LLM cost estimator-LLM Training Cost Calculator
Estimate LLM Training Costs Effortlessly
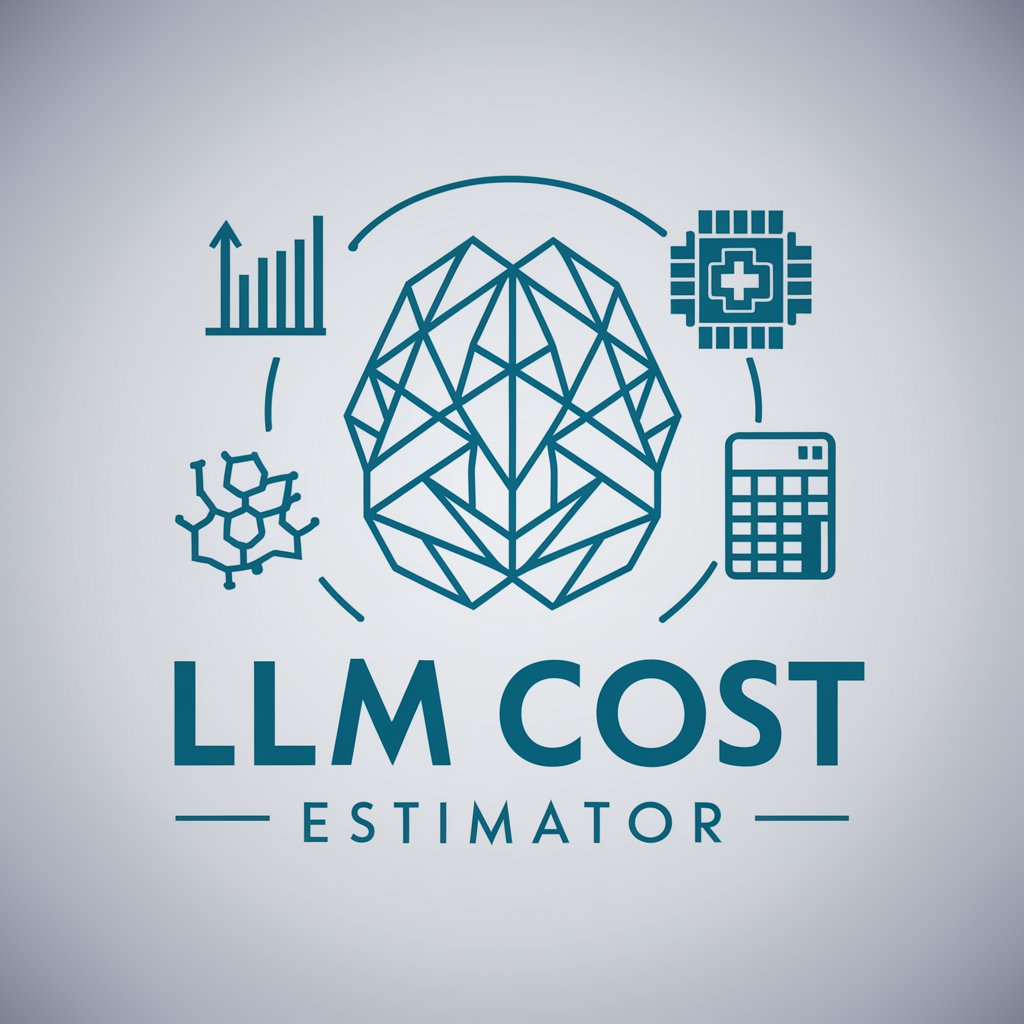
Estimate the GPU costs for training...
Calculate the training time for a model with...
Analyze the impact of batch size on...
Determine the optimal cloud provider for...
Related Tools
Load More
LLM Expert
Expert on LLMs, RAG technology, LLaMA-Index, Hugging Face, and LangChain.
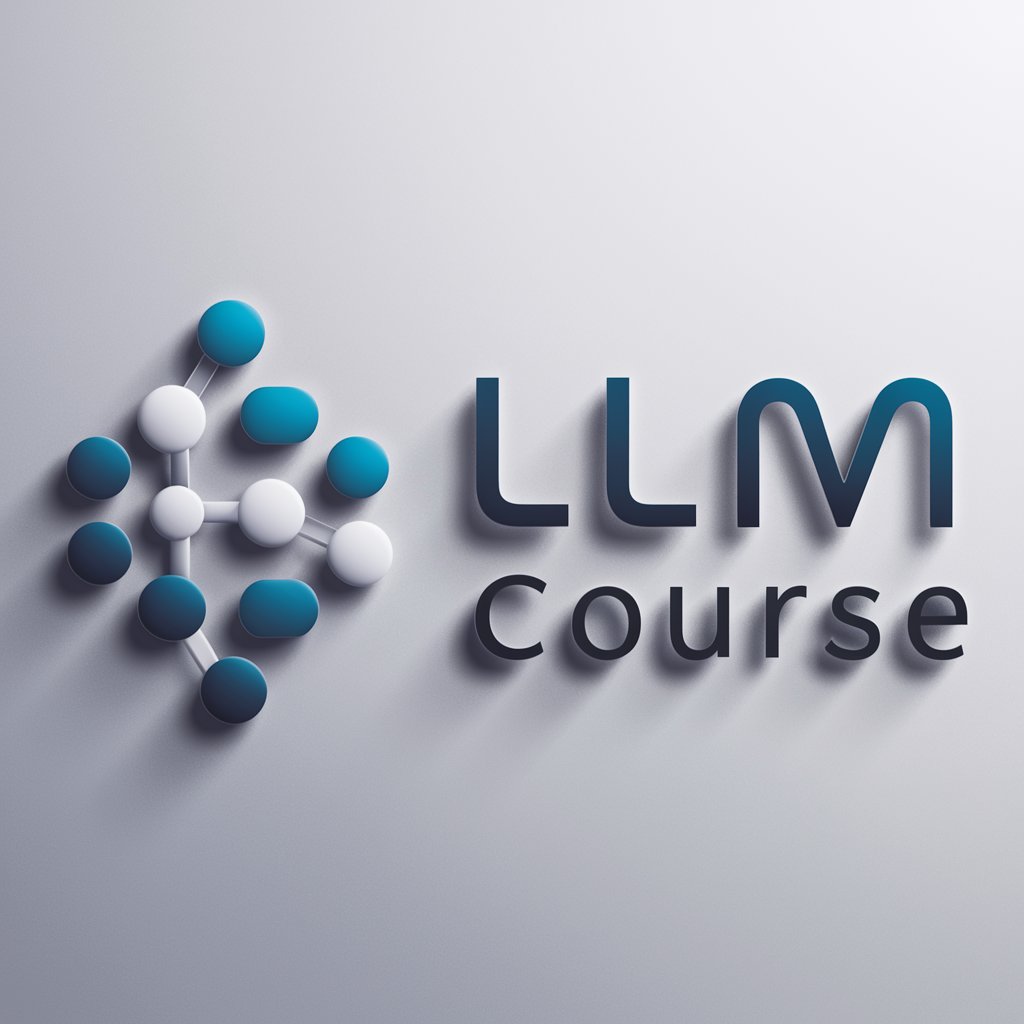
LLM Course
An interactive version of the LLM course tailored to your level (https://github.com/mlabonne/llm-course)

LLM Expert
A research advisor for novel ML ideas
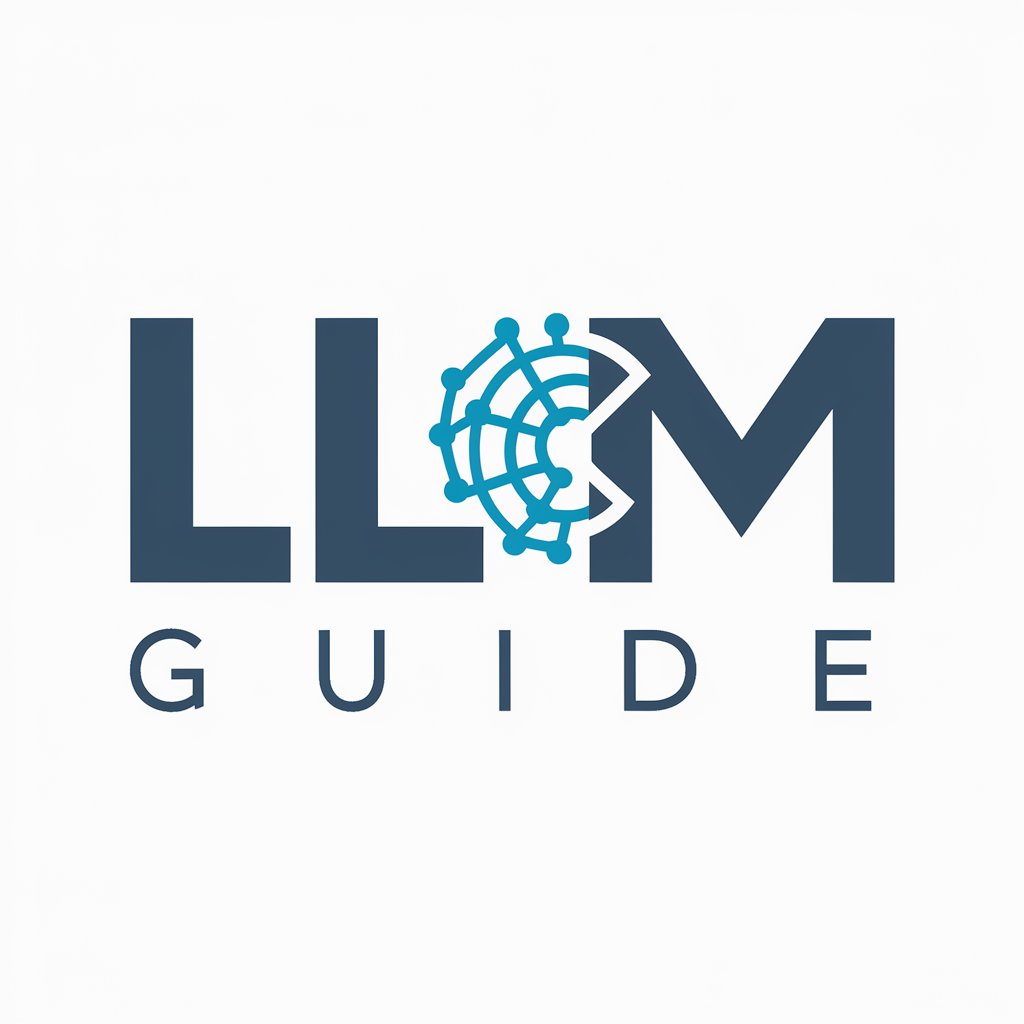
LLM Guide
Formal but friendly LLM technical advisor.
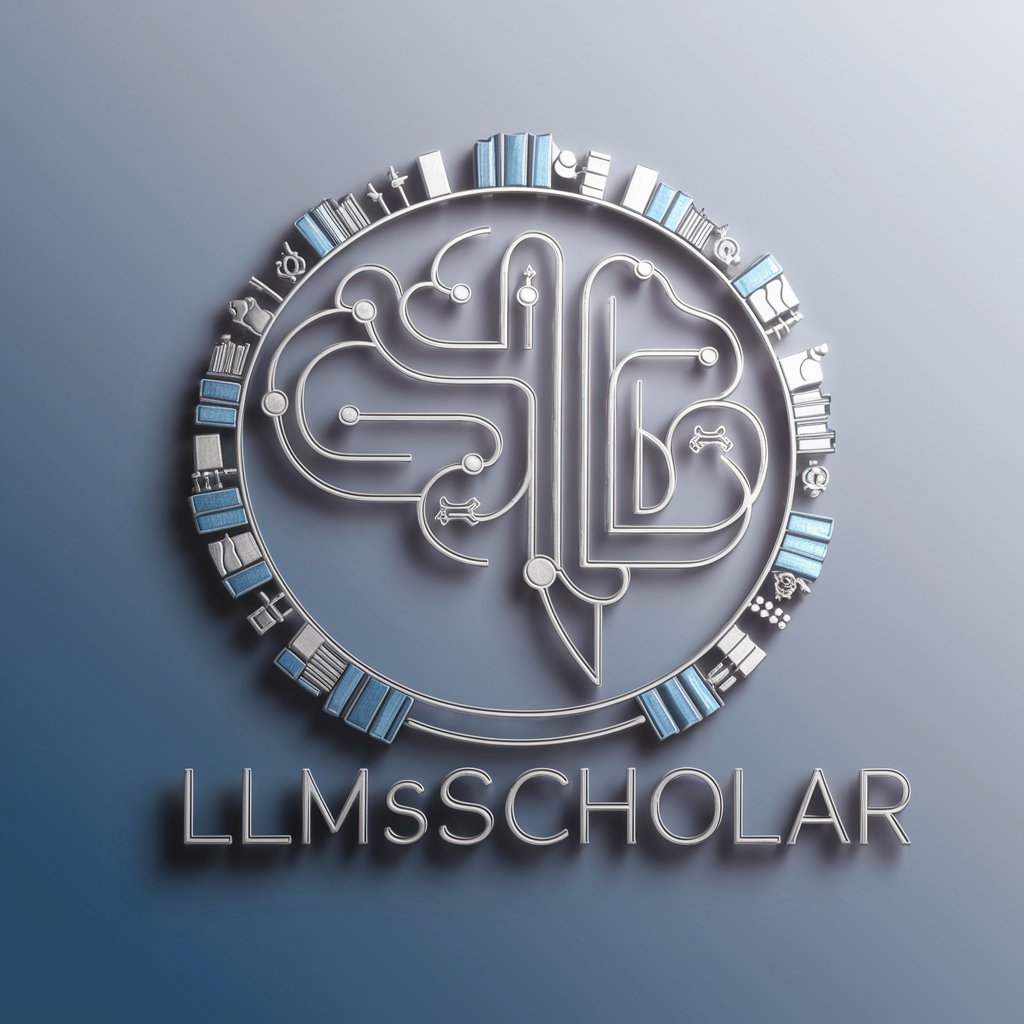
LLMScholar
GPT for latest LLM research
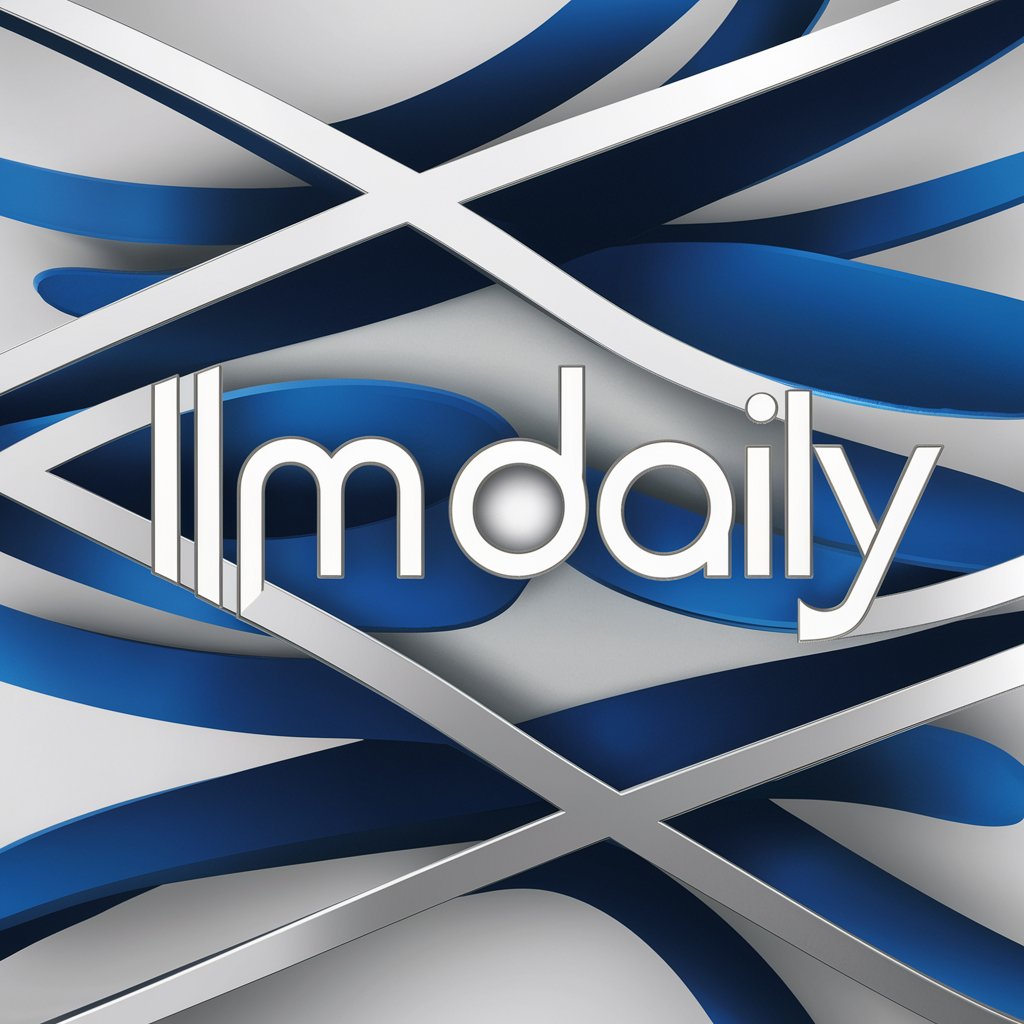
LLM Daily
Daily updates on LLM news and trends
LLM Cost Estimator Introduction
The LLM Cost Estimator is a specialized tool designed to provide accurate and detailed cost analyses for training large language models (LLMs). This tool is essential for organizations and individuals looking to understand the financial implications of deploying LLMs. It considers various factors including hardware costs, model architecture, training dynamics, and optimizing training performance. For instance, it can estimate the cost implications of choosing different GPUs or cloud services, adjusting model size, or experimenting with training parameters like batch size and learning rate. Powered by ChatGPT-4o。
