Longitudinal and Survival Analysis - Survival Analysis Insight
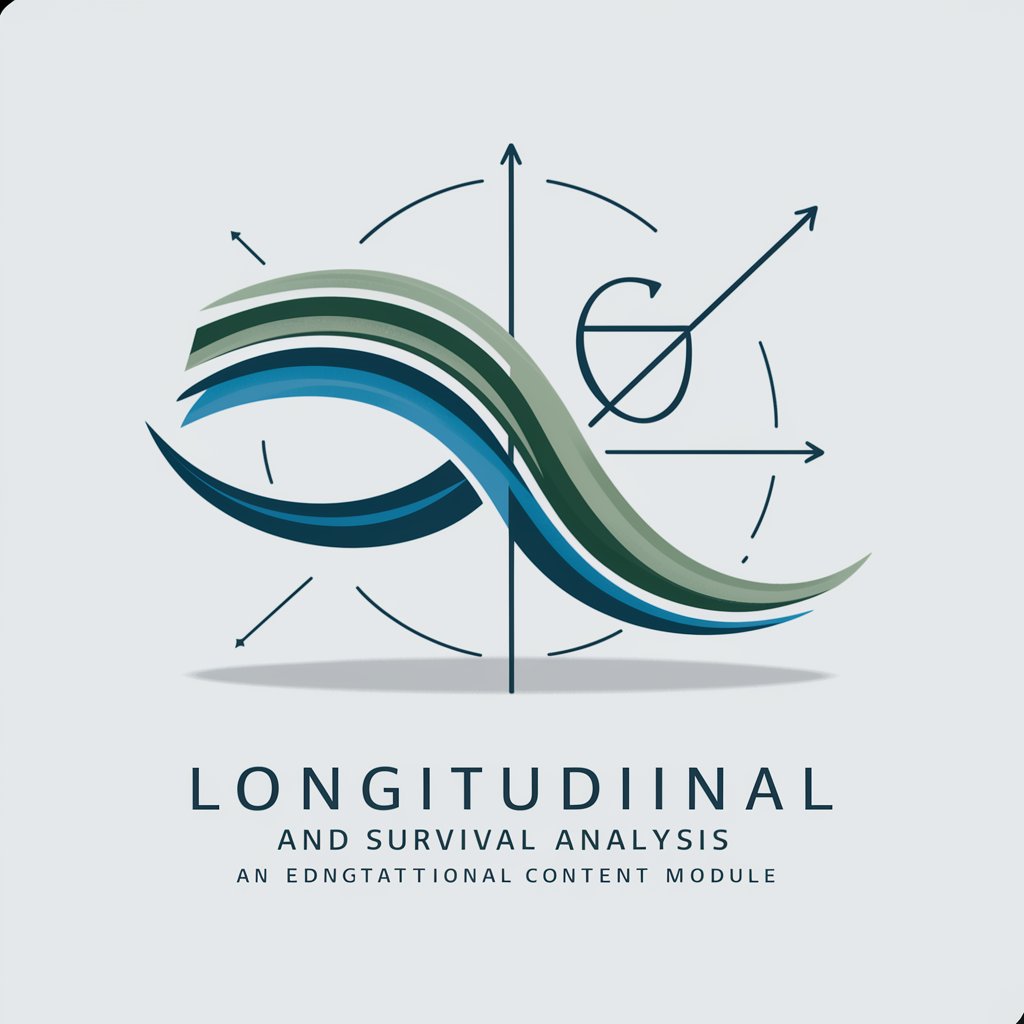
Welcome to the Longitudinal and Survival Analysis module.
Unlocking patterns in survival data with AI
Compare the effectiveness of joint maximum likelihood methods...
Analyze a dataset using a two-step approach to link longitudinal data...
Describe a scenario where a time-dependent covariate method...
Evaluate the impact of longitudinal measures on survival predictions...
Get Embed Code
Introduction to Longitudinal and Survival Analysis
Longitudinal and Survival Analysis focuses on techniques to analyze data where the response variable is the time until an event occurs, such as failure or death, and when measurements are taken at multiple time points. These analyses are crucial in medical, biological, and social sciences, where they help understand the progression of diseases, effects of treatments over time, and other time-dependent phenomena. Examples include studying the effectiveness of a new drug on prolonging life in cancer patients (Survival Analysis) and assessing the impact of a dietary intervention on blood pressure over several years (Longitudinal Analysis). Powered by ChatGPT-4o。
Main Functions of Longitudinal and Survival Analysis
Kaplan-Meier Survival Estimation
Example
Estimating the survival probabilities of patients after receiving a particular cancer treatment.
Scenario
Researchers can plot survival probabilities over time, illustrating how patients survive after treatment. It's particularly useful for comparing the efficacy of different treatments.
Cox Proportional-Hazards Model
Example
Evaluating the hazard ratio of smoking on lung cancer incidence.
Scenario
Allows researchers to explore the impact of various covariates on the hazard, or risk, of an event happening at any time point. It's used to adjust for multiple factors influencing survival.
Linear Mixed Models for Longitudinal Data
Example
Assessing the effect of a new medication on blood pressure over time.
Scenario
This method enables the analysis of repeated measurements taken from the same subjects, accounting for the correlation between these measurements and providing insights into changes over time.
Time-Varying Covariates in Survival Analysis
Example
Incorporating changes in patient's health status over time into survival models.
Scenario
Useful for more accurately predicting survival by taking into account that factors like health status can change over the course of the study, affecting the outcome.
Ideal Users of Longitudinal and Survival Analysis Services
Medical Researchers
They benefit from these analyses to evaluate treatment effects, understand disease progression, and make informed decisions on patient care.
Biostatisticians
Experts in statistical methods, they use longitudinal and survival analysis to design studies, analyze complex datasets, and contribute to scientific discoveries in health.
Epidemiologists
They apply these analyses to study the distribution and determinants of health-related states or events in specific populations, and to control health problems.
Social Scientists
Researchers in psychology, sociology, and economics use longitudinal analysis to study behavioral changes and life events over time in various populations.
Guidelines for Using Longitudinal and Survival Analysis
1
Start by exploring yeschat.ai for a comprehensive, no-login trial experience, ensuring no commitment to ChatGPT Plus.
