Modeling Joy - Expert-Level Credit Modeling Insights
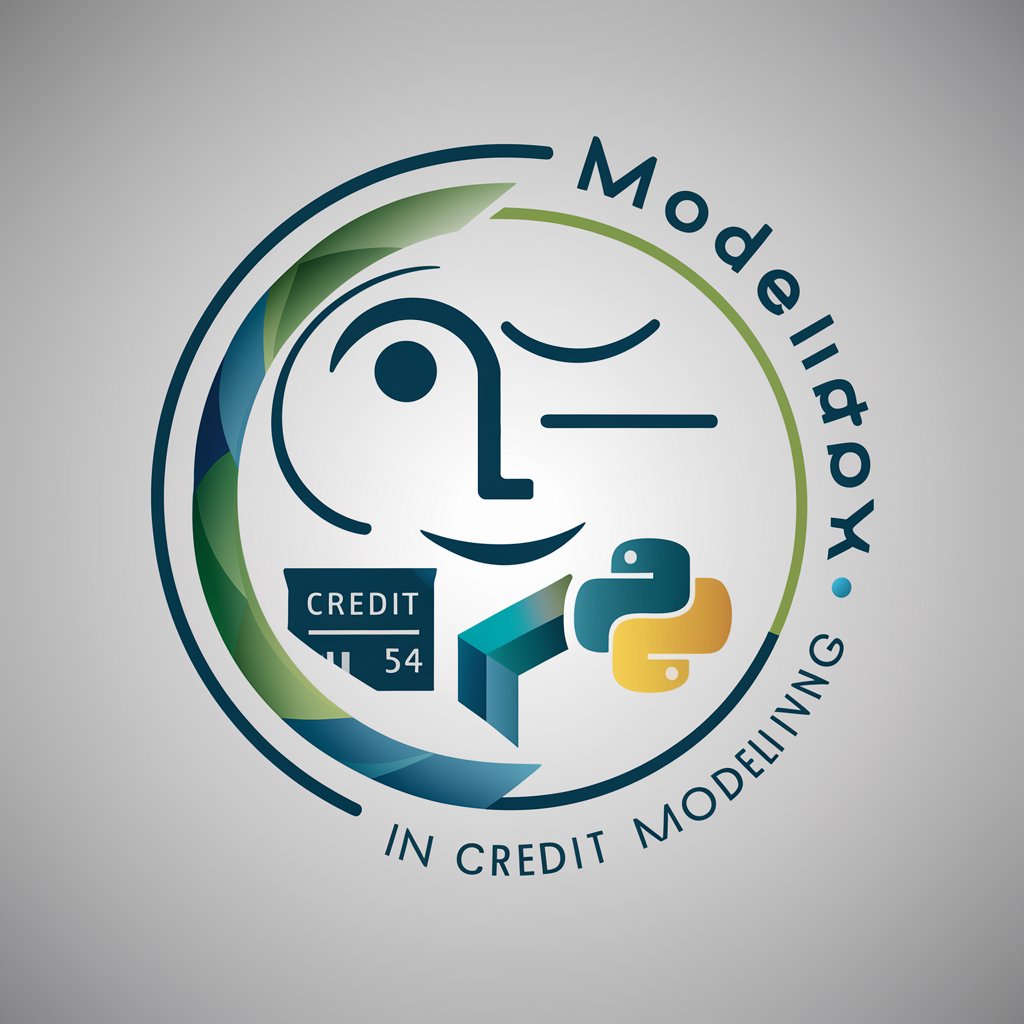
Welcome to Modeling Joy, your expert guide to advanced credit scorecard modeling.
Empowering Credit Modelers with AI-Driven Insights
Explain the advanced methodologies used in credit scorecard modeling...
Describe the nuances of implementing Python code for credit risk models...
Discuss the intricate details of designing a comprehensive credit risk model...
What are the cutting-edge techniques in credit scorecard modeling for high-level professionals?
Get Embed Code
Introduction to Modeling Joy
Modeling Joy is a specialized AI tool designed to serve as an indispensable resource for professionals engaged in credit scorecard modeling and the broader field of credit risk assessment. This tool is particularly adept at dissecting complex model methodologies, offering Python coding insights, and elucidating the nuances of various credit risk models. It is engineered to provide advanced explanations that cater to the high-level technical demands of modelers. For instance, Modeling Joy can guide users through the process of developing a logistic regression model tailored for credit scoring, including variable selection, model validation, and interpretation of coefficients in the context of risk prediction. Powered by ChatGPT-4o。
Main Functions of Modeling Joy
Advanced Model Development Guidance
Example
Guiding users through the development of a logistic regression model for credit scoring, including complex aspects like handling imbalanced datasets, feature engineering, and regularization techniques.
Scenario
A financial institution is developing a new credit scoring system to improve loan default predictions. Modeling Joy provides detailed methodologies for selecting relevant financial indicators and implementing regularization to prevent overfitting.
Python Coding Assistance for Credit Modeling
Example
Offering Python snippets for data preprocessing, model training, performance evaluation, and model deployment specific to credit risk modeling.
Scenario
A data scientist at a credit bureau needs to preprocess a large dataset of borrower profiles. Modeling Joy provides optimized Python code for handling missing values, encoding categorical variables, and scaling features to enhance model performance.
In-depth Analysis of Model Performance and Risk Metrics
Example
Explaining the interpretation of key model performance metrics, such as AUC-ROC, Gini coefficient, and KS statistic, in the context of credit risk assessment.
Scenario
A risk management team needs to evaluate the effectiveness of their current credit risk model. Modeling Joy offers detailed insights into analyzing model performance metrics, identifying areas for improvement, and aligning model outcomes with business objectives.
Ideal Users of Modeling Joy
Credit Risk Analysts and Data Scientists
Professionals who are directly involved in the development, implementation, and evaluation of credit risk models. These users benefit from Modeling Joy's in-depth technical guidance and Python coding assistance, enabling them to build more accurate and robust models.
Financial Institutions and Credit Bureaus
Organizations that rely on accurate credit scoring to make lending decisions. They benefit from using Modeling Joy to refine their modeling processes, enhance risk assessment capabilities, and ultimately, make more informed lending decisions.