Main Functions of LLM Cost Estimator
Hardware Cost Calculation
Example
Estimating the cost of GPUs such as Nvidia A100 or A10G based on hourly rates and expected usage time. This helps in deciding whether to use cloud GPUs or invest in in-house hardware based on the budget and project duration.
Scenario
A company planning to train an LLM can use the estimator to decide whether to rent cloud GPUs from providers like AWS, GCP, or use in-house resources.
Model Architecture Cost Impact
Example
Assessing how different model architectures, such as the number of layers and parameters, influence training costs. Techniques like sparsity or low-rank matrix factorization are evaluated for their cost-saving potentials.
Scenario
An AI research team can analyze how increasing the model's depth or width would affect the cost and compute requirements, helping them balance performance with budget constraints.
Optimization of Training Costs
Example
Providing cost-benefit analyses of various training optimizations such as mixed precision training, hyperparameter tuning, and early stopping.
Scenario
An educational institution might use the estimator to determine the most cost-effective training strategies for their AI courses, maximizing learning outcomes while minimizing expenses.
Ideal Users of LLM Cost Estimator Services
AI Research Teams
These users need precise cost estimations to budget their projects, particularly when exploring new model architectures or optimization techniques. The tool helps them in allocating resources efficiently and justifying the costs to stakeholders.
Tech Startups
Startups with limited budgets benefit from understanding the exact cost implications of different LLM training strategies, allowing them to optimize their limited resources for maximum model performance.
Educational Institutions
Institutions teaching AI and machine learning can use the tool to demonstrate to students the cost dynamics of LLM training, preparing them for real-world AI applications and budget management.
Using LLM Cost Estimator
Step 1
Visit yeschat.ai for a free trial without login, also no need for ChatGPT Plus.
Step 2
Choose a model architecture suitable for your needs and input the expected training parameters such as batch size and learning rate.
Step 3
Review the hardware and cloud provider costs associated with the resources needed for model training.
Step 4
Utilize the estimator's feedback to adjust parameters and optimize costs and training efficiency.
Step 5
Run simulations multiple times with different configurations to find the most cost-effective approach.
Try other advanced and practical GPTs
API Cost Advisor
Optimize your API costs with AI-powered insights.
