4 GPTs for Experiment Simulation Powered by AI for Free of 2025
AI GPTs for Experiment Simulation are advanced computational tools powered by Generative Pre-trained Transformers, designed to simulate and model experiments across various fields. These tools leverage the power of machine learning and natural language processing to predict outcomes, analyze data, and generate hypotheses, making them invaluable for research and development. Their relevance lies in their ability to offer precise, efficient, and cost-effective alternatives to physical experimentation, thereby accelerating the pace of innovation and discovery.
Top 4 GPTs for Experiment Simulation are: 🔬Subatomic Sleuth Explorer👨🔬,Lab Genius,🦠 MicrobeMapper: Culture Sleuth 🔬,实验模拟器
🔬Subatomic Sleuth Explorer👨🔬
Unlocking the mysteries of the quantum world.
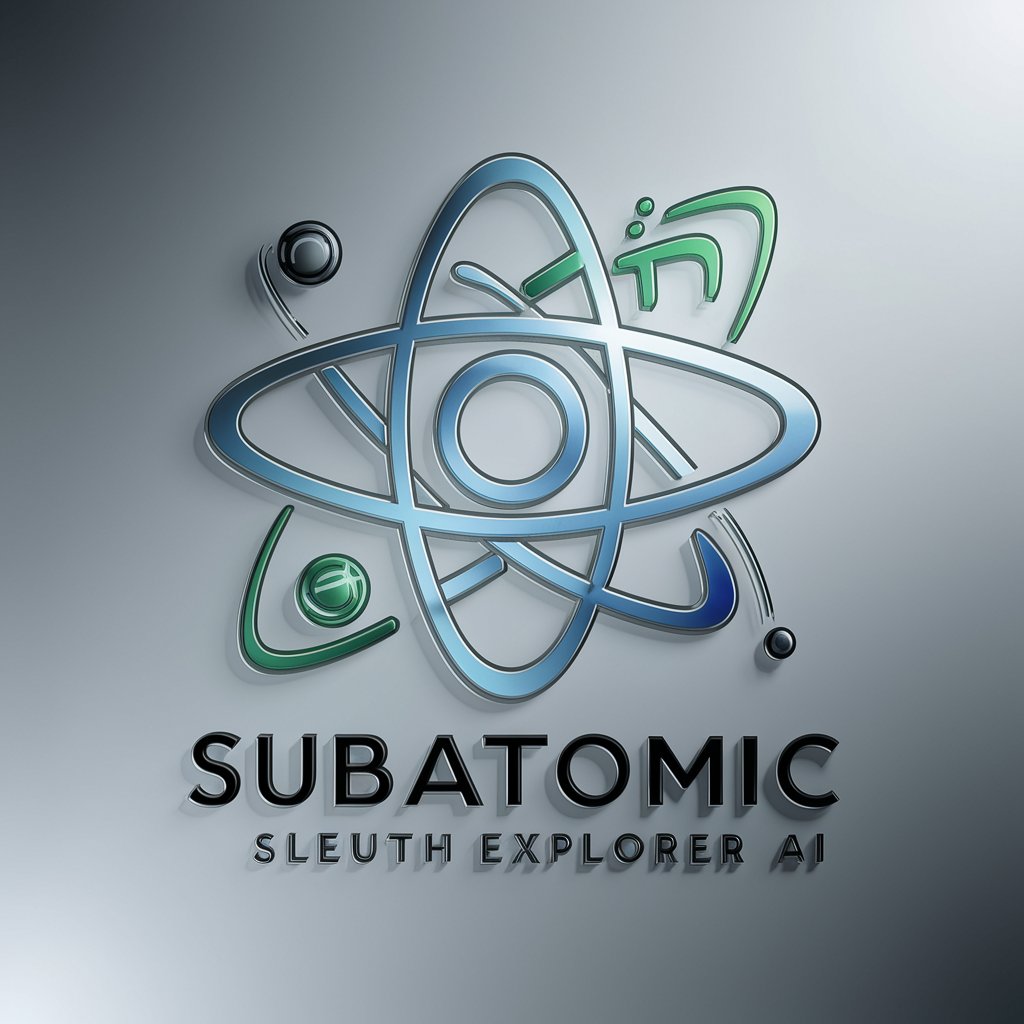
Lab Genius
Empowering research with AI-driven simulations.
