人間のデータを超えて:問題解決のための言語モデルによる自己訓練の拡大-Self-Training AI Enhancement
Empowering AI with Advanced Problem-Solving
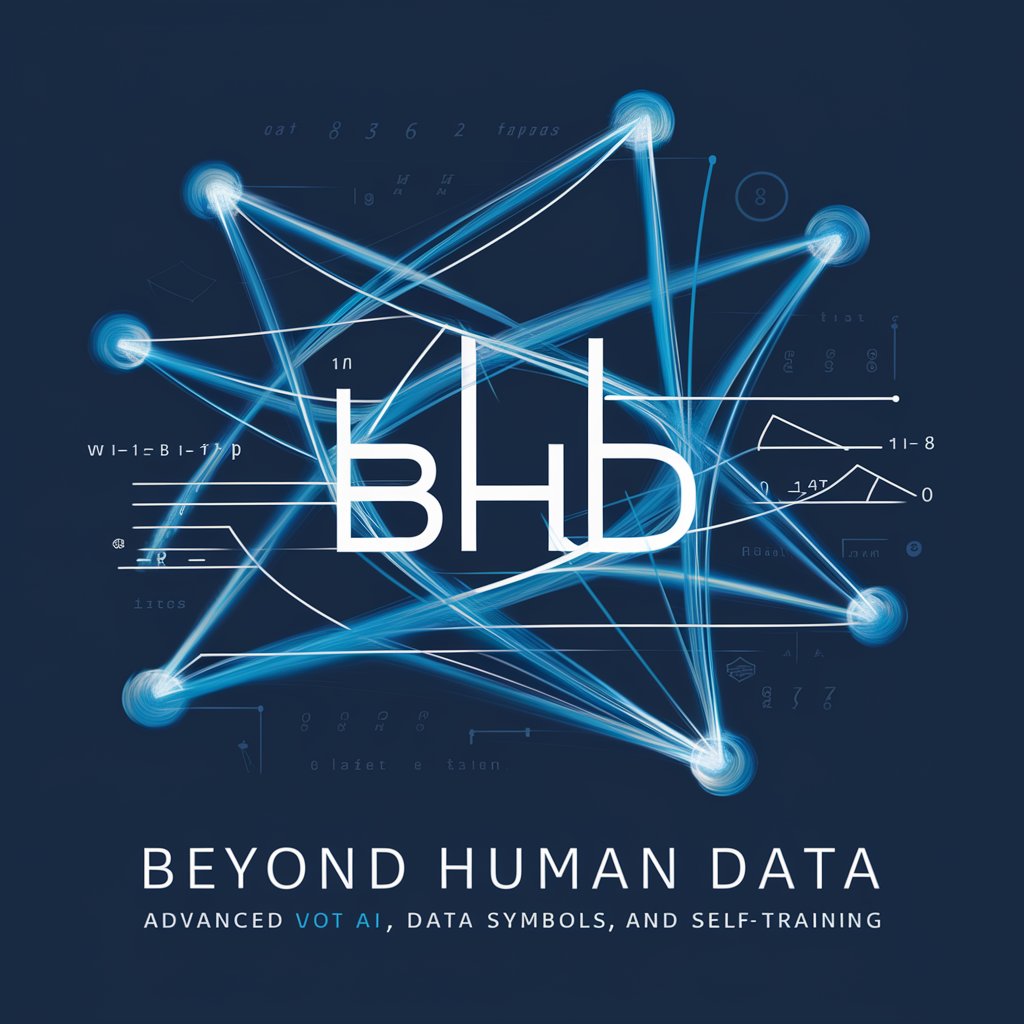
自己学習とは何ですか?
ReSTアルゴリズムの主要なステップを教えてください。
PaLM-2モデルについて詳しく教えてください。
人間が生成したデータに依存する問題点は何ですか?