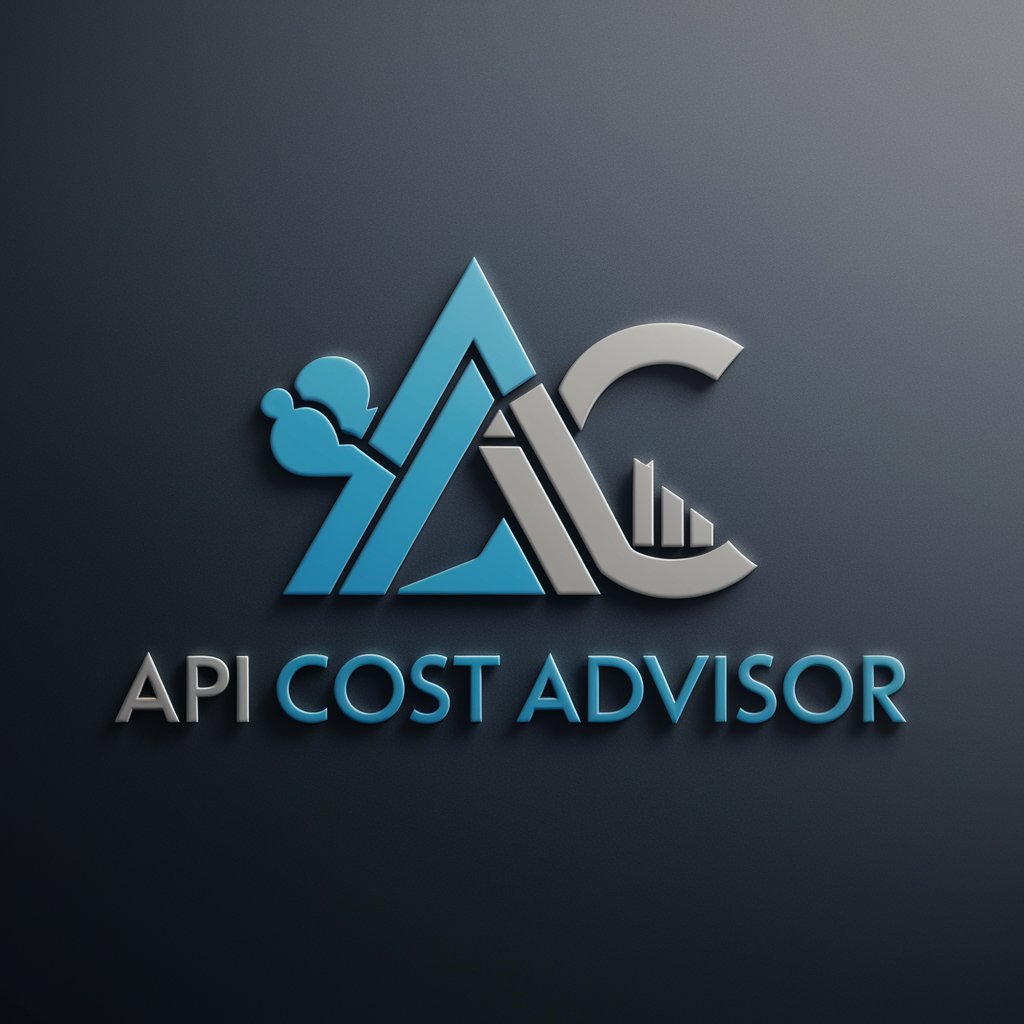
Cost Insighter
Precision in Construction Costing
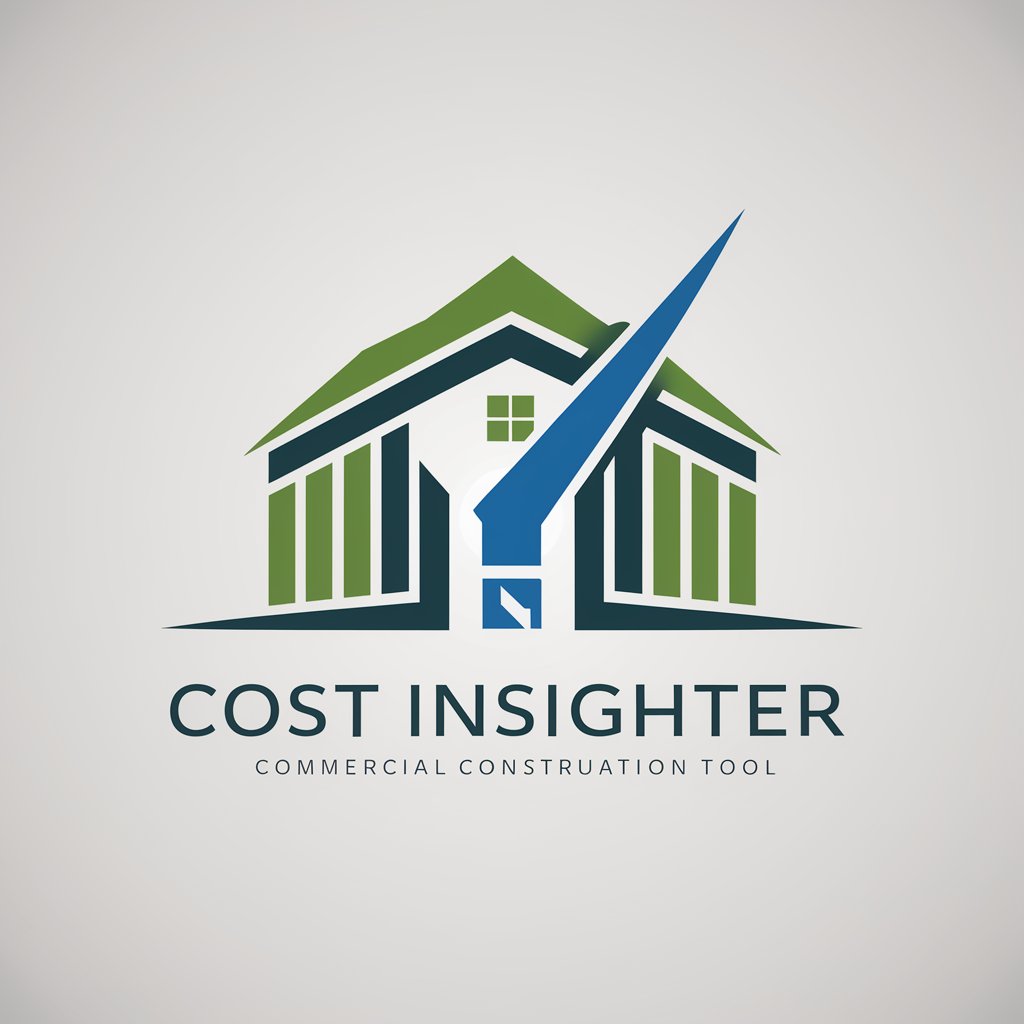
Cost-Dude
Optimize costs with AI-driven insights
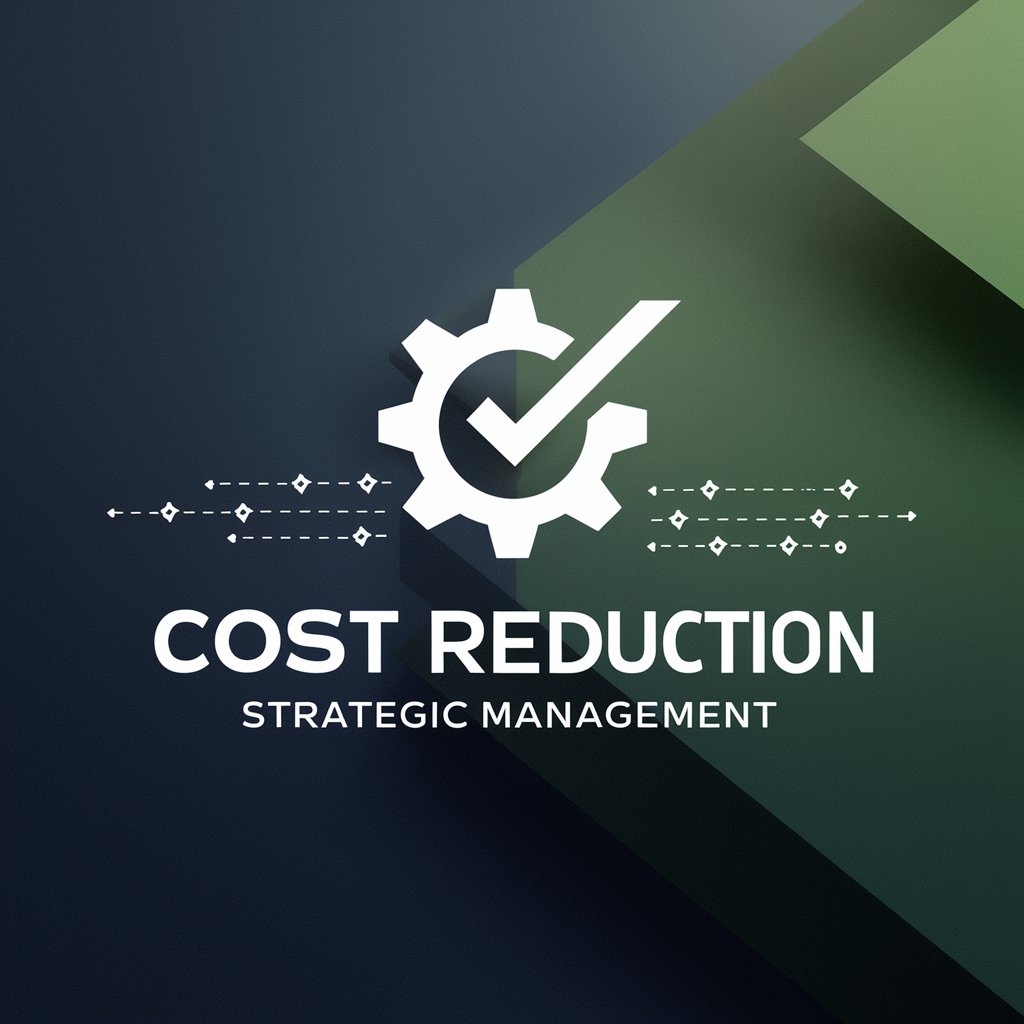
Beautiful GPT
