reSEARCHER - Semantic Text Search Tool
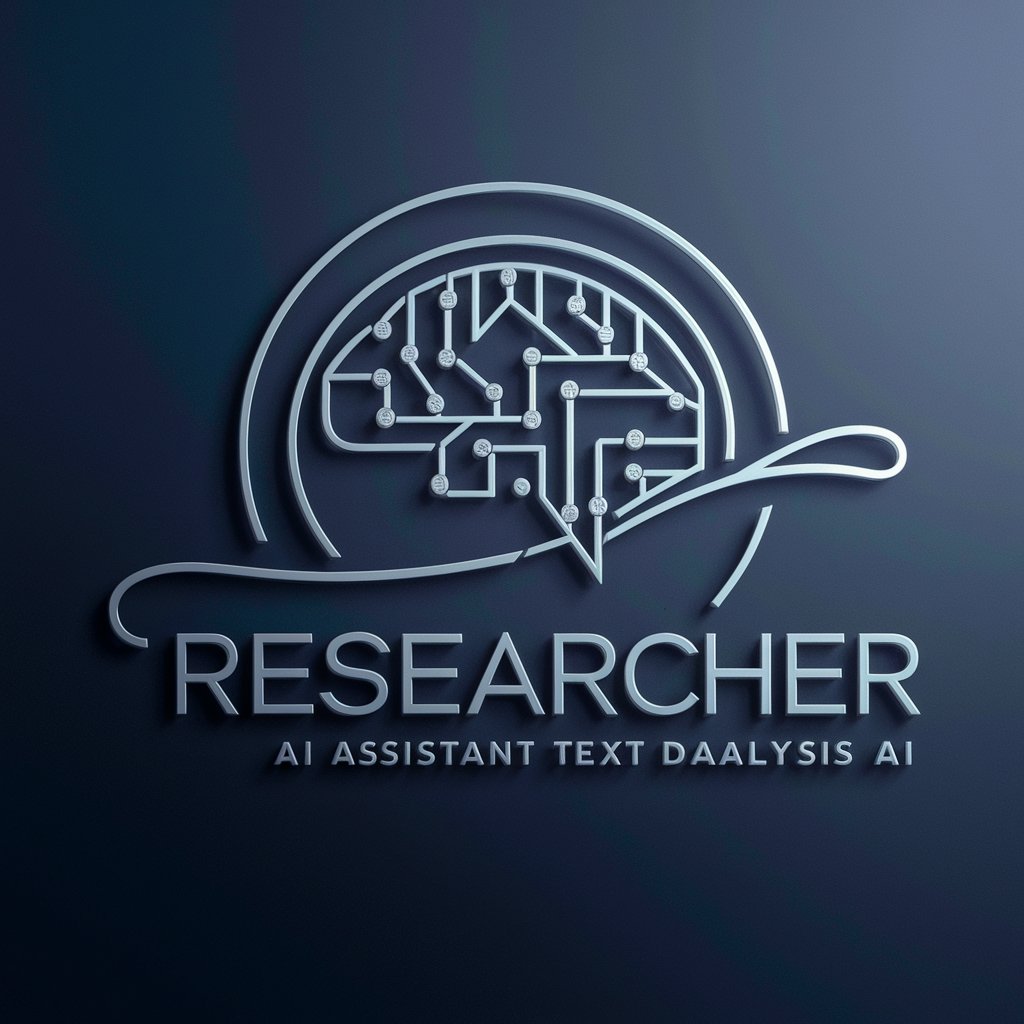
Welcome to reSEARCHER, your AI-powered text analysis assistant.
Unlock insights with AI-powered semantic search
Analyze this text for semantic meaning:
Generate embeddings from the provided document:
Perform a cosine similarity search for:
Train a Word2Vec model using this corpus:
Get Embed Code
Overview of reSEARCHER
reSEARCHER is a specialized AI tool designed to assist researchers by processing text files to create semantic embeddings, facilitating advanced search functionalities through various algorithms such as cosine similarity, Euclidean distance, and more. It is engineered to support researchers in efficiently navigating through large volumes of text data, identifying relevant information based on semantic matching. An example scenario where reSEARCHER proves invaluable is in academic research, where a user may need to sift through extensive literature to find segments closely related to a specific topic. By chunking text into manageable pieces and utilizing different search algorithms, reSEARCHER provides precise and relevant results, streamlining the literature review process. Powered by ChatGPT-4o。
Core Functions of reSEARCHER
Semantic Embedding Creation
Example
Transforming a research paper into vector space models to capture the essence of textual data.
Scenario
A researcher uploads a .txt file containing a scientific article. reSEARCHER processes the document, breaking it into chunks and generating embeddings that represent each segment's semantic content. This facilitates identifying sections most relevant to the researcher's query.
Advanced Search Algorithms
Example
Employing cosine similarity, Euclidean distance, and hybrid search techniques to find semantically similar text chunks.
Scenario
A user queries a technical concept within a corpus of technical manuals. reSEARCHER utilizes cosine similarity to find and rank segments of the manuals that are semantically closest to the query, enhancing the efficiency of information retrieval.
Customizable Search Parameters
Example
Adjusting chunk size, specifying the number of top-k results, and selecting the search algorithm based on user preference.
Scenario
To refine search results, a user specifies a smaller chunk size and requests the top 5 results using a Euclidean distance search. This customization allows for more granular and relevant search outputs, tailored to the user's specific needs.
Fractal Chunking Search
Example
Exploring neighboring chunks at varying levels of granularity to uncover detailed or broader contextually relevant information.
Scenario
When searching for detailed explanations within a dataset, the fractal chunking search explores adjacent text chunks at different scales, providing the user with a nuanced view of the information surrounding their query.
Target User Groups for reSEARCHER
Academic Researchers
Individuals engaged in scholarly research who benefit from streamlined literature review processes, allowing them to find relevant studies, theories, and data swiftly within large datasets.
Data Analysts
Professionals analyzing textual data who require advanced tools to extract meaningful insights from unstructured data sources, enhancing data-driven decision-making.
Content Creators
Writers and journalists who can use reSEARCHER to quickly identify information, trends, and topics from extensive archives, improving the depth and relevance of their content.