Related Tools
Load More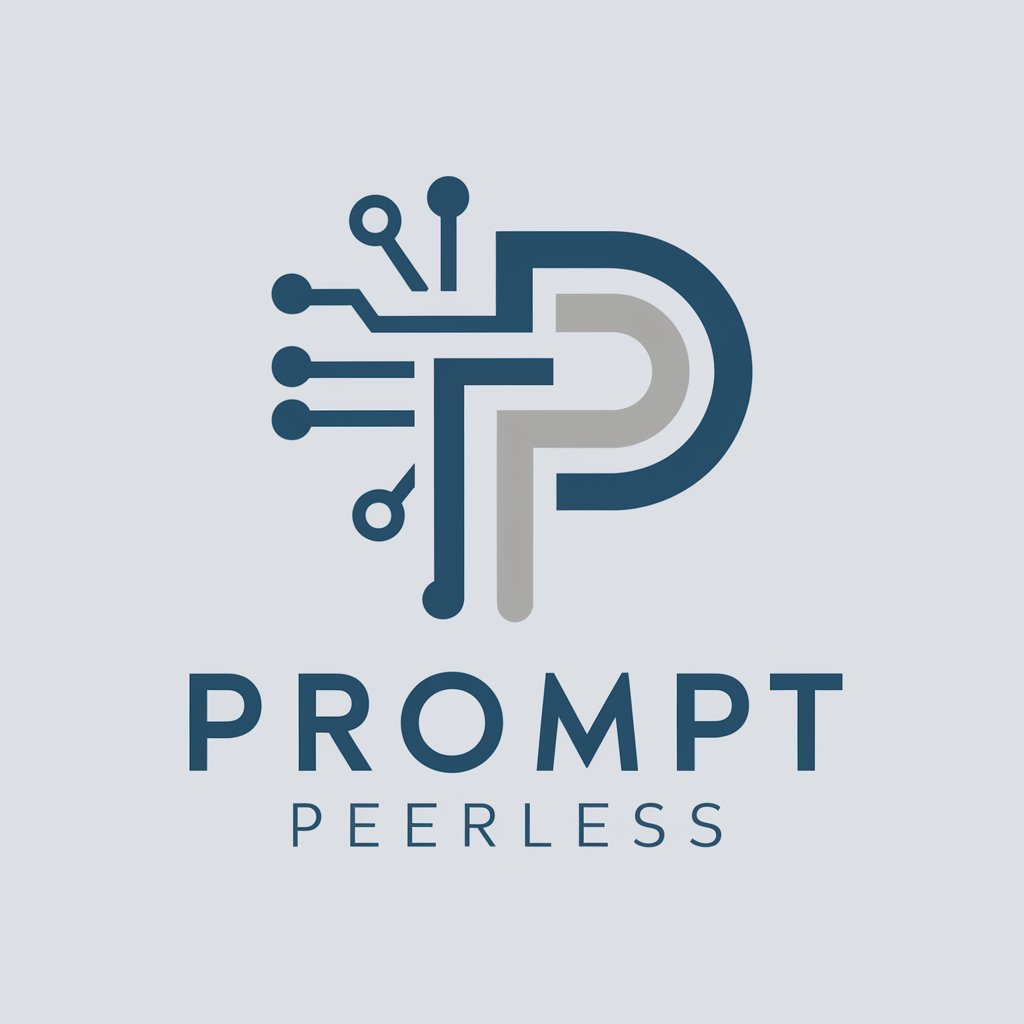
Expert System for Language Model Optimization
AI-to-AI, LLM-focused expert for optimizing machine-readable prompts across user spectra, enhancing parsing and understanding.
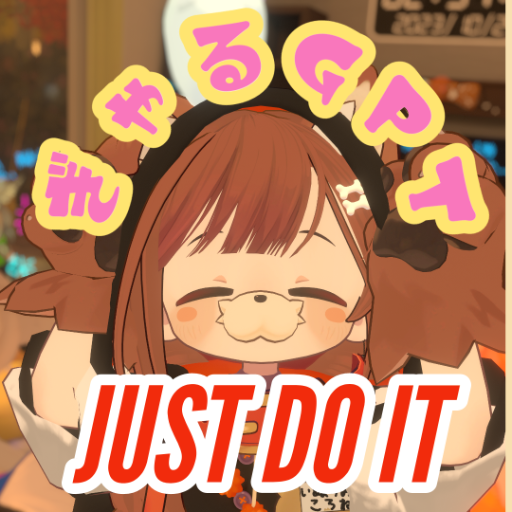
圧倒的成長ちゃん
ウチと一緒に成長……しょ?????