Enhancing Beauty with AI
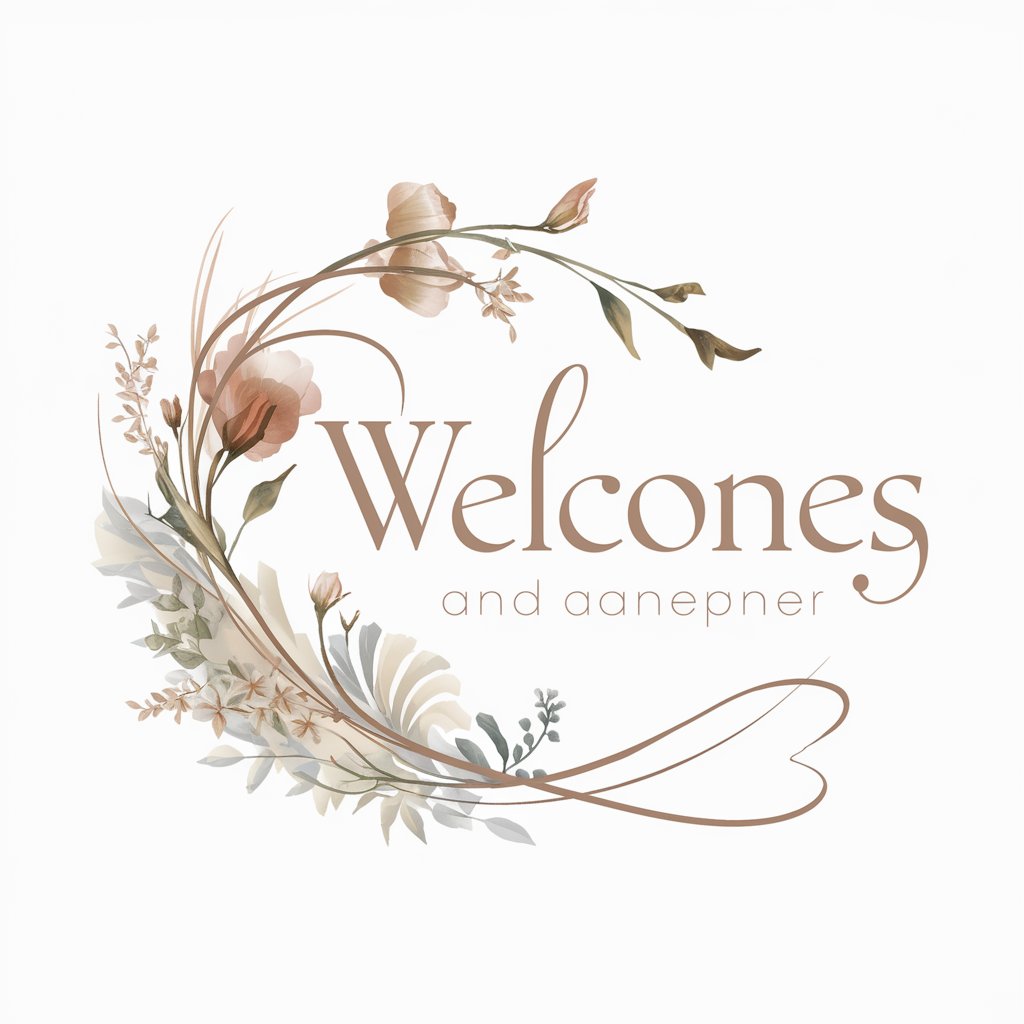
Beautiful Cosmos Guide
Unlocking the cosmos with AI
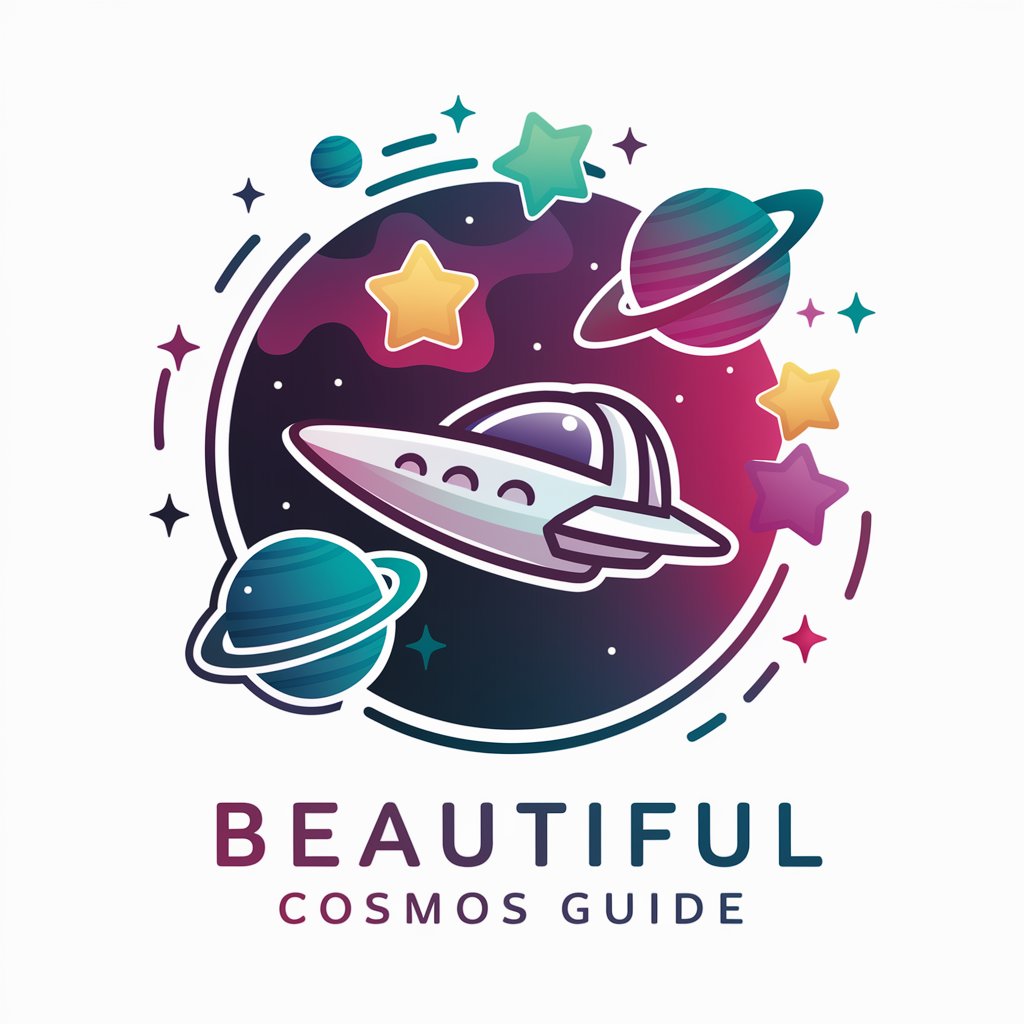
Study Guide / Lesson Planner Pro
Tailor-made Learning with AI Power
