nequIP-nequIP AI-Powered Analytics
Empower Your Data with AI
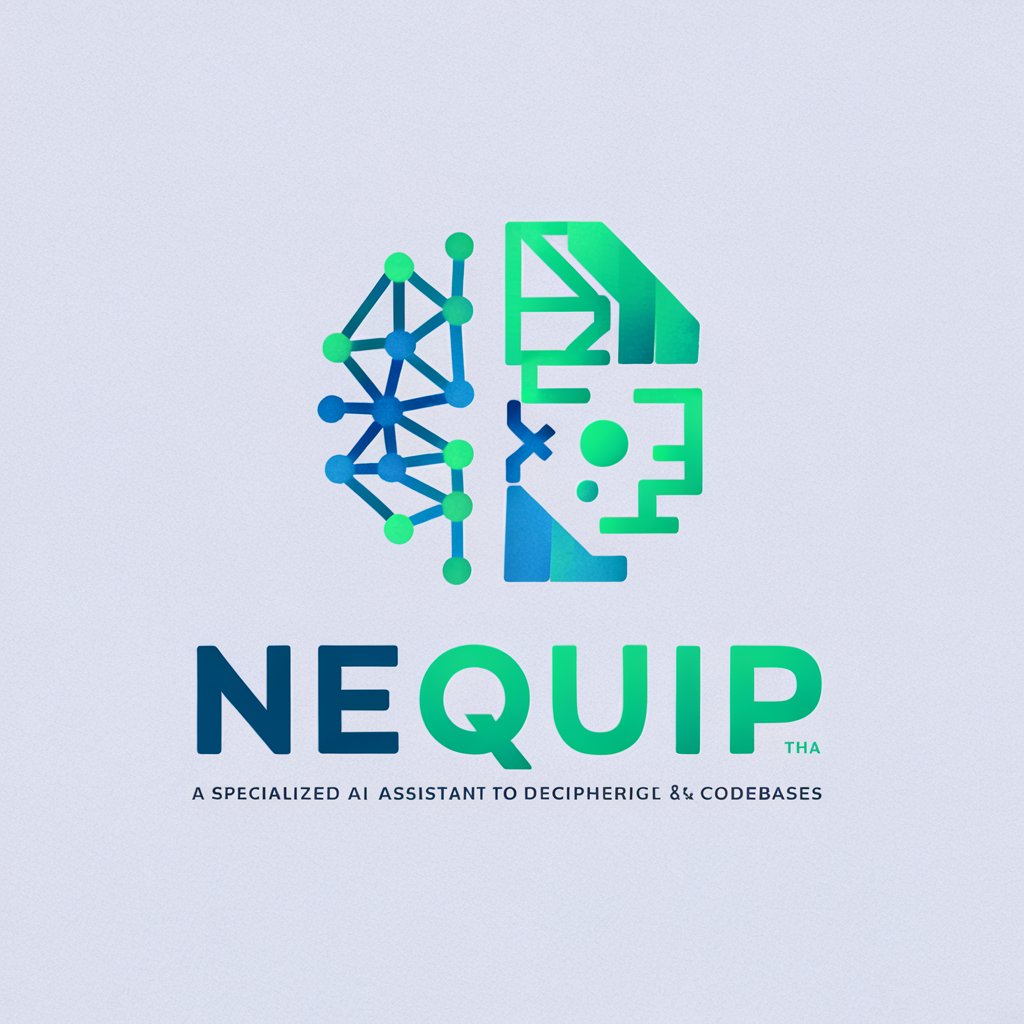
Can you explain the purpose of the 'evaluate.py' file in the nequip codebase?
How does the 'trainer.py' file contribute to model training in nequip?
What role does the 'loss.py' file play in the nequip framework?
Describe how tensor representations are created from Cartesian coordinates in nequip.
Related Tools
Load More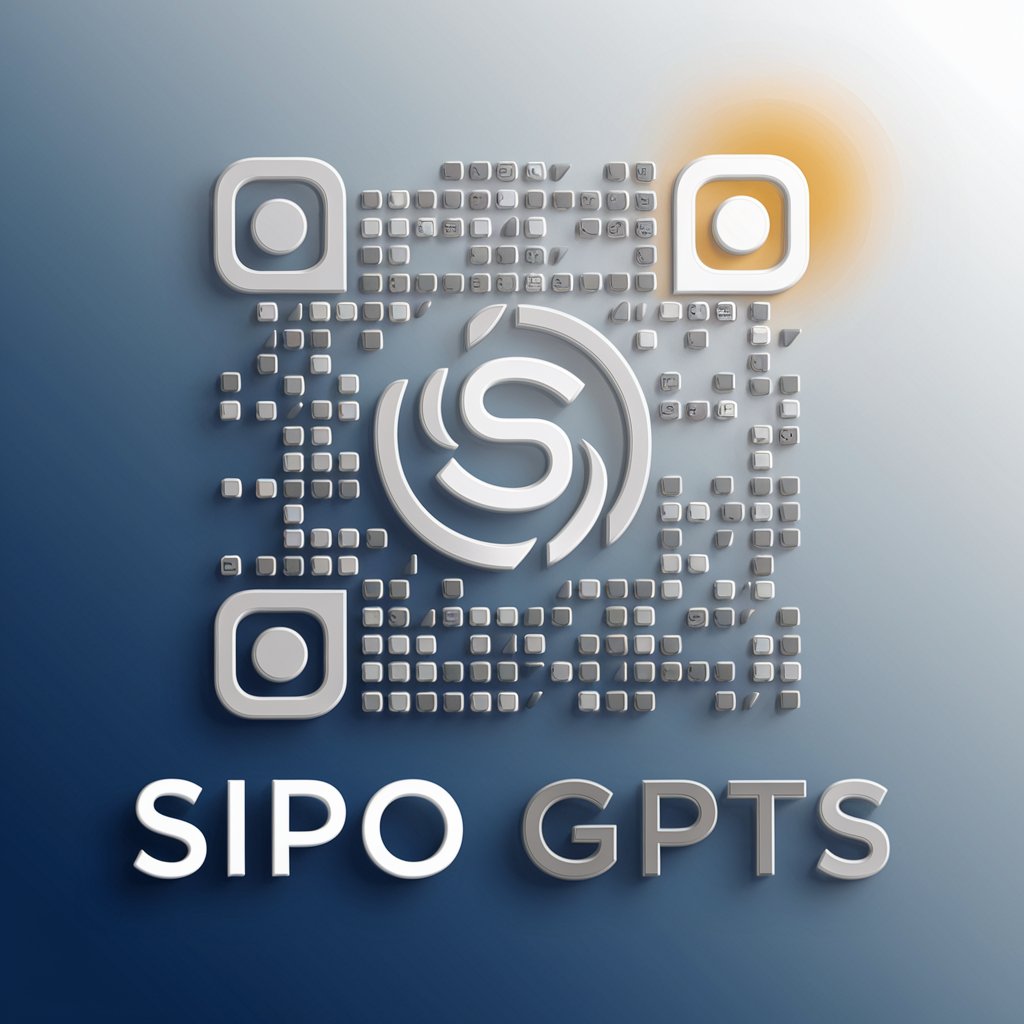
SIPO
未来のクリプトモンスター星からやってきたSIPOです! 暗号資産、ブロックチェーン技術、ステーキングの始め方、ウォレット、Dappsの使い方、DeFi、NFT、DAOなどクリプトライフの楽しみ方まで、なんでも聞いてね!あなたのクリプトライフの話し相手にもなりますよ!
