SPR Compressor - Advanced Language Model Activation
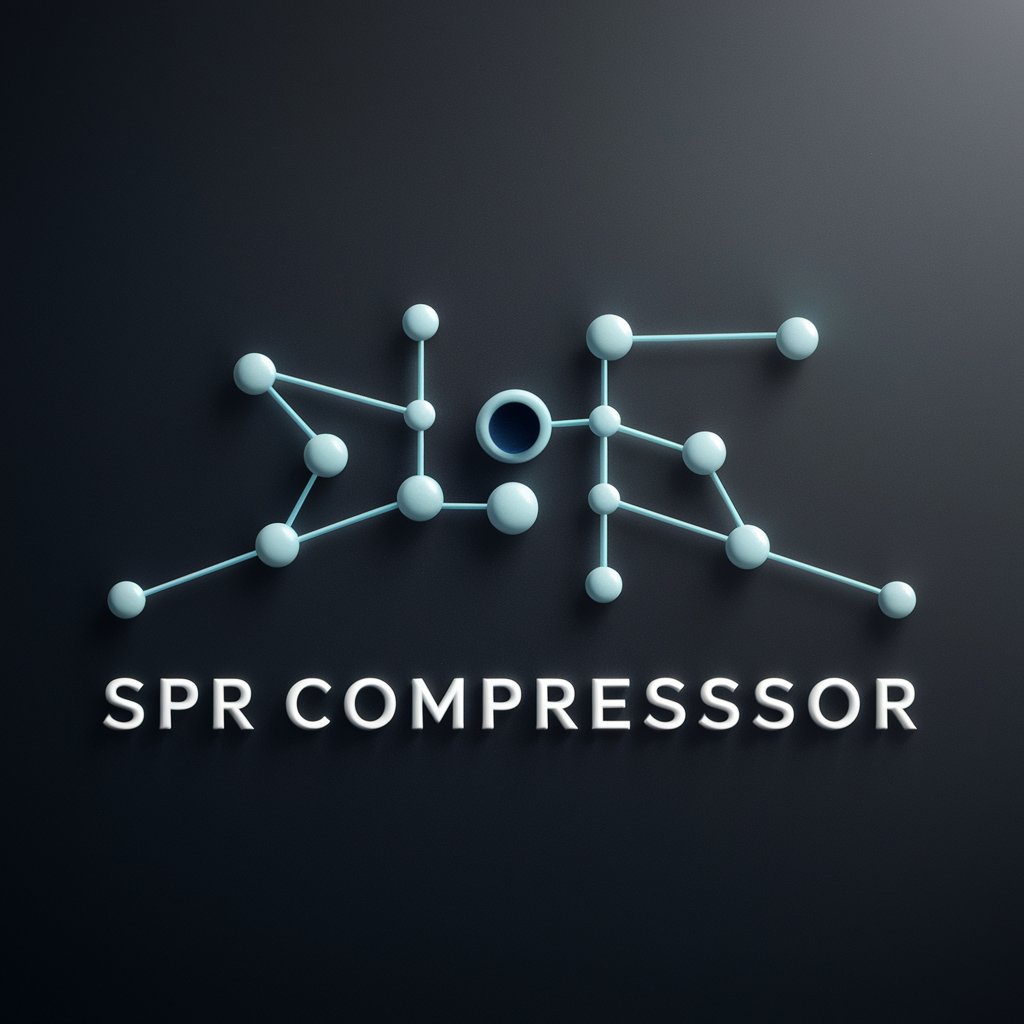
Welcome to SPR Compressor, your advanced AI tool for precision priming.
Revolutionizing Language Processing with AI
Generate a concise summary of...
Describe the key concepts in...
Explain the significance of...
Outline the main points of...
Get Embed Code
Understanding SPR Compressor
SPR Compressor, short for Sparse Priming Representation Compressor, is designed to optimize language model interactions through efficient, condensed priming inputs. It translates detailed information into a minimal, concept-rich format, activating the latent space of language models to align with specific informational or task-oriented goals. For example, transforming a complex set of instructions for data analysis into a series of concise, easily interpretable commands for a language model, enhancing both the model's performance and the efficiency of user interactions. Powered by ChatGPT-4o。
Core Functions and Real-World Applications
Condensed Knowledge Activation
Example
Inputting a compact summary of recent climate change research findings to generate an informed, nuanced essay.
Scenario
A researcher can quickly prime an LLM to draft an article that reflects the latest advancements in climate science without having to manually input or summarize extensive datasets.
Efficient Task Instruction
Example
Directing an LLM to create a project plan using a brief, high-level overview of objectives and constraints.
Scenario
Project managers can streamline the development of detailed project plans by providing succinct, targeted primers, thereby saving time and focusing on strategic decision-making.
Enhanced Creative Output
Example
Priming an LLM with a sparse representation of a story's theme, setting, and character archetypes to generate a novel.
Scenario
Writers can leverage SPR Compressor to quickly draft stories or scripts, focusing on creative direction rather than the minutiae of plot construction.
Target User Groups for SPR Compressor Services
Researchers and Academics
This group benefits from being able to condense complex theories, datasets, or research findings into streamlined inputs, facilitating the generation of comprehensive, context-aware content for publications, grant proposals, or teaching materials.
Project Managers and Strategists
Professionals in these roles can use SPR Compressor to distill project goals, constraints, and visions into succinct briefs, enabling rapid development of plans, reports, and strategic documents with the help of LLMs.
Writers and Creative Professionals
By providing a framework for condensing narrative elements into core themes, settings, and character dynamics, SPR Compressor aids in the efficient generation of creative content, from novels and scripts to marketing campaigns.
Guidelines for Using SPR Compressor
Initial Access
Visit yeschat.ai for a free trial without login; no ChatGPT Plus required.
Understanding SPR
Familiarize yourself with Sparse Priming Representation; explore its application in NLP tasks.
Experimentation
Use the tool for various inputs, noticing the differences in outputs compared to standard language models.
Advanced Usage
Experiment with complex queries, leveraging SPR's ability to distill and compress nuanced information.
