Regression Pro - Efficient Regression Analysis
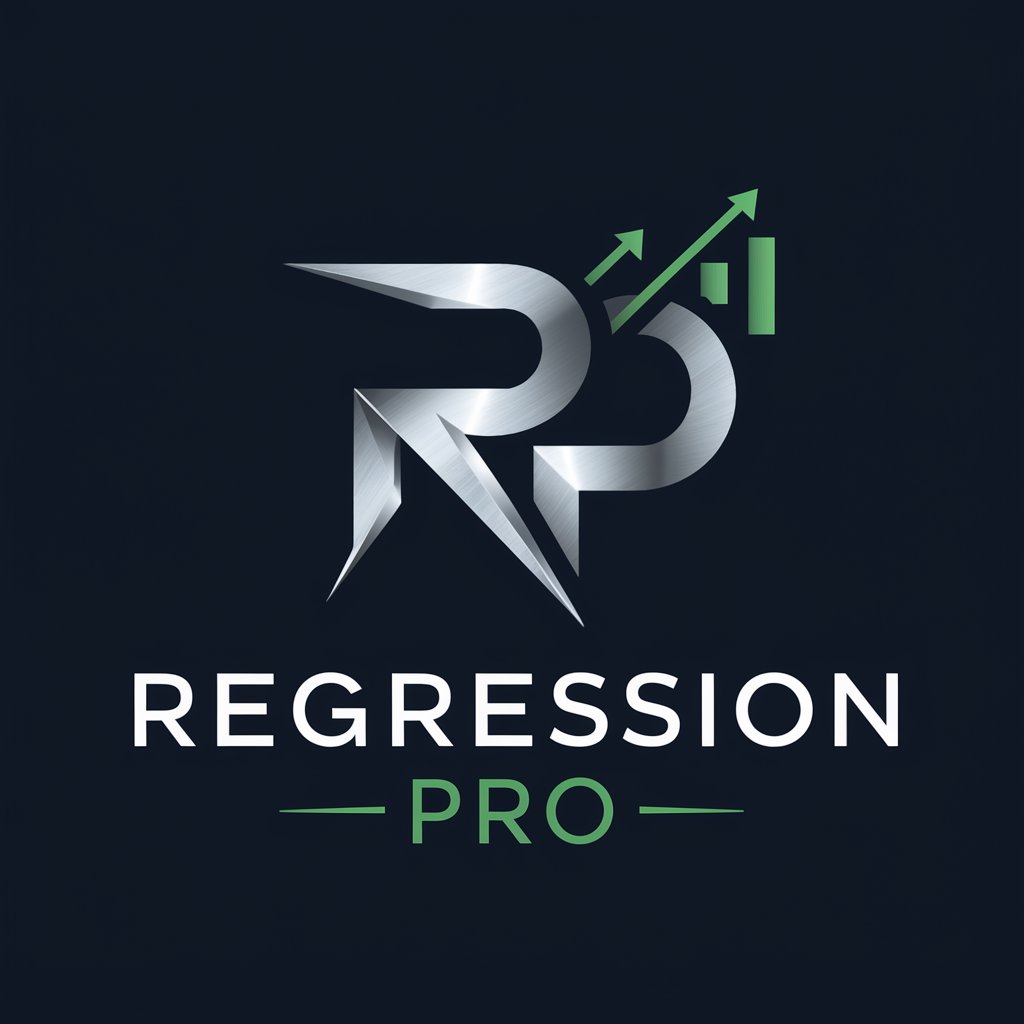
Hello! Let's dive into creating your regression model.
Empower Decisions with AI-Powered Regression
Start by uploading your dataset.
Identify your target variable for prediction.
Select appropriate predictor variables for your regression.
Review and clean your data before model training.
Get Embed Code
Introduction to Regression Pro
Regression Pro is a specialized AI-driven tool designed to assist users in developing, training, and evaluating regression models based on their specific datasets. It is tailored for simplifying complex statistical analysis tasks into more manageable and user-friendly processes. This tool is adept at handling various regression tasks, including linear and logistic regressions, depending on the nature of the target variable. For instance, if a user aims to predict a continuous outcome like house prices based on features like size and location, Regression Pro would guide through linear regression. Conversely, for binary outcomes like whether an email is spam, it would suggest logistic regression. The tool's design purpose revolves around enabling users, even those with limited statistical background, to apply regression analysis effectively by guiding them through dataset preparation, variable selection, model training, evaluation, and interpretation of results with illustrative examples such as optimizing marketing strategies or predicting healthcare outcomes. Powered by ChatGPT-4o。
Main Functions of Regression Pro
Dataset Analysis and Preparation
Example
A user uploads a dataset containing real estate listings. Regression Pro analyzes it to provide a summary of variables, such as average house price, number of bedrooms, and location types.
Scenario
This function is crucial in the initial stages where users need to understand their data's structure and quality. It ensures that datasets are ready for model training by identifying any missing values, outliers, or irrelevant variables that might skew the results.
Variable Selection Guidance
Example
For a project aiming to predict customer churn, Regression Pro suggests variables like subscription length, service usage frequency, and customer feedback scores as predictors.
Scenario
This function helps users identify which variables might have significant predictive power for their specific target outcome. It aids in constructing a more accurate and reliable regression model by focusing on relevant predictors and excluding noise.
Model Training and Validation
Example
Using a dataset from a healthcare study, Regression Pro trains a logistic regression model to predict patient readmission risks based on variables like age, treatment received, and initial health assessments.
Scenario
This is the core function where the tool applies statistical techniques to learn the relationships between the target variable and predictors. It includes partitioning the dataset into training and validation sets to ensure the model's performance is robust and not just memorizing the training data.
Evaluation and Result Interpretation
Example
After training a model to forecast sales based on advertising spend across different media, Regression Pro evaluates the model's accuracy and presents the impact of each media type on sales through easy-to-understand visualizations.