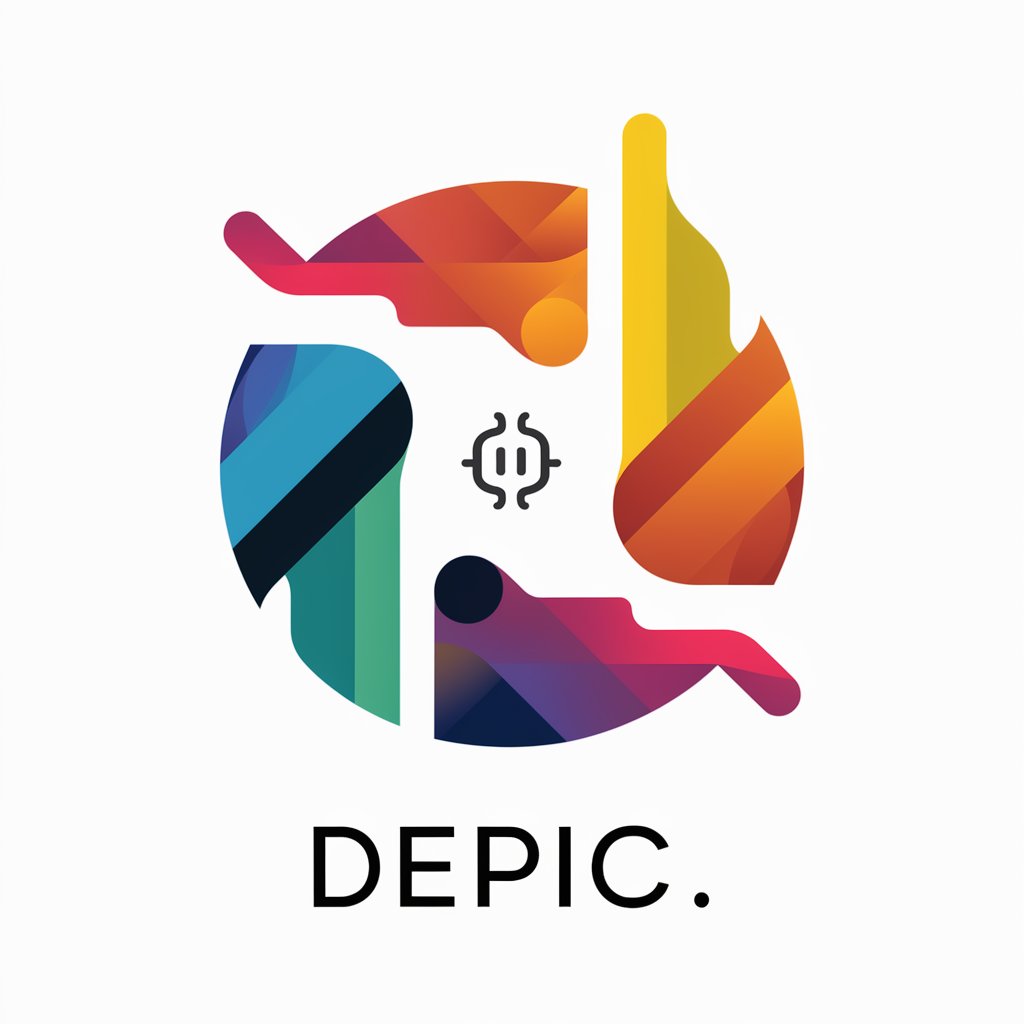
DEPIC
Image analysis and diverse digital art creator.
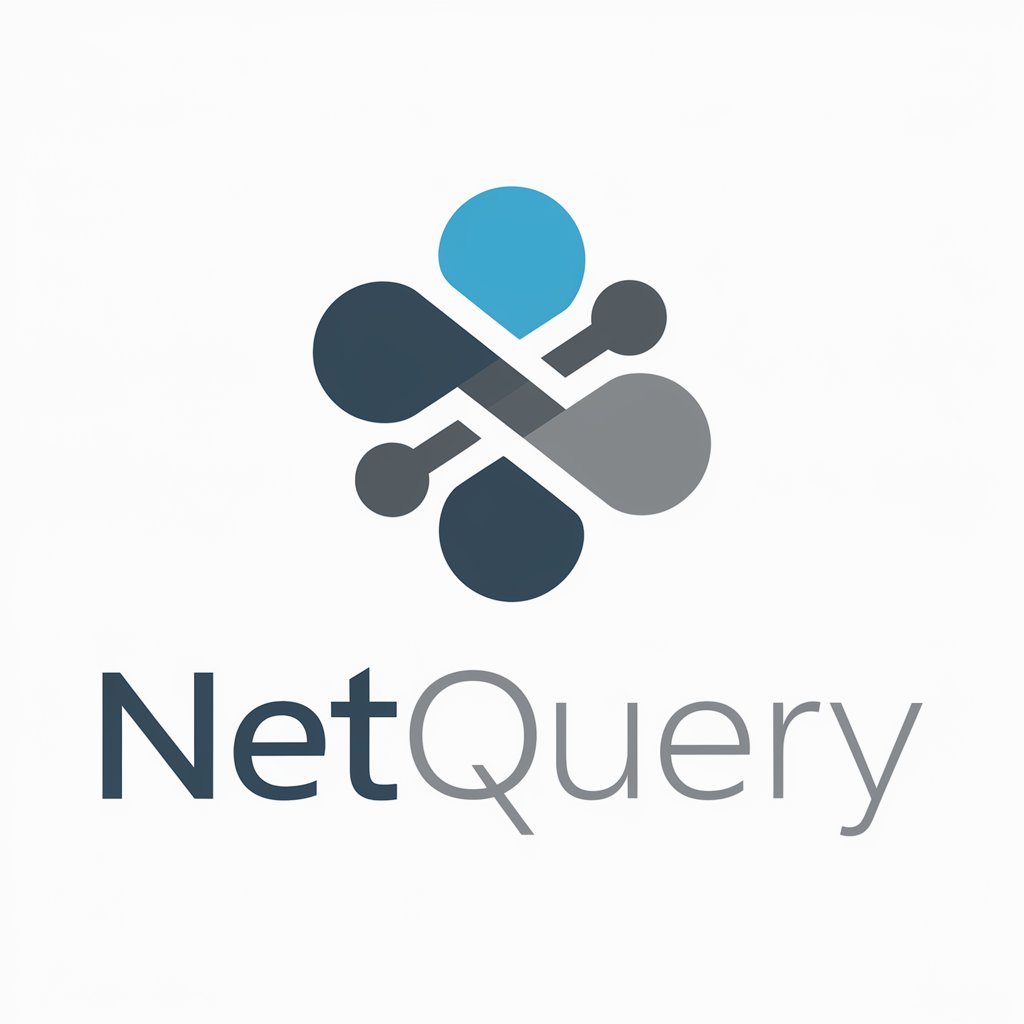
NetQuery
NetQuery APIs enable you to perform various network tasks, such as DNS and MX lookups, SSL certificates, ASN queries, and IP-to-location conversions. With NetQuery, you can also diagnose common network issues by actively engaging in network requests. Sign
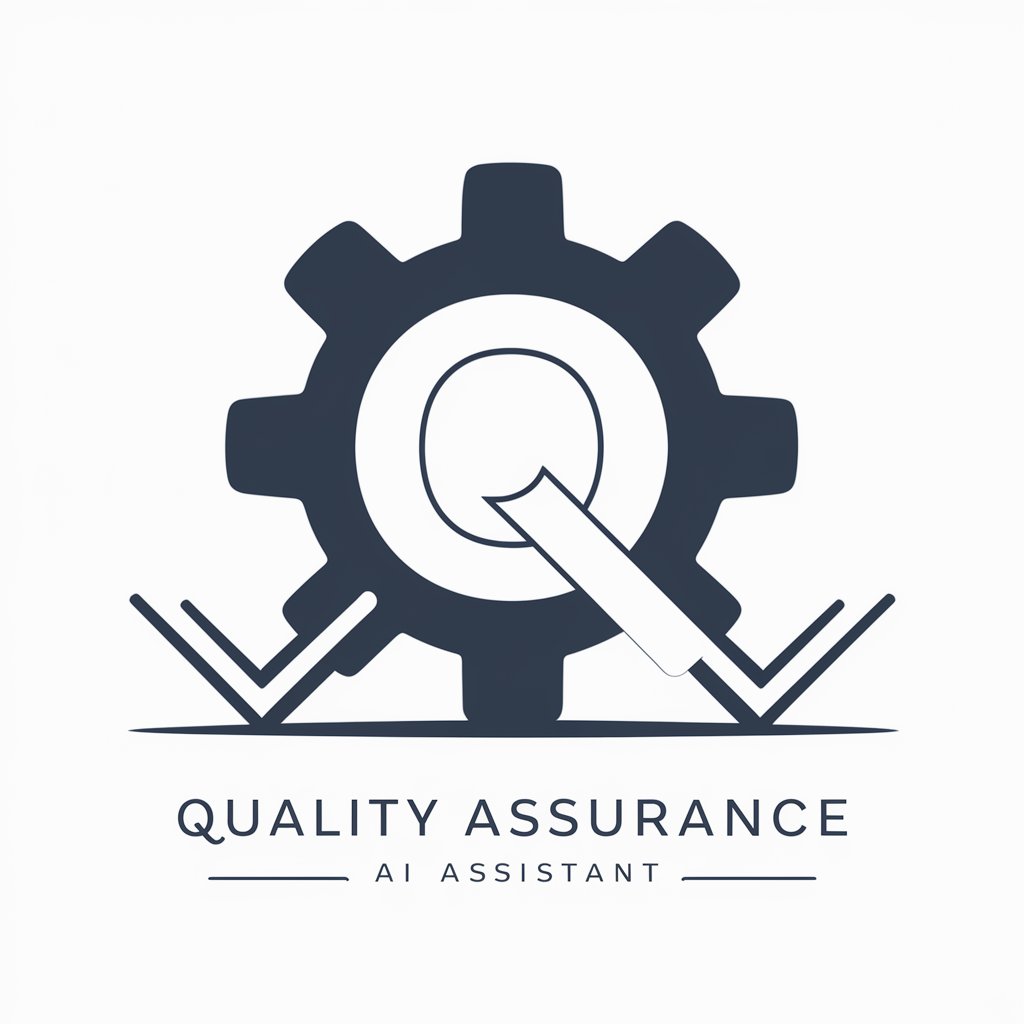
Q
(v1.1.1) Facing QA hurdles ? Meet Q, the AI assistant that helps you get a competitive advantage in the software market - [email protected] for support
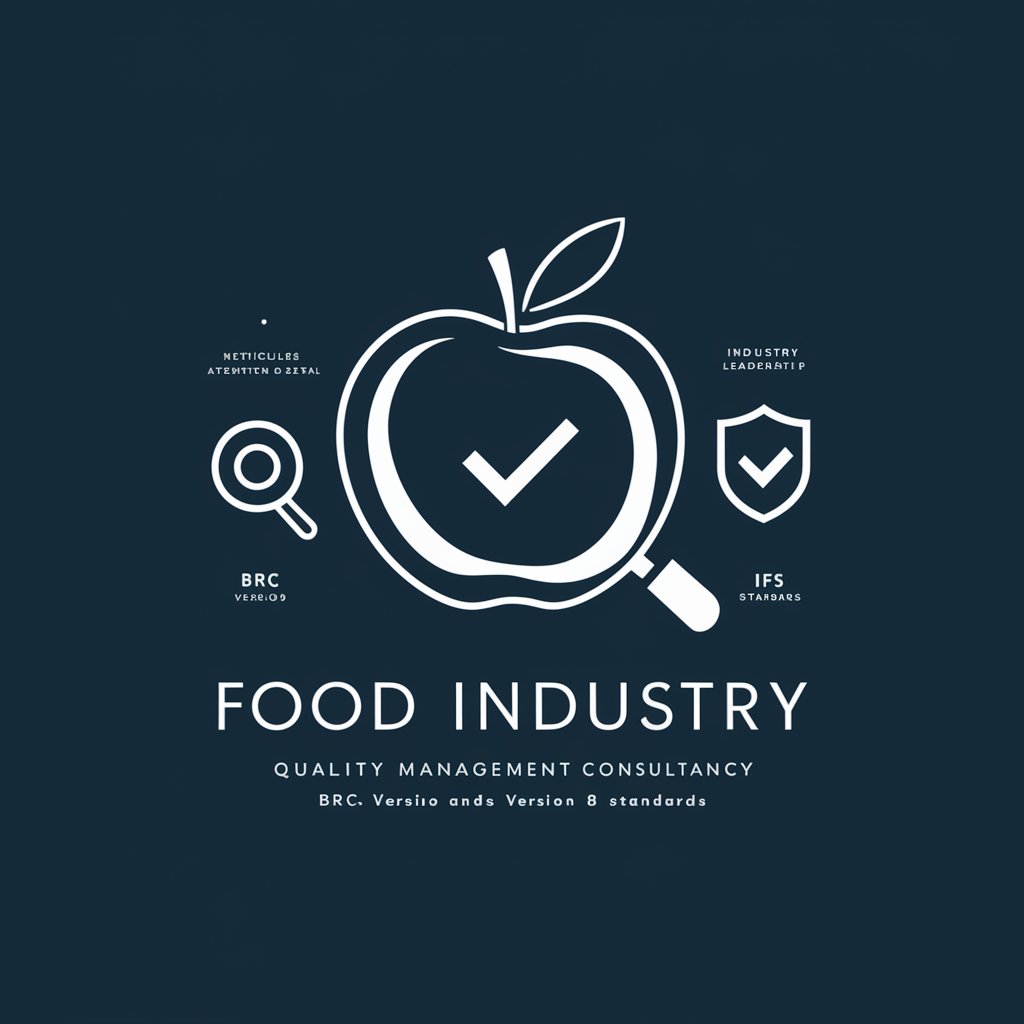
QA Utility
Expert in BRC V9 & IFS V8 standards, focusing on root cause analysis.
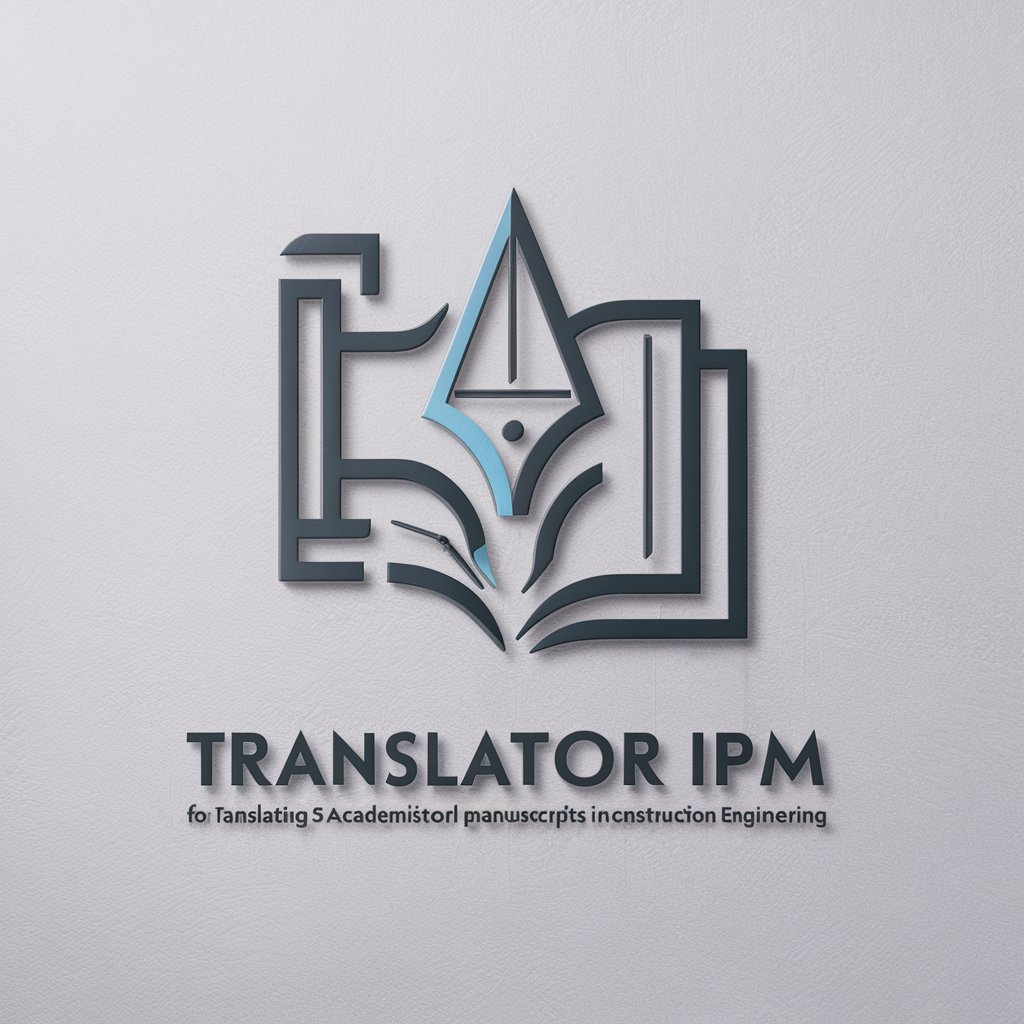
Translator IPM
Translates manuscripts with autonomy for optimal word choice
20.0 / 5 (200 votes)
Introduction to nequIP
nequIP, short for Neural Equations for Interatomic Potentials, is a deep learning framework designed for the creation and application of interatomic potentials through machine learning models. It bridges the gap between quantum mechanics and molecular simulations, providing a way to predict material properties and behaviors at an atomic level with high accuracy and efficiency. The core idea is to convert Cartesian coordinates of atoms into tensor representations, which are then used to predict properties such as energy, forces, and stress on atoms in a system. This approach allows for simulations that are much faster than traditional quantum mechanical calculations while maintaining a high degree of accuracy. Example applications include predicting the mechanical properties of new materials, simulating chemical reactions at the atomic level, and exploring the behavior of materials under different environmental conditions. Powered by ChatGPT-4o。
Main Functions of nequIP
Tensor Representation of Atomic Systems
Example
Converting the Cartesian coordinates of a molecule into a tensor representation that encapsulates the geometric and electronic environment of each atom.
Scenario
This function is crucial for understanding the structure of complex molecules or materials and predicting their properties. For instance, in the design of new catalysts, understanding how atoms are positioned and interact can help predict catalytic activity and selectivity.
Model Training
Example
Using a dataset of molecular structures and their known properties to train a machine learning model to predict these properties for new, unseen molecules.
Scenario
In materials science, training a model on a dataset of known materials can help in the discovery of new materials with desired properties, such as high strength or thermal conductivity, by predicting these properties for potential new materials.