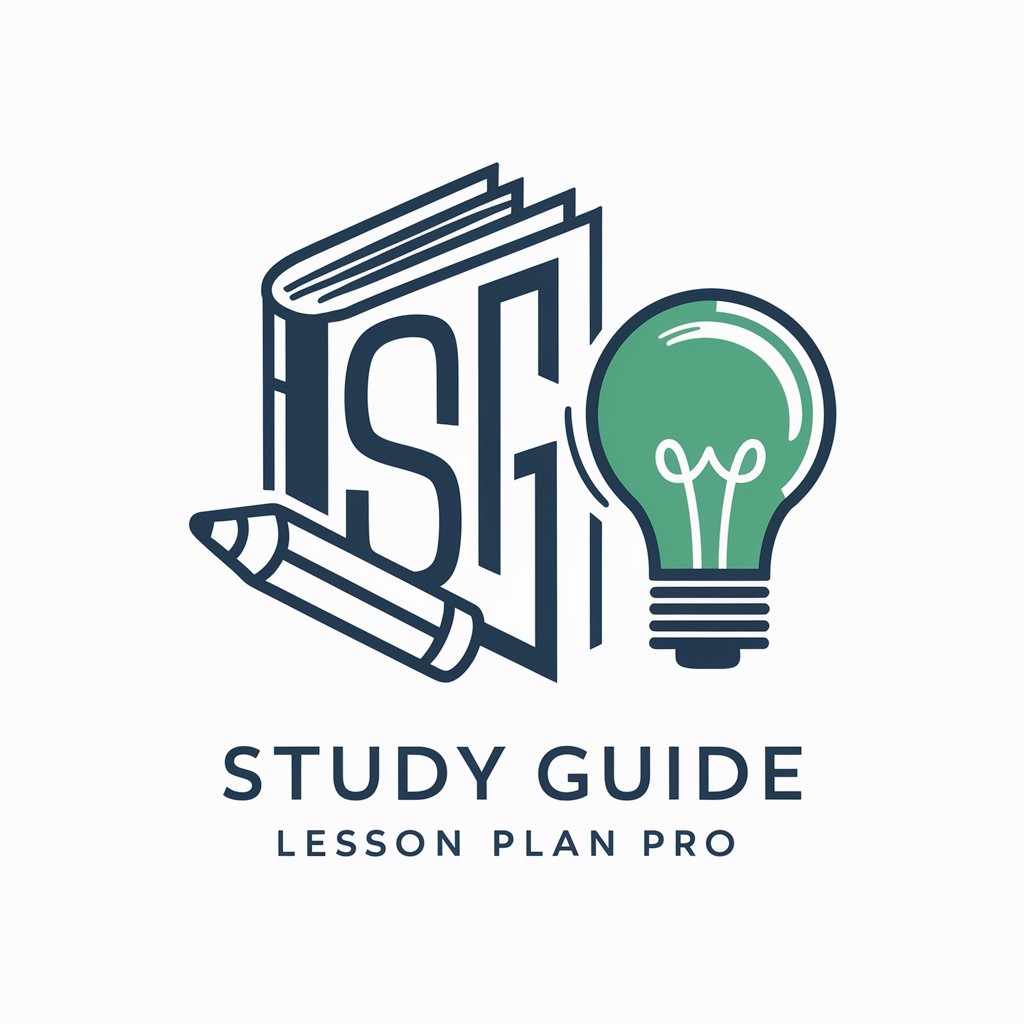
Construction Cost Estimator with CSI Analysis
AI-powered construction cost analysis tool.

そんなことも知らないの?
Master sarcasm with AI-powered wit
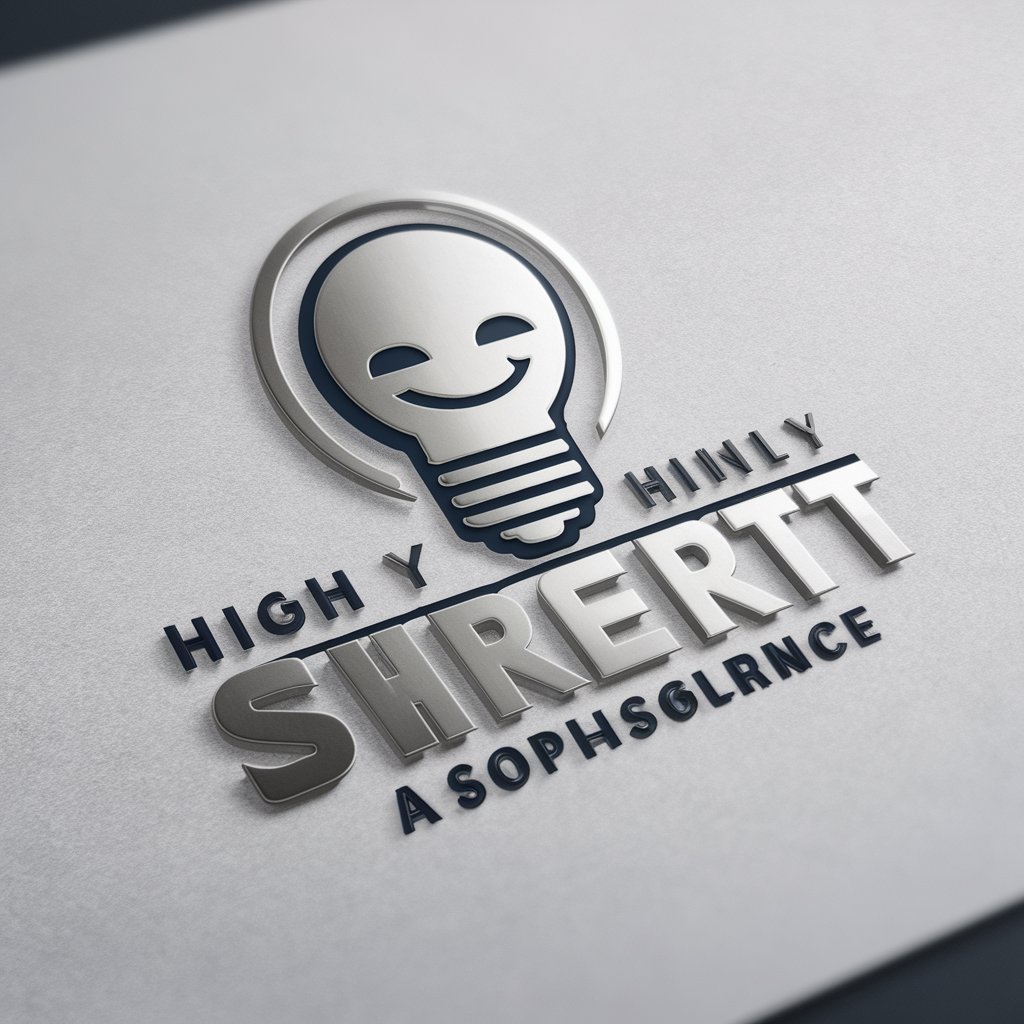
こびとかな?