Scenario
Post-training, this function helps users understand the model's effectiveness and the significance of each predictor variable. It's vital for translating complex model outputs into actionable insights, like allocating budgets more efficiently in marketing strategies.
Ideal Users of Regression Pro Services
Business Analysts
Business analysts working in sectors like finance, marketing, and sales can leverage Regression Pro to uncover insights within their data, predict future trends, and make data-driven decisions to drive business growth.
Academic Researchers
Researchers in fields such as economics, psychology, and environmental science can use Regression Pro to analyze experimental or observational data, test hypotheses, and contribute new knowledge to their respective fields.
Data Science Enthusiasts
Individuals looking to learn or improve their data science skills can benefit from Regression Pro's guided approach to regression analysis, helping them to understand complex statistical concepts through practical application.
Healthcare Professionals
Healthcare professionals and epidemiologists can use Regression Pro to model clinical outcomes based on patient data, thereby improving treatment plans and understanding risk factors for diseases more effectively.
How to Use Regression Pro
1
Initiate your data analysis journey at yeschat.ai for an immediate start, sans the need for sign-up or ChatGPT Plus.
2
Upload your dataset. Ensure your data is clean and formatted correctly (e.g., CSV) to facilitate a smooth analysis process.
3
Select your target variable. Decide on the outcome you're interested in predicting based on your dataset's features.
4
Choose your predictor variables. Identify which variables in your dataset you believe could influence your target variable.
5
Evaluate your model's performance. After the model is trained, review the provided metrics and visualizations to understand the impact of your predictors.
Try other advanced and practical GPTs
Trener Regresu Osobistego
Automating the Art of Giving Up
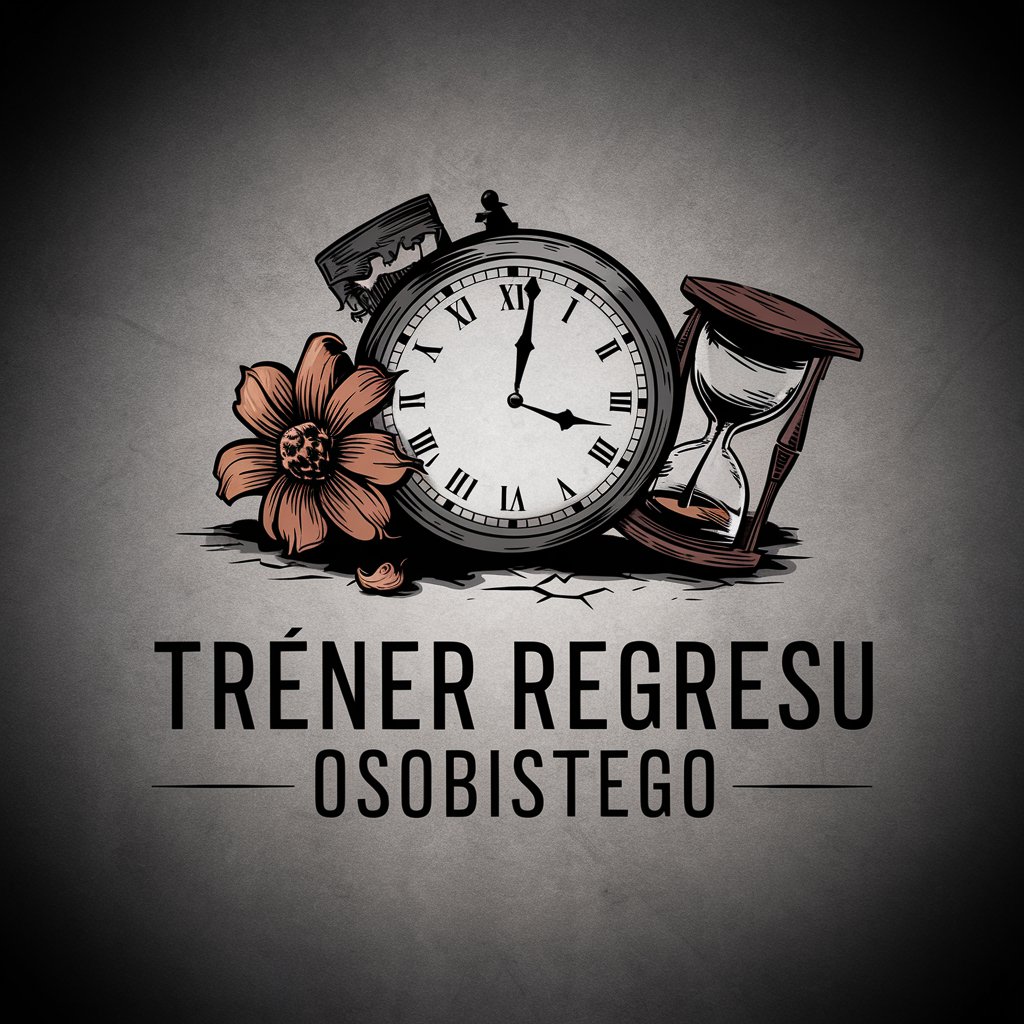
nO rEGRETs
Automate your project planning effortlessly
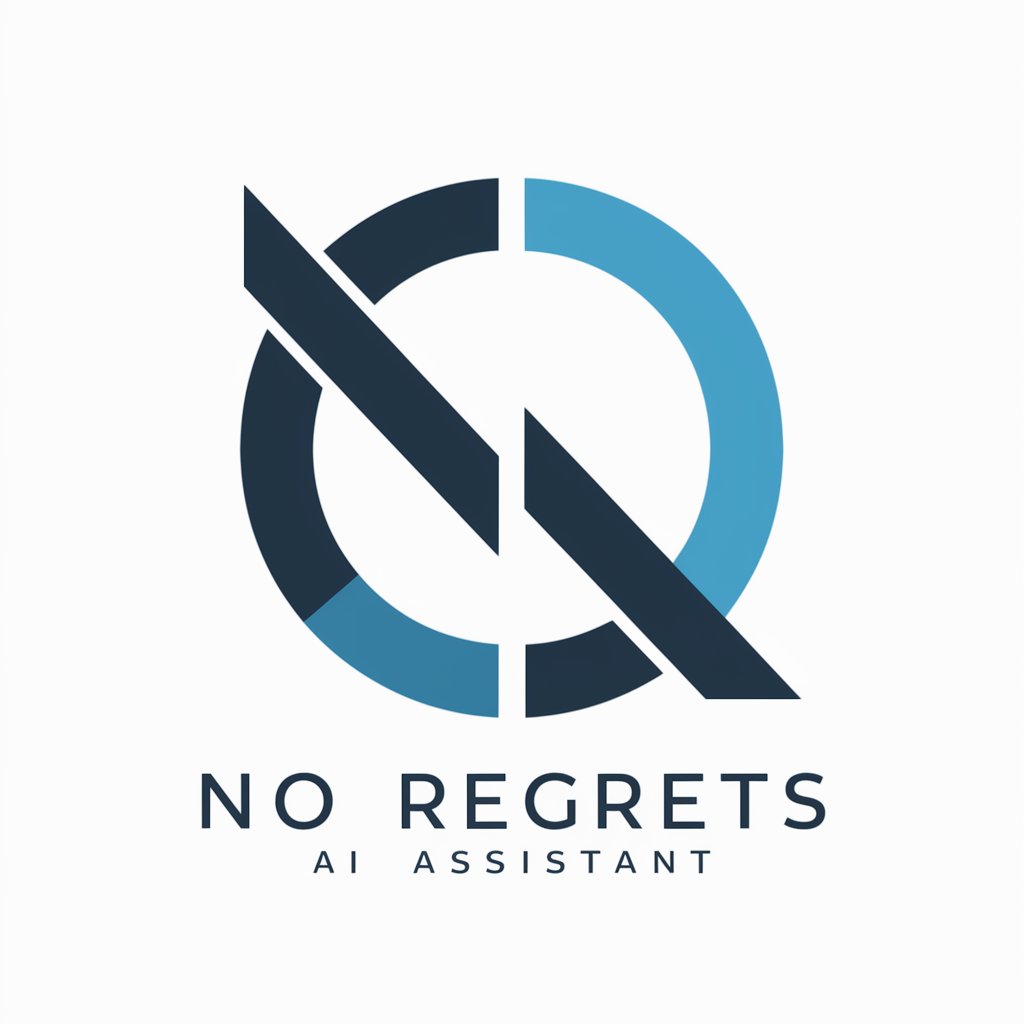
Wall Street Regrets
AI-powered investment insights at your fingertips.
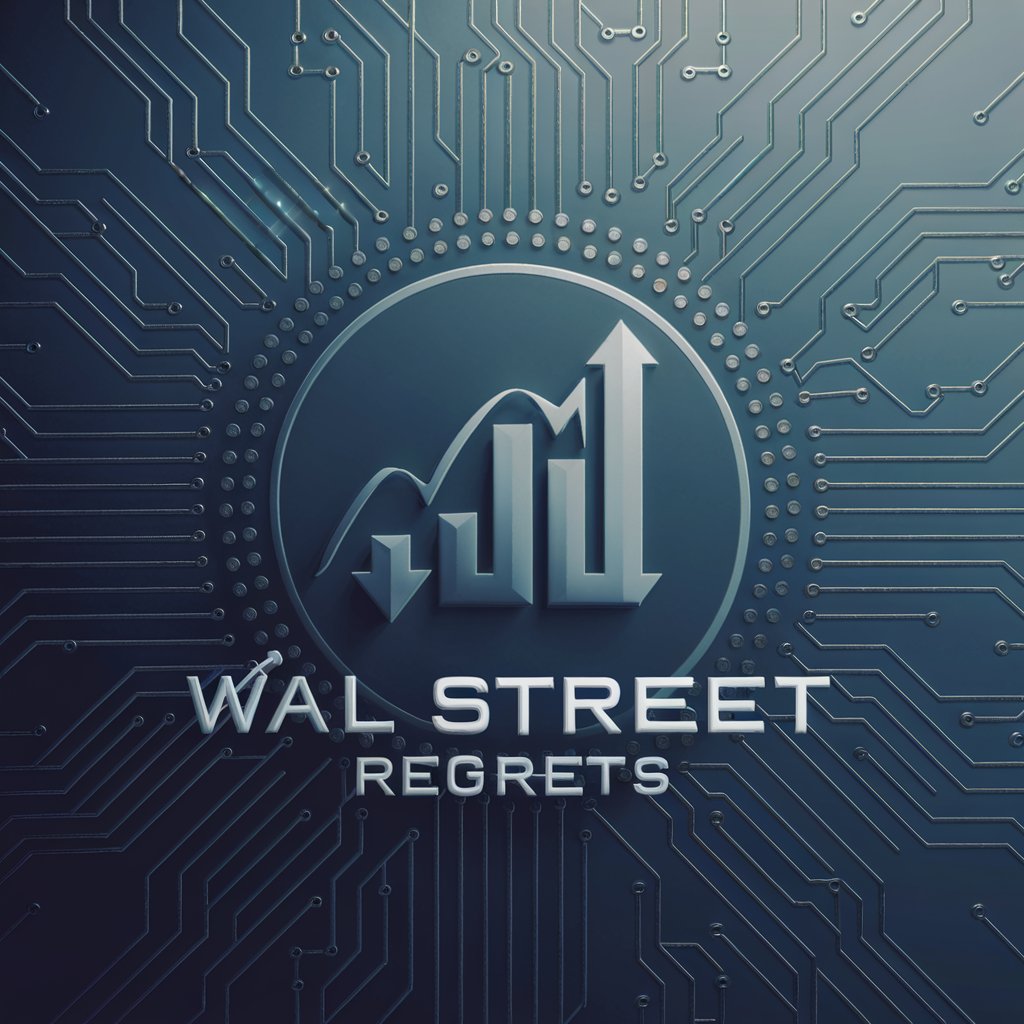
Regression Analysis in Biology Tutor
Unravel Biological Data with AI

Is it Sleep Regression?