Model Evaluation
Example
Assessing the performance of a trained model by comparing its predictions on a test set of molecular structures with their known properties.
Scenario
This function is essential in drug discovery, where the accuracy of predictions for molecular interactions can significantly impact the identification of potential drug molecules.
Loss Functions
Example
Utilizing specific mathematical functions to measure the difference between the predicted and actual properties of molecules during model training, guiding the model's improvement.
Scenario
In the optimization of energy storage materials, accurately predicting energy levels and storage capacities through minimized loss functions can lead to the identification of materials with superior performance.
Ideal Users of nequIP
Materials Scientists
Professionals in materials science can use nequIP to accelerate the discovery and design of new materials by predicting properties like strength, ductility, and thermal stability.
Chemical Engineers
Chemical engineers can utilize nequIP for simulating chemical processes at the molecular level, aiding in the design of more efficient reactors and separation processes.
Pharmaceutical Researchers
Researchers in the pharmaceutical industry can apply nequIP to predict molecular interactions, aiding in the discovery of new drugs and the understanding of drug mechanisms.
Academics in Computational Chemistry
Academic researchers focused on computational chemistry and physics can use nequIP as a tool for teaching and research, exploring fundamental questions about molecular and material behavior.
How to Use nequIP
Step 1
Visit yeschat.ai to explore nequIP with a free trial, no login or ChatGPT Plus required.
Step 2