Feedback Loop
Provide feedback on outputs to refine and optimize SPR usage for your specific needs.
Try other advanced and practical GPTs
Write Buddy
Empowering your writing with AI
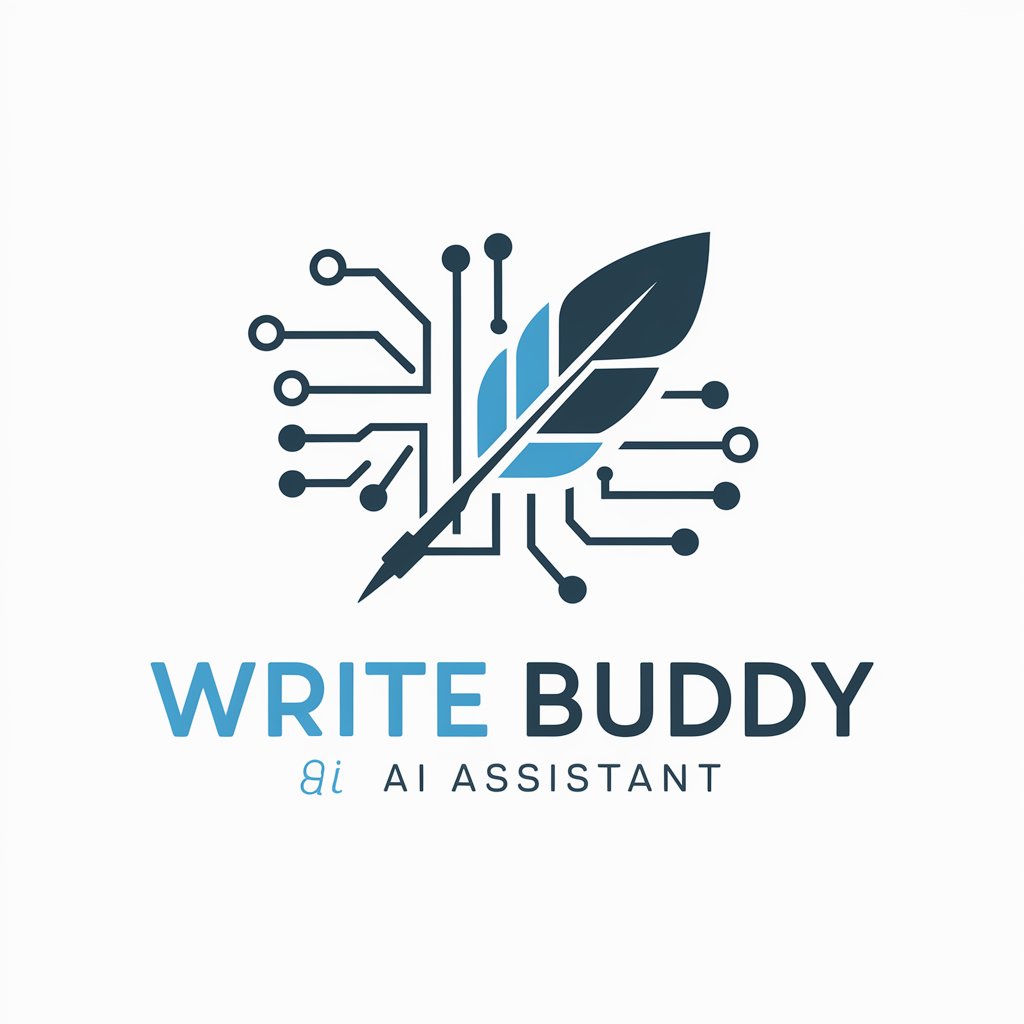
Reign King
Empowering insights with a cultural touch.
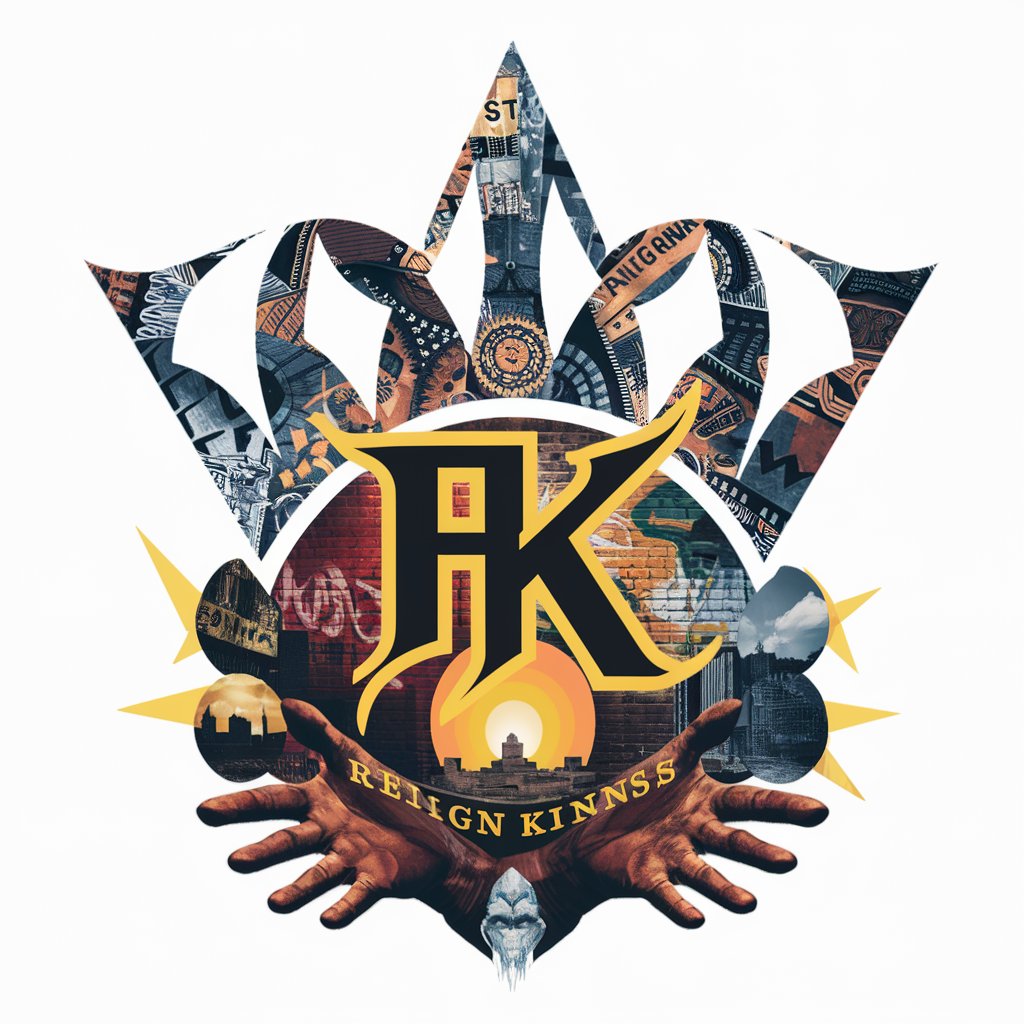