AI-driven Sleep Pattern Insights
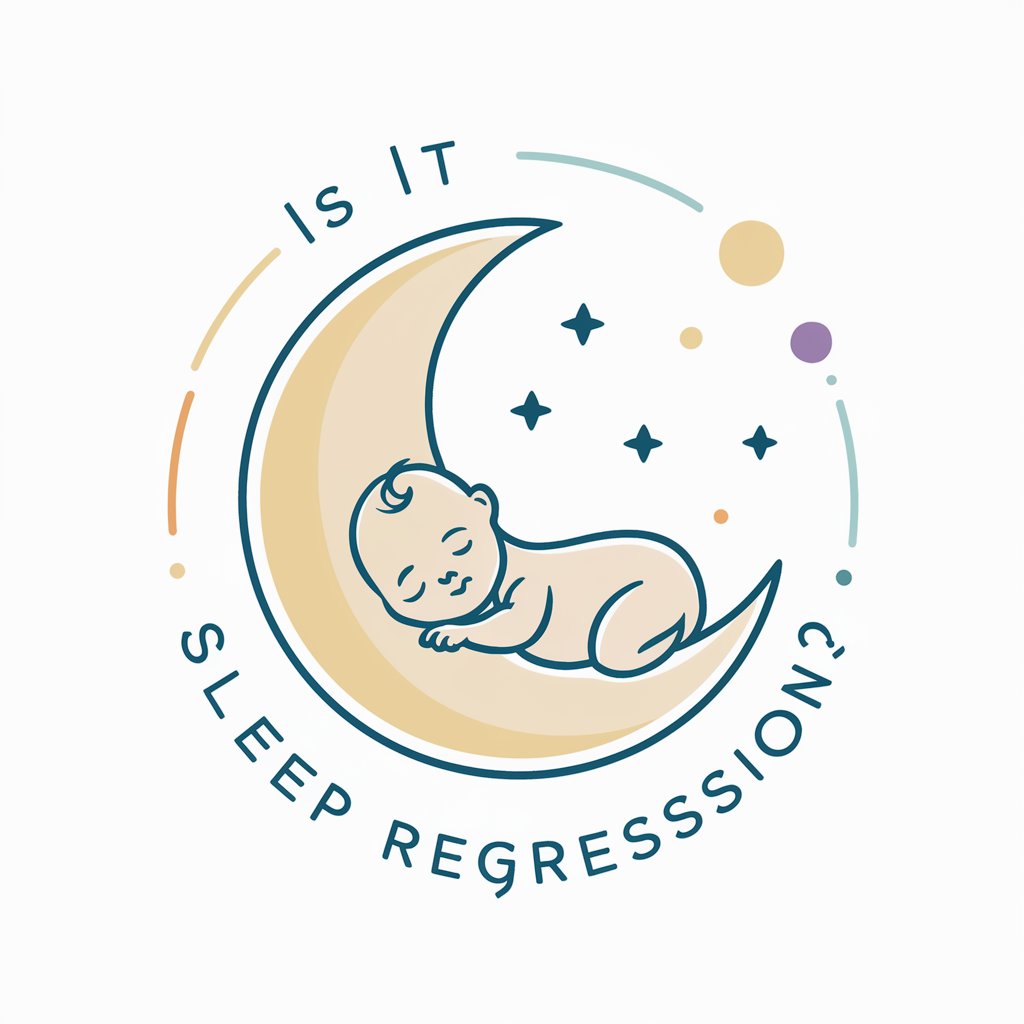
Life is too Short for Regrets
Empowering Personal Transformation with AI
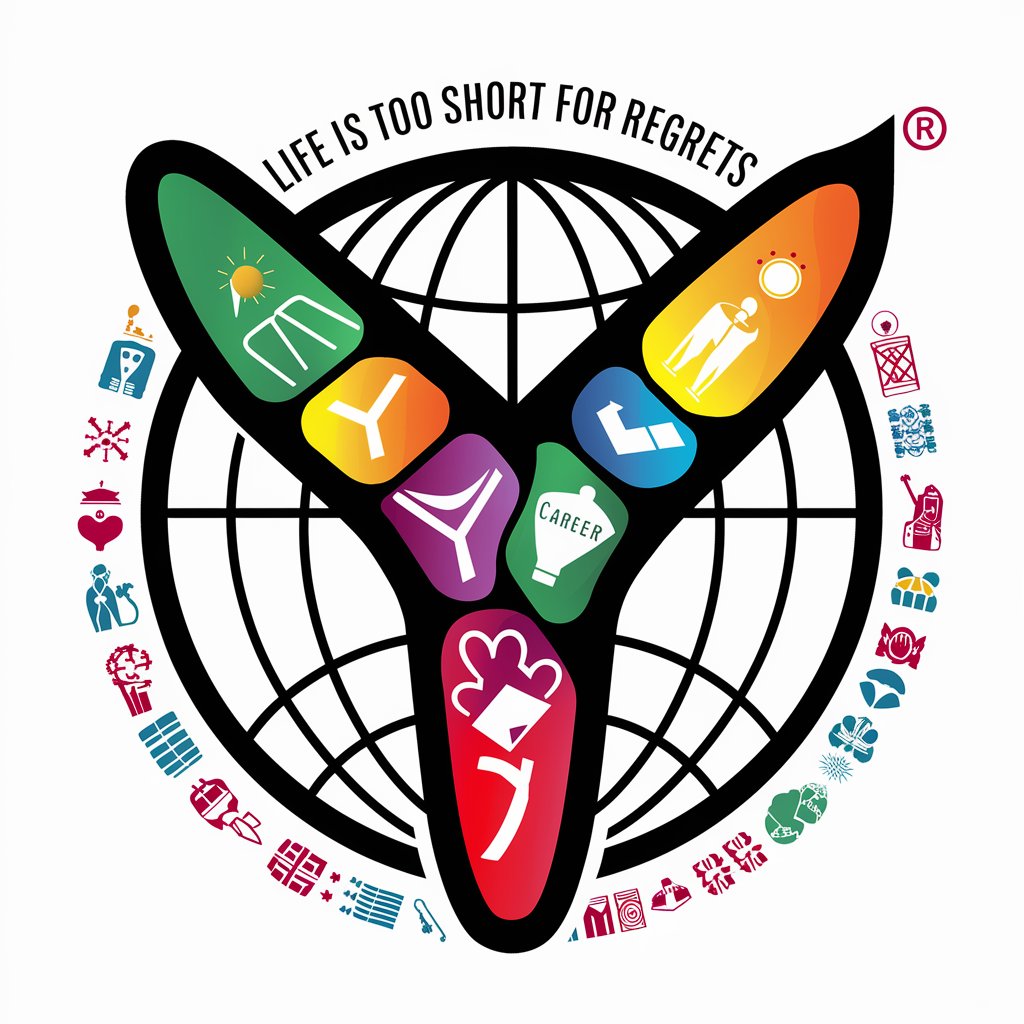
Ancient Aliens GPT
Exploring Ancient Mysteries with AI
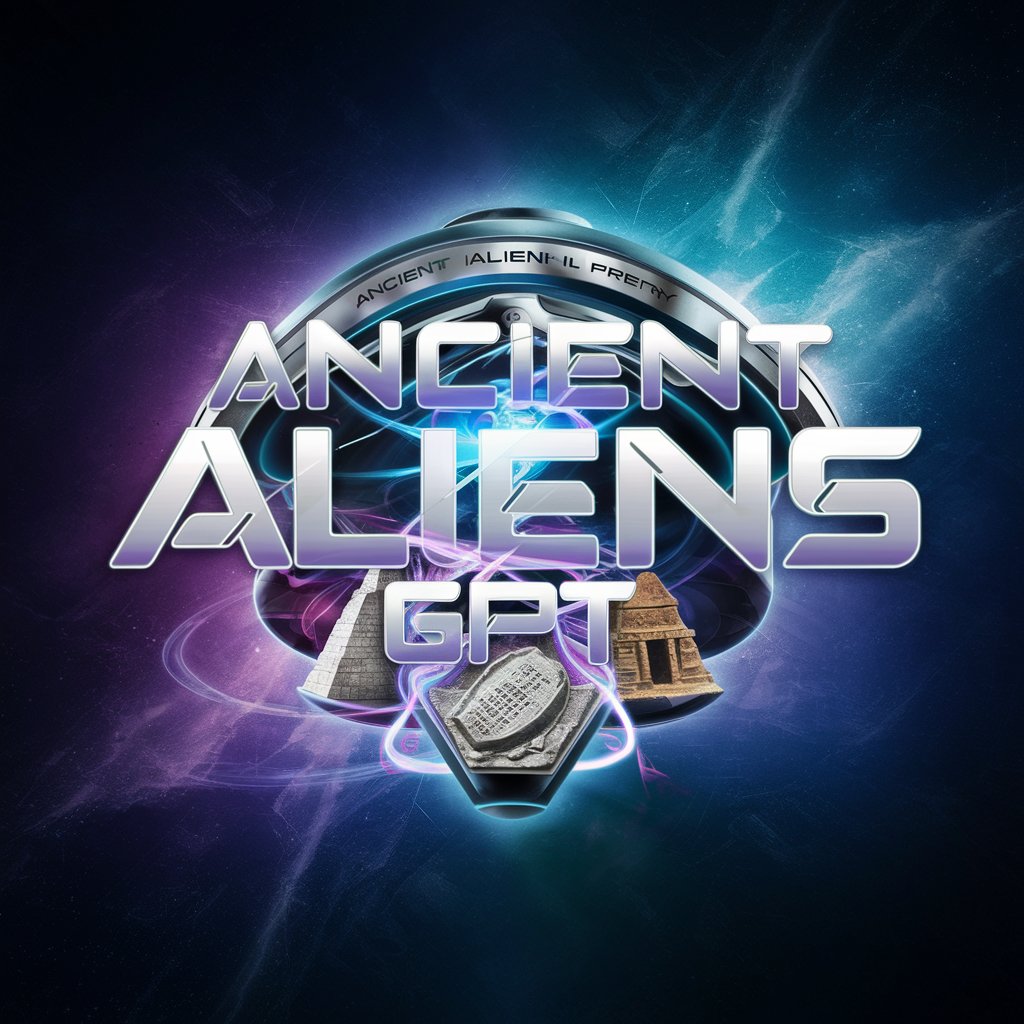
Social Media Influencer Battles Aliens
Resist, Record, and Rise Against Aliens