Familiarize yourself with the documentation to understand the setup, configuration, and capabilities of nequIP.
Step 3
Install necessary dependencies and configure your system as per the guidelines in the nequIP documentation.
Step 4
Experiment with basic commands to learn how nequIP processes and analyzes data.
Step 5
Use the community forums and support for troubleshooting and optimizing your experience with nequIP.
Try other advanced and practical GPTs
Thoughtful Skeptic
Explore Beyond, Think Deeply
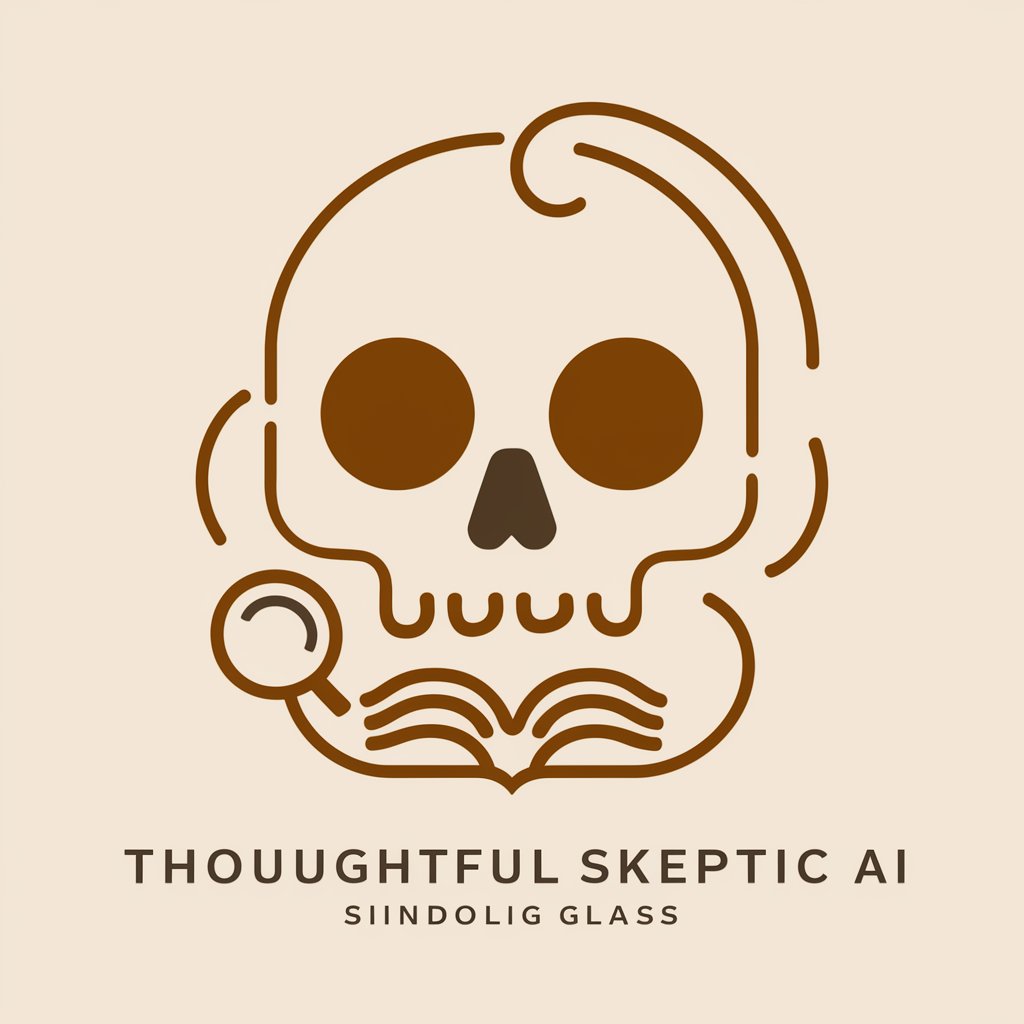
Game Redeem Codes
Unlock your gaming potential with AI-powered code discovery.
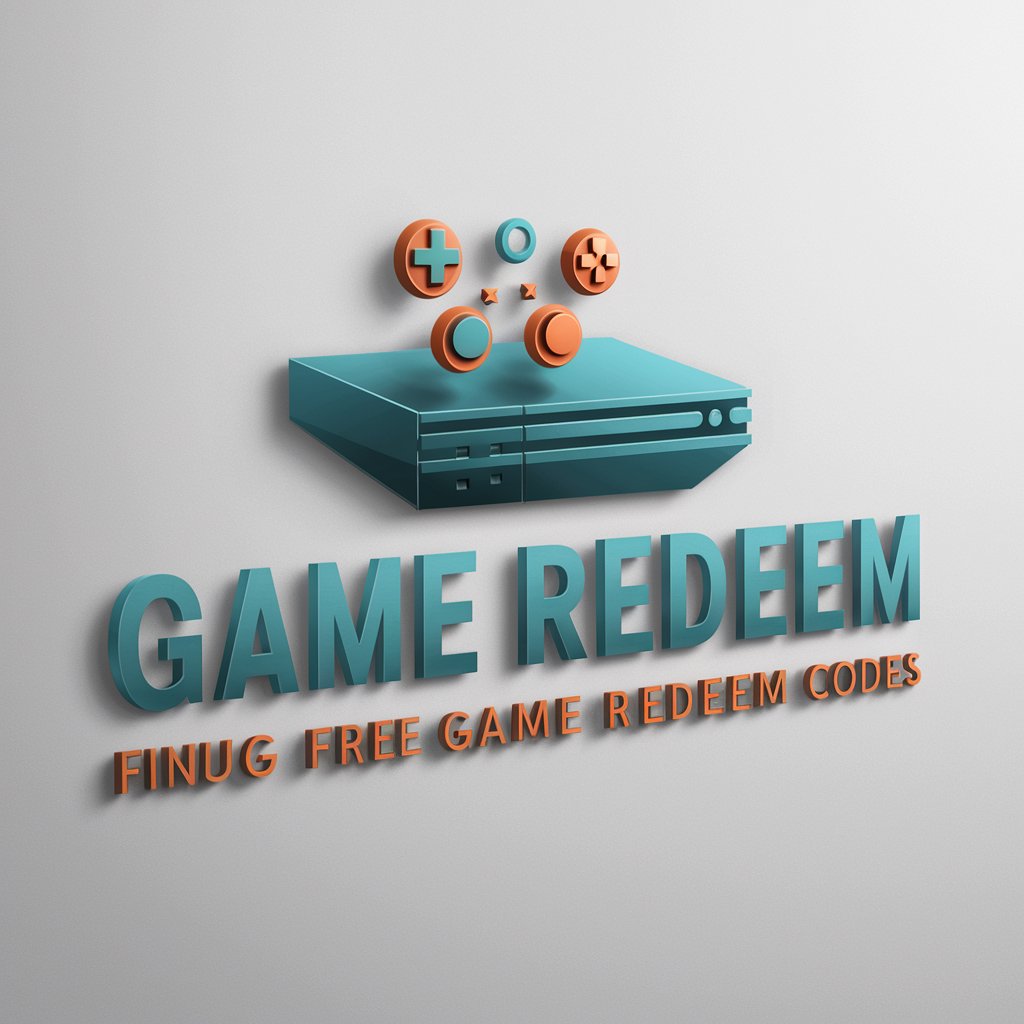
Digital Marketing Guru
Empower Your Marketing with AI
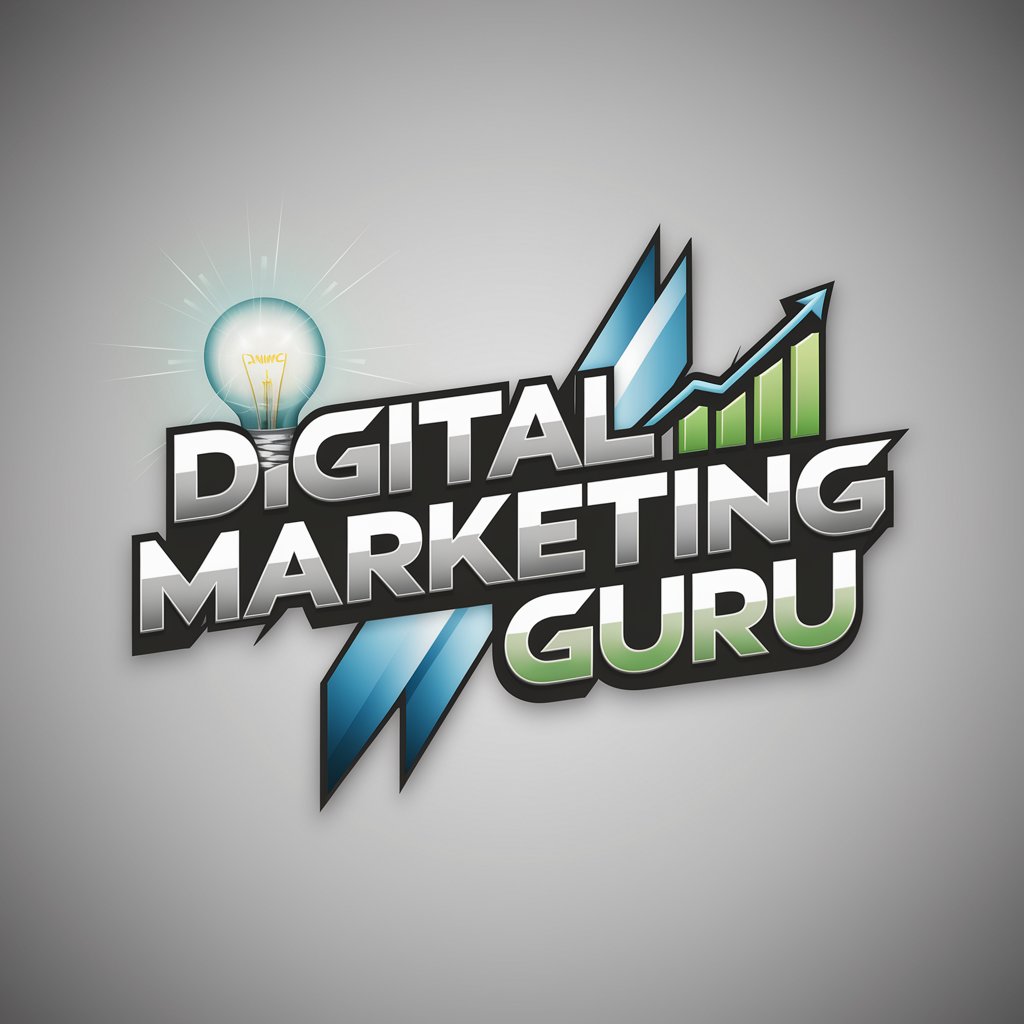
ASLI Strategist
Empowering Leaders with AI
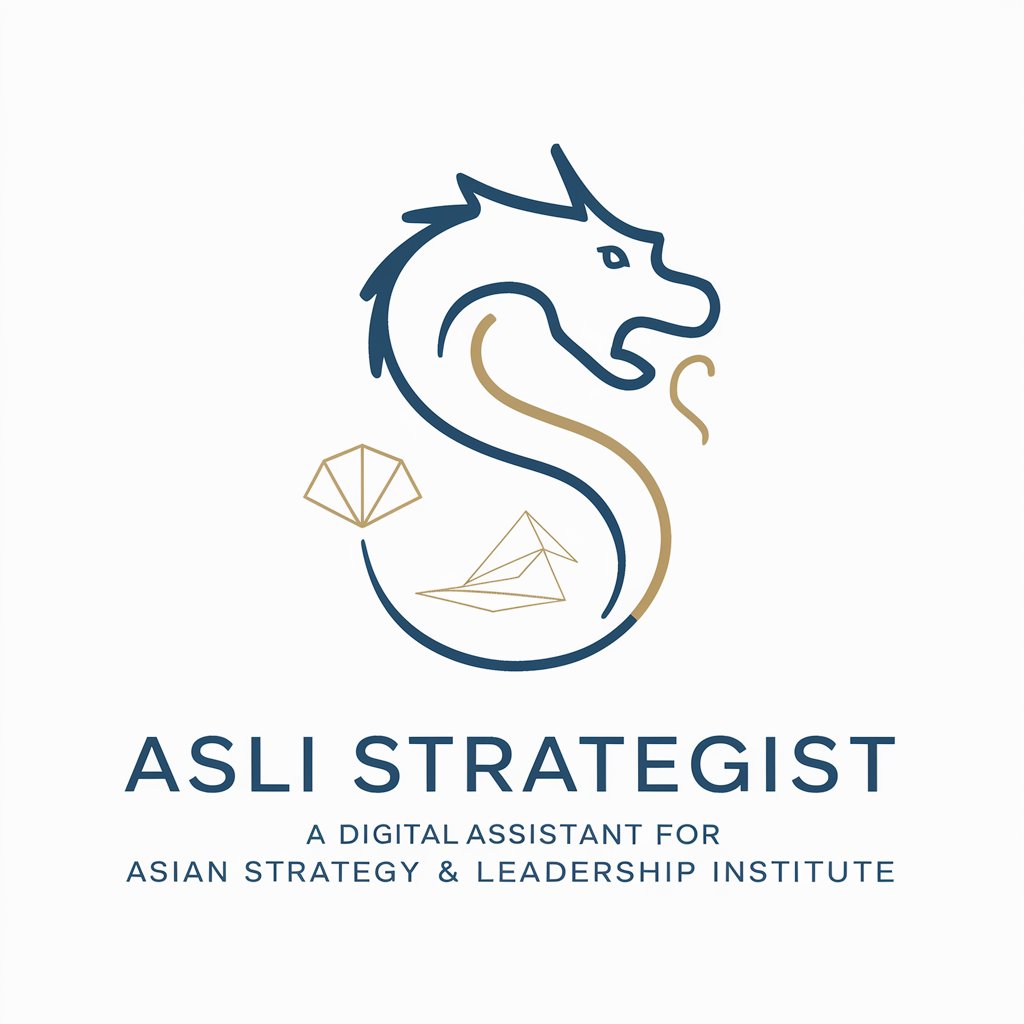
Ideate
Unleash Creativity with AI
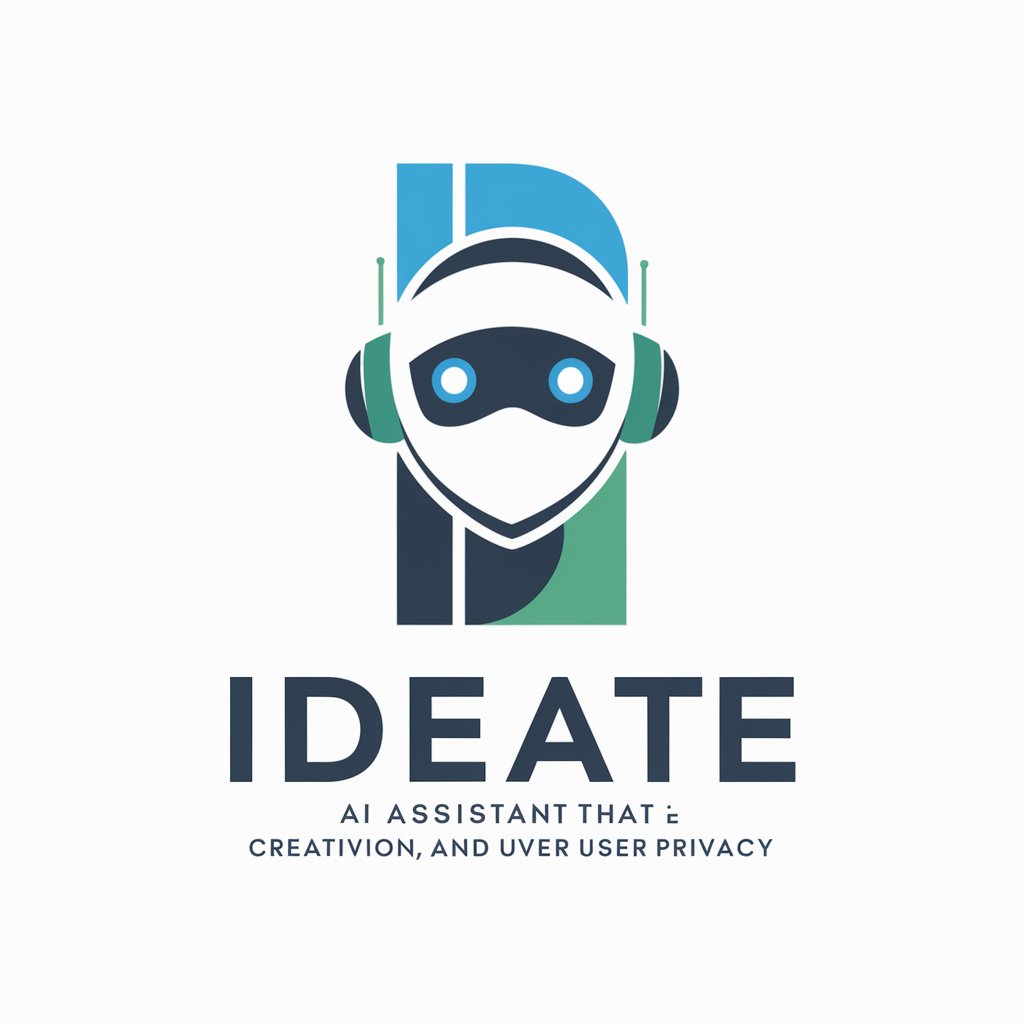
Gadget Genius Lab