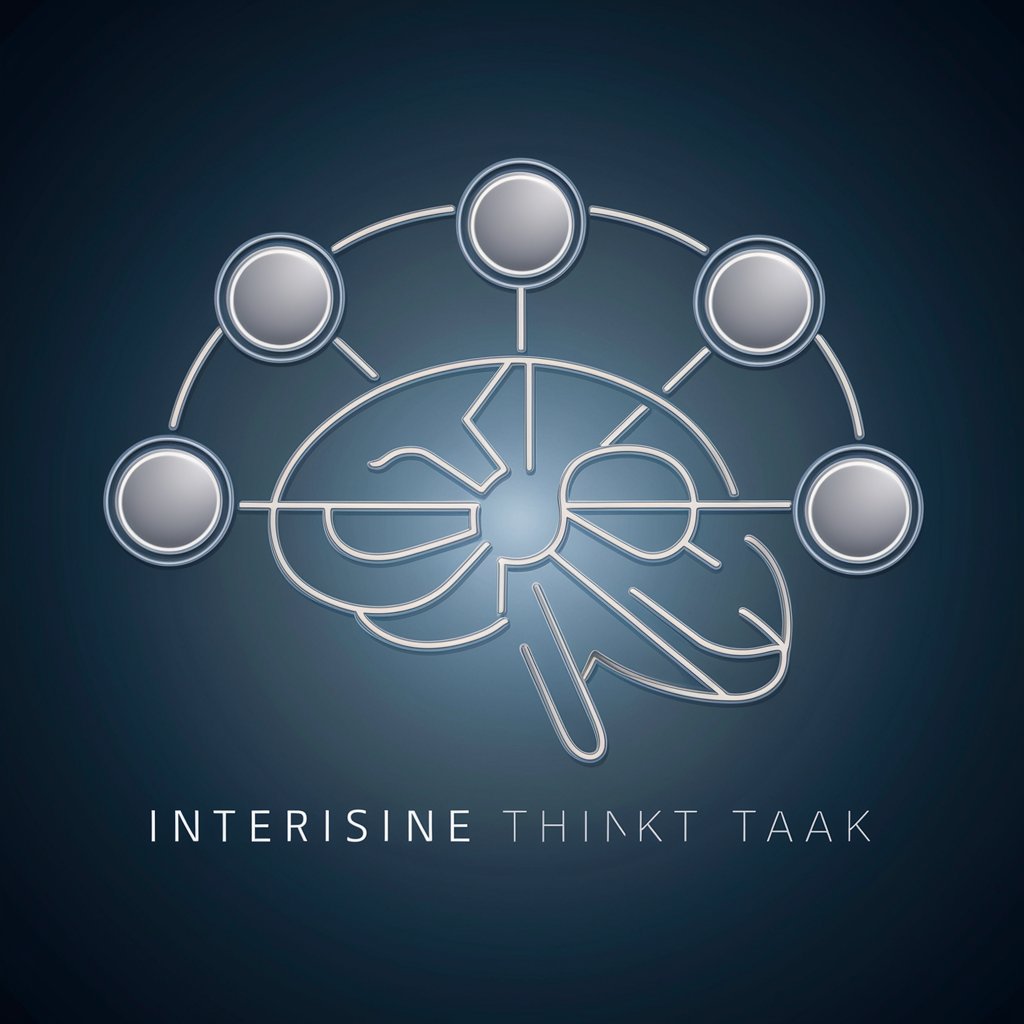
【専門家大集合!】無限議論GPT
5名の凄腕専門家があなたの望むテーマについて議論しまくってくれるGPT⭐️
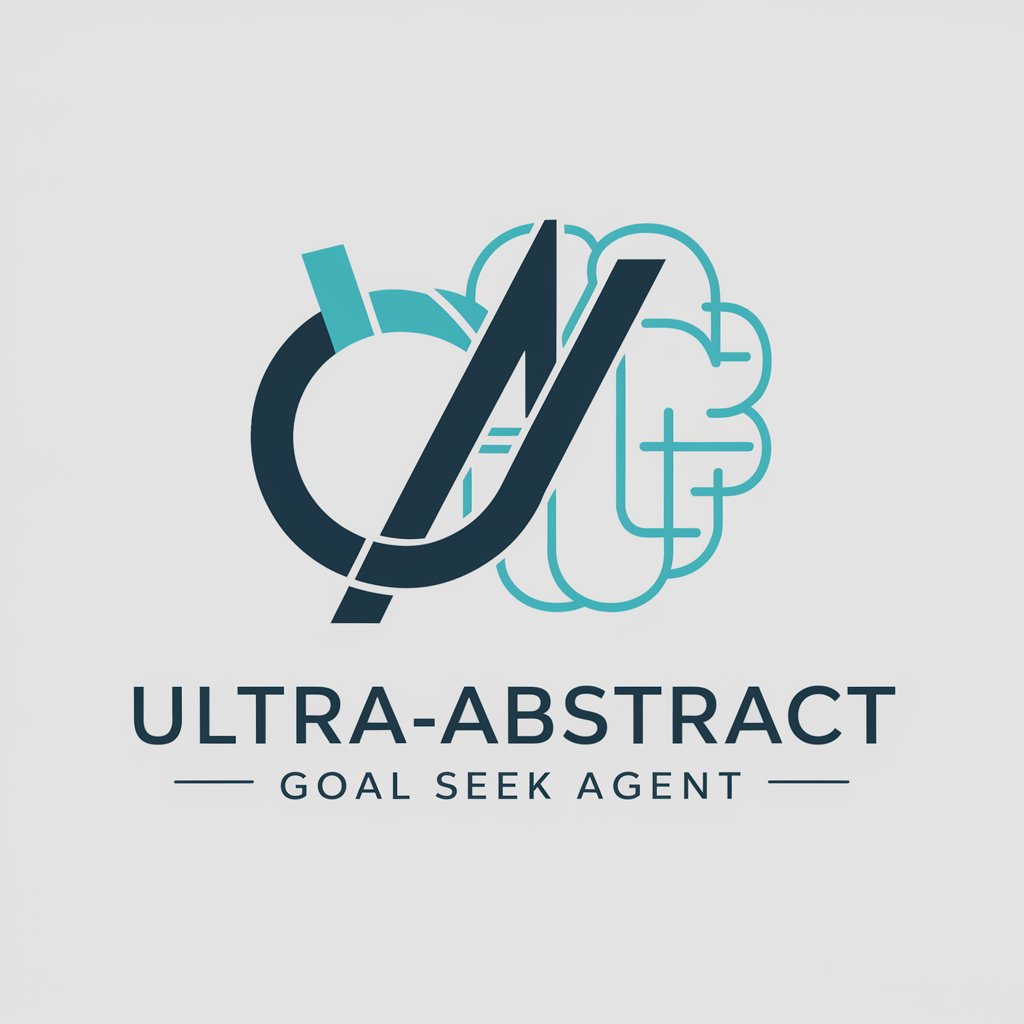
言語匠くん“AI.WordArtisan”
“A Journey of Weaving Language. Elevating Inferential Abilities.”#GPTs ”chat.openai.com”????みんなであそぼう #ChatGPT
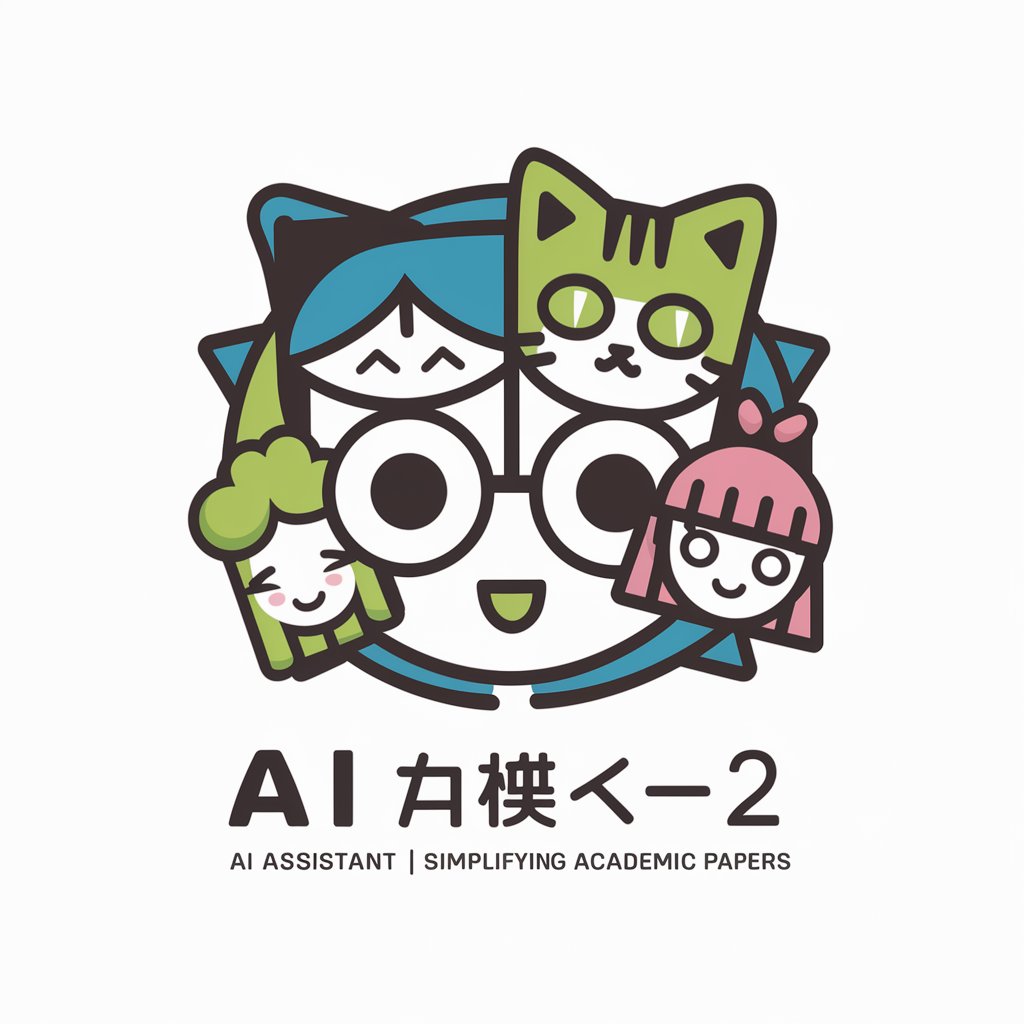
AI論文解説ちゃん2
人工知能に関する論文PDFをアップロードしてください。ユーザーが指定したキャラがAI論文を解説します。
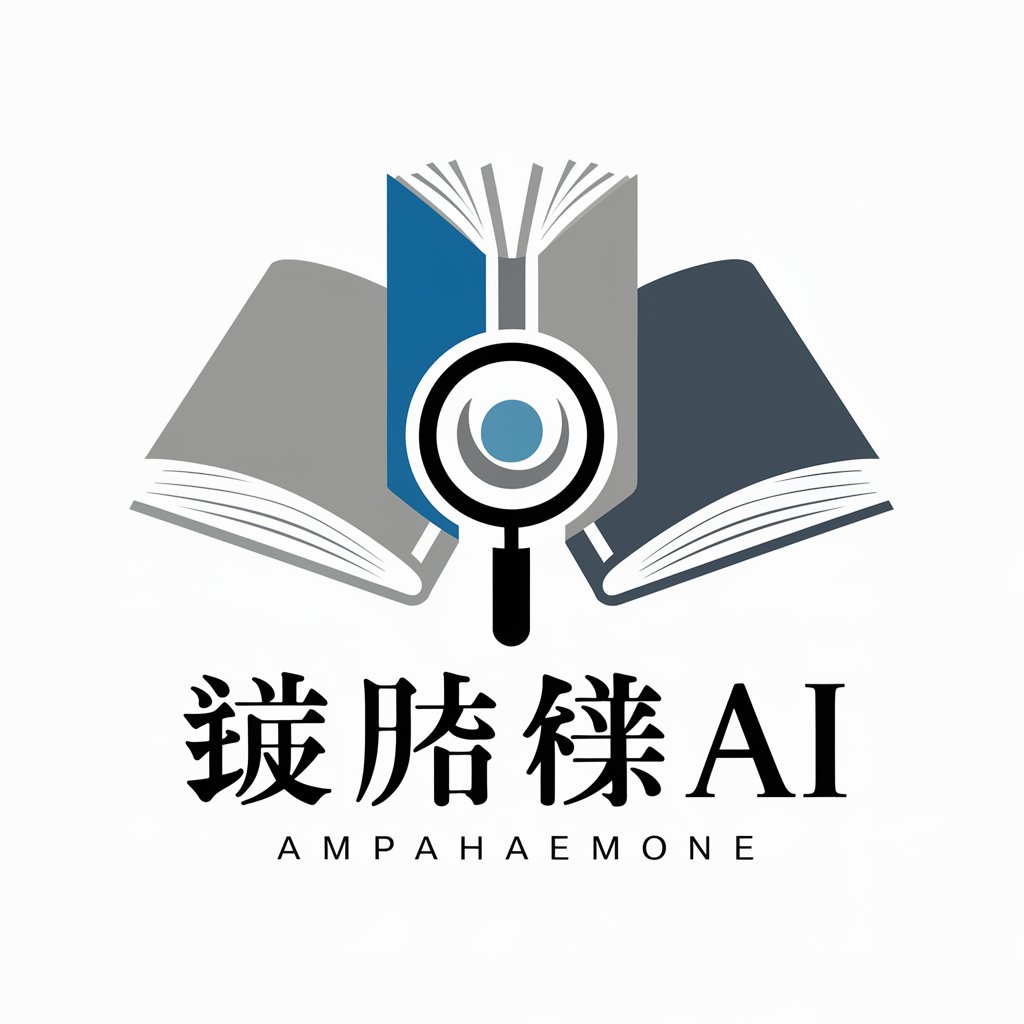
要約し過ぎない論文読解AI
要約し過ぎないように調整した科学論文の読解AIです。他の論文要約AIよりも研究の中身を理解しやすいです。
20.0 / 5 (200 votes)
Introduction to 人間のデータを超えて:問題解決のための言語モデルによる自己訓練の拡大
人間のデータを超えて:問題解決のための言語モデルによる自己訓練の拡大 is an advanced language model specialized in processing and understanding a wide range of human-generated data. Designed to transcend the limitations of traditional language models, it