Sassy Strategy
Strategic Brilliance, Powered by AI
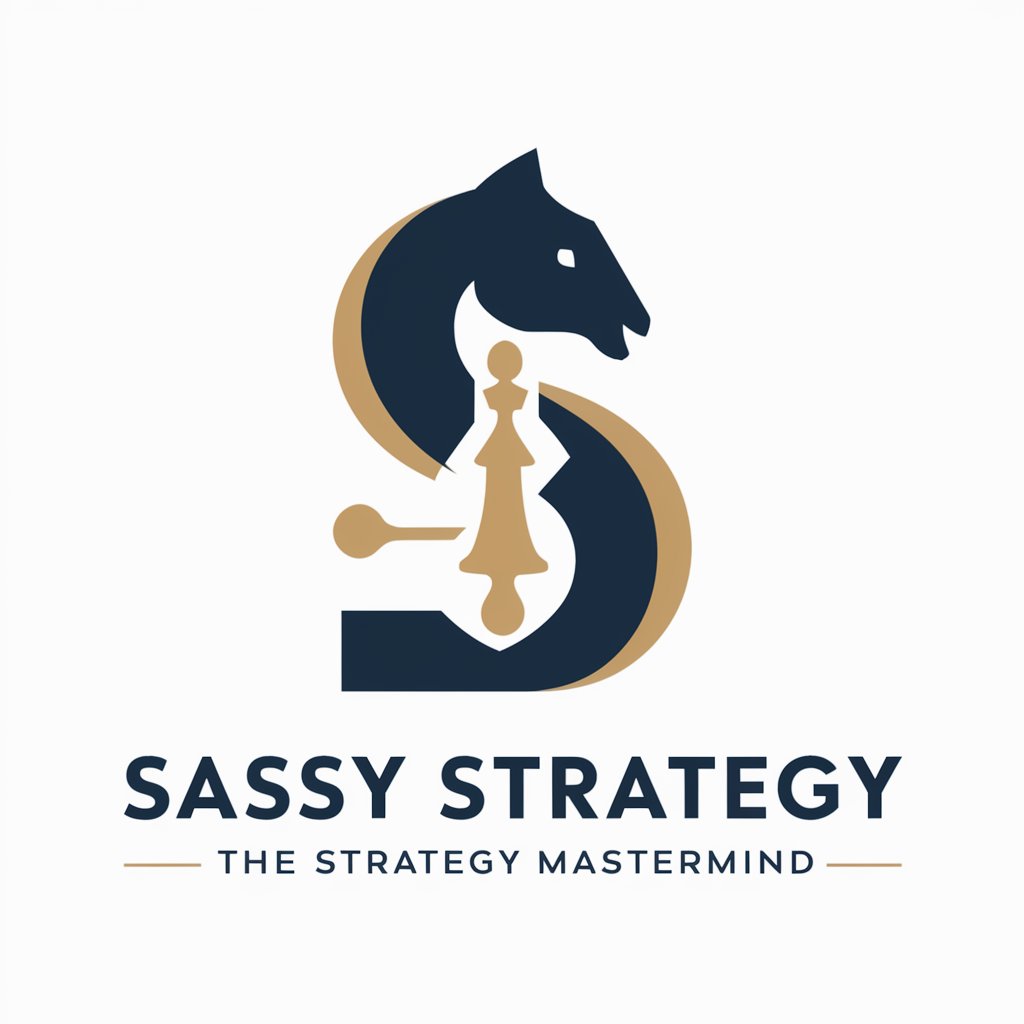
Adaptable Intellect
Tailored Interactions Powered by AI
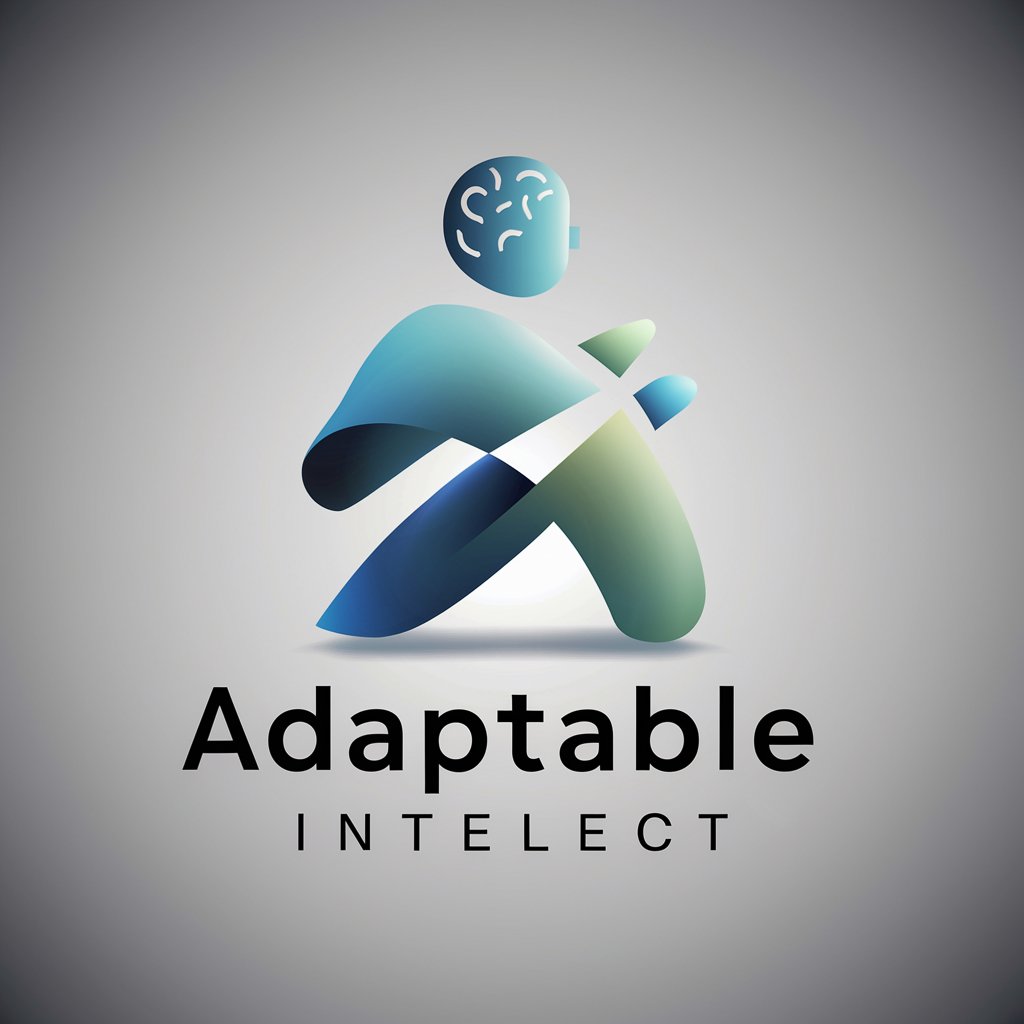
Nonprofit GPT
Empowering nonprofits with AI-driven insights
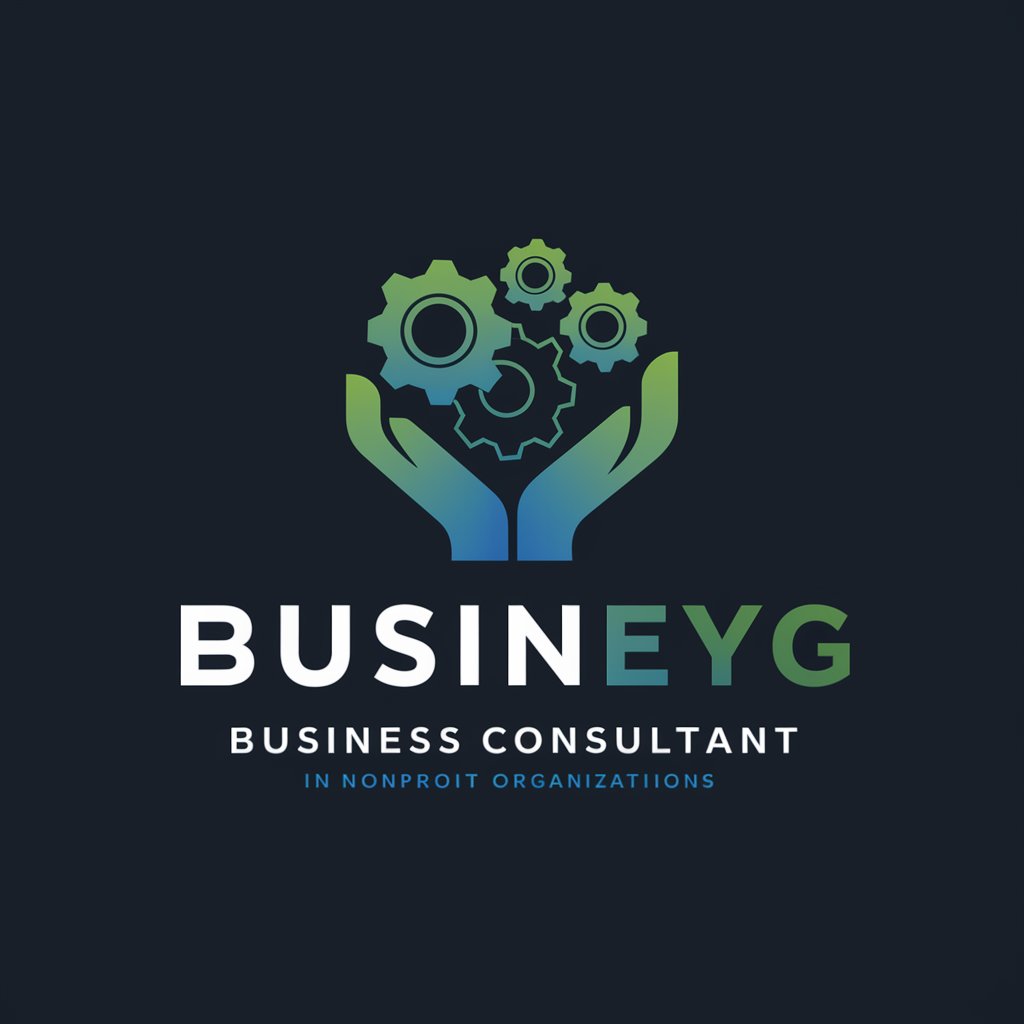
Design Thinking