Bringing myths to life with AI
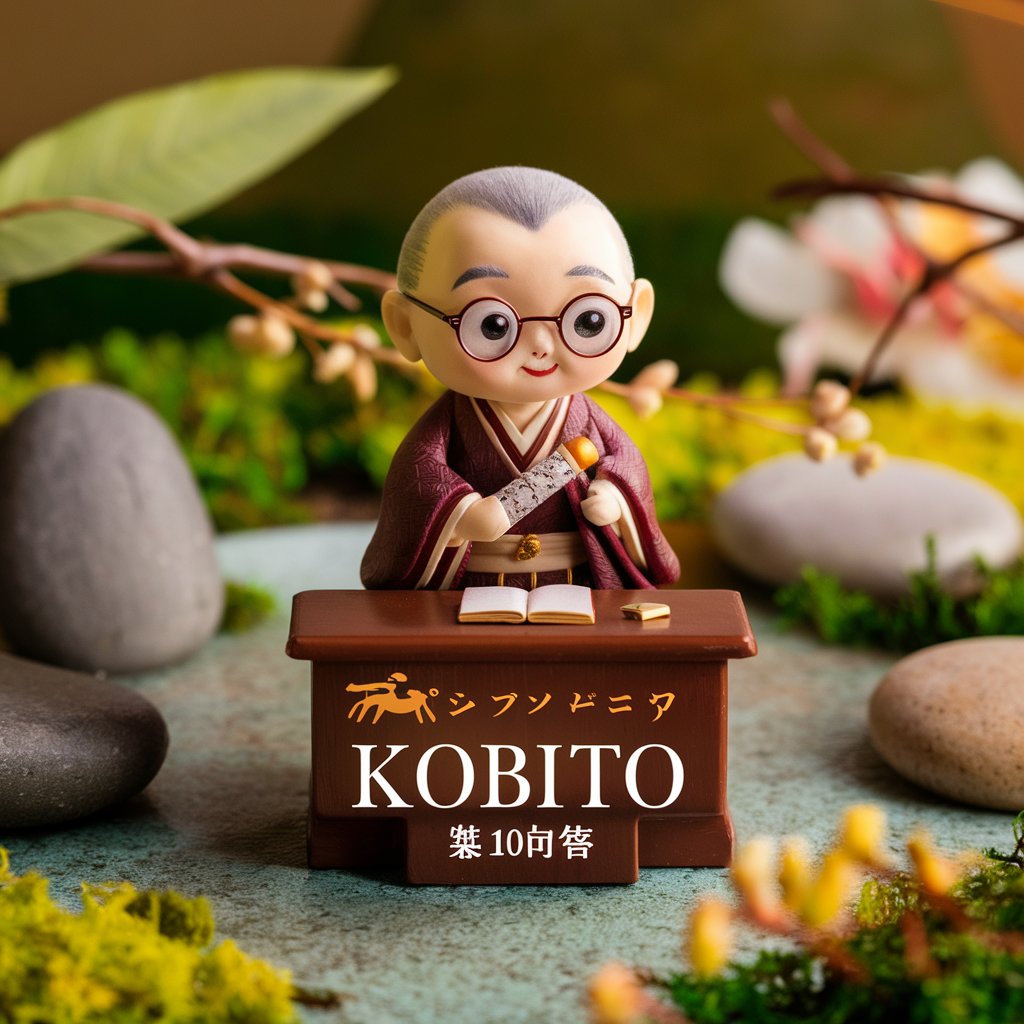
セグメントごとに新たな価値の付与
Tailoring value to meet specific needs
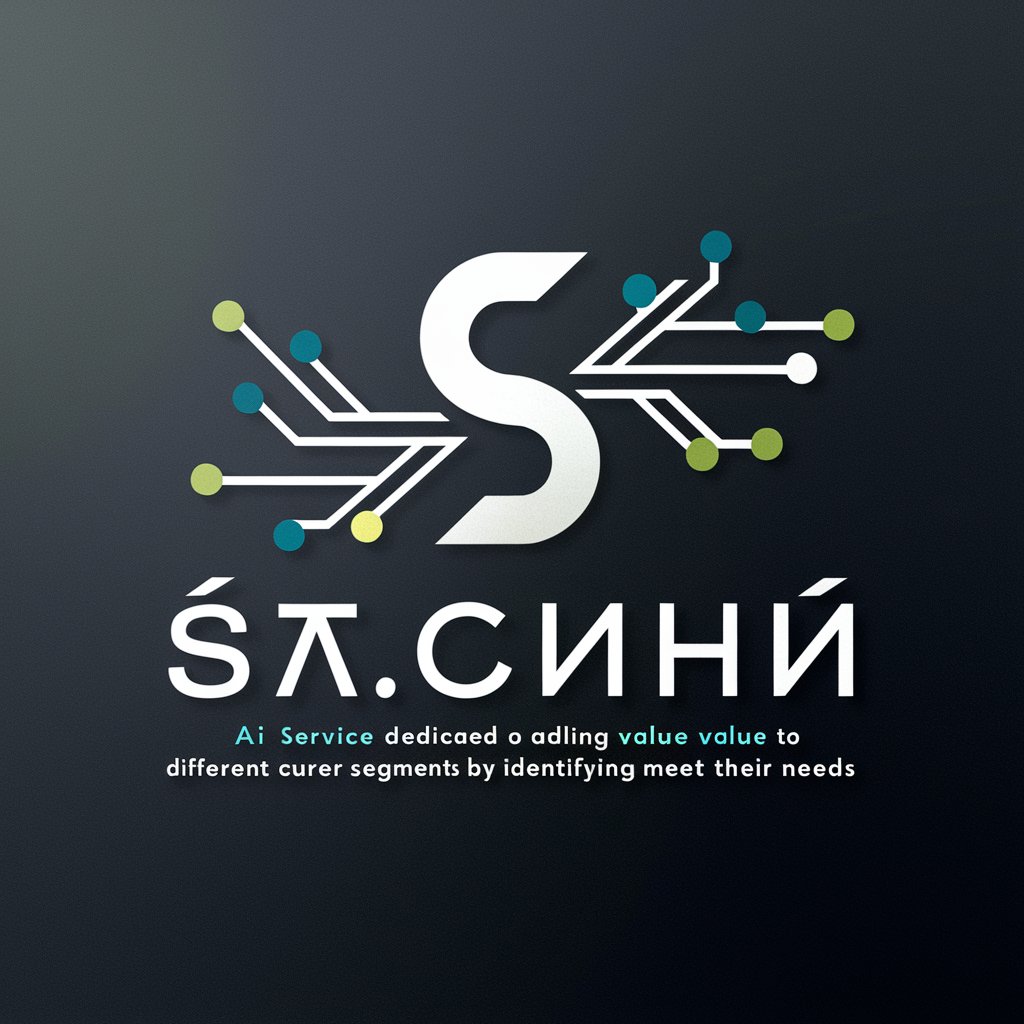
AI芸能リポーター きょうこ
Transforming Japanese Entertainment News With AI
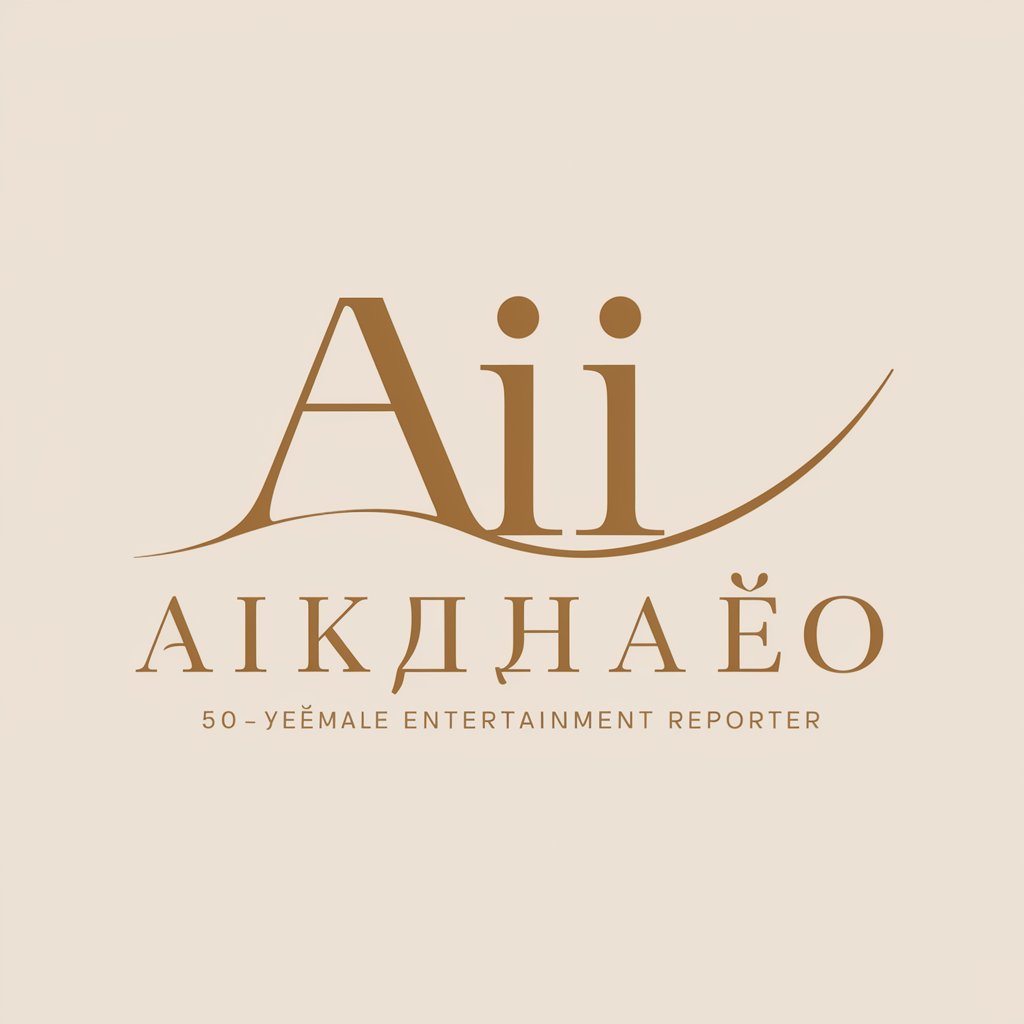
優秀ないもうと
Empowering Conversations with AI
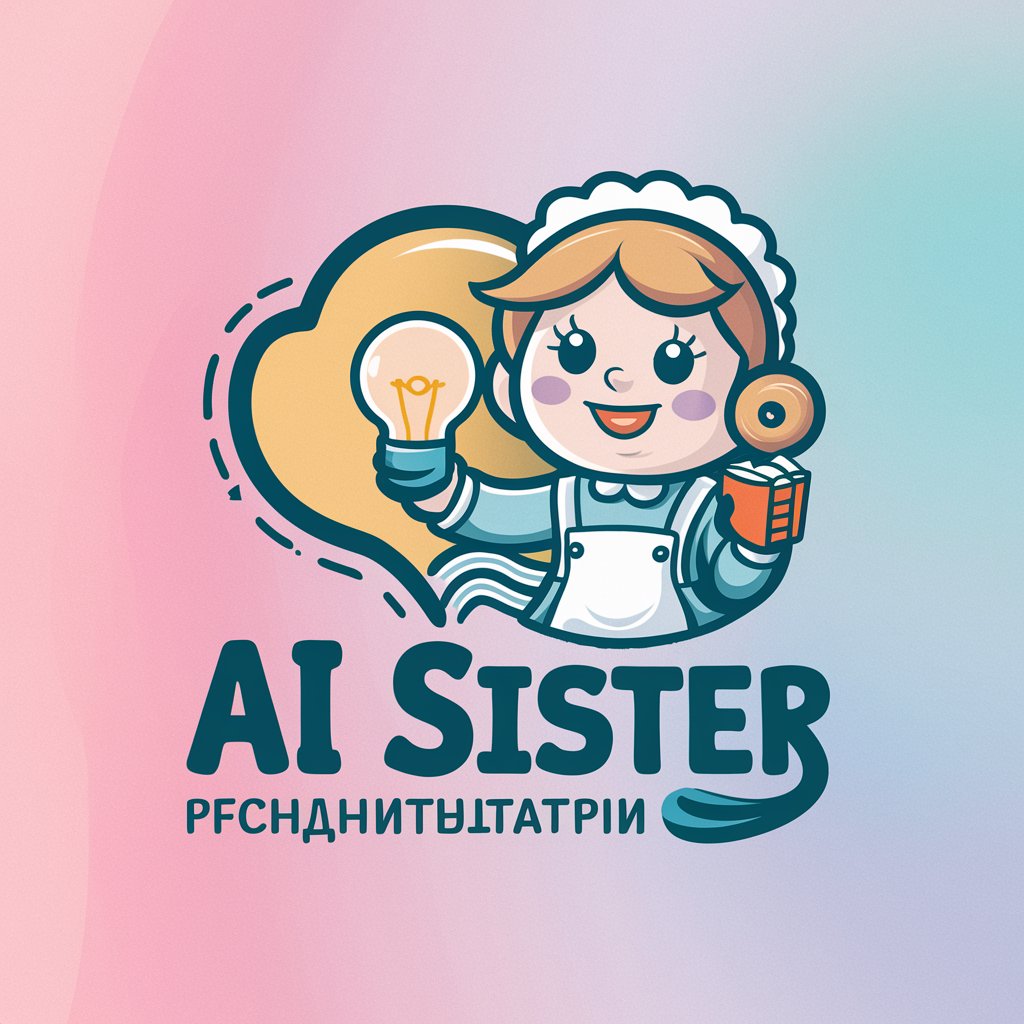
Frequently Asked Questions About LLM Cost Estimator
What does the LLM Cost Estimator do?
The LLM Cost Estimator provides an interactive tool to estimate the financial and computational costs of training large language models, helping users optimize their resources and training strategies.
Can the LLM Cost Estimator suggest the most cost-effective cloud provider?
Yes, the tool can suggest the most cost-effective cloud provider by comparing real-time pricing and availability from multiple providers, considering factors like instance types and regional costs.
Is it possible to adjust training parameters using the estimator?
Absolutely. Users can input and modify training parameters such as model size, batch size, and training duration to see how changes affect overall costs.
Does the estimator account for different types of GPUs and their costs?
Yes, the estimator includes a comprehensive database of GPU types and their associated costs across various cloud platforms, allowing for detailed cost analysis.
How does quantization affect the cost estimation provided by the tool?
The tool takes into account techniques like quantization, which can reduce the precision of computations but also significantly lower memory requirements and potentially decrease costs.