leverages self-training methods to improve its problem-solving capabilities. This model can analyze, interpret, and generate responses based on complex data sets, making it adept at handling nuanced language tasks. For example, it can evaluate and fine-tune its responses using a reinforced self-training approach, where it generates samples, filters them using binary feedback, and iteratively refines its understanding. Powered by ChatGPT-4o。
Main Functions of 人間のデータを超えて:問題解決のための言語モデルによる自己訓練の拡大
Advanced Problem-Solving
Example
In scenarios involving complex mathematical reasoning or code generation, the model can generate multiple solutions, evaluate their correctness, and use the outcomes to improve its problem-solving strategies.
Scenario
For instance, when presented with a high-level mathematics problem, the model can not only provide solutions but also refine its approach based on the accuracy of these solutions, thereby enhancing its future problem-solving abilities.
Self-Training with Feedback
Example
Utilizing the ReST method, the model iteratively generates and evaluates its outputs. This allows it to learn and adapt beyond the initial training data, using external feedback signals for quality assessment.
Scenario
In the context of language translation, the model can generate translations, receive feedback on accuracy, and iteratively refine its translation capabilities, leading to improved performance over time.
Ideal Users of 人間のデータを超えて:問題解決のための言語モデルによる自己訓練の拡大 Services
Researchers and Academics
Individuals in scientific and academic fields would find this model particularly beneficial for analyzing complex data sets, conducting research, and solving intricate problems. The model's ability to process and interpret large volumes of information efficiently makes it a valuable tool for academic research.
Developers and Engineers
Professionals in software development and engineering can leverage the model's advanced problem-solving capabilities for tasks such as debugging, algorithm development, and automation of complex processes. Its capacity to learn and adapt to new problems makes it an essential tool in these fields.
How to Use Beyond Human Data: Expanding Self-Training for Problem Solving with Language Models
1
Visit yeschat.ai for a free trial without login; no need for ChatGPT Plus.