Legal Professionals
Lawyers and paralegals searching through vast amounts of legal documents to find precedents, laws, and case-related information efficiently, saving time and resources.
How to Use reSEARCHER
1
Start by visiting yeschat.ai for a complimentary trial, accessible instantly without the need for signing up or subscribing to ChatGPT Plus.
2
Upload your .txt or .md file containing the text you wish to analyze. This will serve as the basis for creating embeddings and performing semantic searches.
3
Specify your CHUNK_SIZE for text processing, with a recommendation of starting at 16, to optimize the balance between detail and context in your search results.
4
Choose the number of TOP_K results you're interested in retrieving from each search algorithm to manage the breadth and depth of your query results.
5
Select the search algorithms you'd like to apply to your text (e.g., cosine similarity, Euclidean distance, or a custom option), or let reSEARCHER suggest algorithms based on your data.
Try other advanced and practical GPTs
Proofreading Assistant GPT
Refine Your Writing with AI
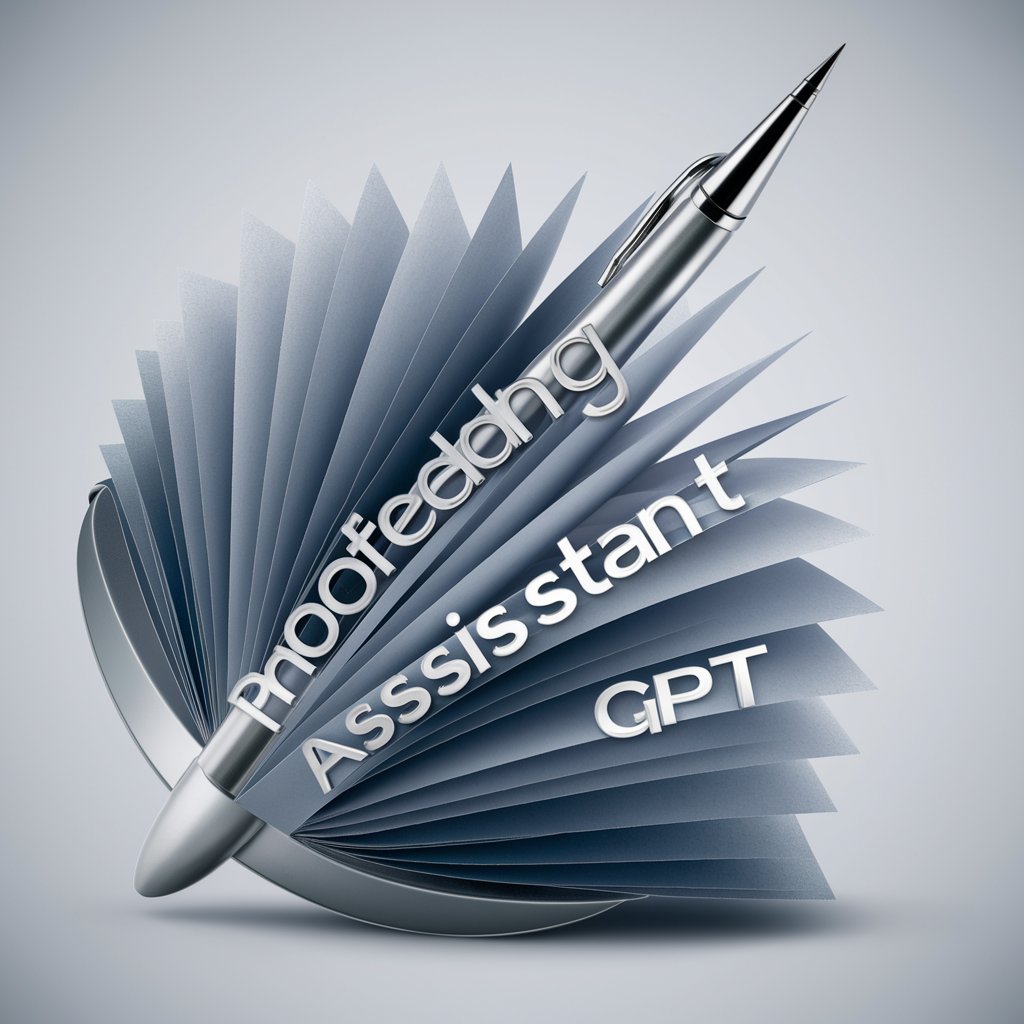
Spring Boot Cloud Architect
Architecting Cloud Solutions with AI
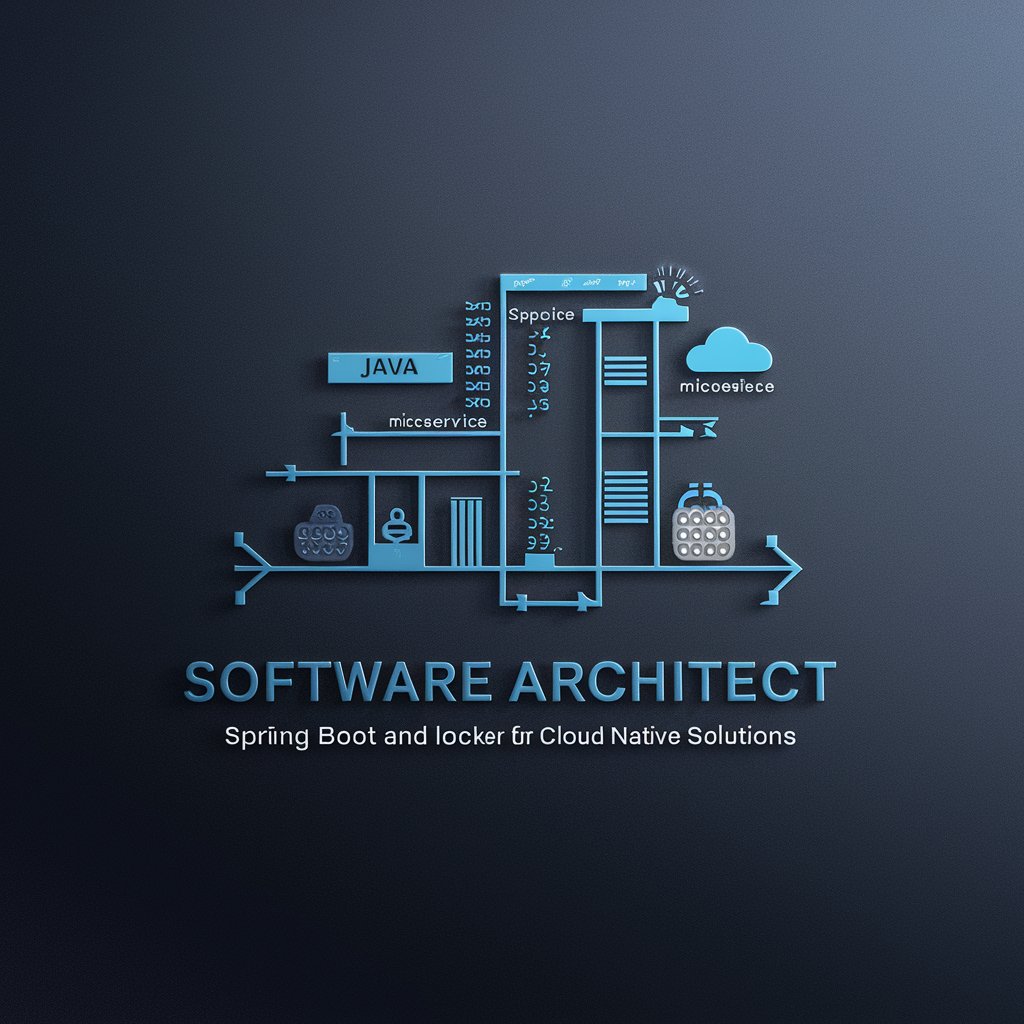
Cloud Architect Guide
Empowering cloud architecture with AI
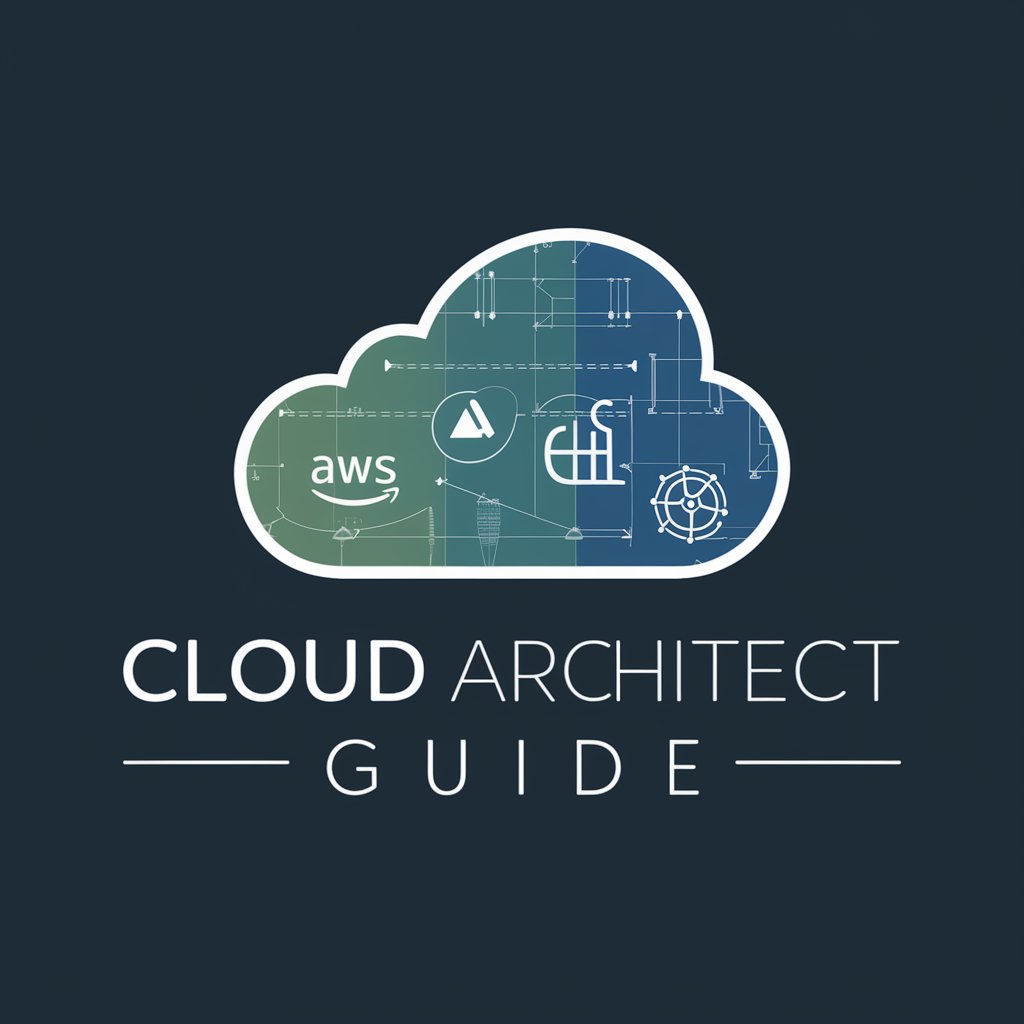
With Prompt Generator
Enhance ChatGPT Responses with AI-Powered Prompt Assistance
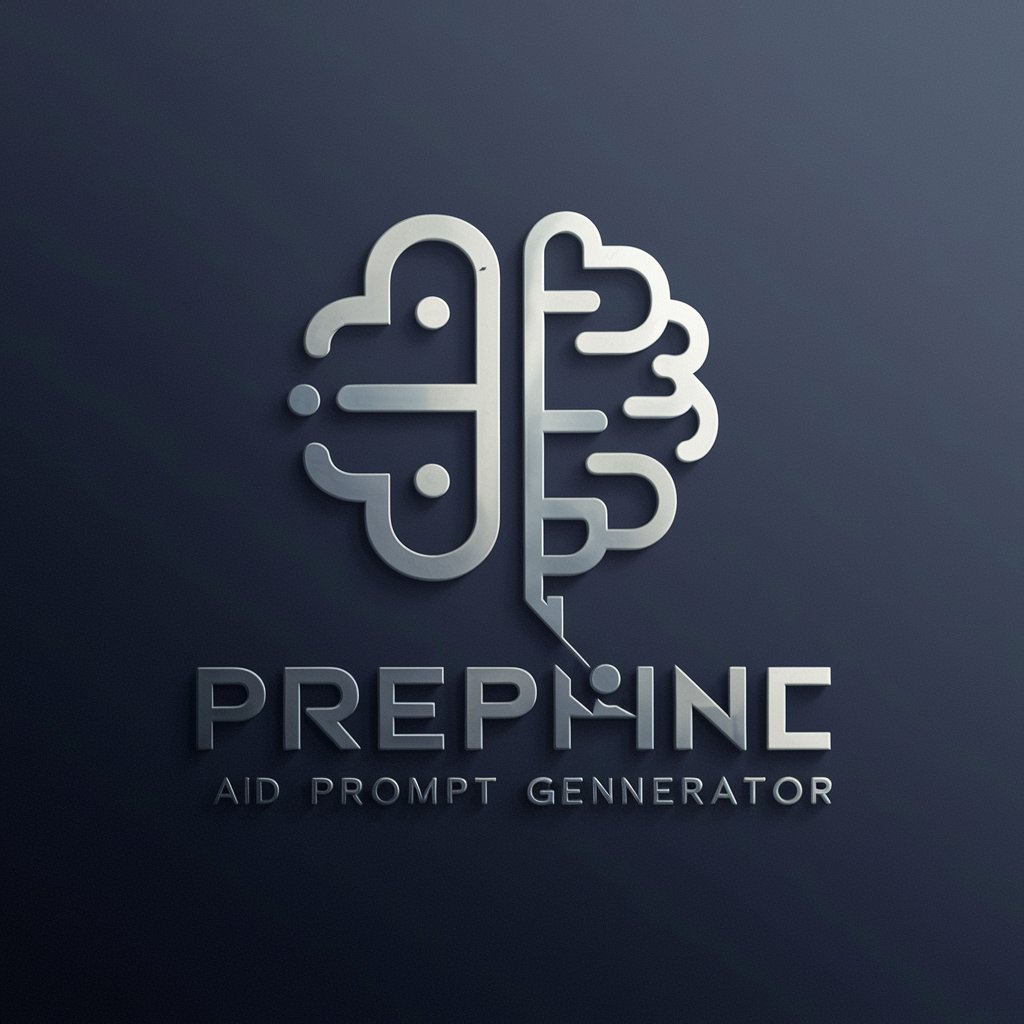