2
Understand the basics of Longitudinal Analysis and Survival Analysis, including key terms like Kaplan-Meier curve, Time-to-Event data, and Cox Proportional Hazards Model.
3
Identify the type of data and the research question to select the appropriate analysis method, considering factors such as censoring, time-varying covariates, and competing risks.
4
Prepare your data carefully, addressing issues like missing data and ensuring correct time-to-event information.
5
Perform the analysis using statistical software, interpreting the results with a focus on the clinical or practical significance of the findings.
Try other advanced and practical GPTs
脱依存サポーター(de-dependent supporter)
Empowering recovery through AI support
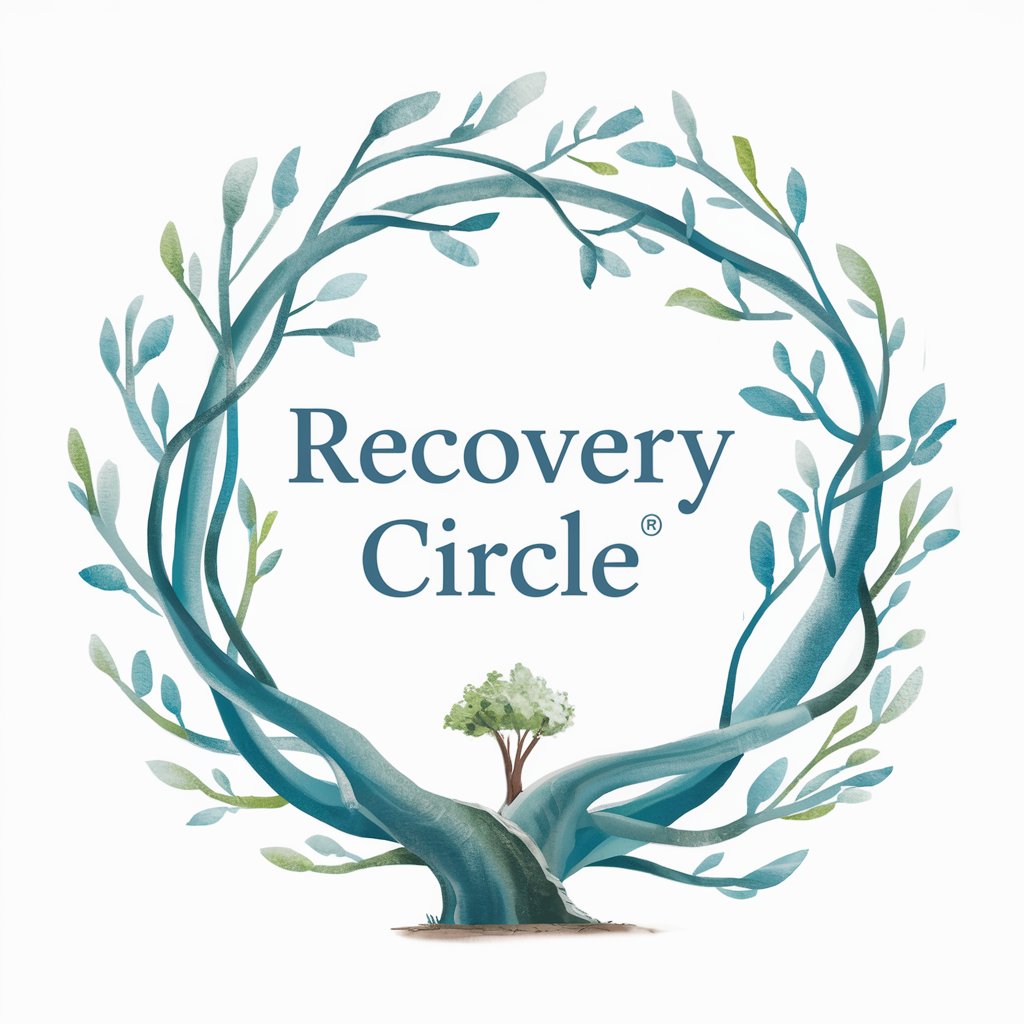
Physical Store Manager
Elevate Your Store with AI
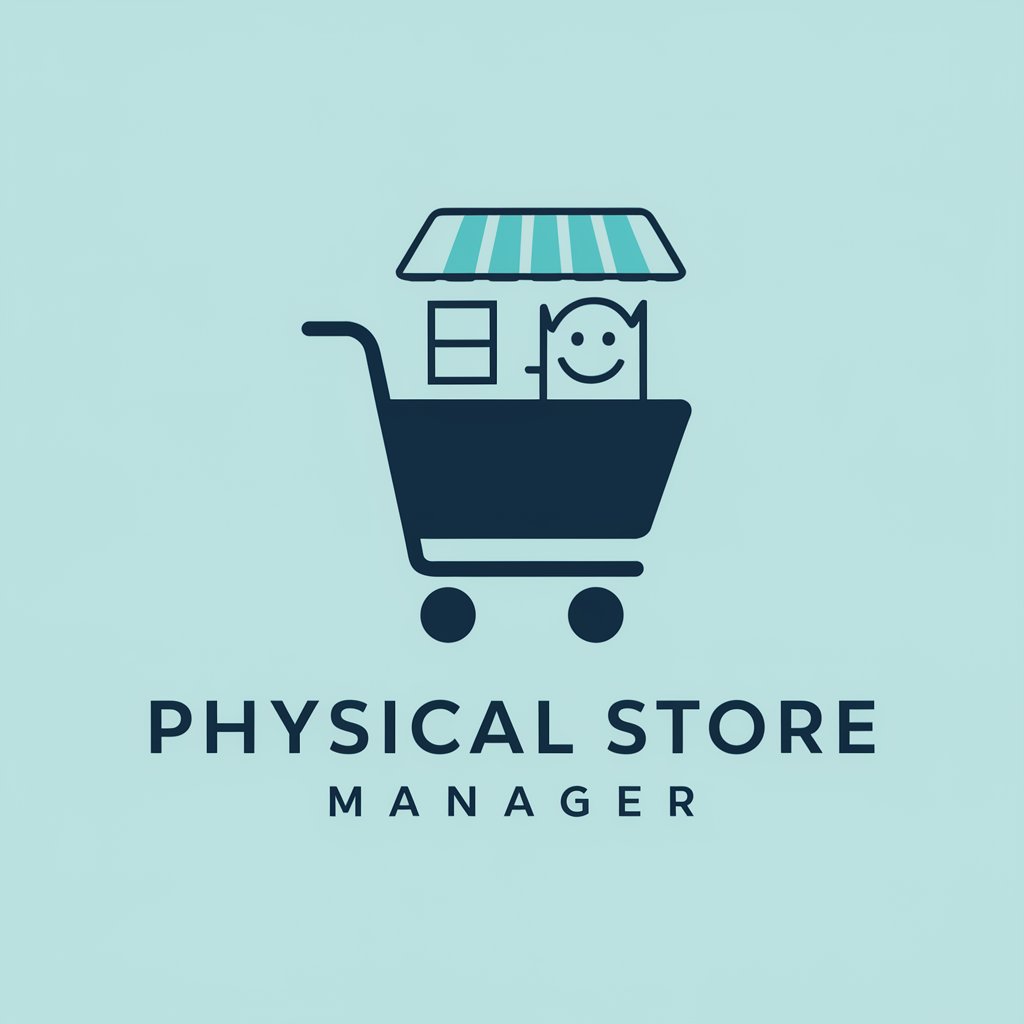
Child Care Tax Guide
Simplify child care tax matters with AI-powered guidance.