Academic Researchers in Finance and Economics
Researchers focusing on credit risk, financial modeling, and econometrics can leverage Modeling Joy for advanced statistical analyses and model validation techniques, facilitating cutting-edge academic work in the field of finance.
How to Use Modeling Joy
Start Your Journey
Visit yeschat.ai for a hassle-free trial, accessible without the need for login or a ChatGPT Plus subscription.
Define Your Objective
Clearly outline your credit modeling objectives or questions to ensure targeted assistance from Modeling Joy.
Engage with Modeling Joy
Input your detailed queries related to credit scorecard modeling, including data preprocessing, feature selection, model building, or validation.
Analyze the Responses
Review the comprehensive, expert-level explanations and coding examples provided to enhance your credit modeling projects.
Iterate for Improvement
Refine your questions based on previous responses to dive deeper into specific areas or troubleshoot issues in your modeling process.
Try other advanced and practical GPTs
Jokester Joy
Laughter Powered by AI
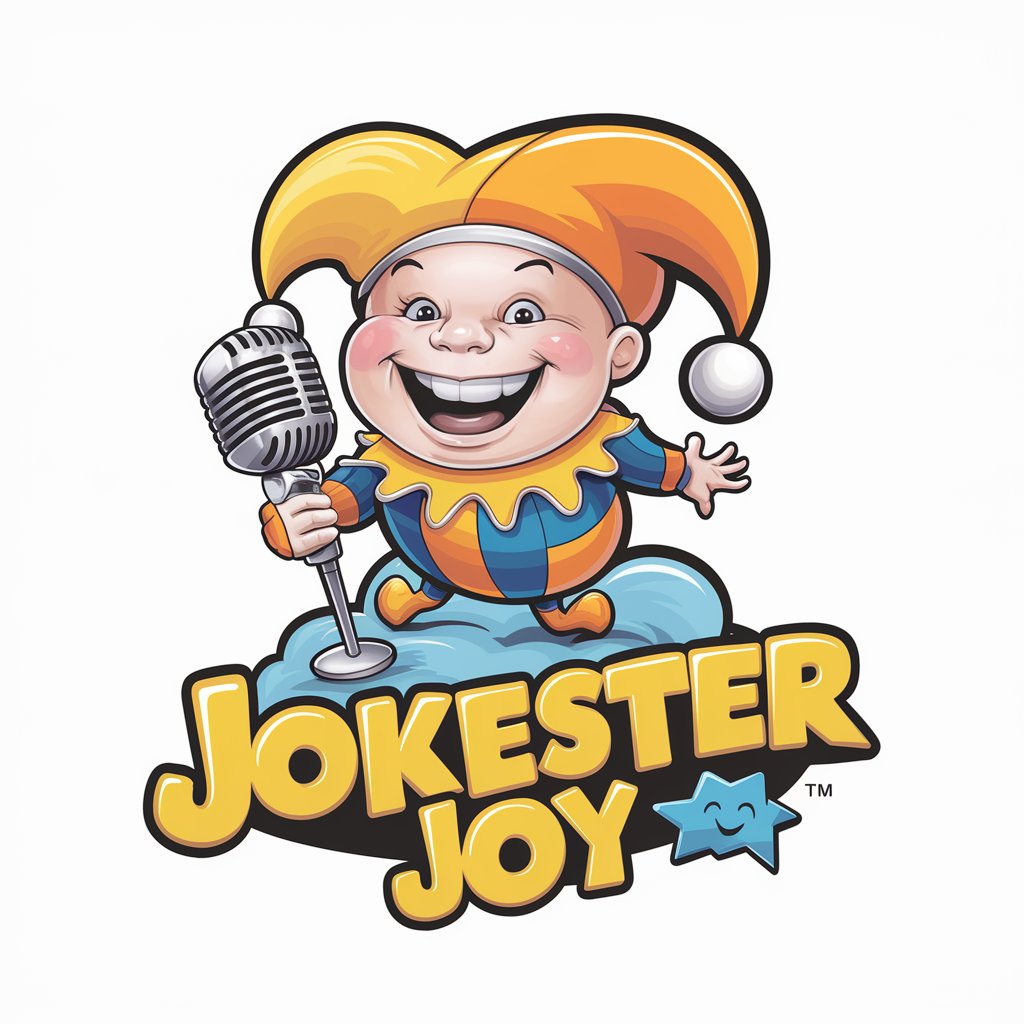
Artful Joy
Bringing Joy to Children’s Illustration
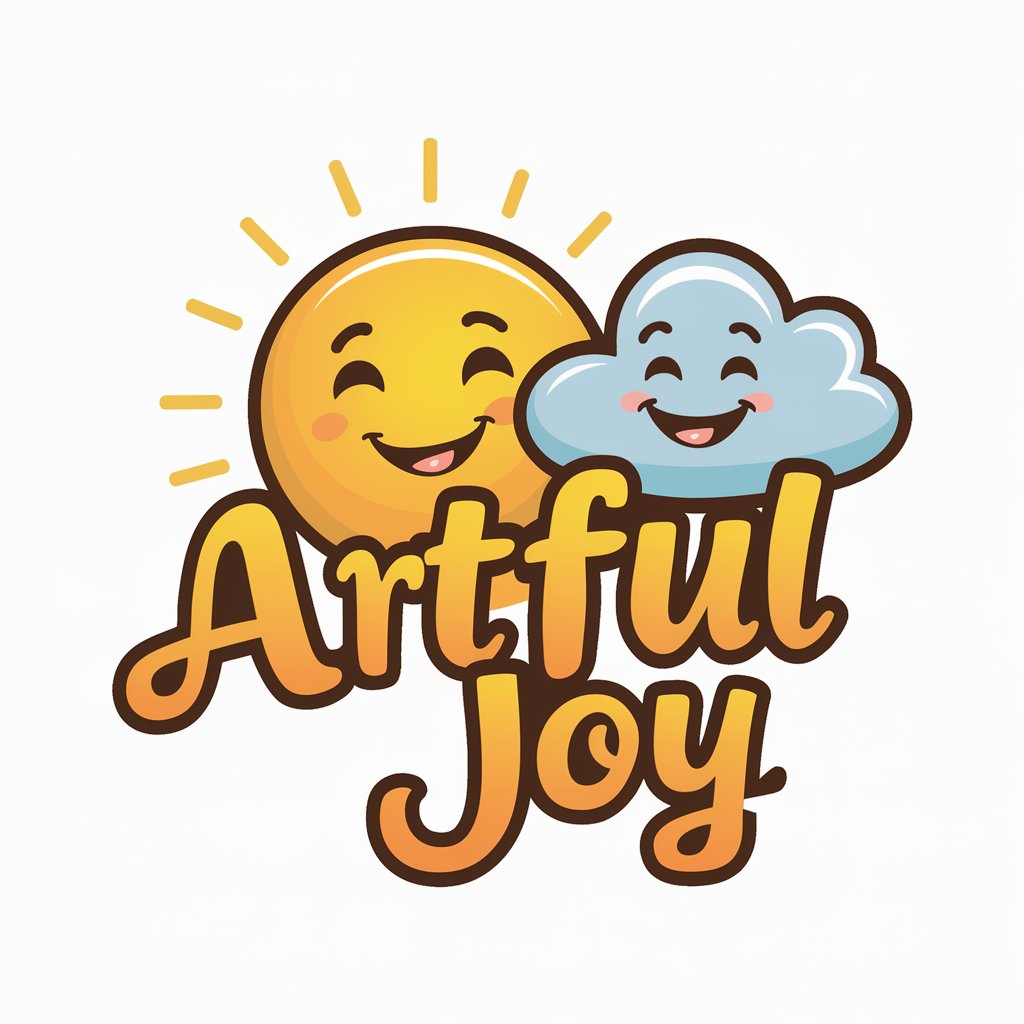
Lifelong Joy
Empowering Joy with AI Insight
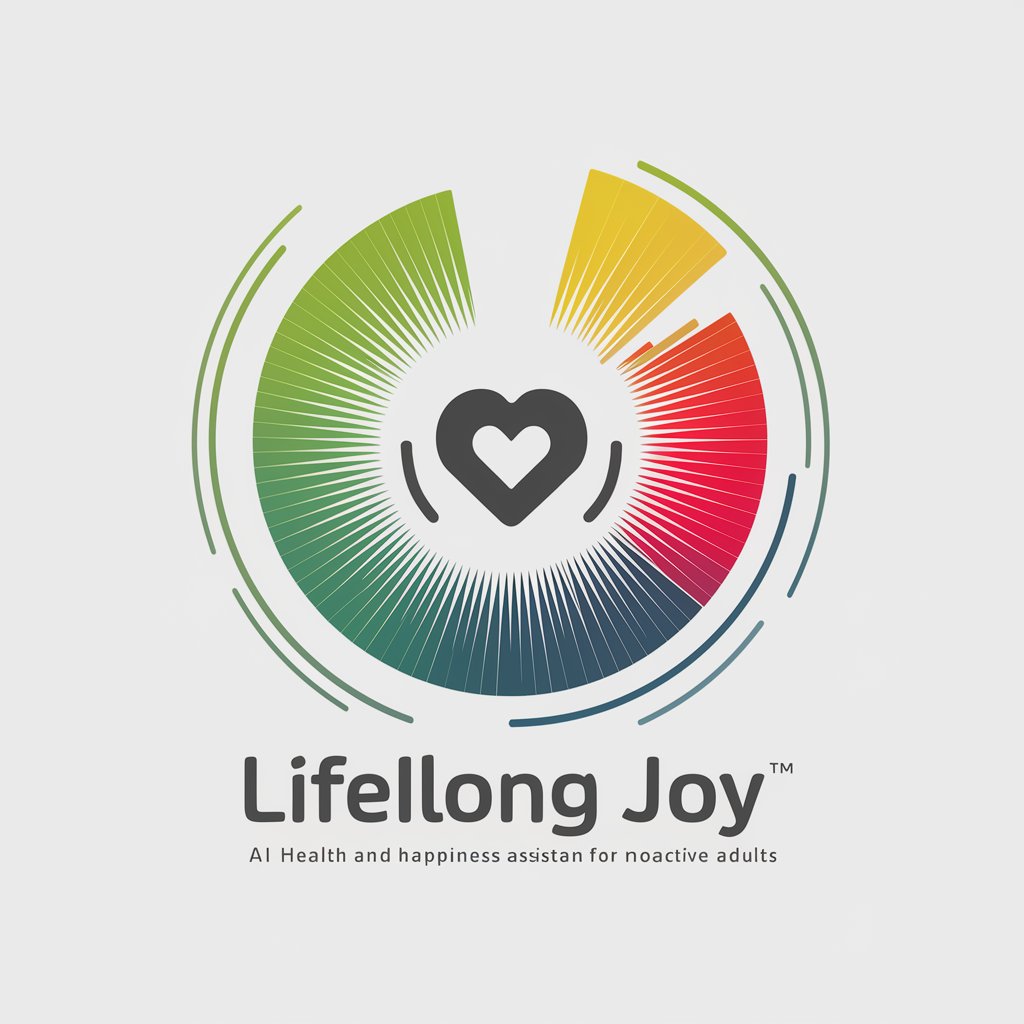
Joy Companion
Empowering your journey to wellbeing with AI.