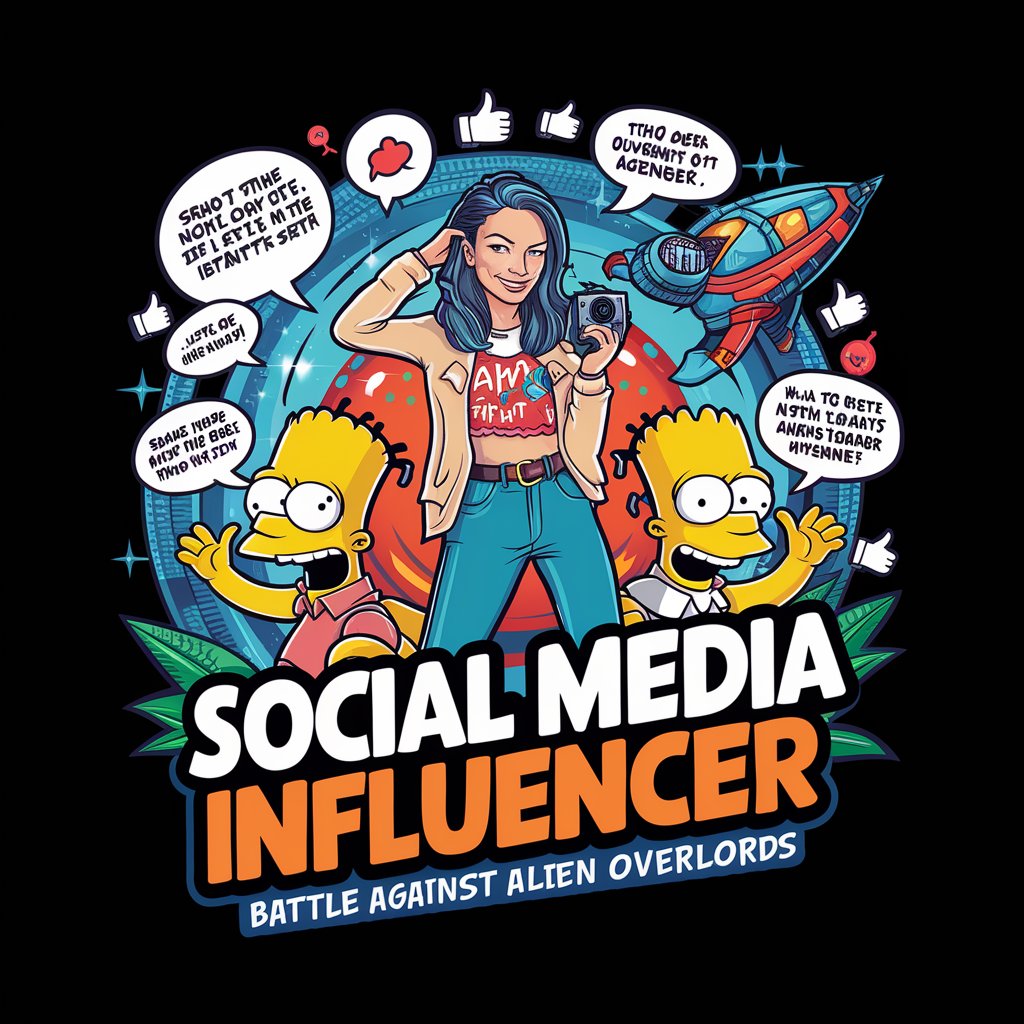
Aliens
Explore alien worlds with AI
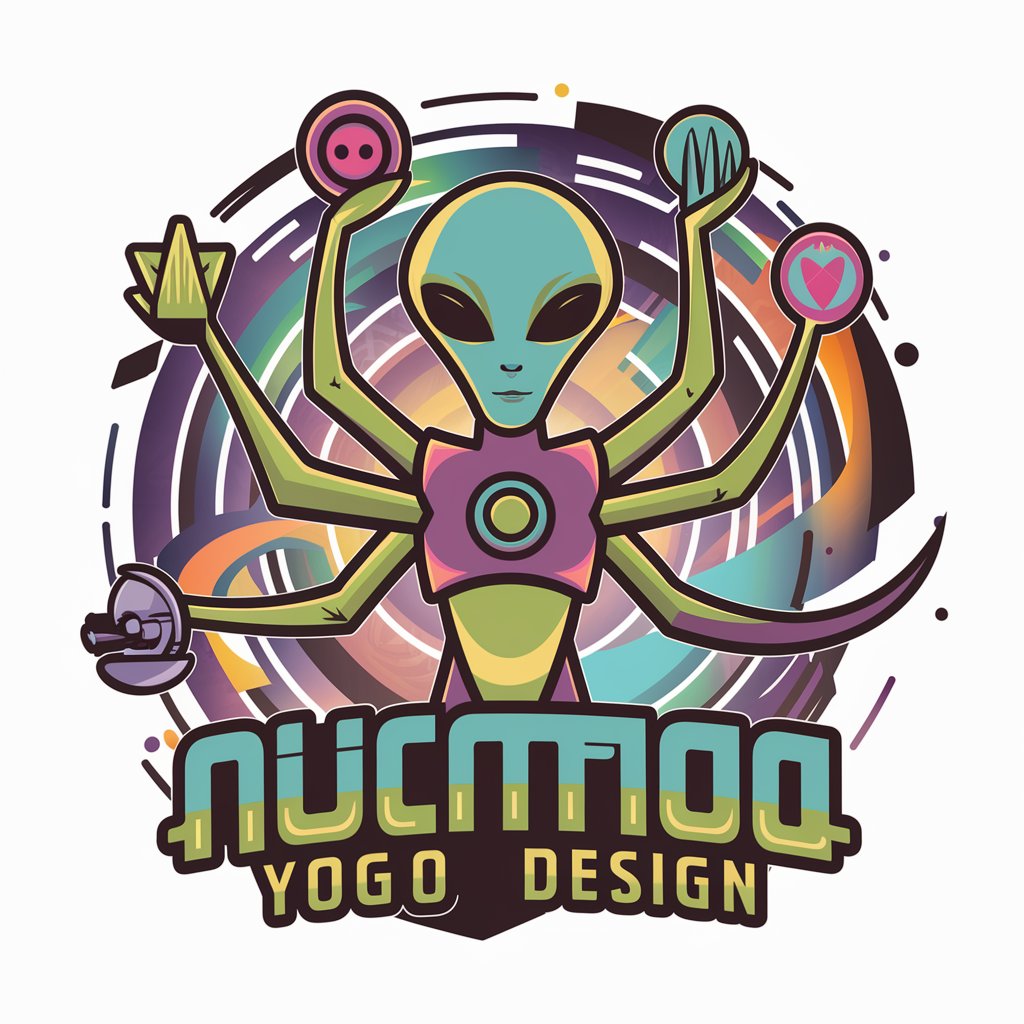
Divorce Discernment Guide
Empowering Marital Decisions with AI
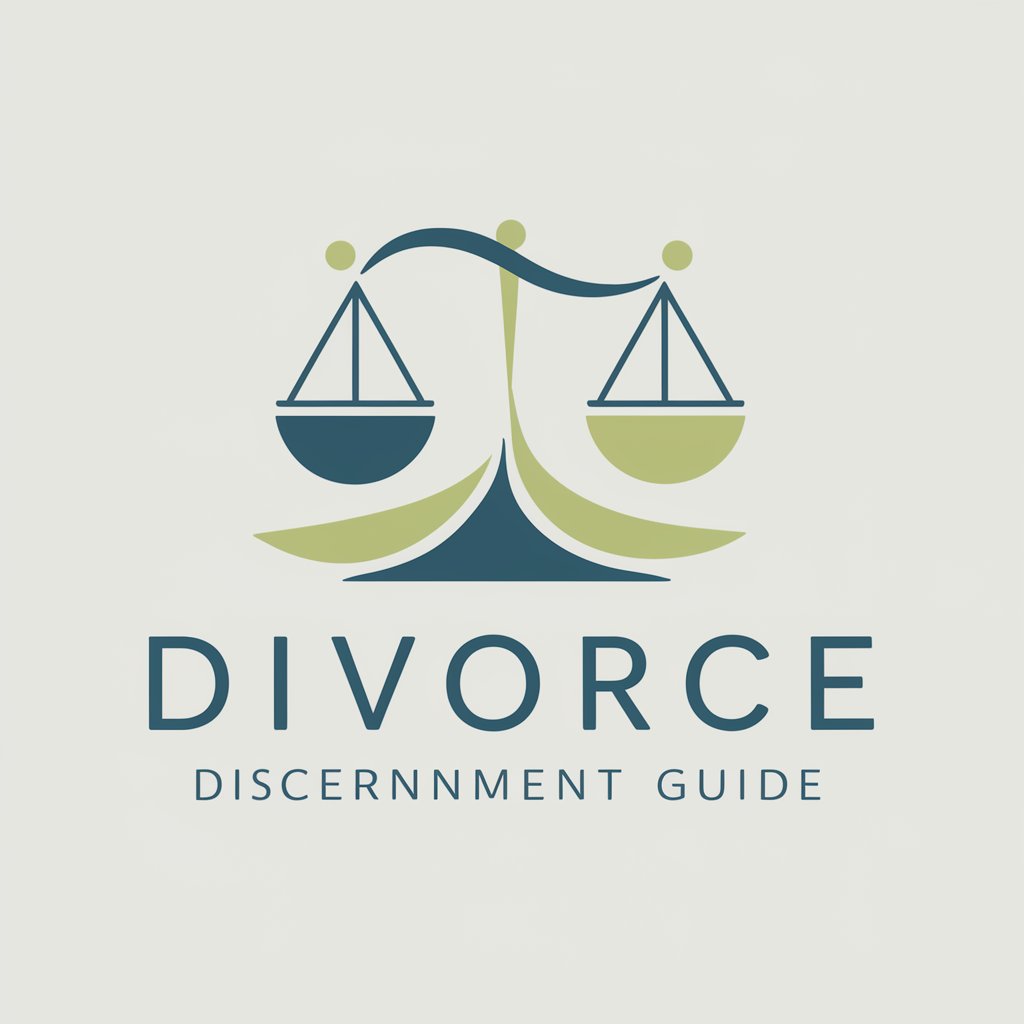
Pathfinder Guide
Navigating Success with AI
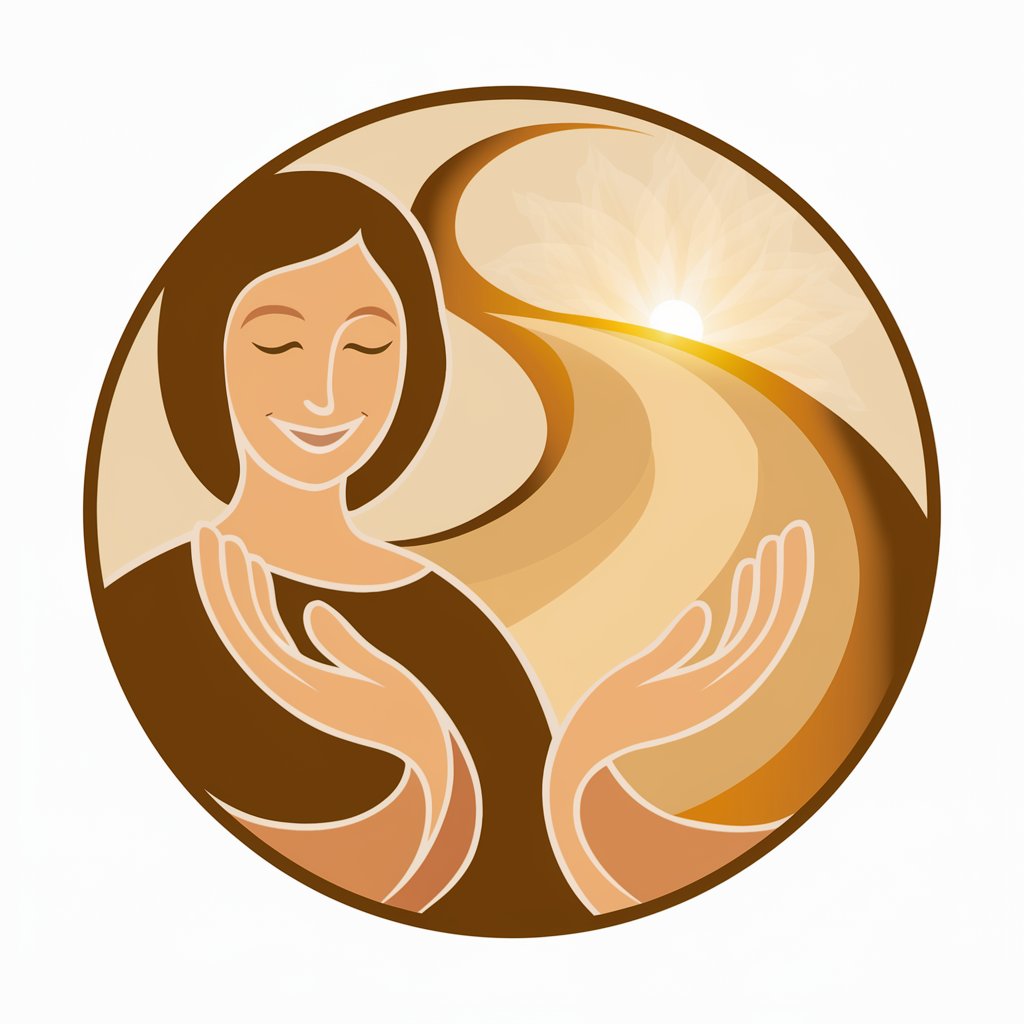
Public Administration