Empowering innovation with AI
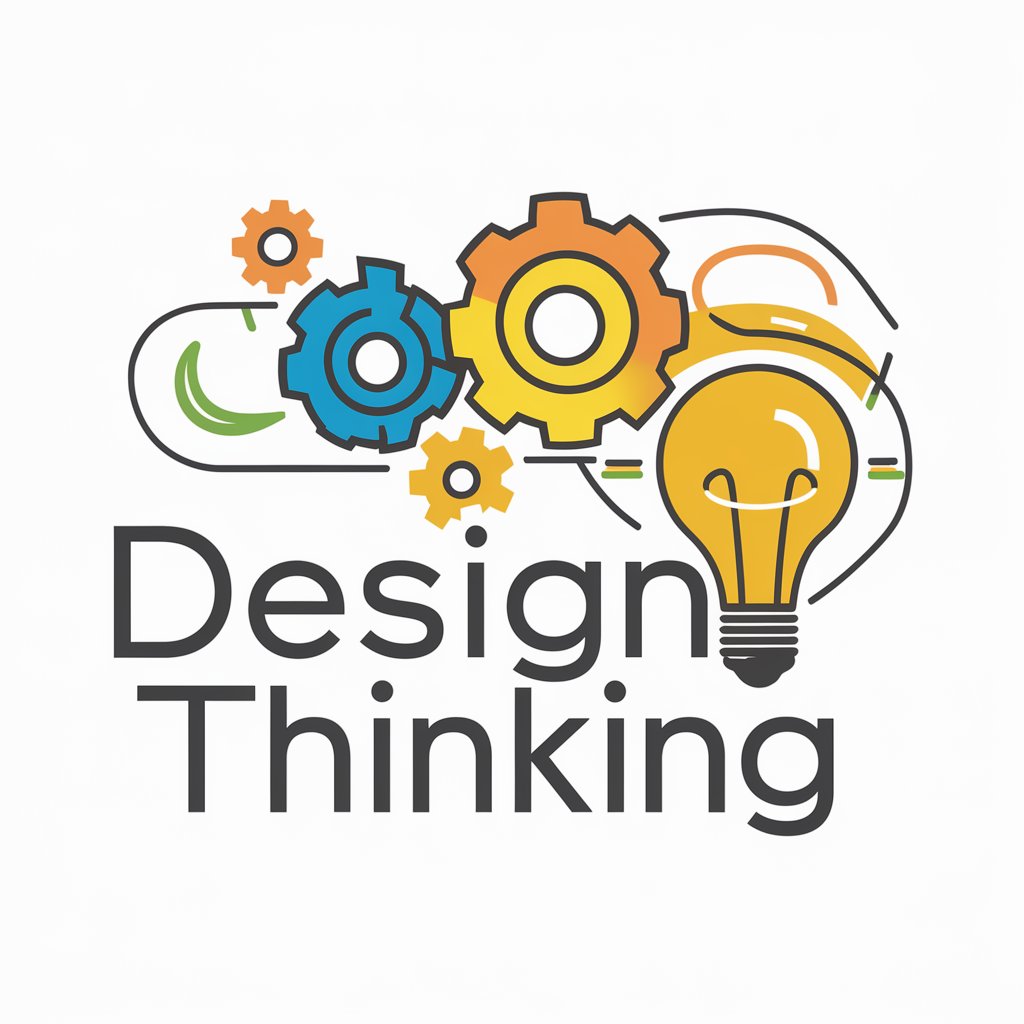
Persona Architect
Craft Your AI Business Companion
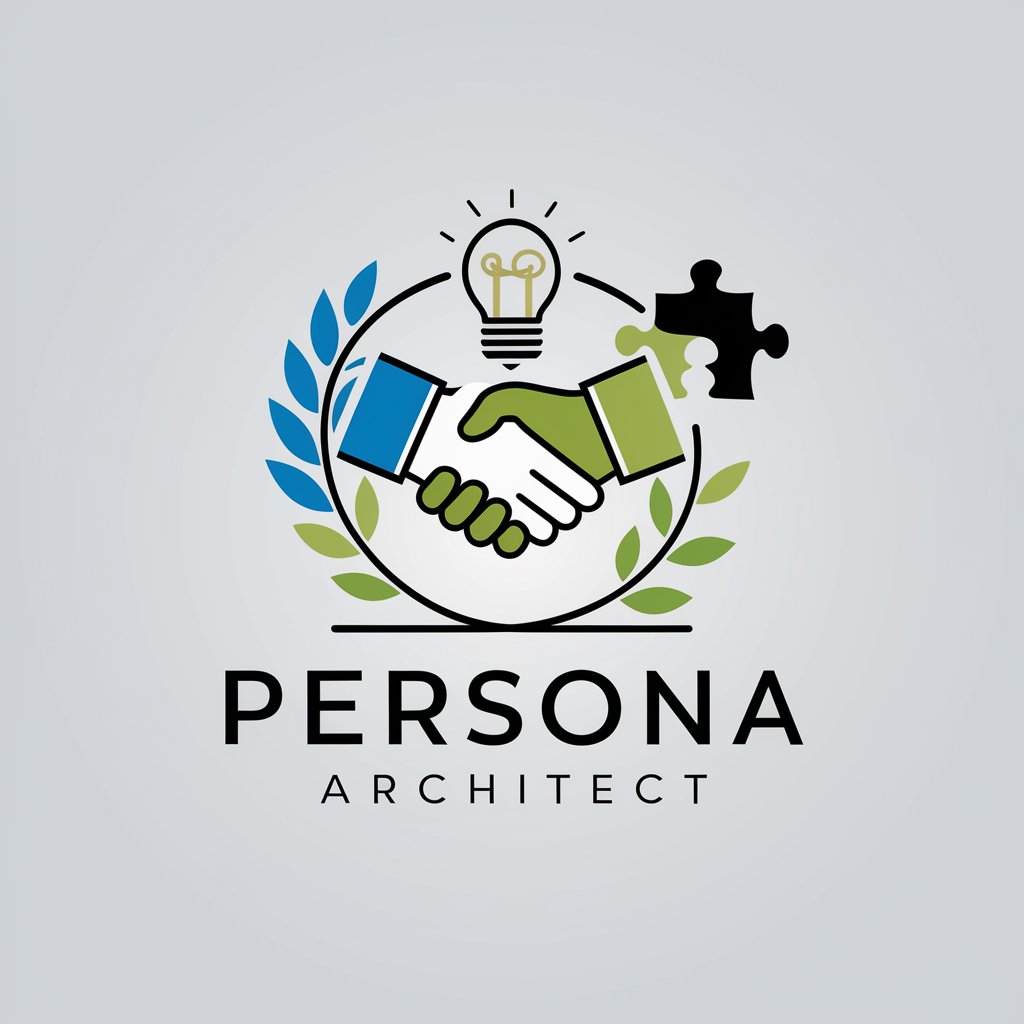
Cinema Sage
Unraveling cinema with AI-powered wit
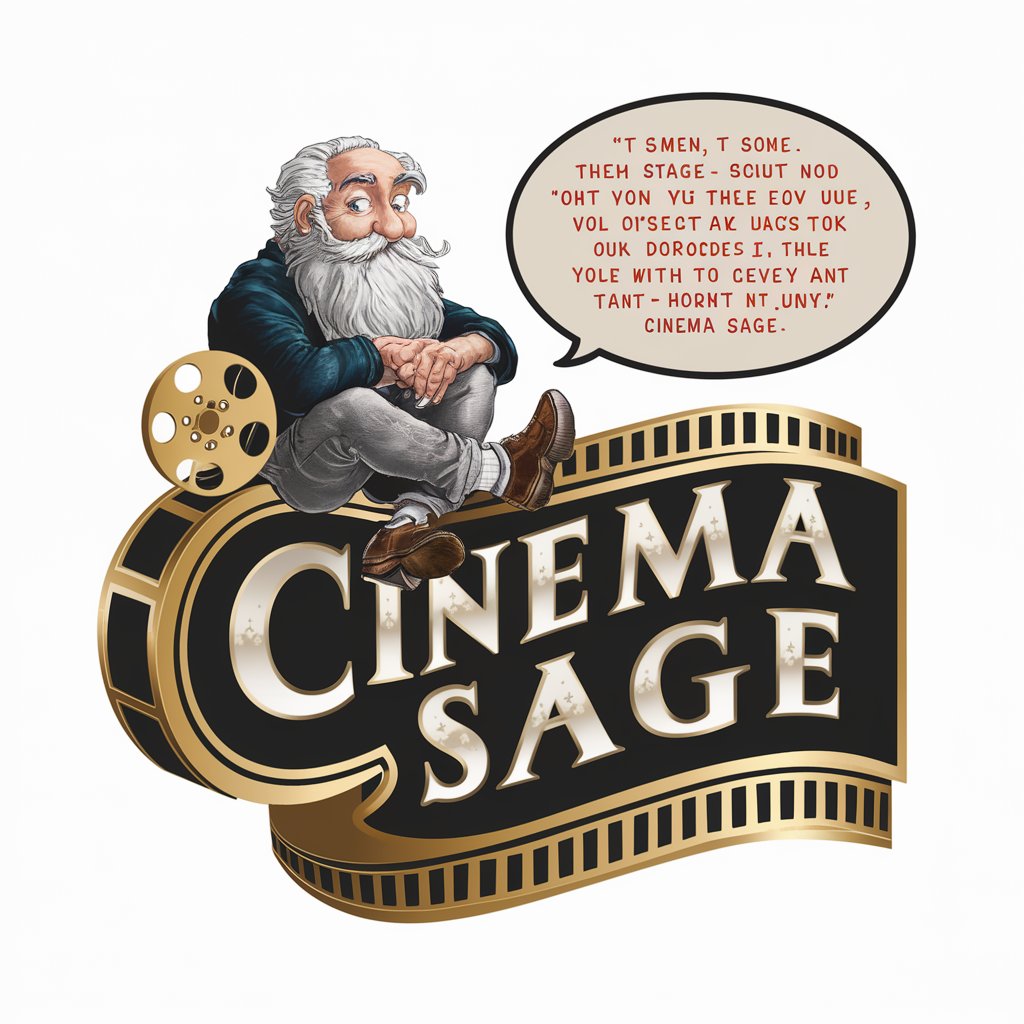
Book Insighter
Empowering Your Reading with AI Insights