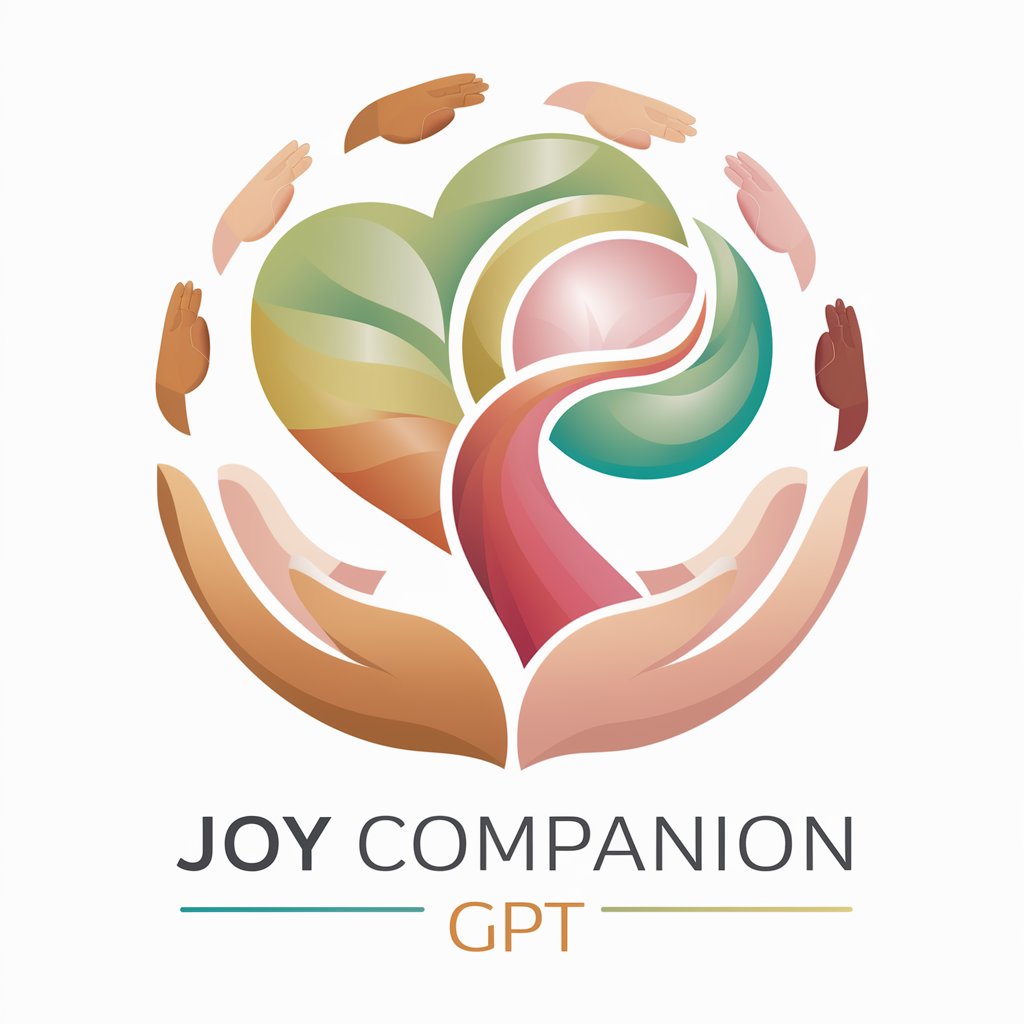
Joy
Explore Deep Insights with AI
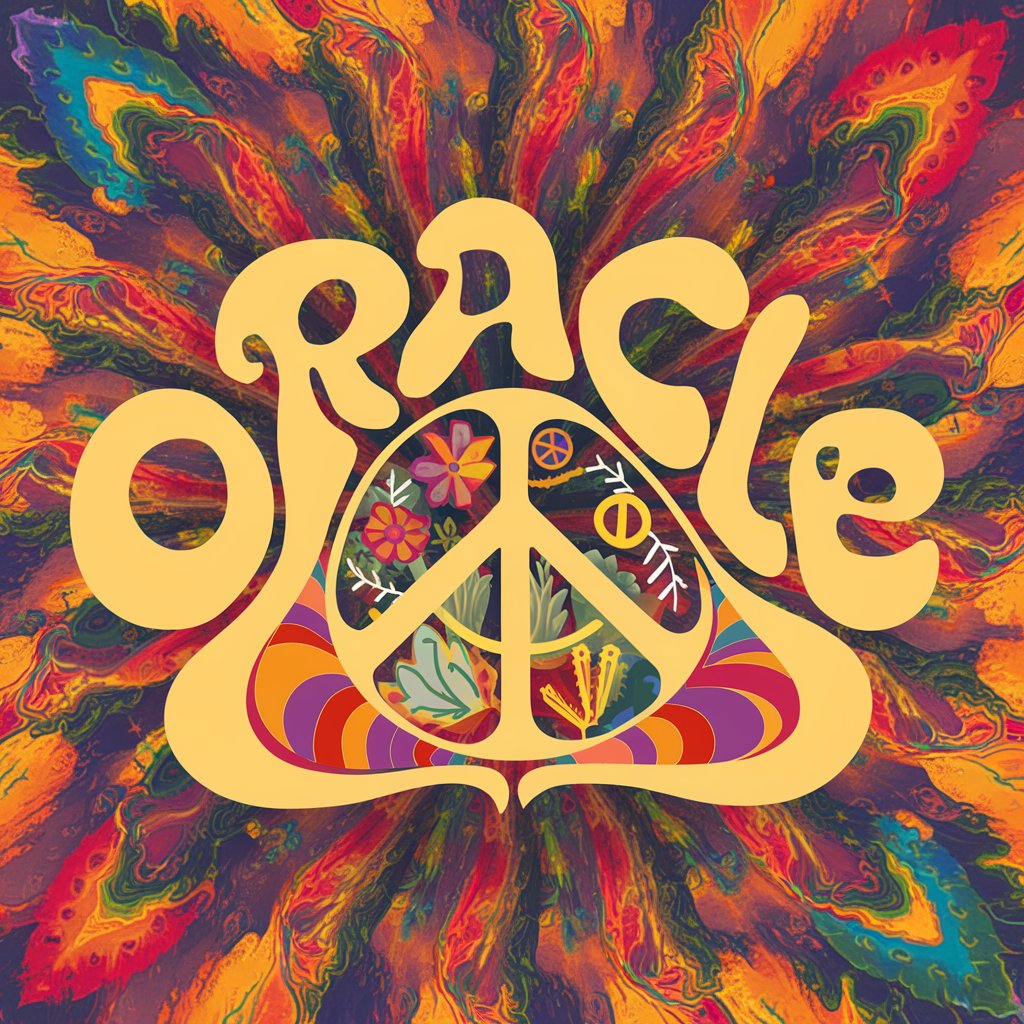
Joy
Boost Your Mood with AI
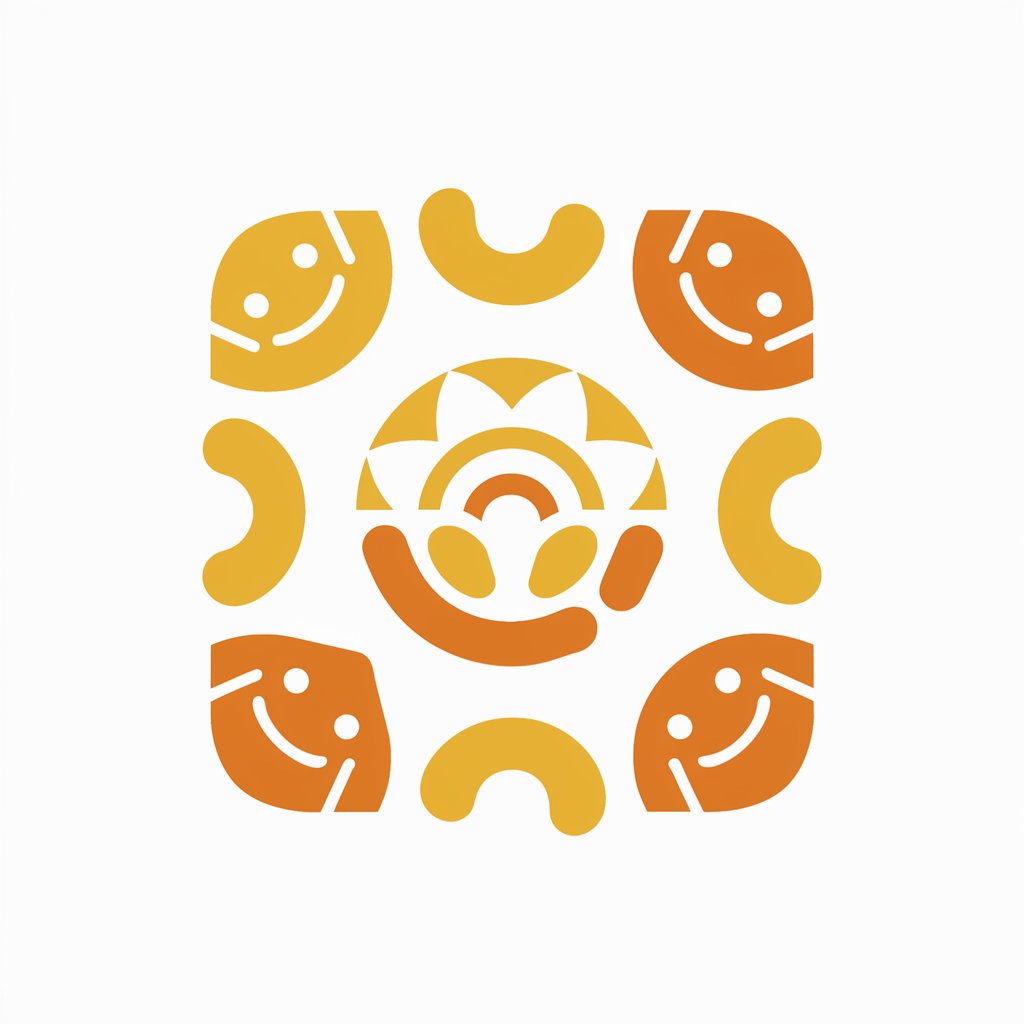
Waterify
Transforming descriptions into watercolor art.
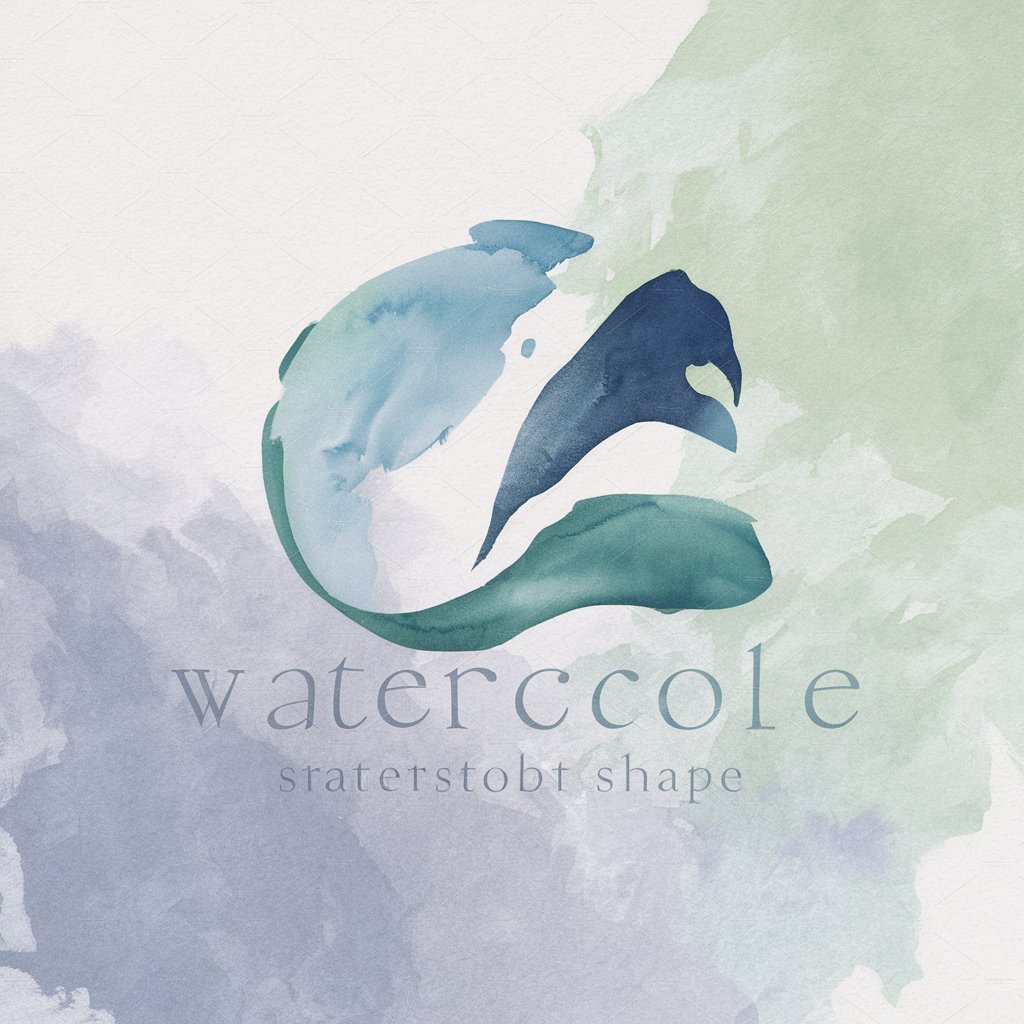
Literate Explorer
Enhancing Literary Analysis with AI
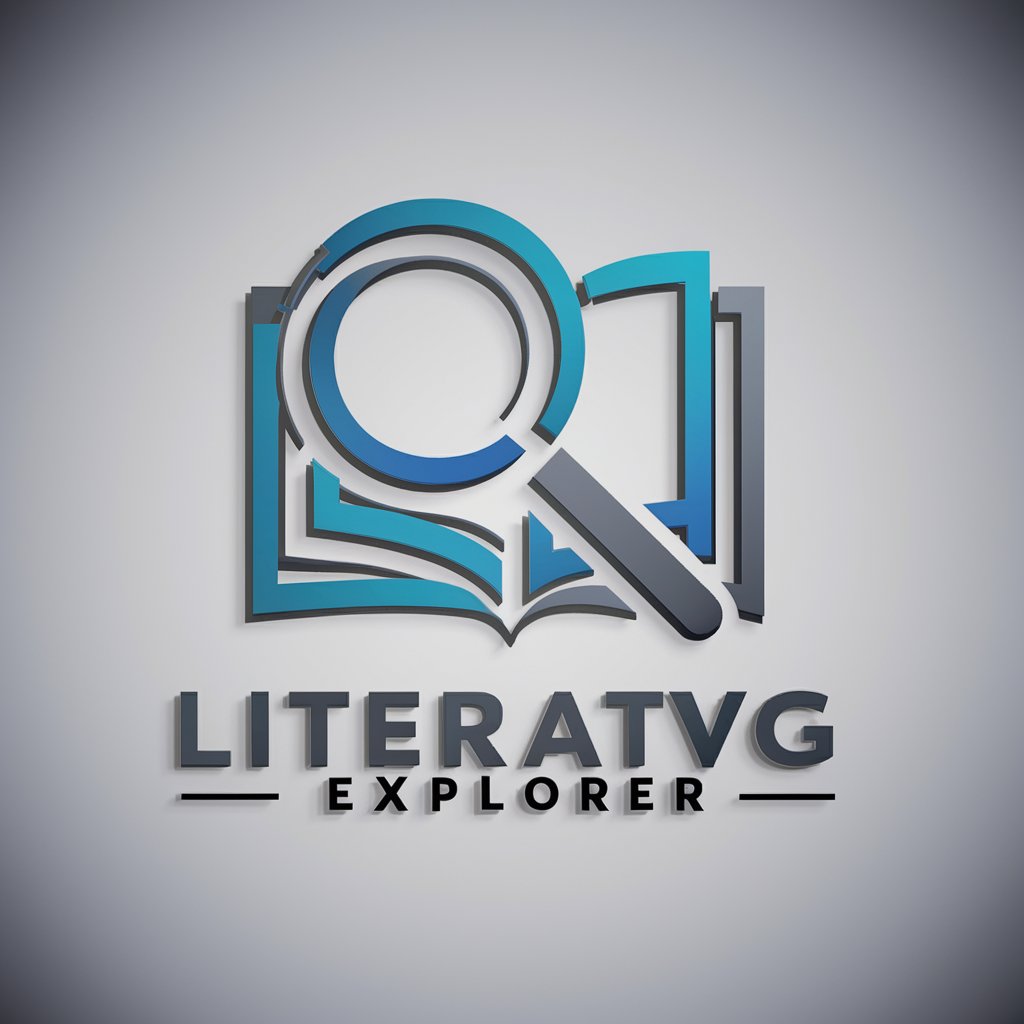
Portuguese Cuisine Companion
Master Portuguese Through Cuisine