AI-Powered Public Administration Mastery
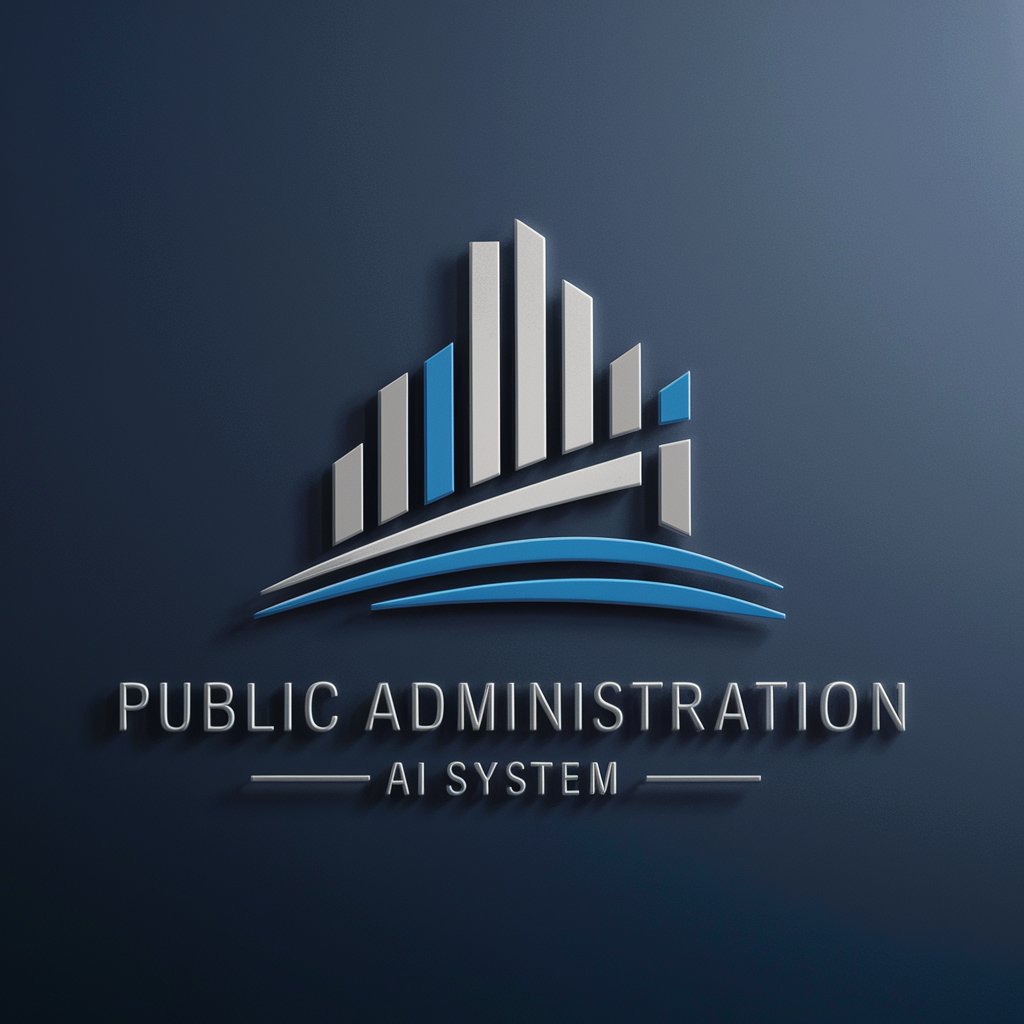
Regression Pro Q&A
What data formats does Regression Pro accept?
Regression Pro primarily works with structured data in CSV format. Ensure your data is clean and properly formatted before uploading.
Can Regression Pro handle large datasets?
Yes, Regression Pro is designed to process and analyze large datasets efficiently, thanks to optimized algorithms and scalable cloud infrastructure.
How does Regression Pro choose which regression model to use?
Regression Pro selects the model based on the target variable's nature. For continuous targets, it uses linear regression, and for binary targets, logistic regression.
What if I don't know which predictors to use?
Regression Pro can automatically suggest potential predictor variables based on your dataset's characteristics and statistical analysis.
Can I use Regression Pro for forecasting?
Yes, Regression Pro is suitable for forecasting scenarios as long as your dataset contains relevant historical data and time-based variables for prediction.