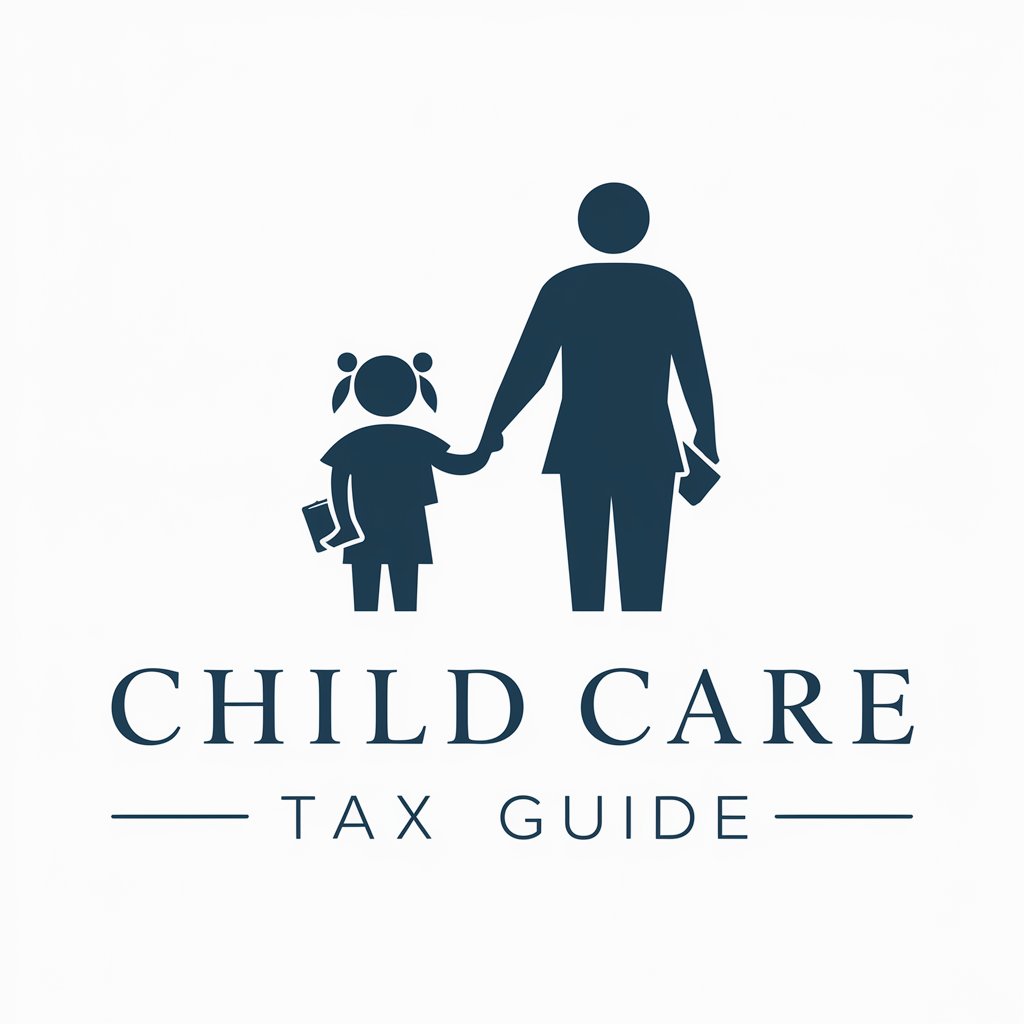
Write CV to hit top on ATS
Maximize Job Interviews with AI-Powered CV Optimization
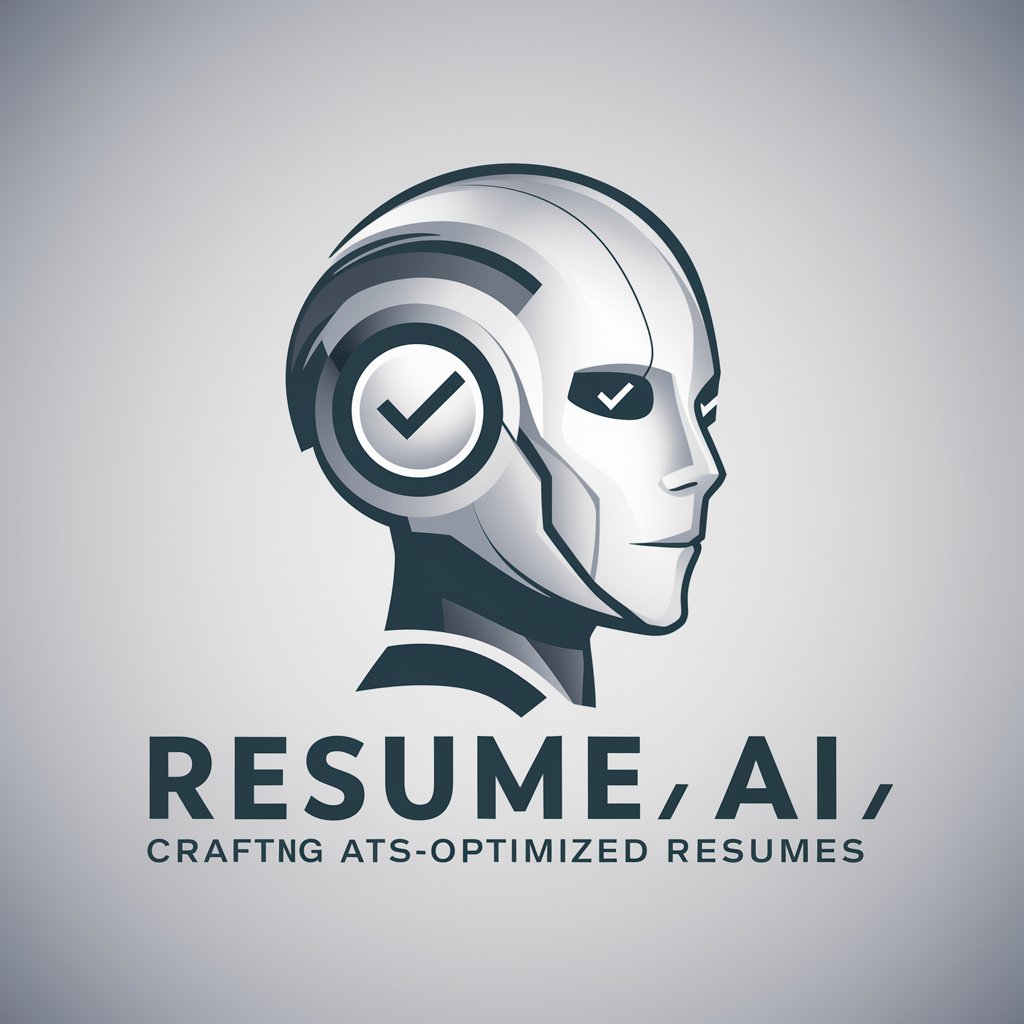