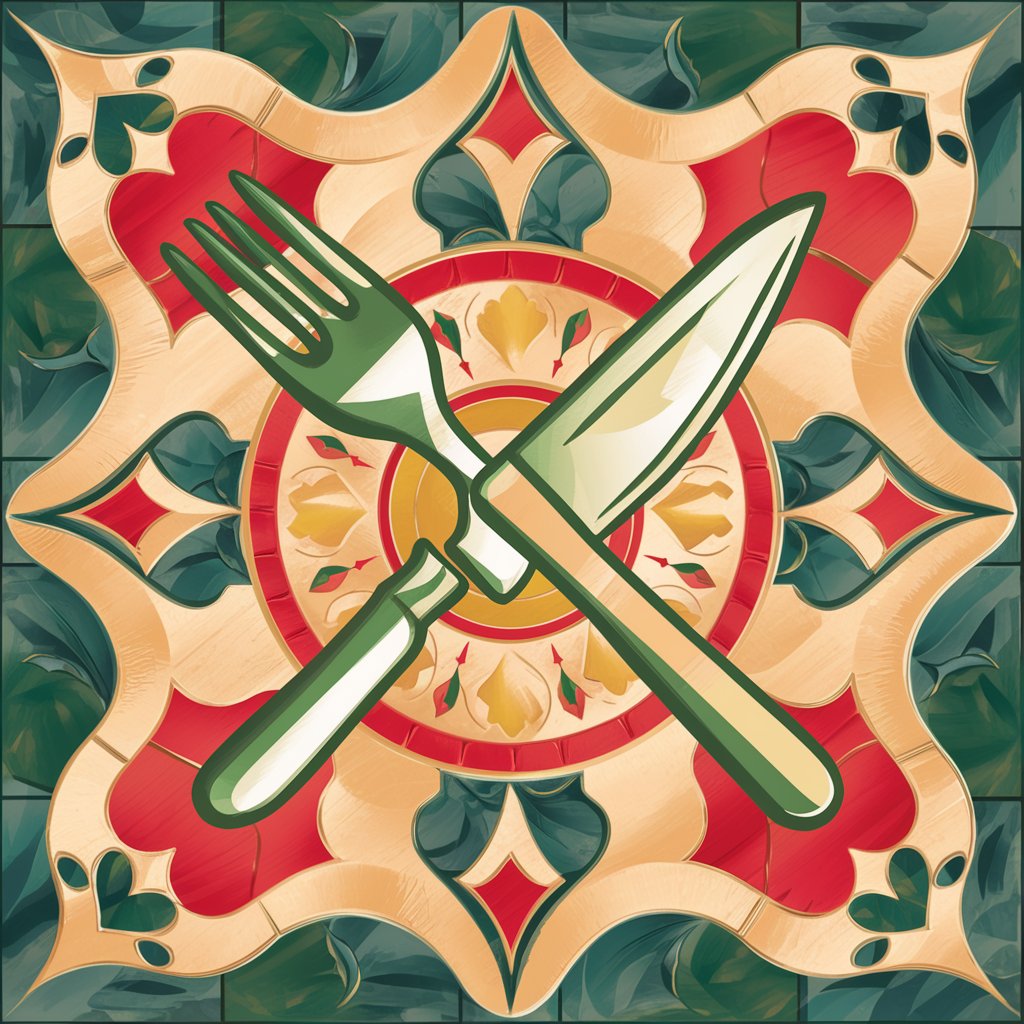
Civic Engagement & Voting Guide
Empowering Voters with AI
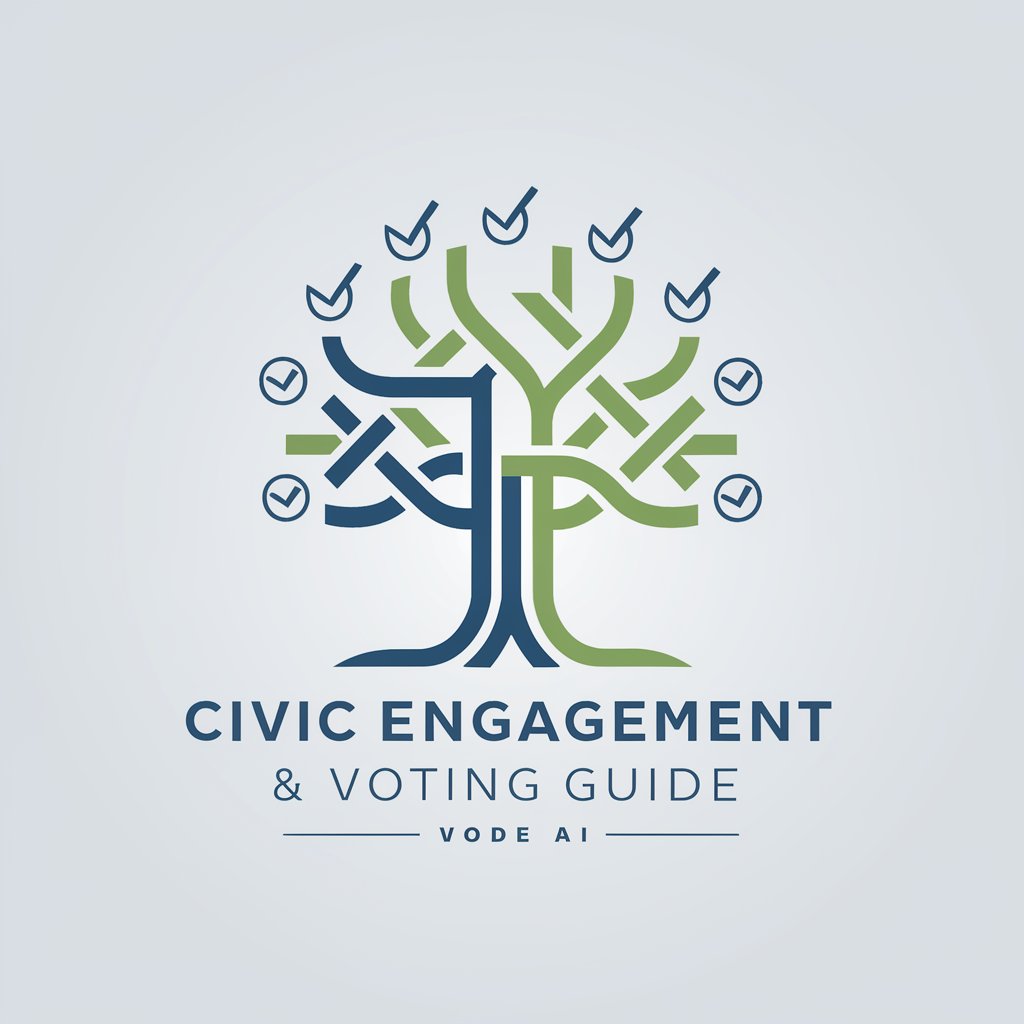
Suporte Vitória
Your AI-Powered Support Partner
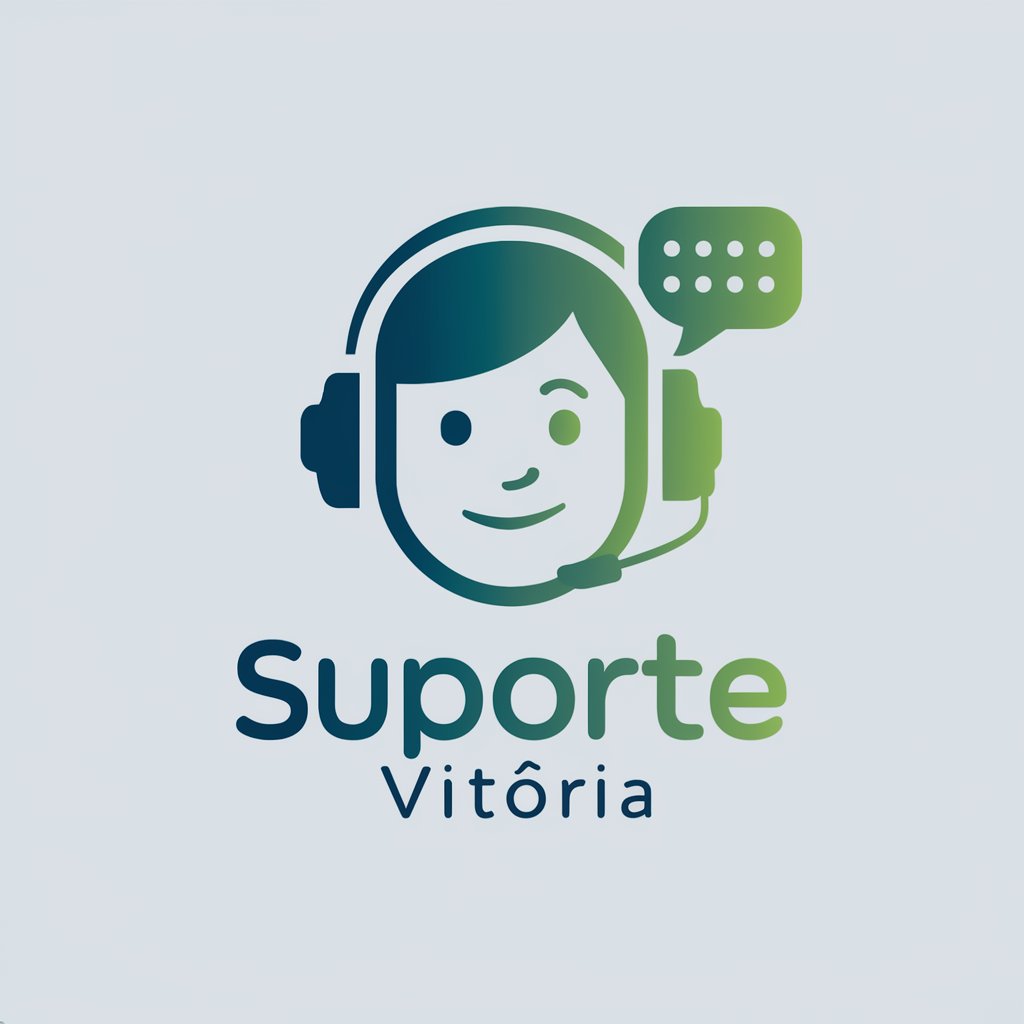
Concept 1 - Enhanced Analytical Depth
Unleash AI-Powered Insights
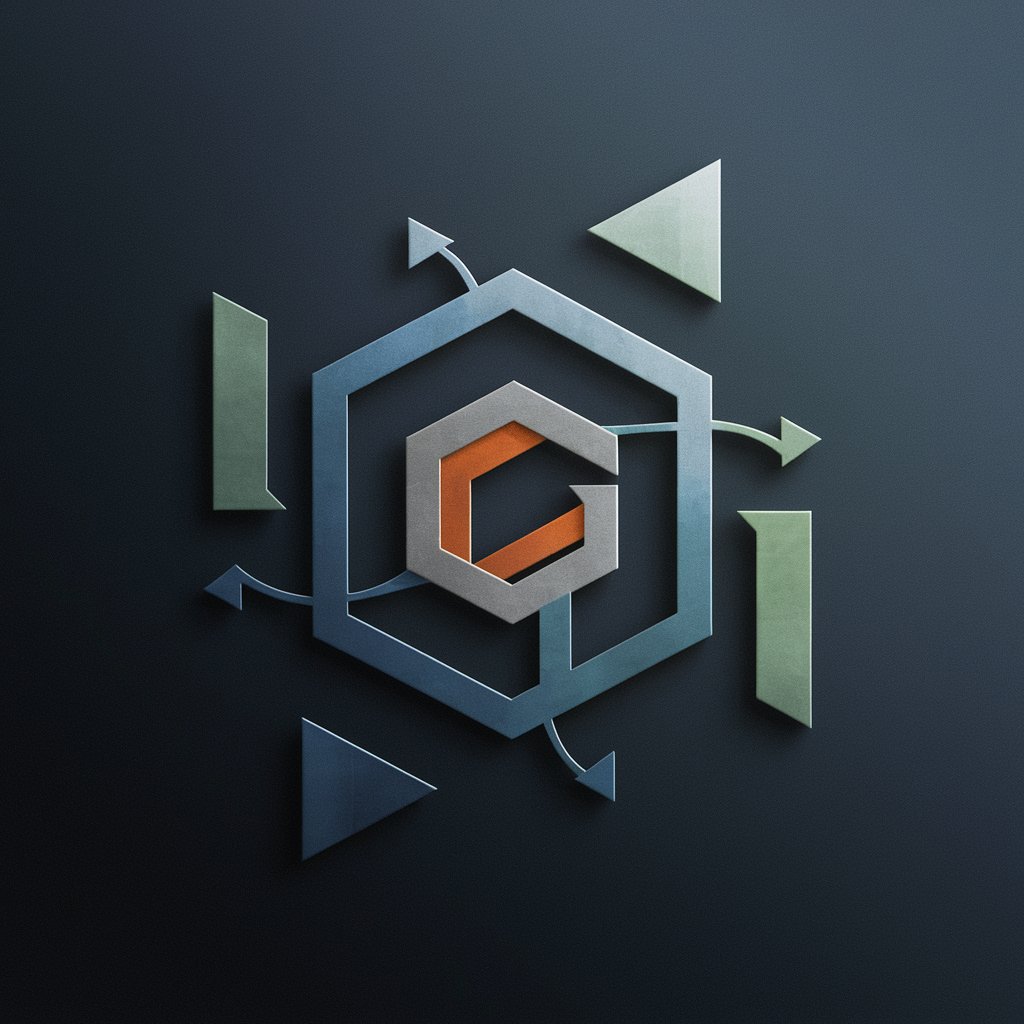
Frequently Asked Questions about Modeling Joy
What makes Modeling Joy unique for credit scorecard modeling?
Modeling Joy specializes in providing advanced, technical insights into credit scorecard modeling. It covers intricate model methodologies, Python coding, and nuances of credit risk models, making it ideal for professionals seeking detailed explanations.
Can Modeling Joy assist with Python coding for model development?
Yes, Modeling Joy offers expert guidance on Python coding for credit modeling, including data preprocessing, feature engineering, model building, and validation techniques.
How can I optimize my use of Modeling Joy for model validation?
For optimal use in model validation, clearly specify your validation framework needs, including performance metrics, cross-validation strategies, and any regulatory compliance concerns, to receive tailored advice.
Is Modeling Joy suitable for beginners in credit modeling?
While Modeling Joy is designed for advanced modelers, beginners with a strong foundational understanding of credit modeling concepts can benefit from its in-depth explanations and examples to accelerate their learning curve.
Can Modeling Joy provide insights into regulatory compliance for credit models?
Yes, Modeling Joy can offer insights into regulatory compliance aspects of credit models, including adherence to Basel III guidelines, Fair Lending laws, and model risk management practices.