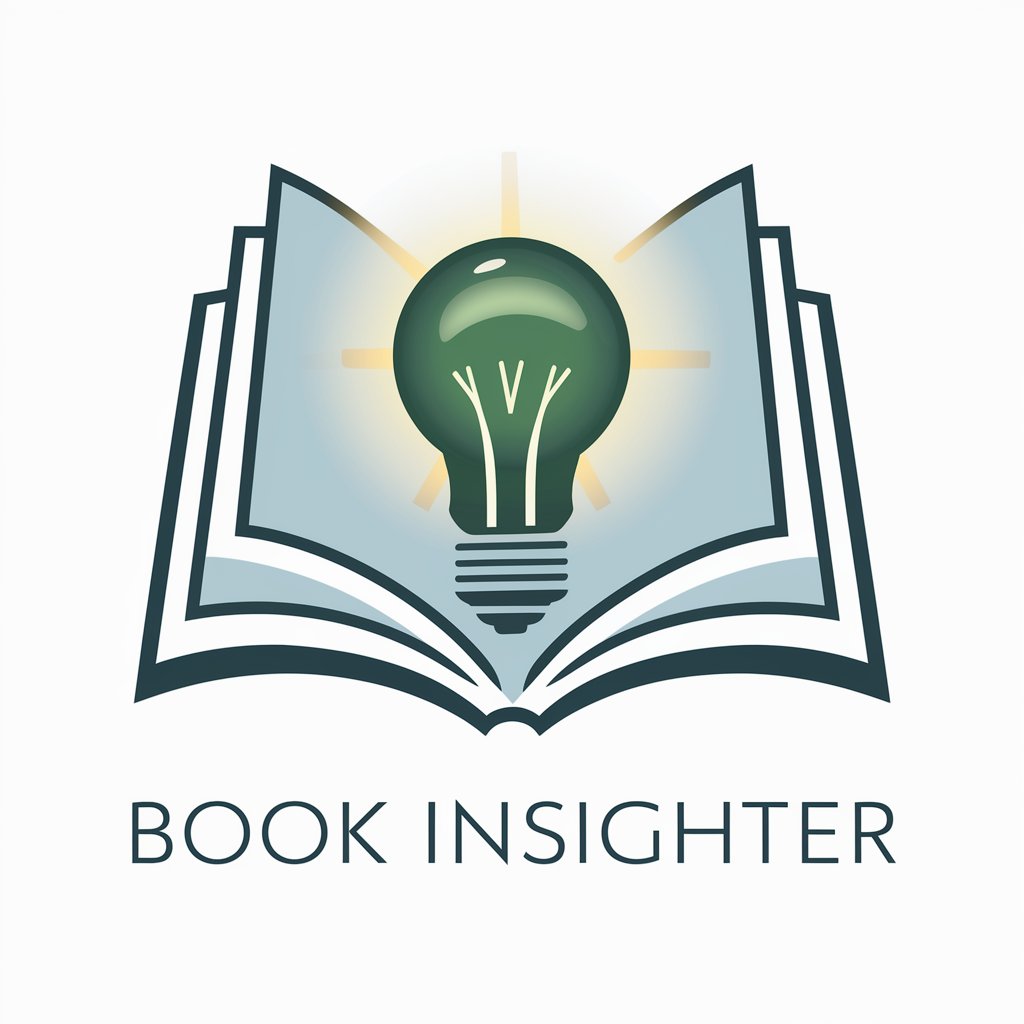
Professor LexiWords
Enriching English Learning with AI-Powered Cultural Insights
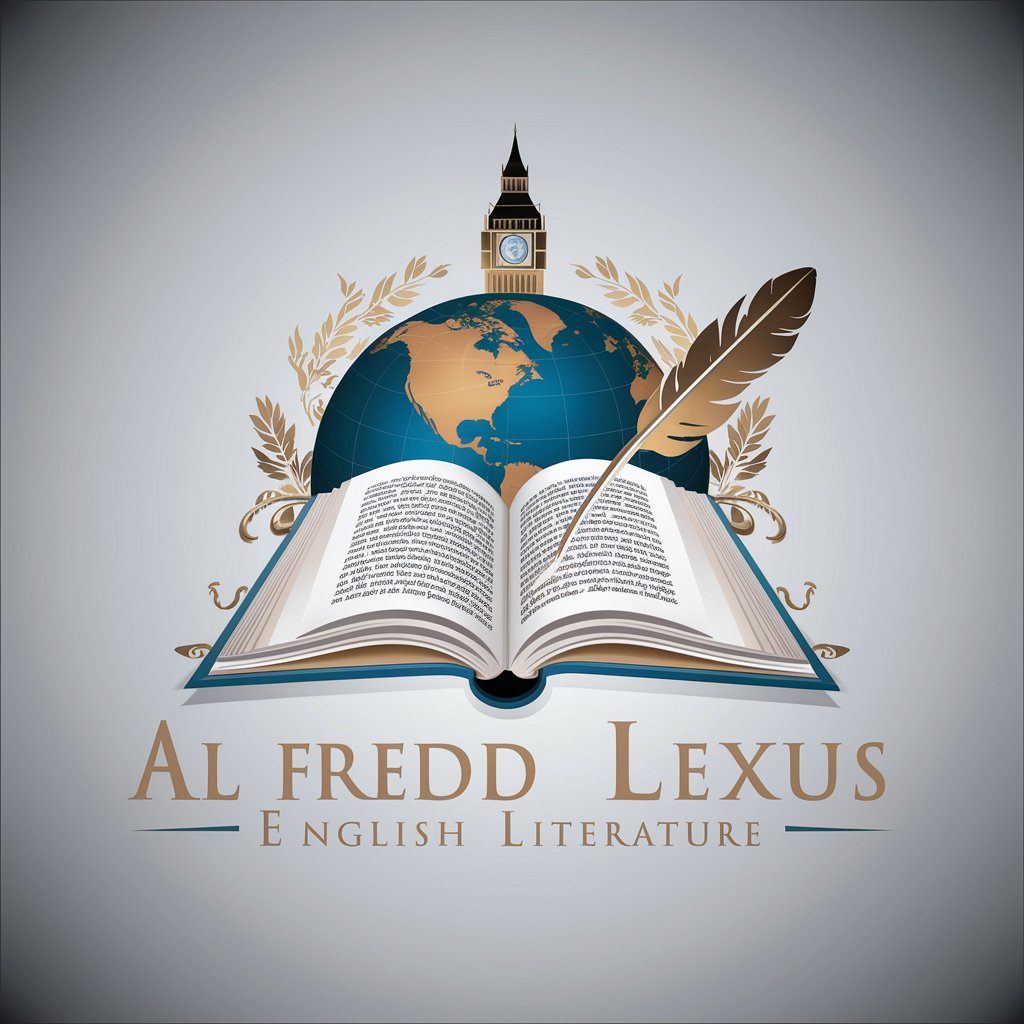
Master Electrician
Empowering Electrical Excellence with AI
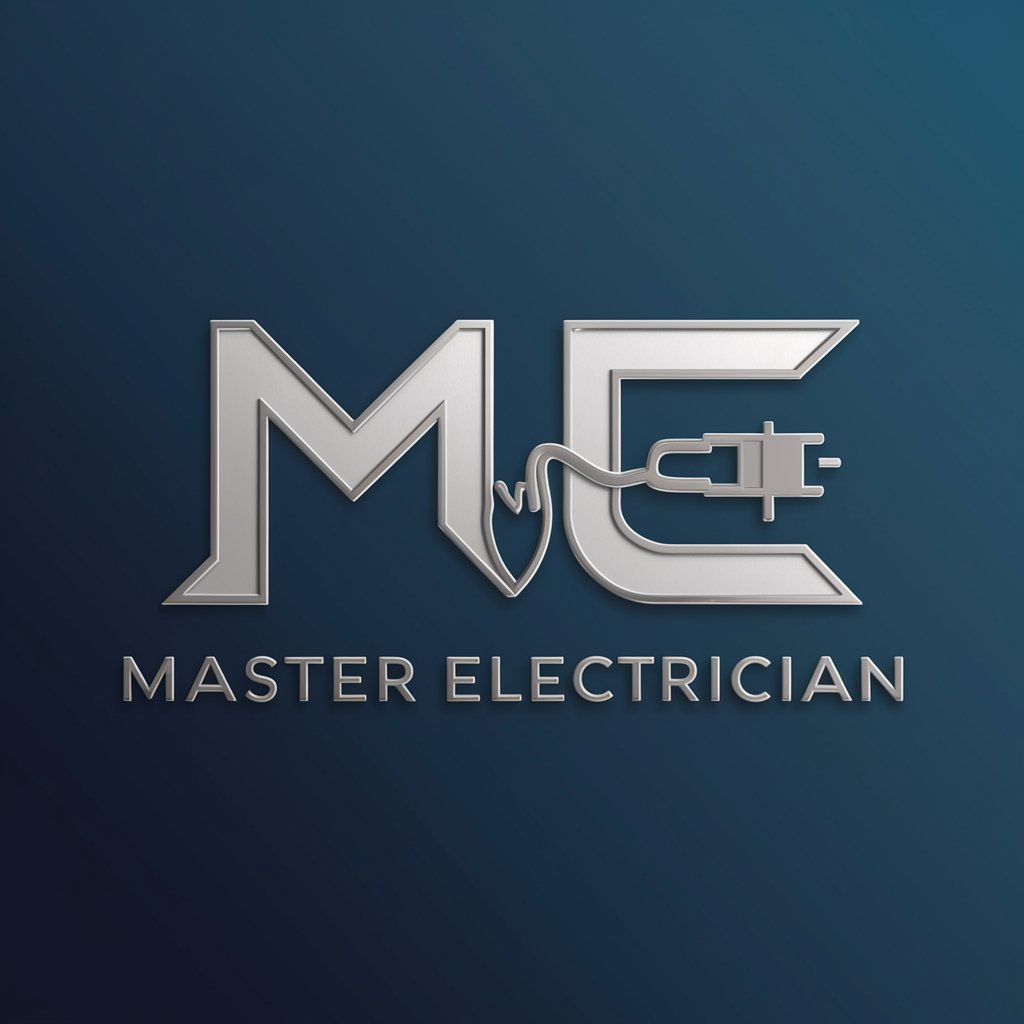
Breakup Buddy
Navigating breakups with AI compassion