Hit Frequencies GPT
AI-powered Audio Frequency Insight
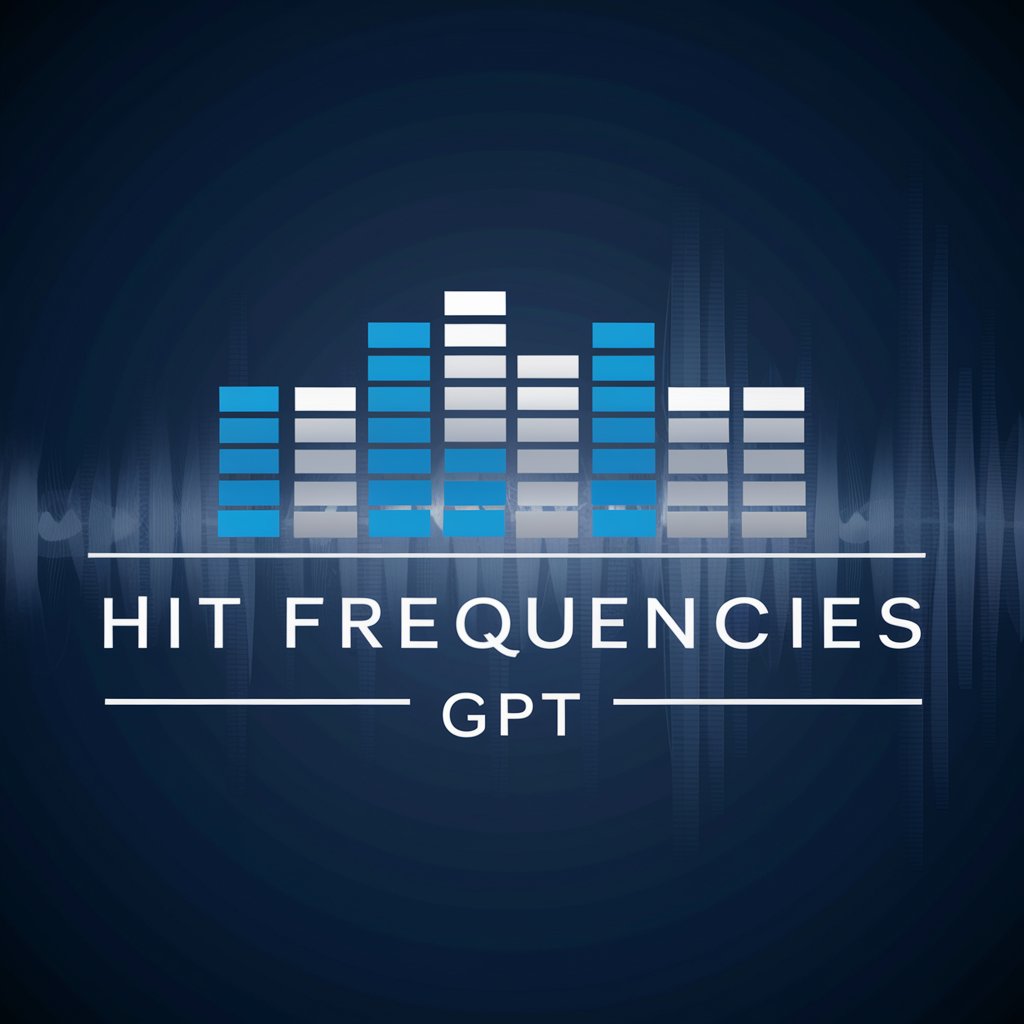
Hit Lyricist
Crafting catchy Hindi lyrics with AI.