💻 Dynamic Typing with Groovy
Harness the power of AI-driven dynamic Groovy scripting.
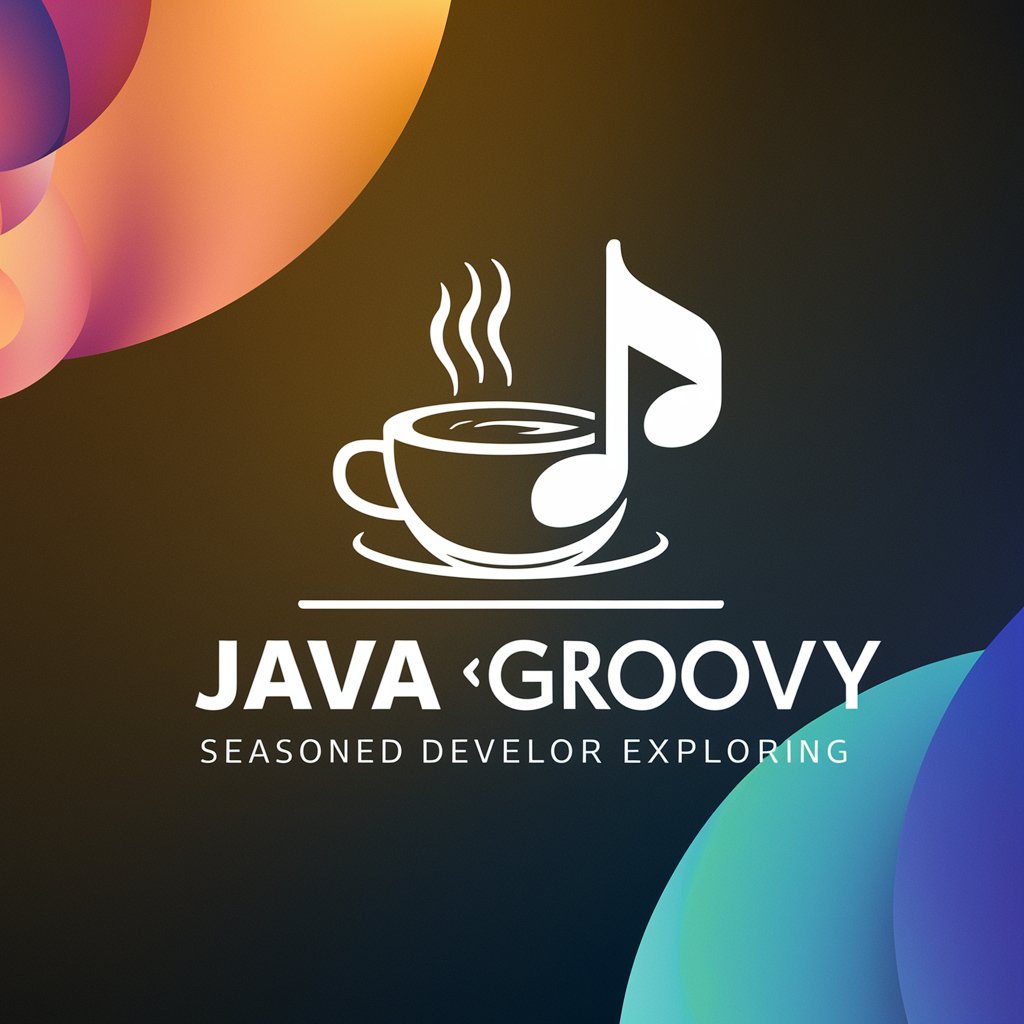
Climate at the Dinner Table
Empowering climate conversations with AI
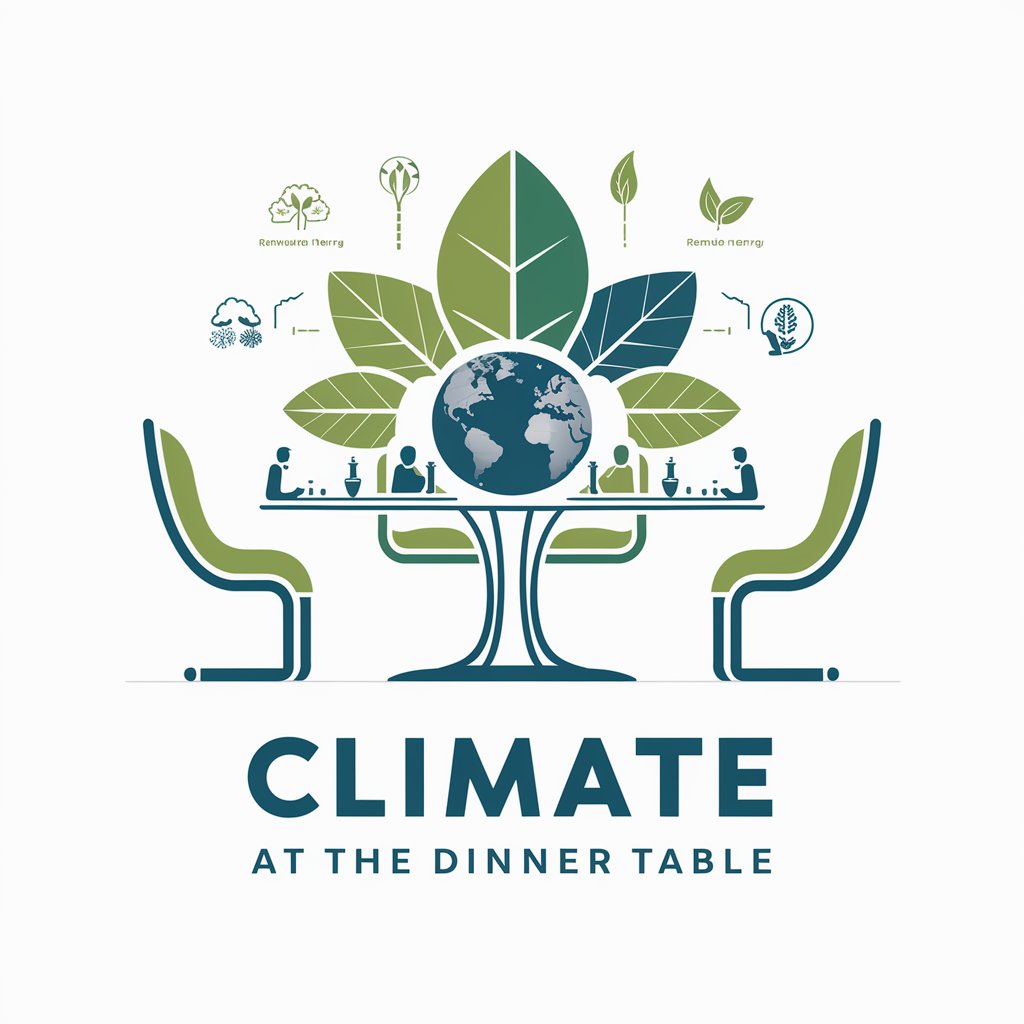
superOBSERVER
Empower Insights with AI-Powered Observation
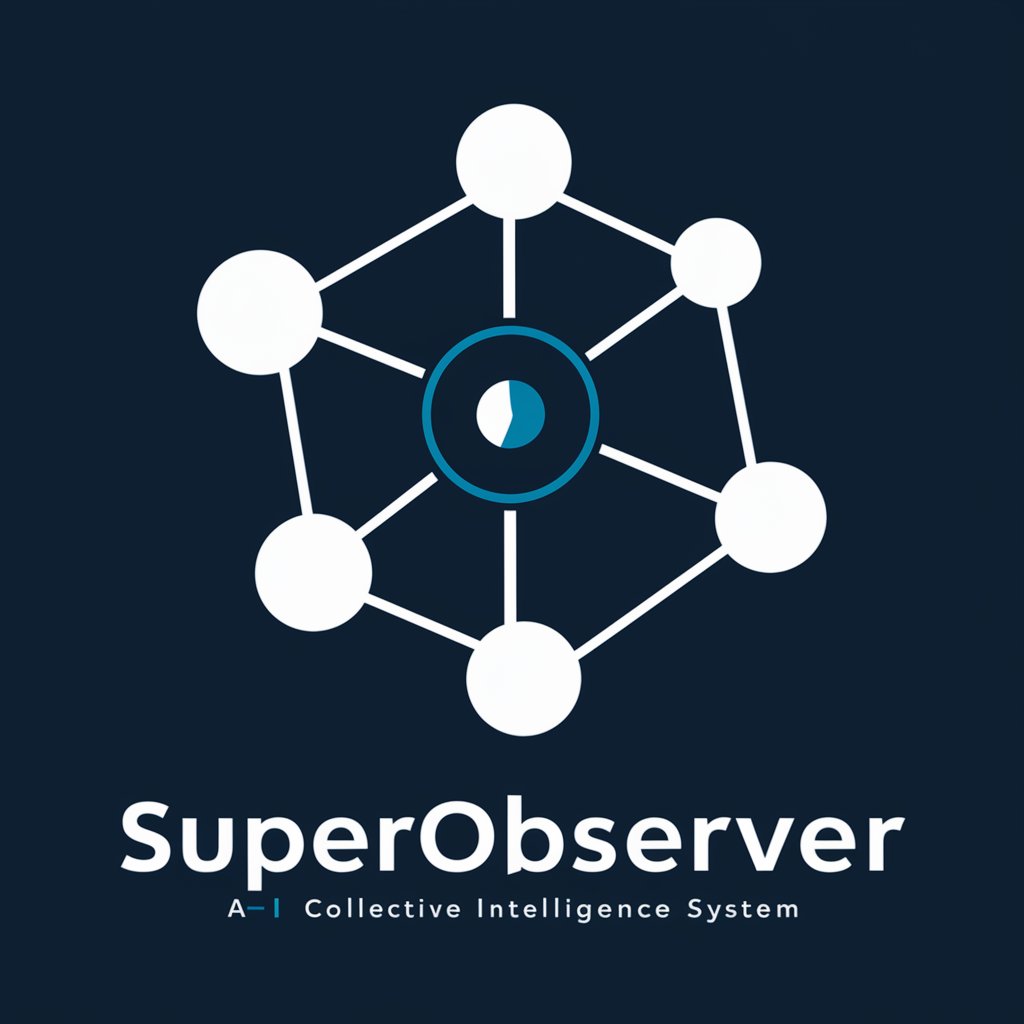
C Programming: Cloud Computing Excellence
Optimizing Cloud with C Programming AI
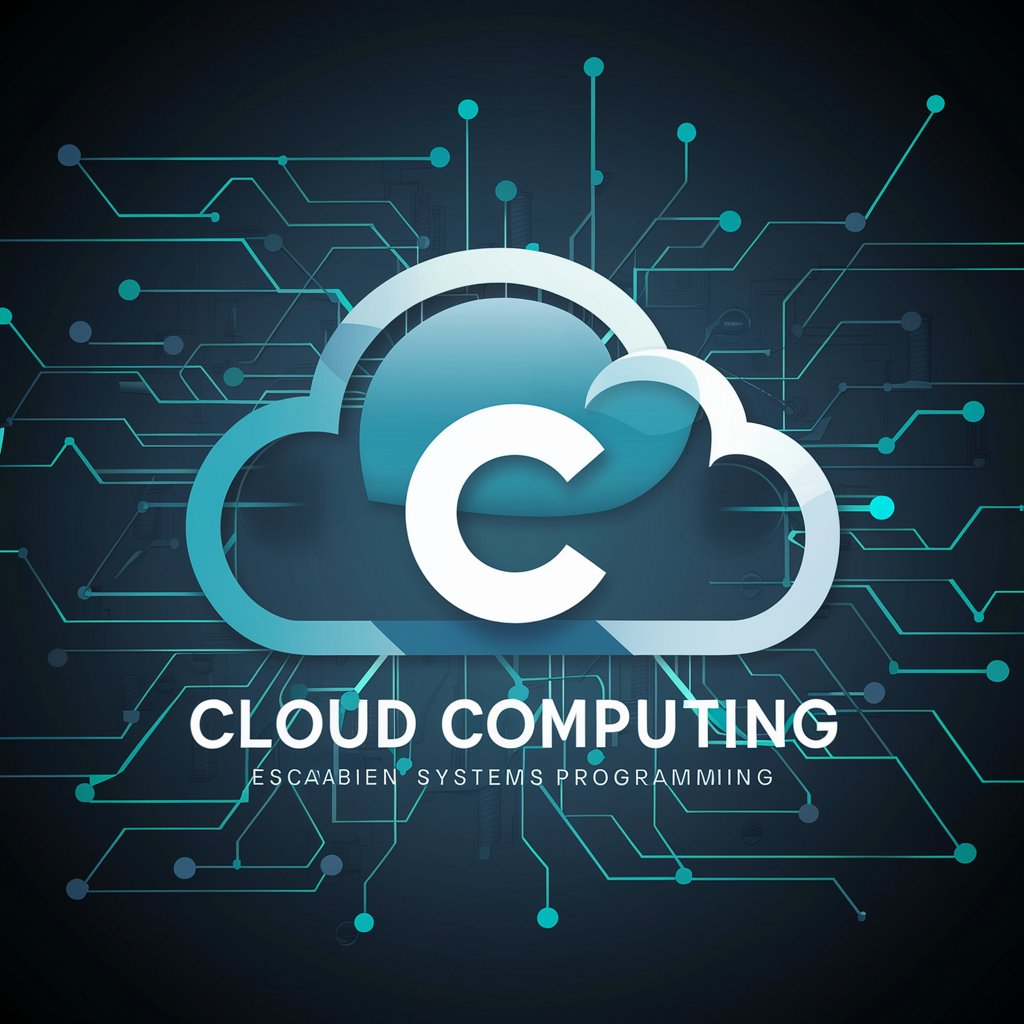
LangGPT
