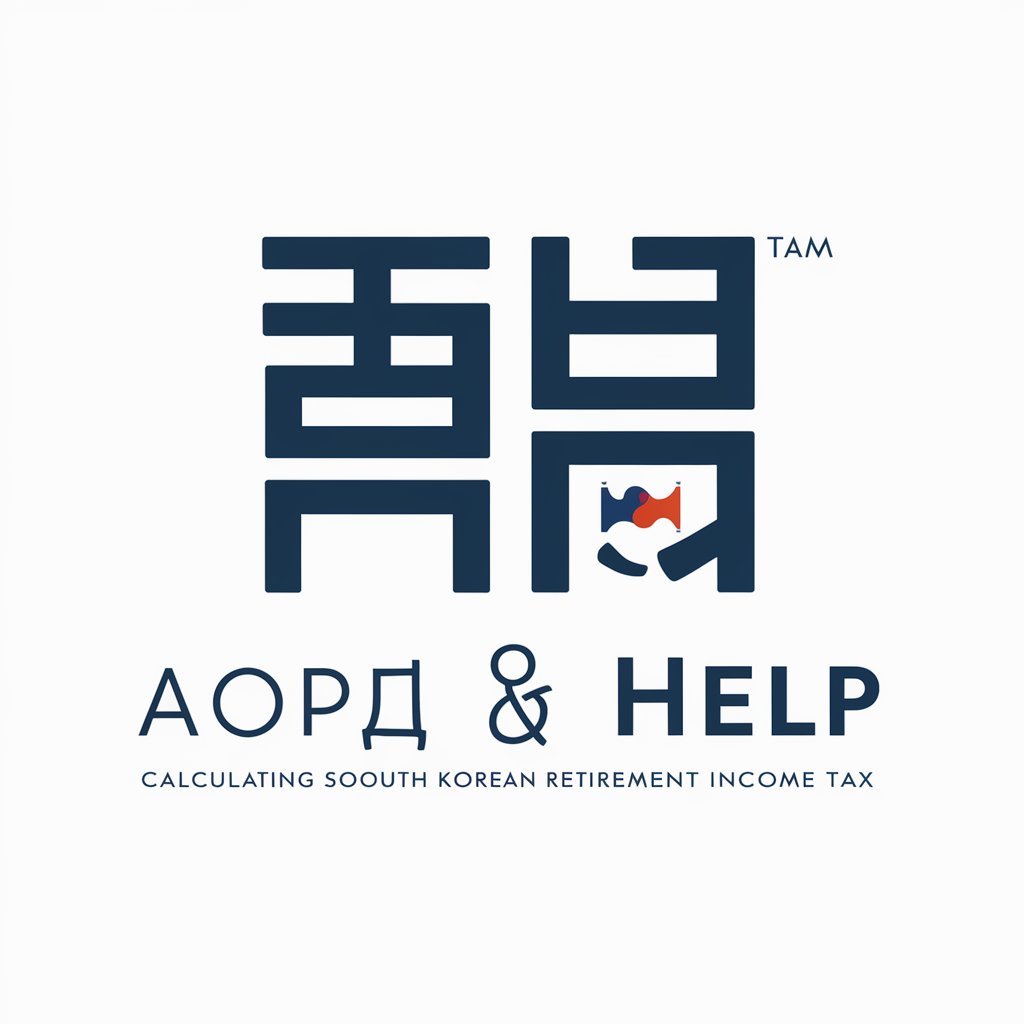
International Tax Advisor GPT España Argentina
Navigate Argentine-Spanish tax laws with AI
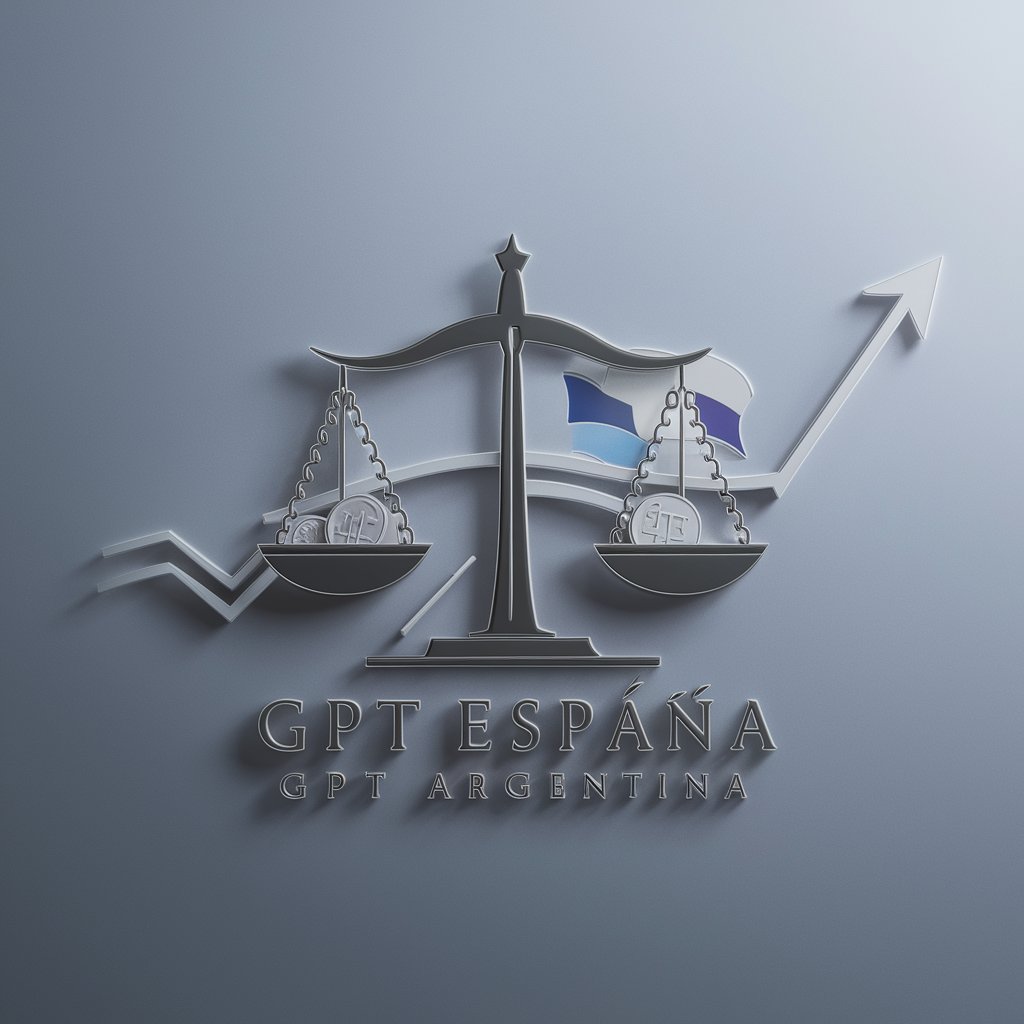
Experto redactor de ensayos académicos APA
AI-powered APA academic essay mastery
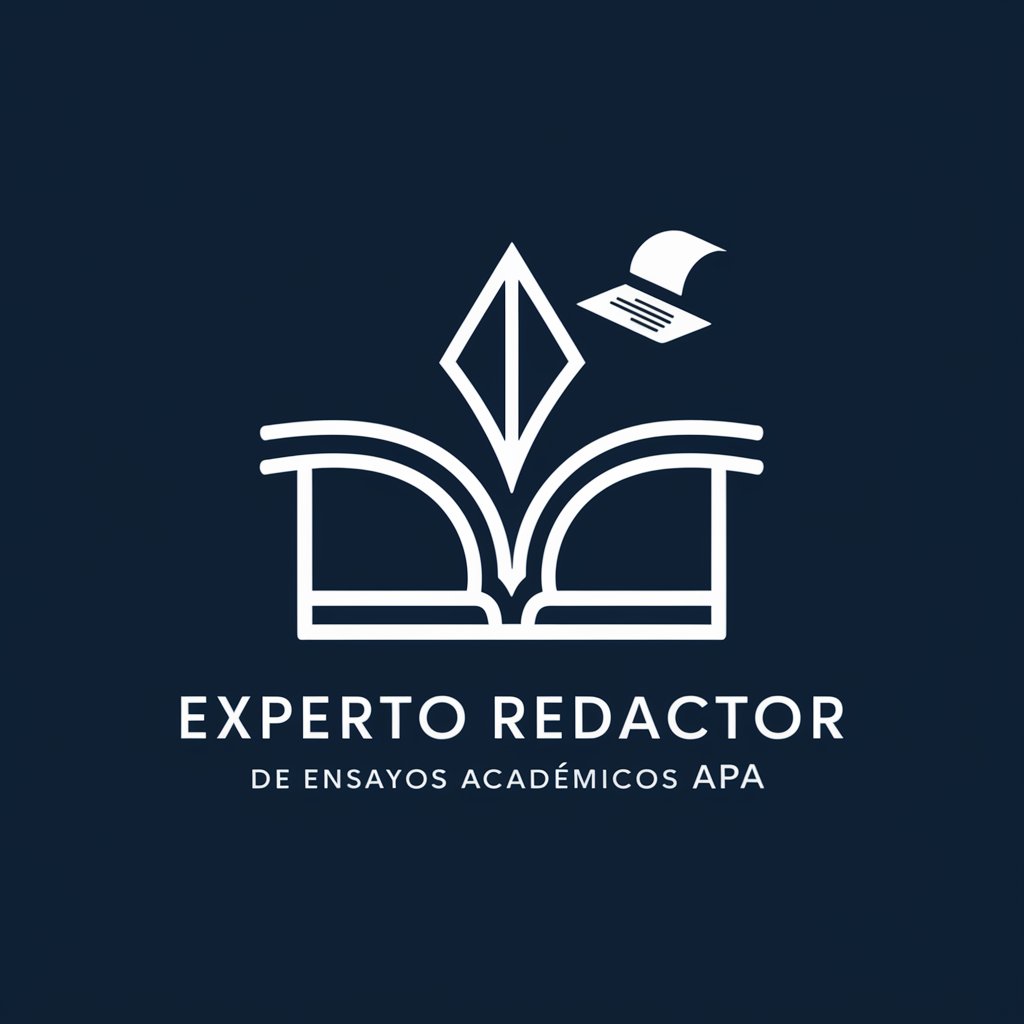
Greenhouse Advisor
Empowering Greenhouses with AI

Personal Branding Rockstar - EN
Elevate Your Professional Brand with AI
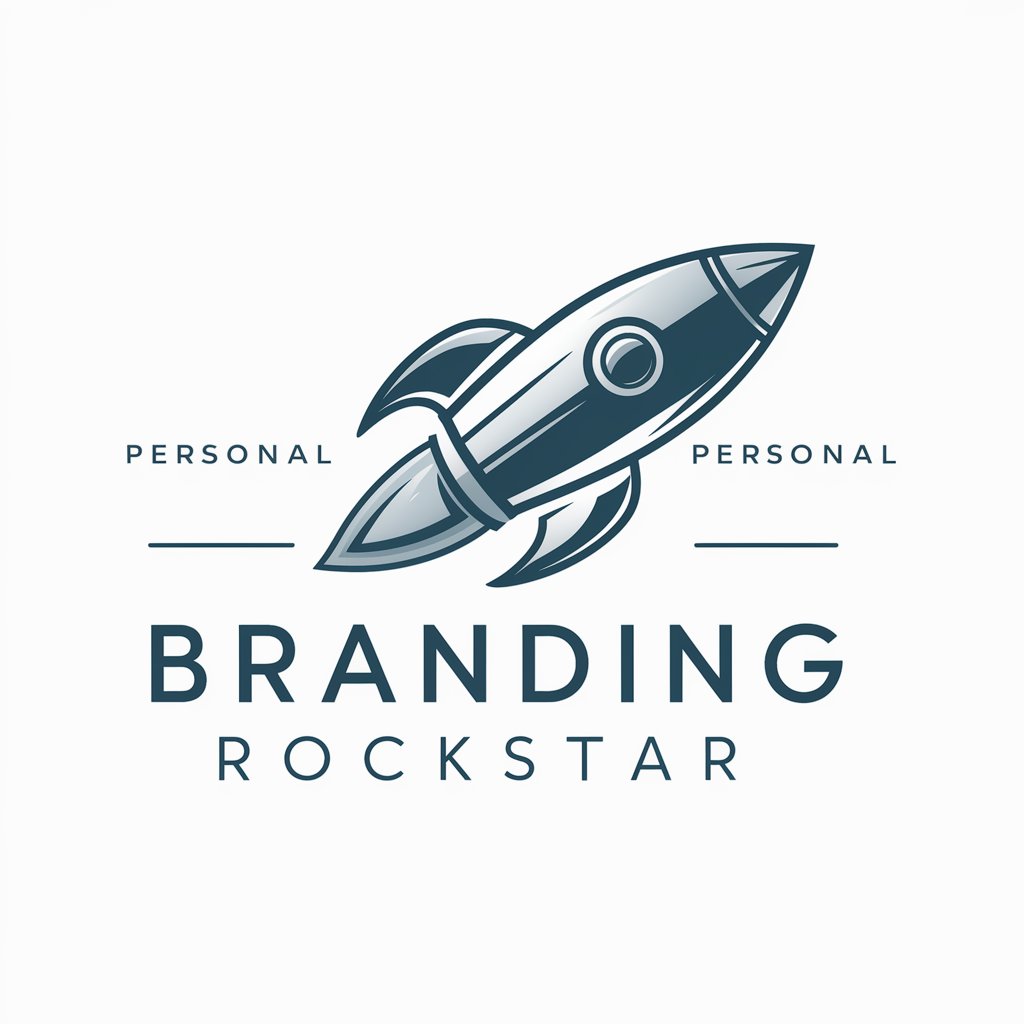
TEST
Empowering your learning with AI

Q&A on Statistical Model for Fantasy Football
What is ridge regression and why is it used in this model?
Ridge regression is a technique used to analyze multiple regression data that suffer from multicollinearity. In this model, it helps to predict NFL fantasy points by stabilizing the predictions when variables are highly correlated, providing more reliable estimates.
How does the model account for different player positions?
The model includes position-specific variables to account for the varying impact of game conditions on different player positions. This allows for more accurate predictions of fantasy points for quarterbacks, running backs, wide receivers, and tight ends.
Can this model predict the performance of defensive players?
While the primary focus of the model is on offensive player positions, it incorporates the quality of defenses as a variable. This indirectly affects predictions but does not directly predict fantasy points for individual defensive players.
How do game location and playing surface affect predictions?
The model considers game location (home or away) and playing surface (grass or artificial) as factors that can influence player performance. These conditions are integrated into the model to refine fantasy point predictions.
Is this model suitable for all fantasy football leagues?
The model is designed with a broad applicability in mind, but its accuracy and relevance might vary across different fantasy football league settings. Users should adjust the model's input based on their league's scoring system and player roster rules.