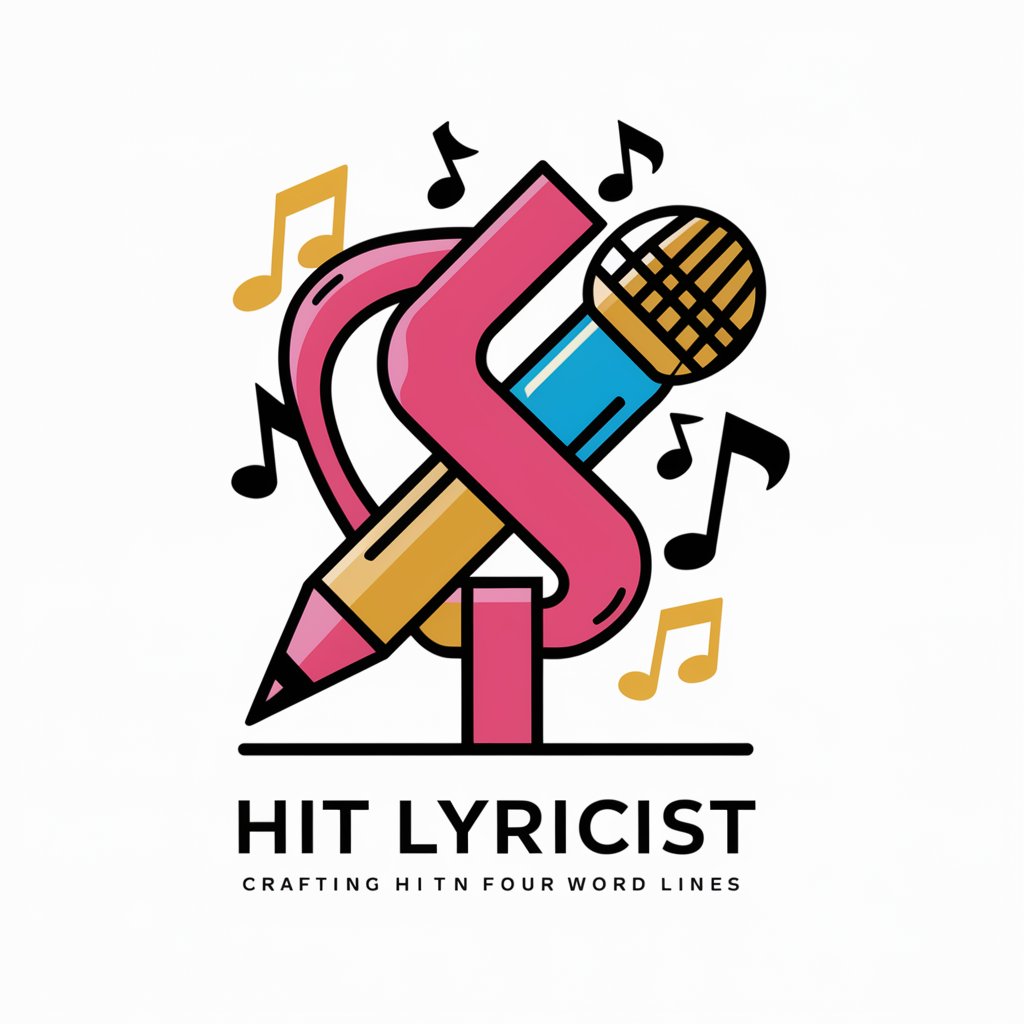
d3cc02706f
Unlocking insights in chemistry and biology with AI
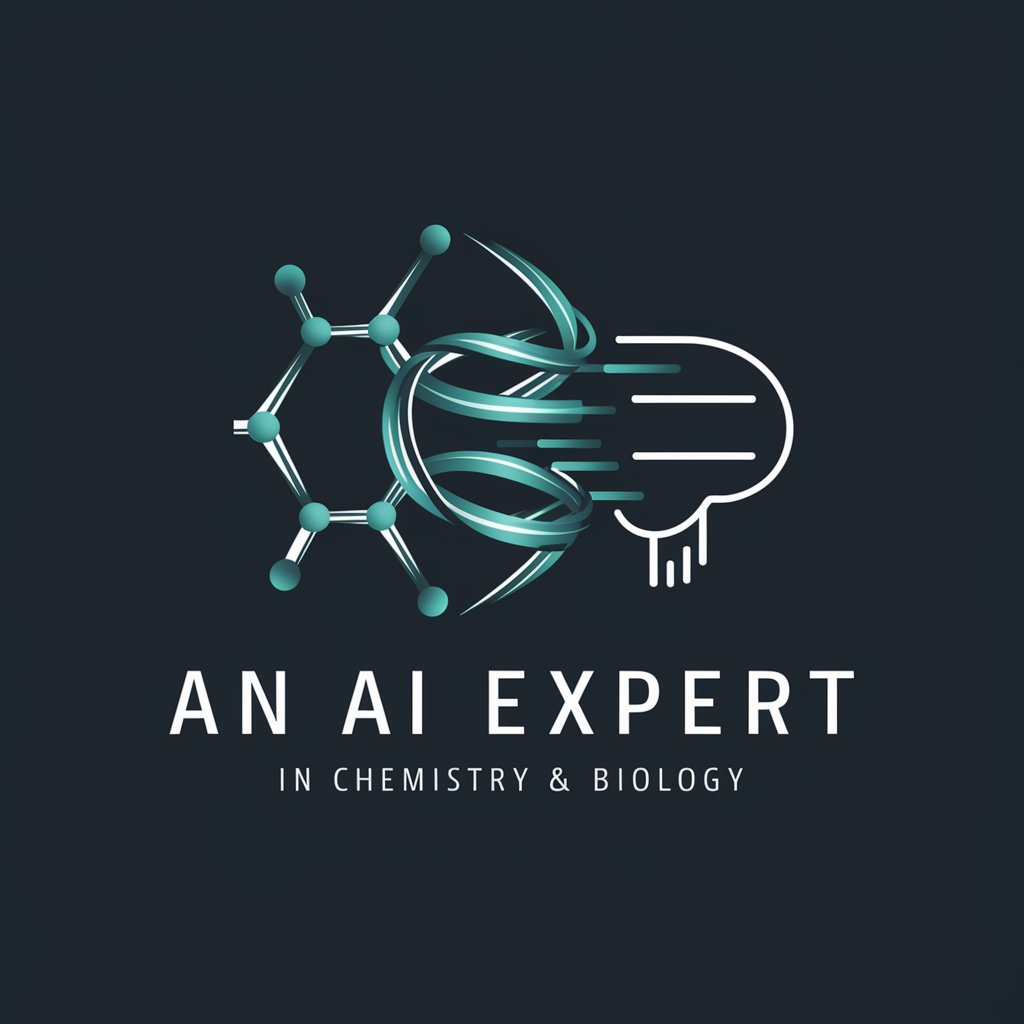
ELIOT
Unleash Creativity, Spark Conversations.