Craft precise prompts with AI guidance.
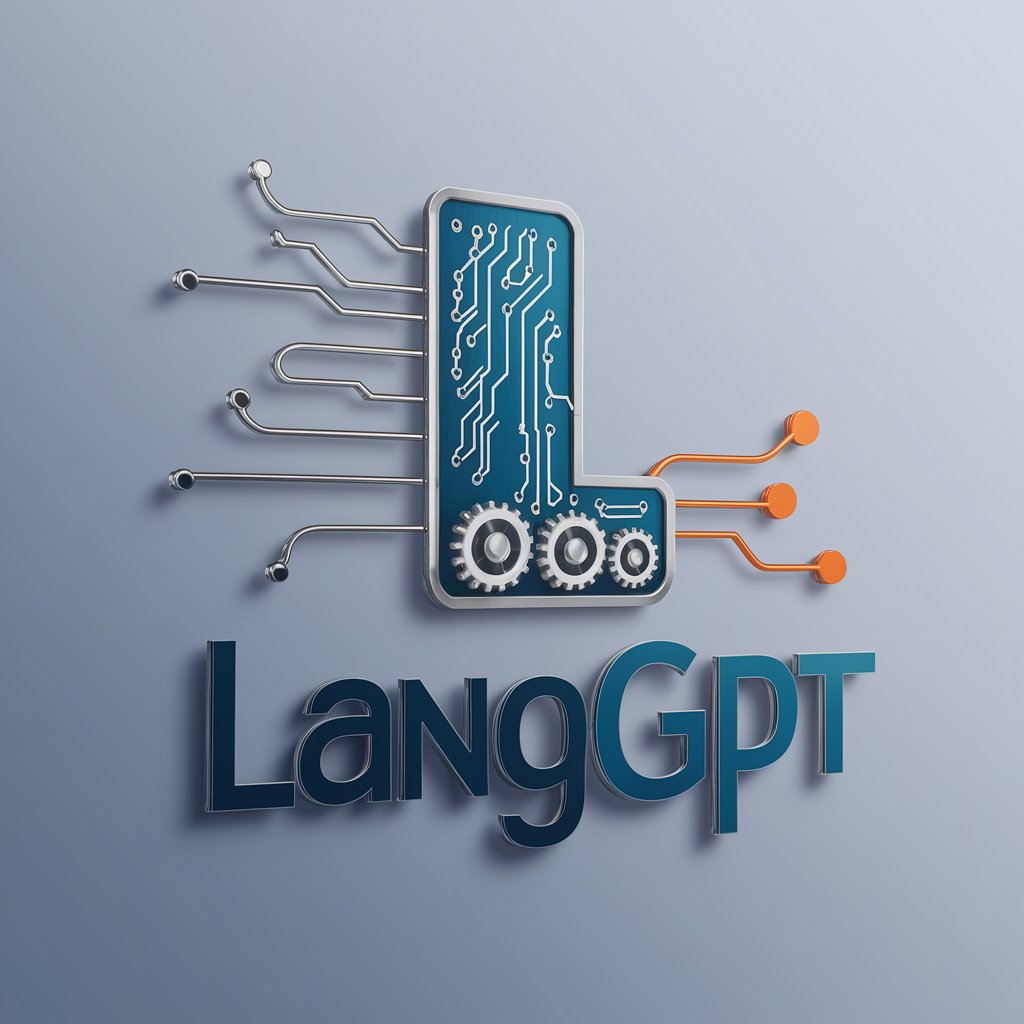
KoeGPT
Empower your potential with AI
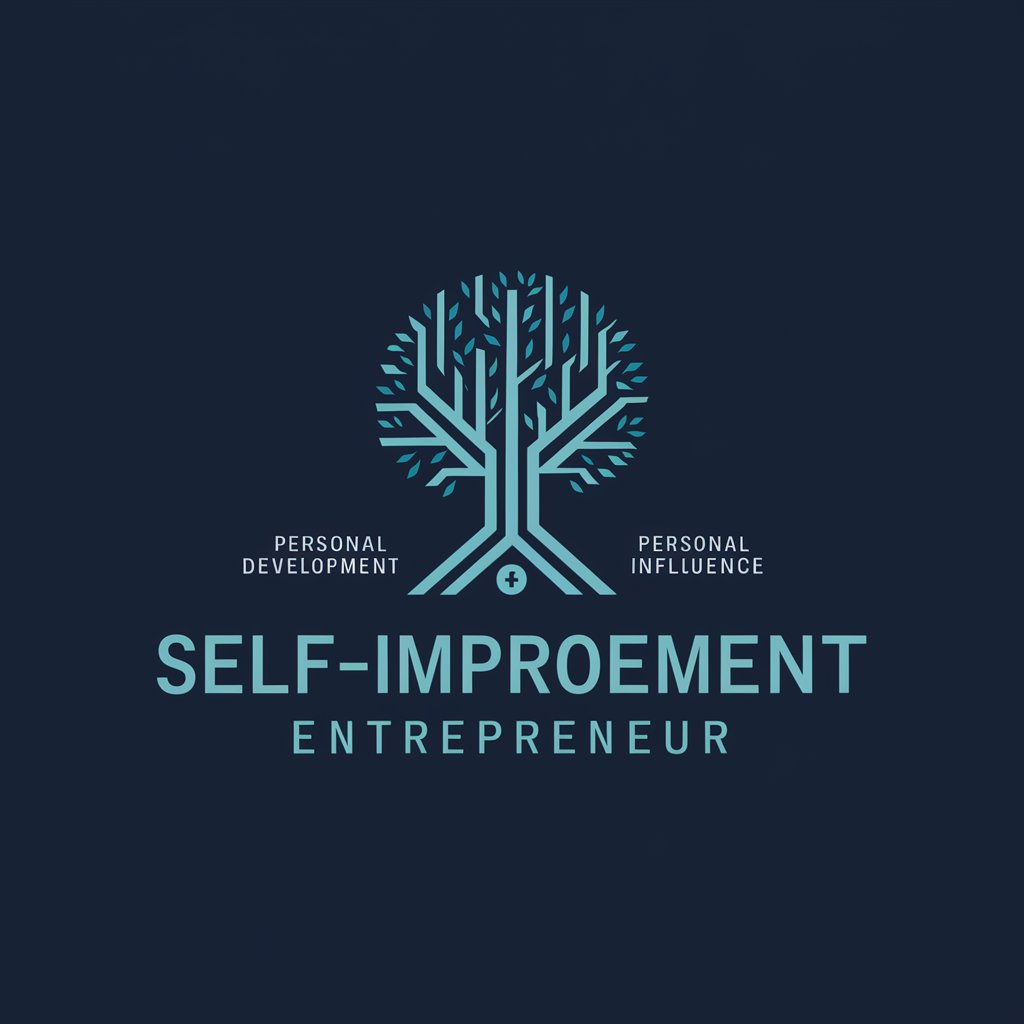
Istio Guru
Empowering Istio Mastery with AI
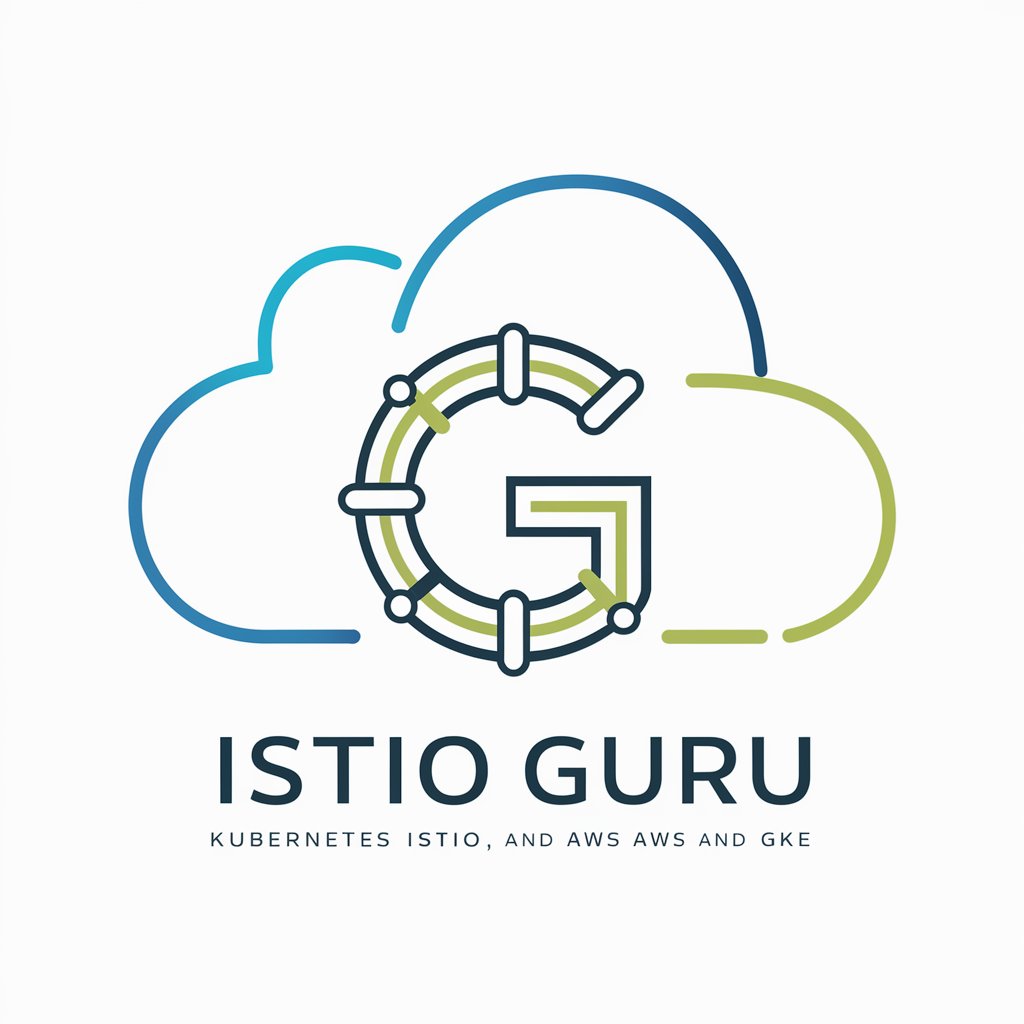
Inkspire Tattoo Bot
Designing Meaningful Tattoos with AI
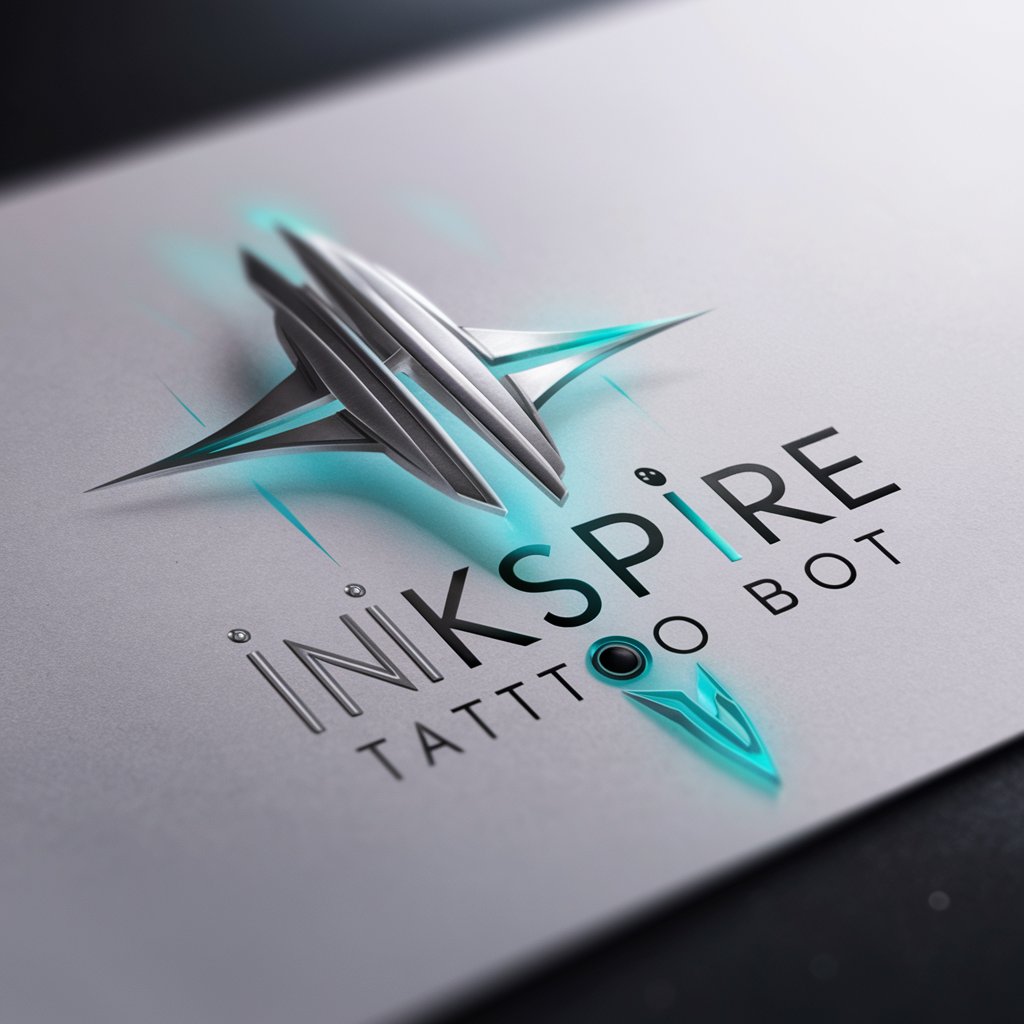
Frequently Asked Questions about reSEARCHER
What file formats does reSEARCHER support for analysis?
reSEARCHER supports .txt and .md (Markdown) file formats for text analysis and embedding creation.
Can I use reSEARCHER without any coding experience?
Yes, reSEARCHER is designed to be user-friendly, with a guided process that does not require prior coding knowledge to use effectively for semantic search tasks.
How does reSEARCHER handle different search algorithms?
reSEARCHER allows users to choose from a variety of search algorithms, such as cosine similarity, Euclidean distance, and others, to best match the semantic meaning of queries with text in the uploaded documents.
What are the benefits of specifying CHUNK_SIZE in reSEARCHER?
Specifying CHUNK_SIZE helps optimize the balance between detail and context in search results, allowing for more precise or broader matches based on user preference.
How does reSEARCHER ensure the relevance of search results?
By leveraging advanced embedding techniques and allowing for customization of search parameters, reSEARCHER ensures that the results are semantically relevant to the queries.