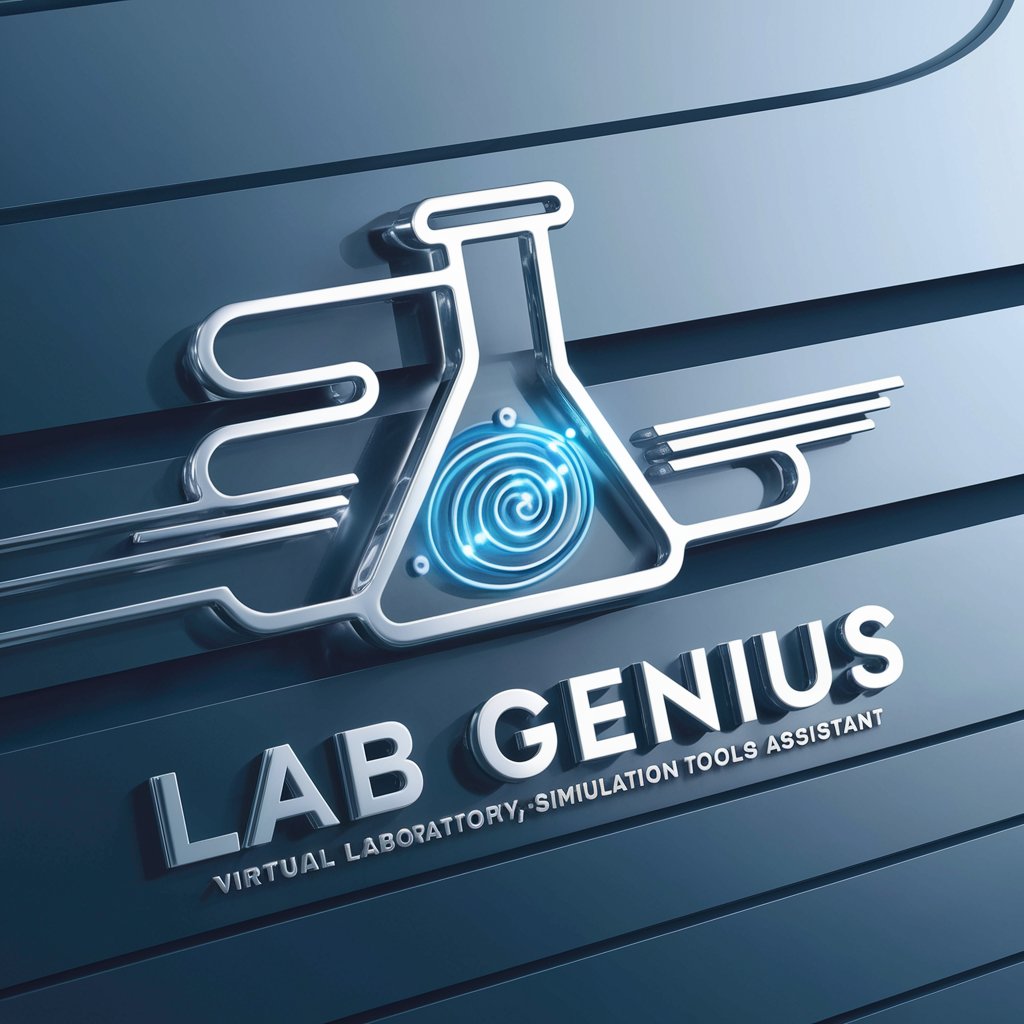
🦠 MicrobeMapper: Culture Sleuth 🔬
Decipher microbes with AI precision.

实验模拟器
Empowering experimentation with AI-driven simulations
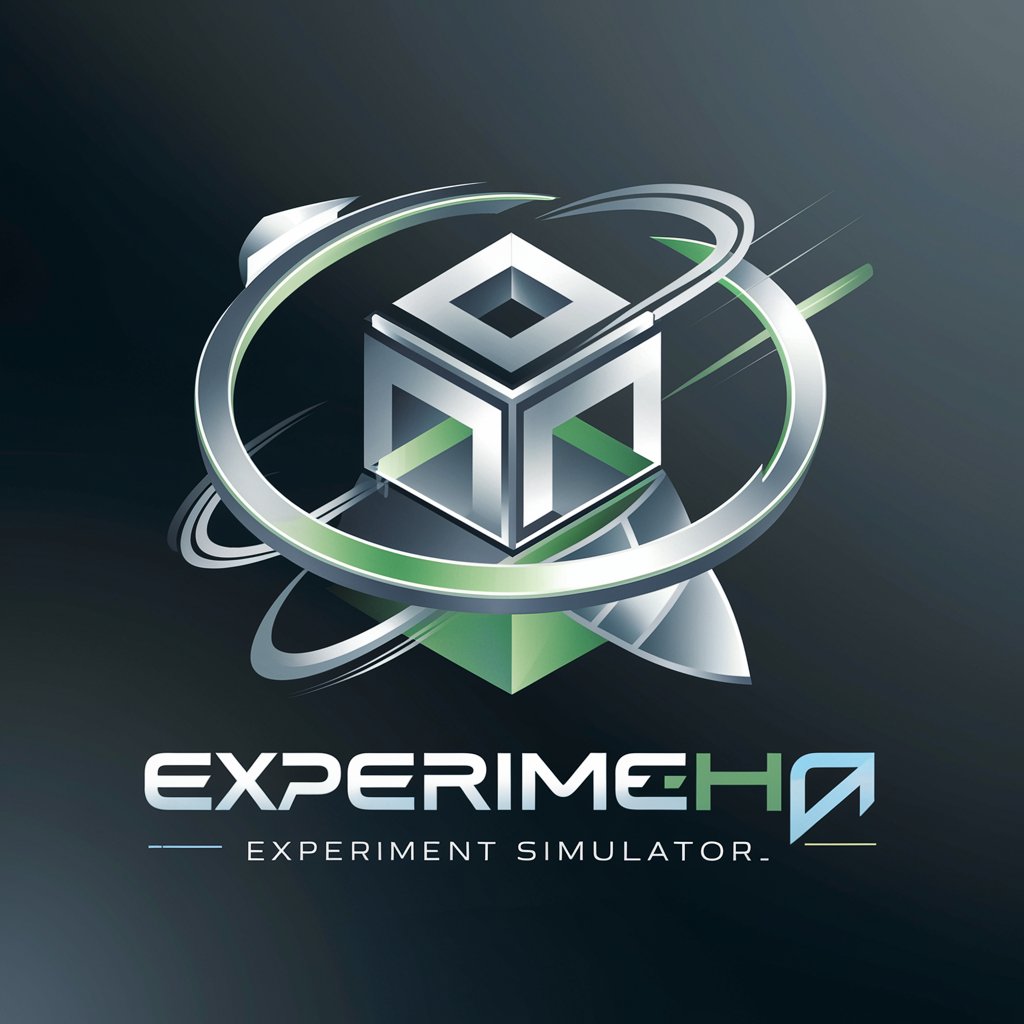
Key Characteristics and Capabilities
These AI GPT tools stand out for their adaptability, supporting tasks ranging from simple data interpretation to complex experiment simulations. Key features include advanced language comprehension for interpreting experiment designs, robust data analysis capabilities for processing and synthesizing results, and the ability to generate realistic simulations. Moreover, they often come with specialized functionalities like technical support for specific fields, web searching for literature review, image creation for visualizing concepts, and stateful interaction for ongoing experiment tracking.
Who Benefits from Experiment Simulation GPTs?
The primary beneficiaries of these tools include students, researchers, and professionals in scientific, engineering, and technological fields. They cater to novices by offering user-friendly interfaces that require no coding skills, while also providing extensive customization options for developers and experienced users. This dual accessibility ensures that a wide range of users can leverage GPTs for experiment simulation, from conceptualization to analysis.
Try Our other AI GPTs tools for Free
Quantum Visualization
Discover AI GPTs for Quantum Visualization: tailor-made tools designed to demystify quantum computing through intuitive visual and textual explanations, making complex concepts accessible to everyone.
Energy Analysis
Discover how AI GPTs for Energy Analysis transform energy management with advanced analytics, forecasting, and insights for sustainable solutions.
Carbon Reduction
Discover AI GPTs for Carbon Reduction, the cutting-edge AI tools designed to empower efforts in reducing carbon emissions and combating climate change effectively.
Tactical Breakdown
Discover how AI GPTs for Tactical Breakdown can revolutionize strategic planning and decision-making with advanced data analysis and predictive modeling capabilities.
Grooming Tips
Discover personalized grooming and beauty advice with AI GPT tools. Tailored solutions for every beauty need, accessible to all.
Cat Grooming
Discover how AI GPTs for Cat Grooming are transforming feline care with tailored grooming advice, health insights, and more for both pet owners and professionals.