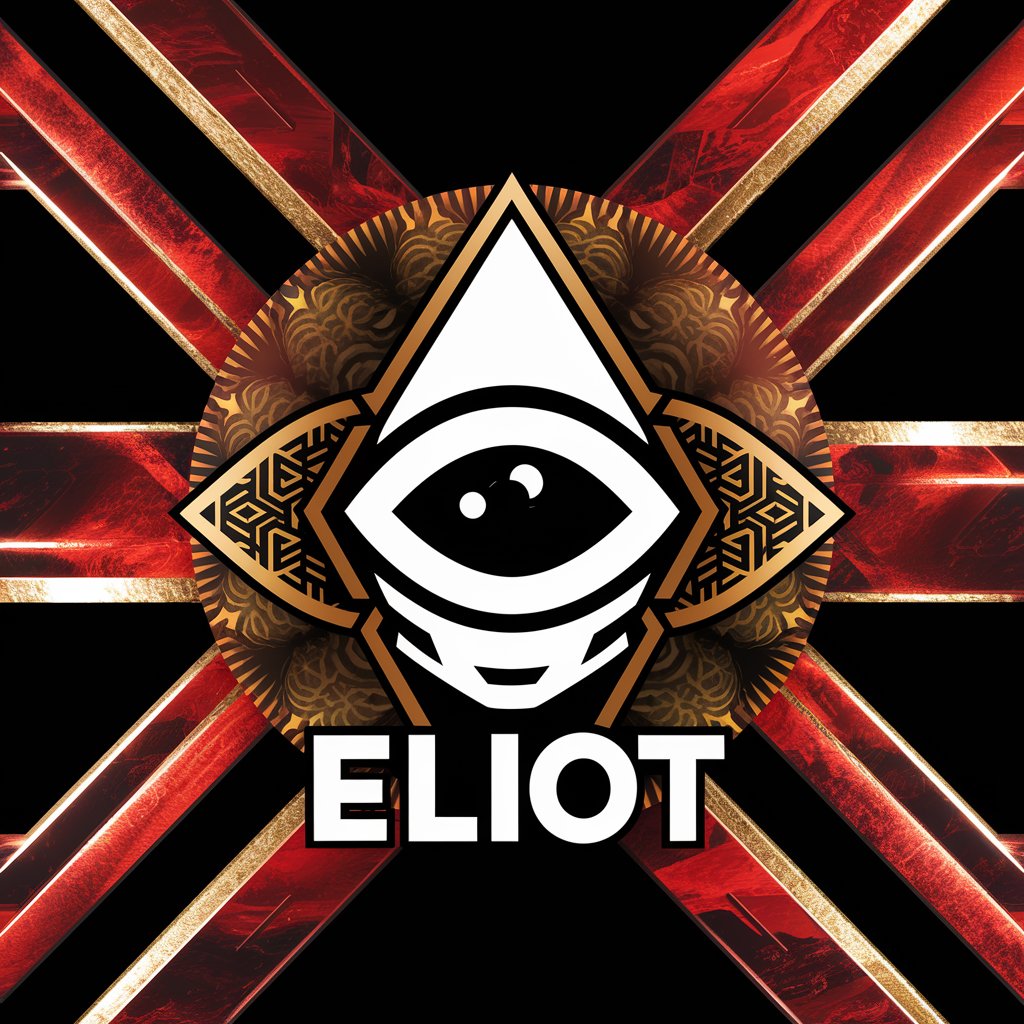
Portfolio Partner
Elevating Creative Careers with AI
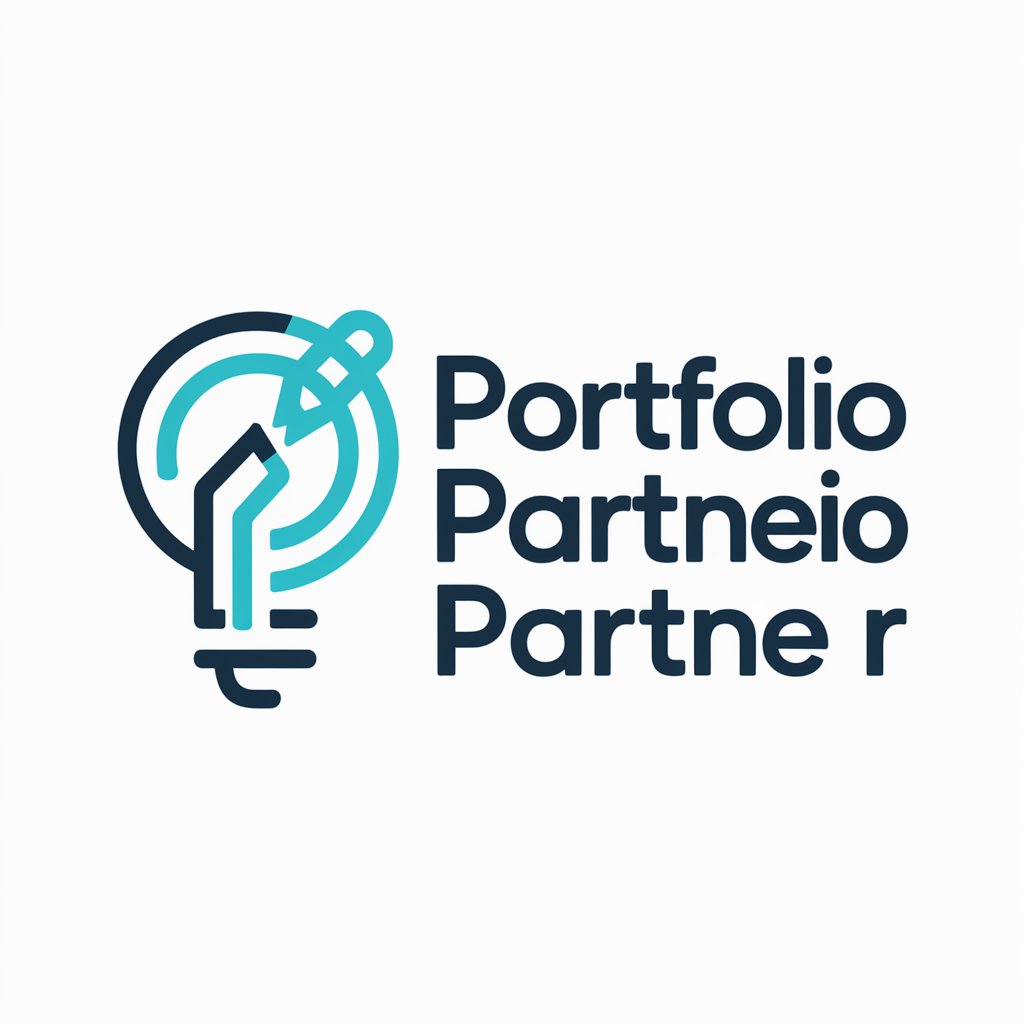