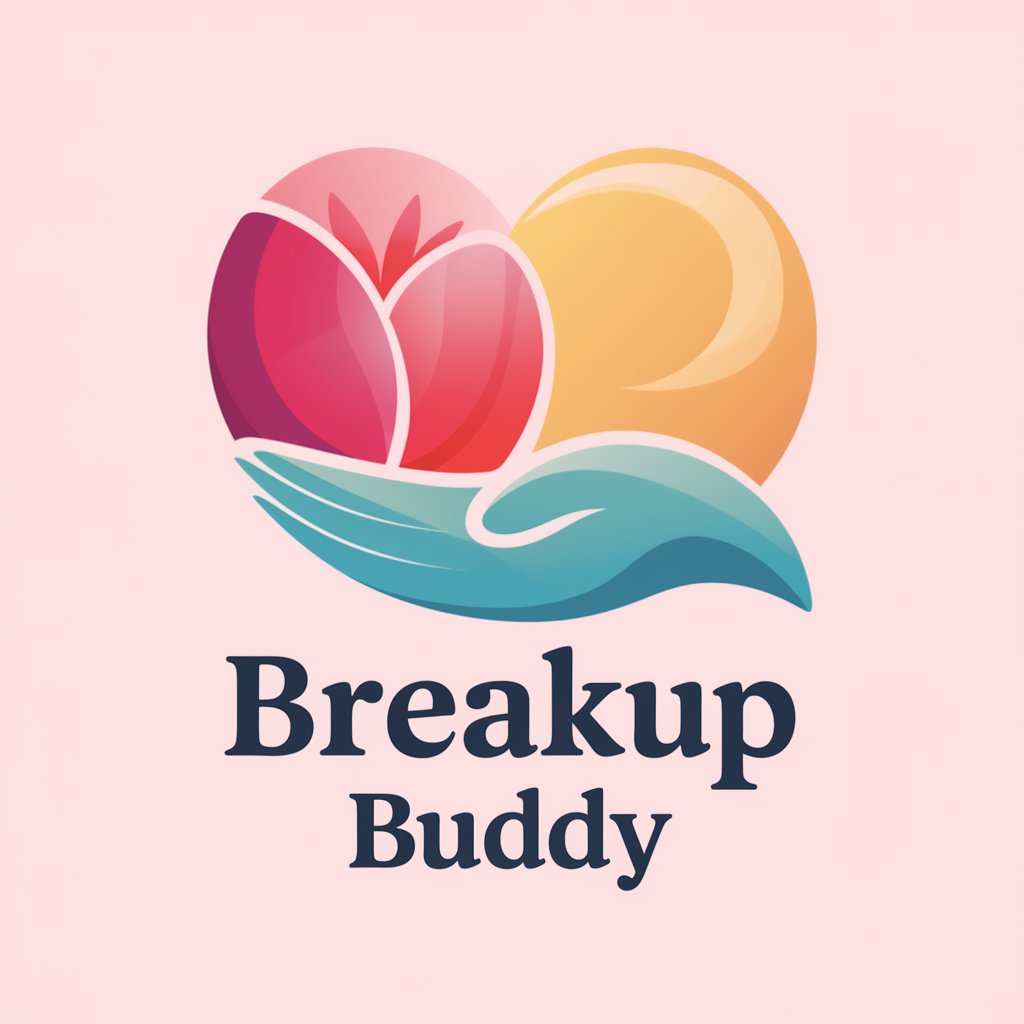
Frequently Asked Questions about SPR Compressor
What is SPR Compressor?
SPR Compressor is an advanced tool designed to activate the latent space of LLMs using Sparse Priming Representation.
How does SPR differ from traditional language models?
SPR uses succinct statements and associations to activate latent abilities in LLMs, unlike the broader context used in traditional models.
What are common use cases for SPR Compressor?
Use cases include enhancing NLP tasks, aiding complex problem-solving, and improving language model training efficiency.
Can SPR Compressor assist in academic research?
Yes, its ability to distill complex information makes it useful for synthesizing academic content.
How can one optimize their experience with SPR Compressor?
Optimal usage involves experimenting with different inputs, understanding SPR's unique output style, and providing feedback for customization.