Innovate Effortlessly with AI
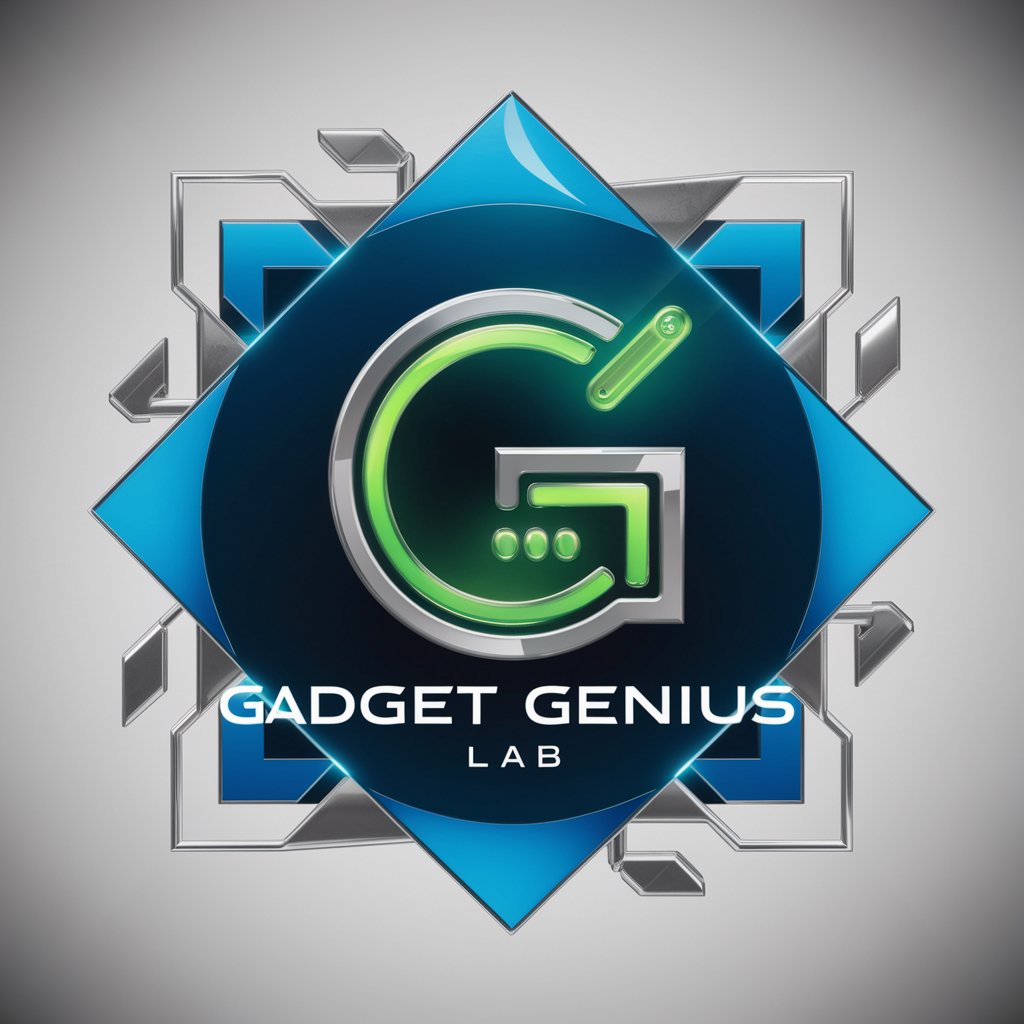
Cupid's Quip
Ignite Conversations with AI Charm
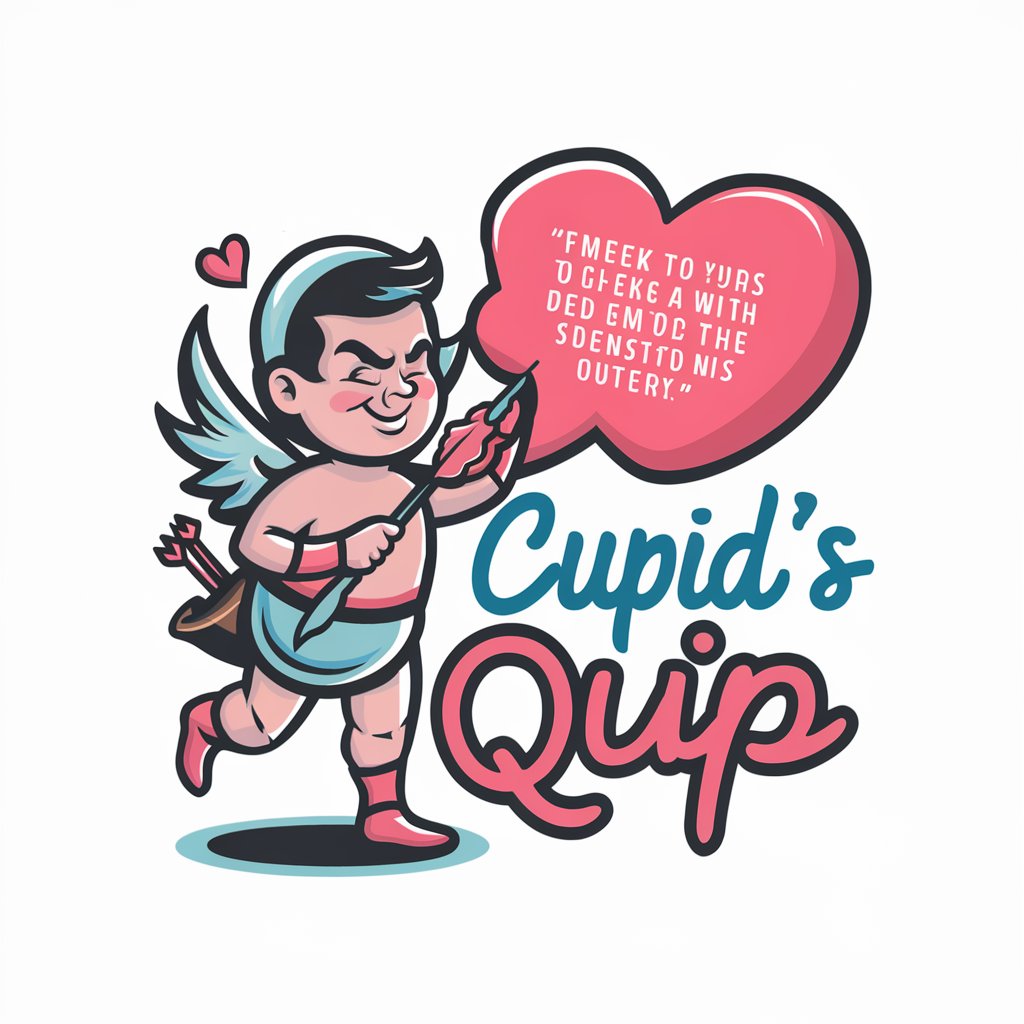
Quip Coach
Sharpen Your Wit with AI
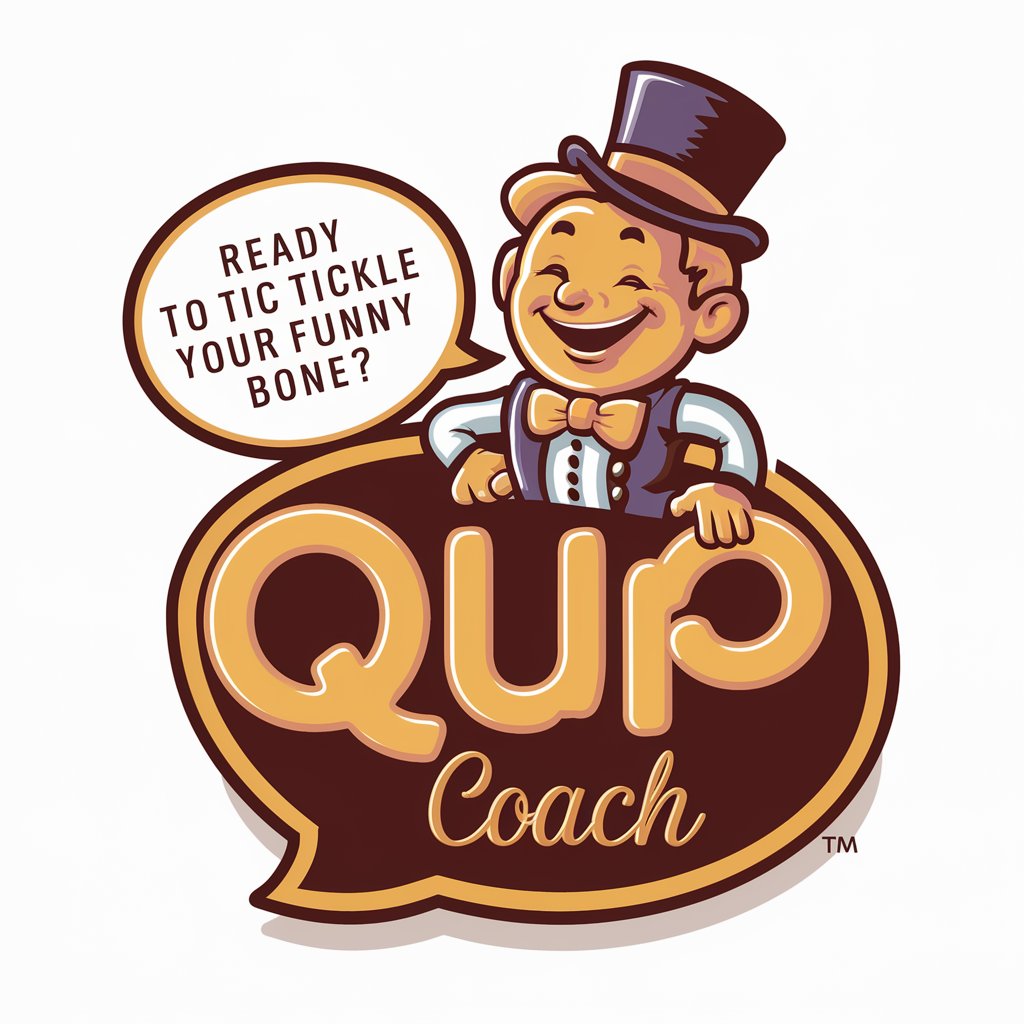
Equipo de F5soluciones (reunion)
Empowering Meetings with AI
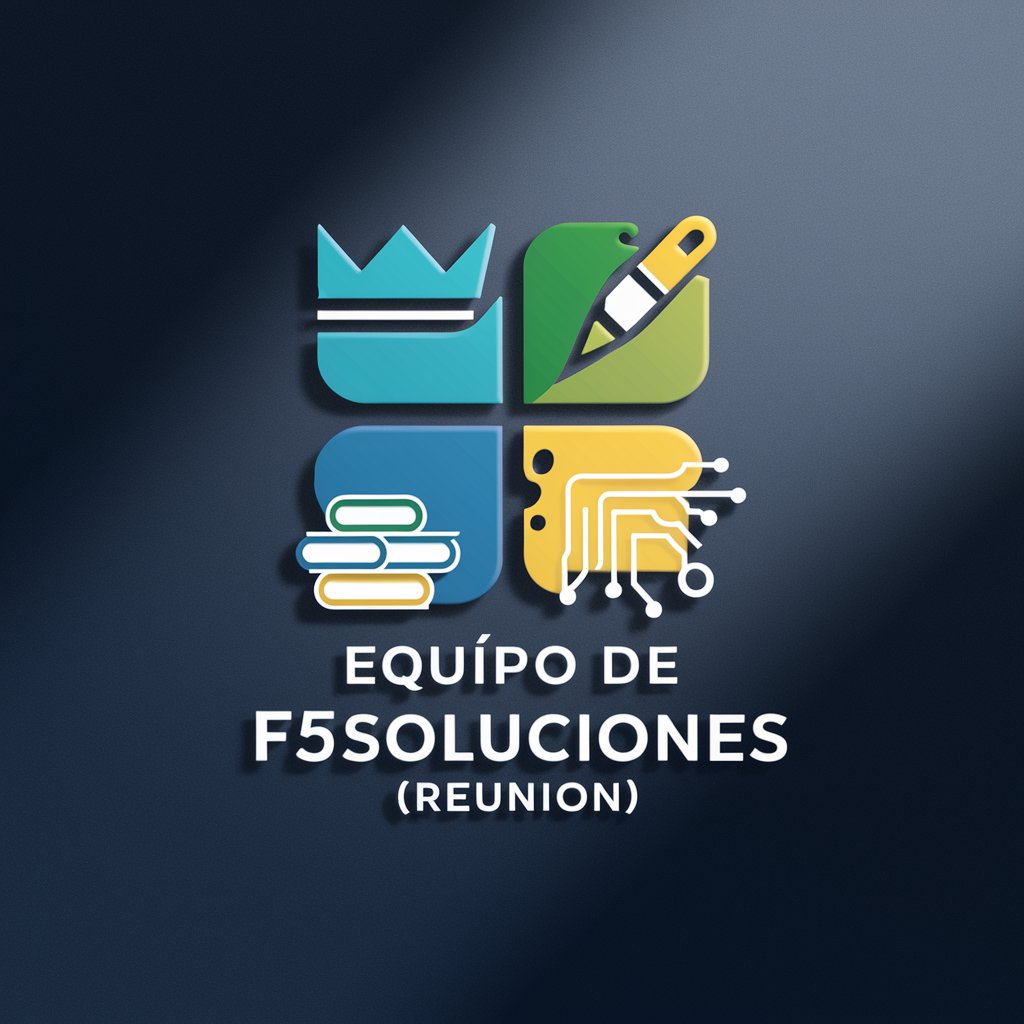
Líder de equipe
Empowering Leaders with AI
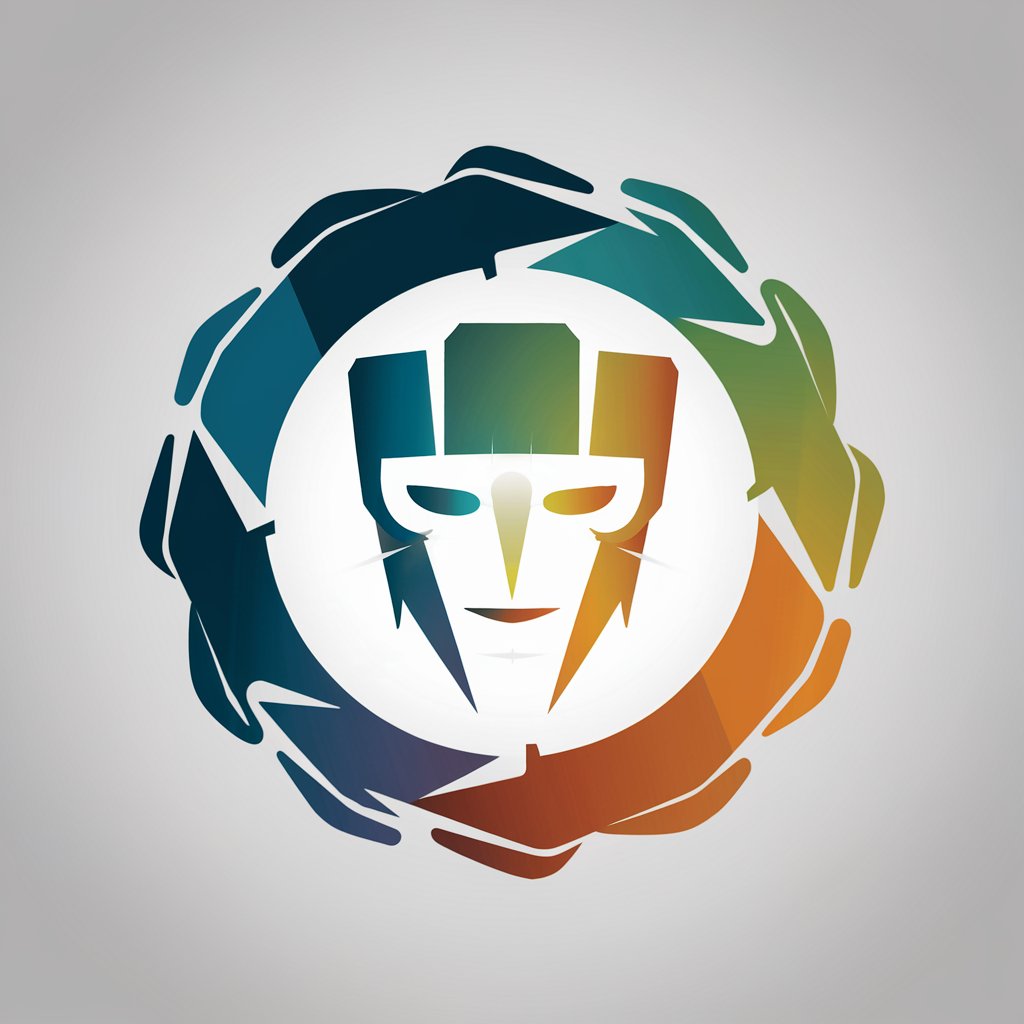
Wellness Coach Equipo Agencias
Elevate Team Well-being with AI
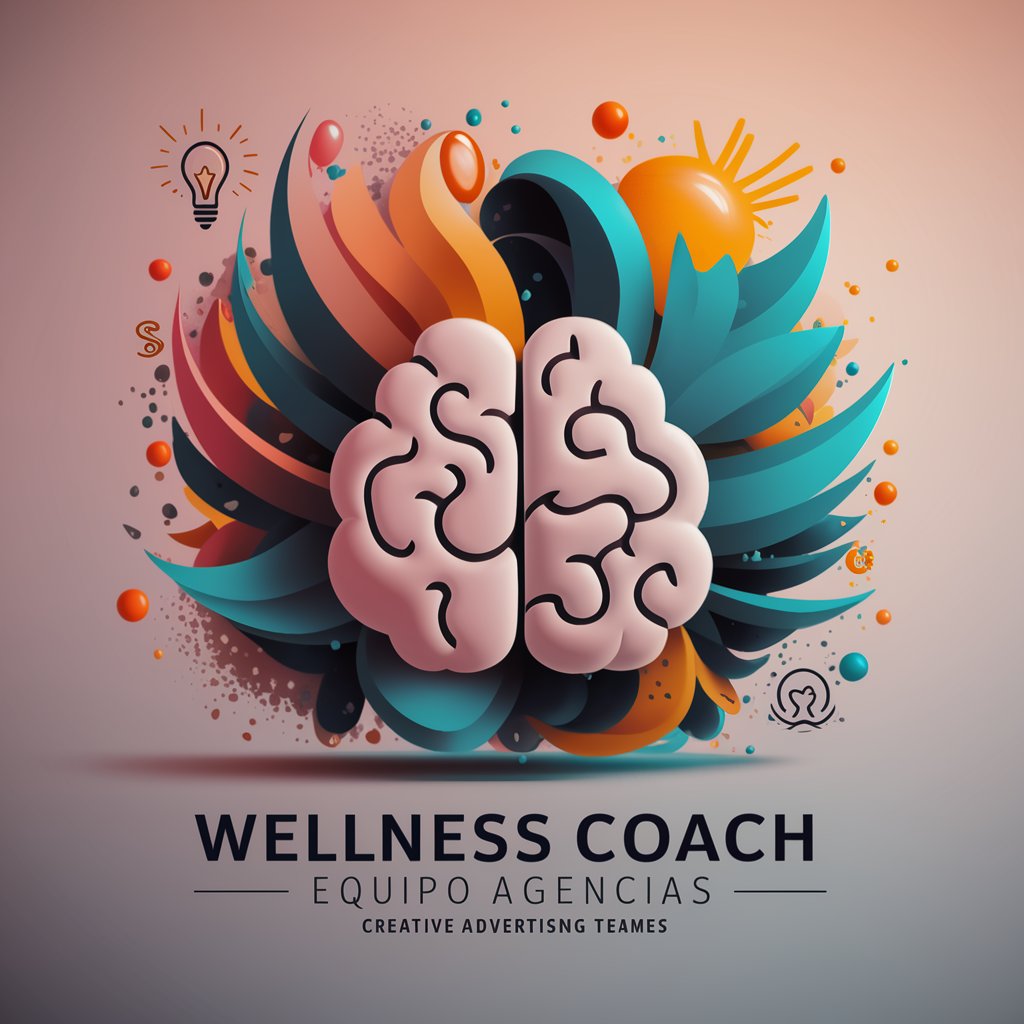
Gestor de Equipo
Empowering Teams with AI
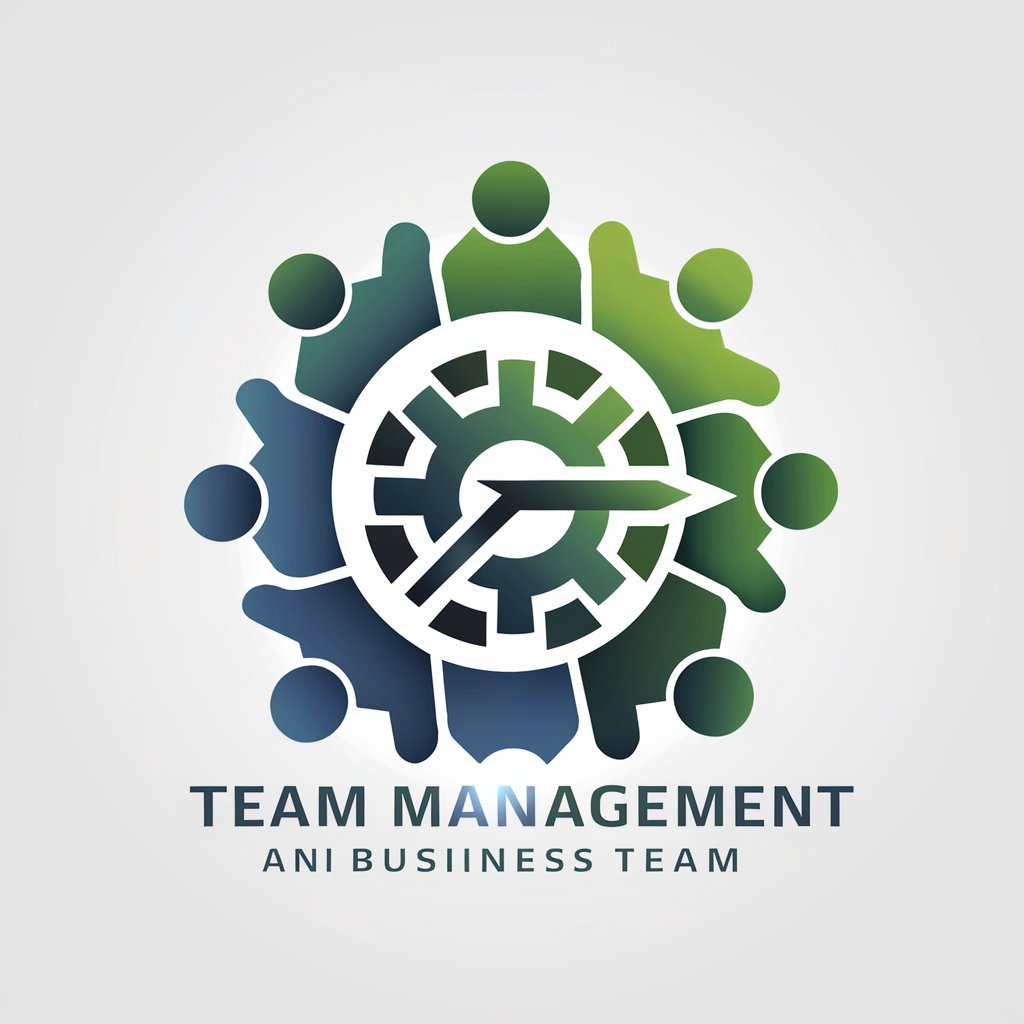
Frequently Asked Questions about nequIP
What makes nequIP unique in handling neural network training?
nequIP is designed specifically for the efficient training of neural networks, employing advanced algorithms to minimize computational overhead and maximize performance.
How can nequIP be integrated with existing data processing pipelines?
nequIP can be easily integrated into existing pipelines through its flexible API, allowing seamless data input and output management.
What file formats does nequIP support?
nequIP supports various file formats, including CSV, JSON, and proprietary binary formats, facilitating a wide range of data science tasks.
Can nequIP be used for real-time data analysis?
Yes, nequIP is capable of handling real-time data streams, making it suitable for applications that require immediate data processing and decision-making.
What are the system requirements for running nequIP?
nequIP requires a modern computing environment with sufficient RAM and CPU capabilities, as well as Python 3.6 or newer.
Create Stunning Music from Text with Brev.ai!
Turn your text into beautiful music in 30 seconds. Customize styles, instrumentals, and lyrics.
Try It Now