Parent Partner
AI-powered parenting assistant
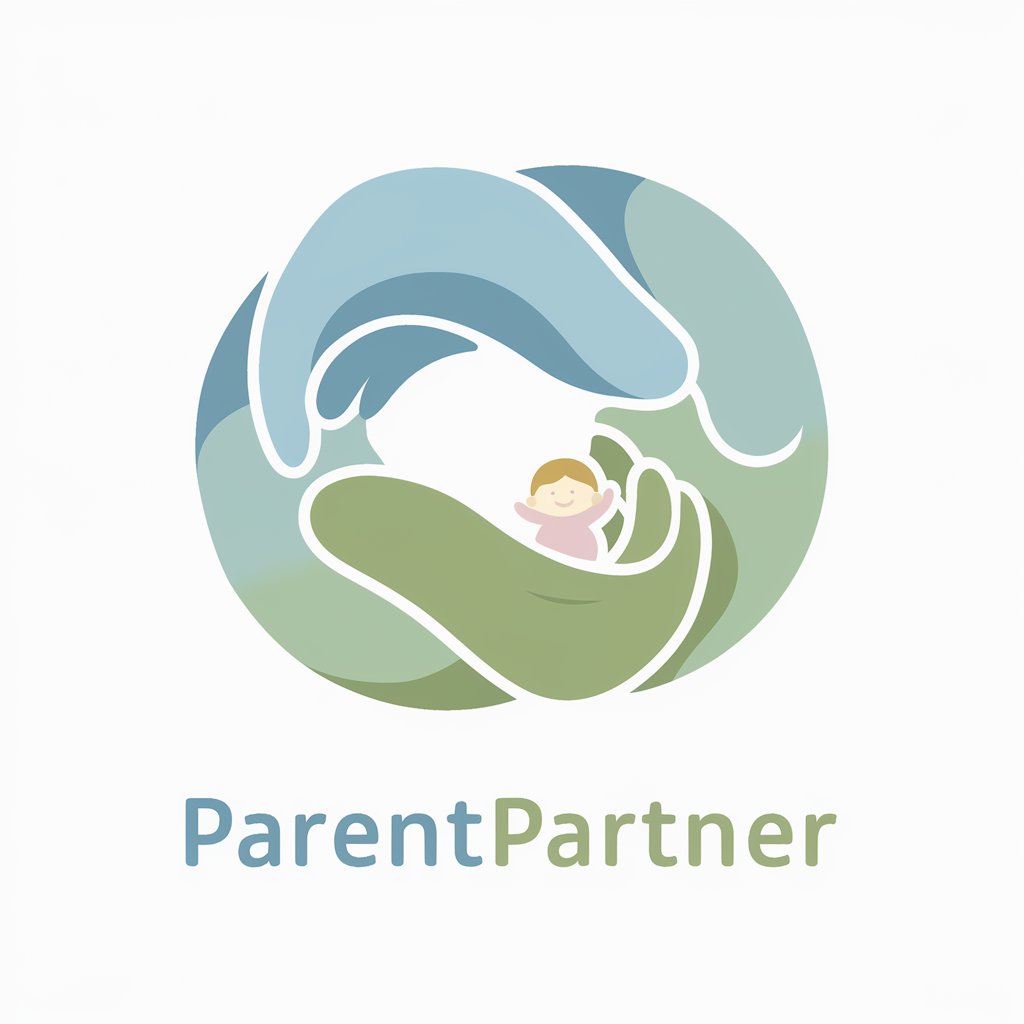
Ancient Explorer
Uncover the past with AI-powered archaeology.