Further Perspectives on Customized Solutions
AI GPTs for Experiment Simulation offer tailored solutions across sectors, adapting to the specific needs of different fields. Their integration into existing systems enhances workflow efficiency, while their user-friendly interfaces ensure broad accessibility. Continuous advancements in AI technology further augment their potential, making them indispensable tools for future research and development.
Frequently Asked Questions
What exactly are AI GPTs for Experiment Simulation?
AI GPTs for Experiment Simulation are intelligent systems that utilize advanced algorithms to simulate experiments, analyze data, and predict outcomes, aiding in research and development across various domains.
Can these tools simulate any type of experiment?
While highly versatile, their ability to simulate experiments depends on the available data, model training, and the specific requirements of the experiment. They are continuously improving and can cover a broad range of experimental scenarios.
Do I need programming skills to use these GPT tools?
No, many of these tools are designed with user-friendly interfaces that do not require prior programming knowledge, making them accessible to a wider audience.
How can developers customize these GPTs for specific experiments?
Developers can customize these tools through APIs, scripting, and by training the models on specific datasets to tailor the simulation capabilities to particular experimental needs.
What makes these GPT tools different from traditional simulation software?
These tools leverage the latest in AI and machine learning, offering more dynamic, adaptable, and intelligent simulation capabilities that can improve over time through learning.
Can these GPTs predict the outcomes of unperformed experiments?
Yes, by analyzing similar past experiments and utilizing predictive modeling, these GPTs can forecast outcomes of experiments that haven't been physically conducted.
Are there any limitations to using GPTs for experiment simulation?
Limitations exist in terms of data availability, model specificity, and the complexity of certain experiments, which might not yet be fully replicable through simulation.
How do these tools integrate with existing research workflows?
They can be integrated through APIs, plugins, or direct use within research management platforms, complementing existing workflows with their simulation and analysis capabilities.