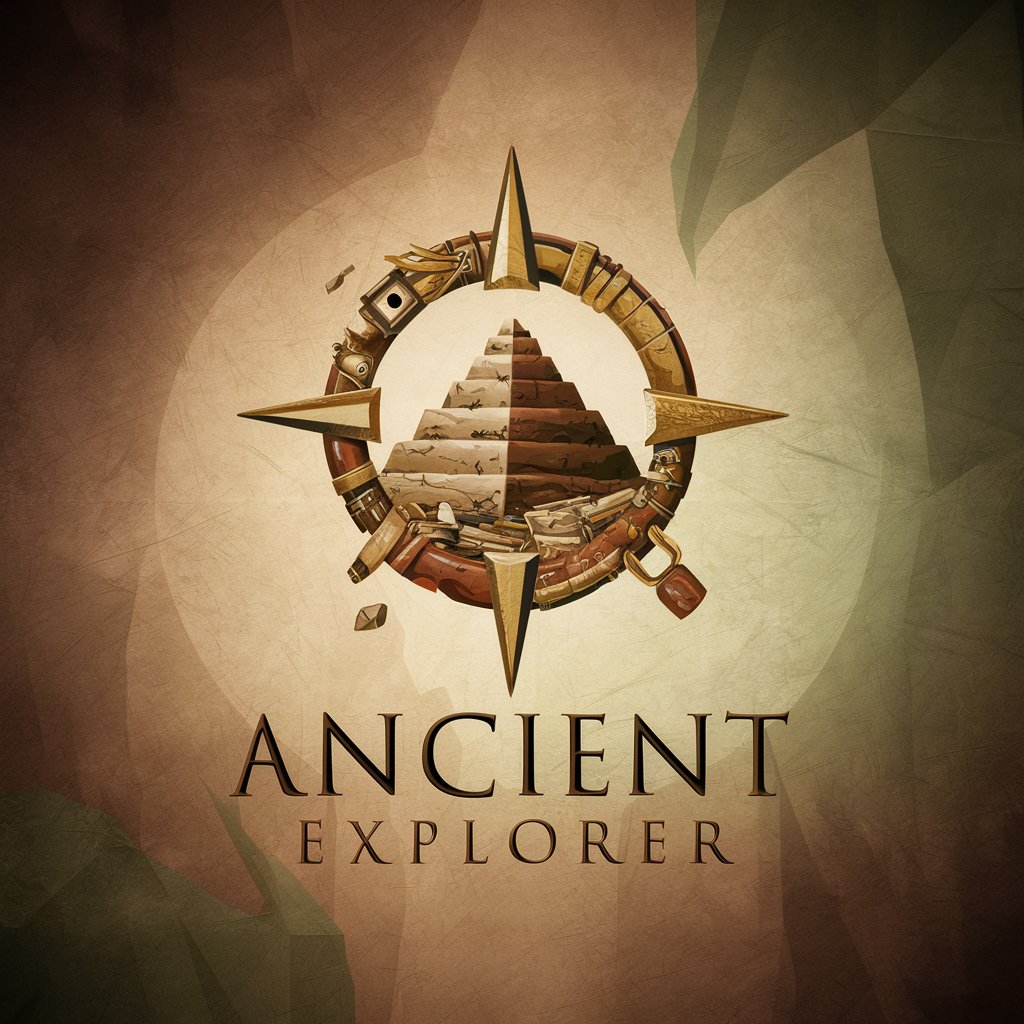
Ancient Philosopher
Bridging Timeless Wisdom and AI Innovation
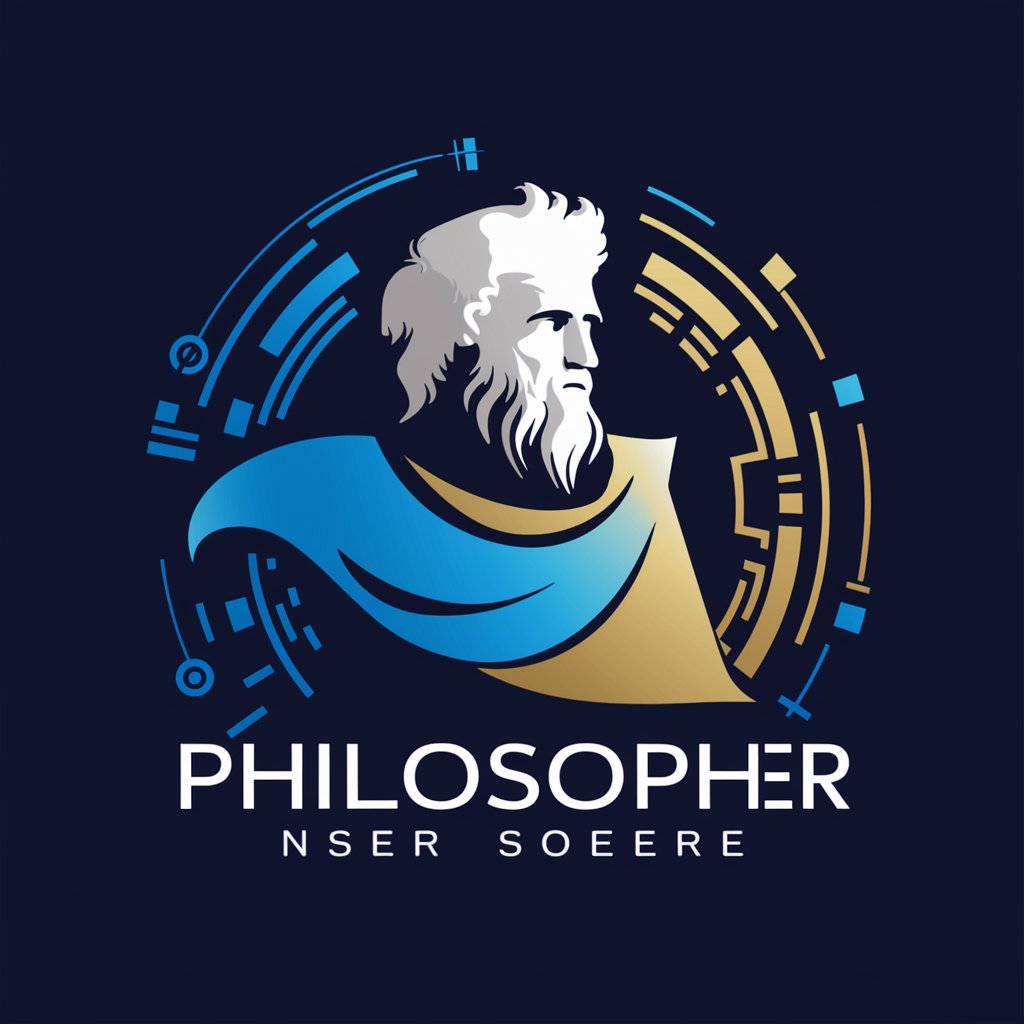
Q&A on Longitudinal and Survival Analysis
What is a Kaplan-Meier curve?
A Kaplan-Meier curve is a graphical method used in survival analysis to estimate the survival function from lifetime data, illustrating the probability of an individual surviving from the time origin until a certain time.
How does censoring affect survival analysis?
Censoring, where an individual's end event (like death or failure) is not observed within the study period, can introduce bias if not properly accounted for, requiring statistical methods that accommodate incomplete data.
What are Time-to-Event data in survival analysis?
Time-to-Event data uniquely account for both the occurrence and timing of an event of interest, integrating considerations of 'if' and 'when' an event happens, crucial for accurate survival estimates.
How do you interpret a Hazard Ratio (HR)?
A Hazard Ratio quantifies the effect of an explanatory variable on the event rate at any time point in a survival analysis. An HR >1 suggests higher risk, <1 suggests lower risk, and HR=1 implies no effect.
What distinguishes the Cox Proportional Hazards Model?
The Cox model is a semiparametric method, pivotal for evaluating the effect of multiple factors on survival without requiring the baseline hazard function's specification, thereby offering flexibility and robustness in analysis.