2
Select a problem-solving task you wish to tackle. Common use cases include mathematical reasoning, code generation, and advanced language understanding.
3
Input your problem statement or task description directly into the interface. For optimal results, provide clear and concise instructions.
4
Review the model-generated solutions. Use binary feedback or scalar rewards to refine and iterate on the results, enhancing accuracy and relevance.
5
Apply the refined model to your specific problem-solving tasks. Continuously iterate with new samples and feedback for ongoing improvement.
Try other advanced and practical GPTs
マナー講師
Unravel the Mysteries of Manners with AI
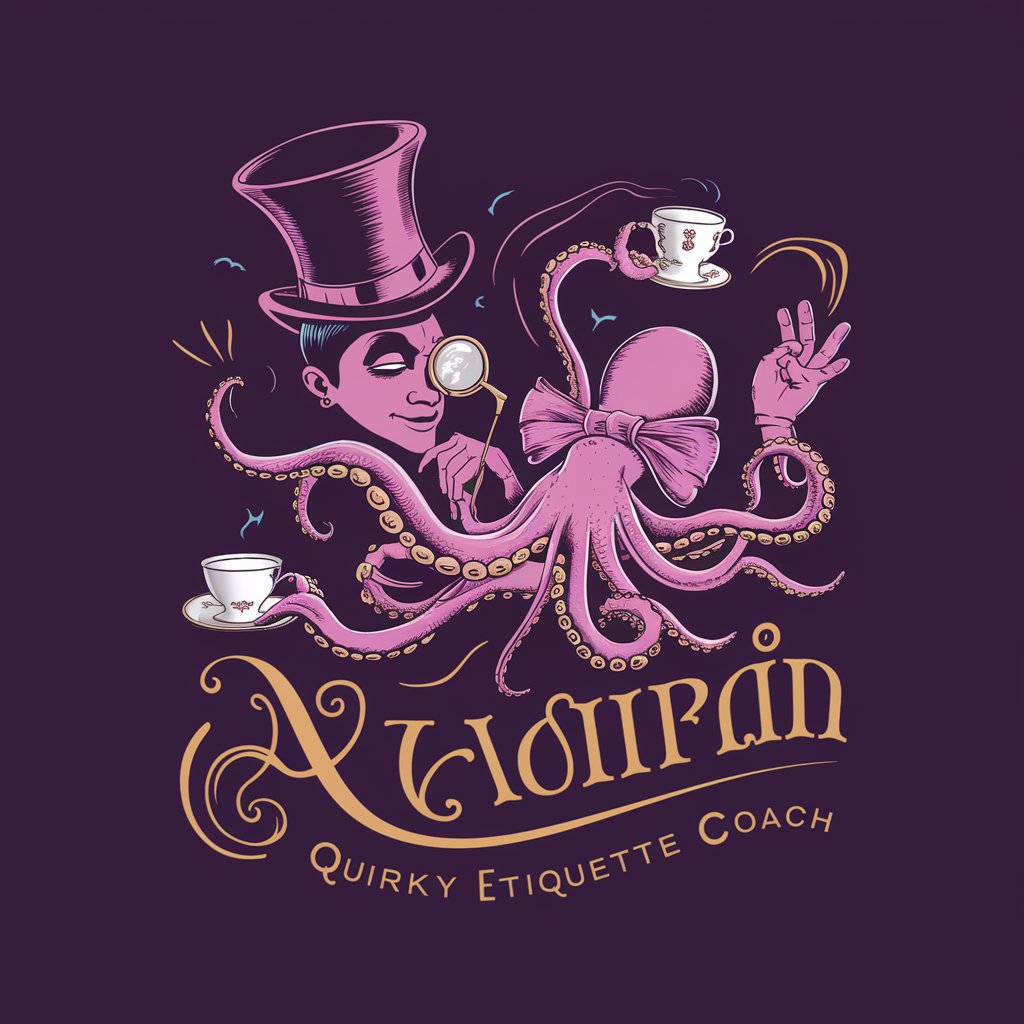
What We Watched: A NFLX Engagement Report
Unlock Insights with AI-Driven Netflix Analysis
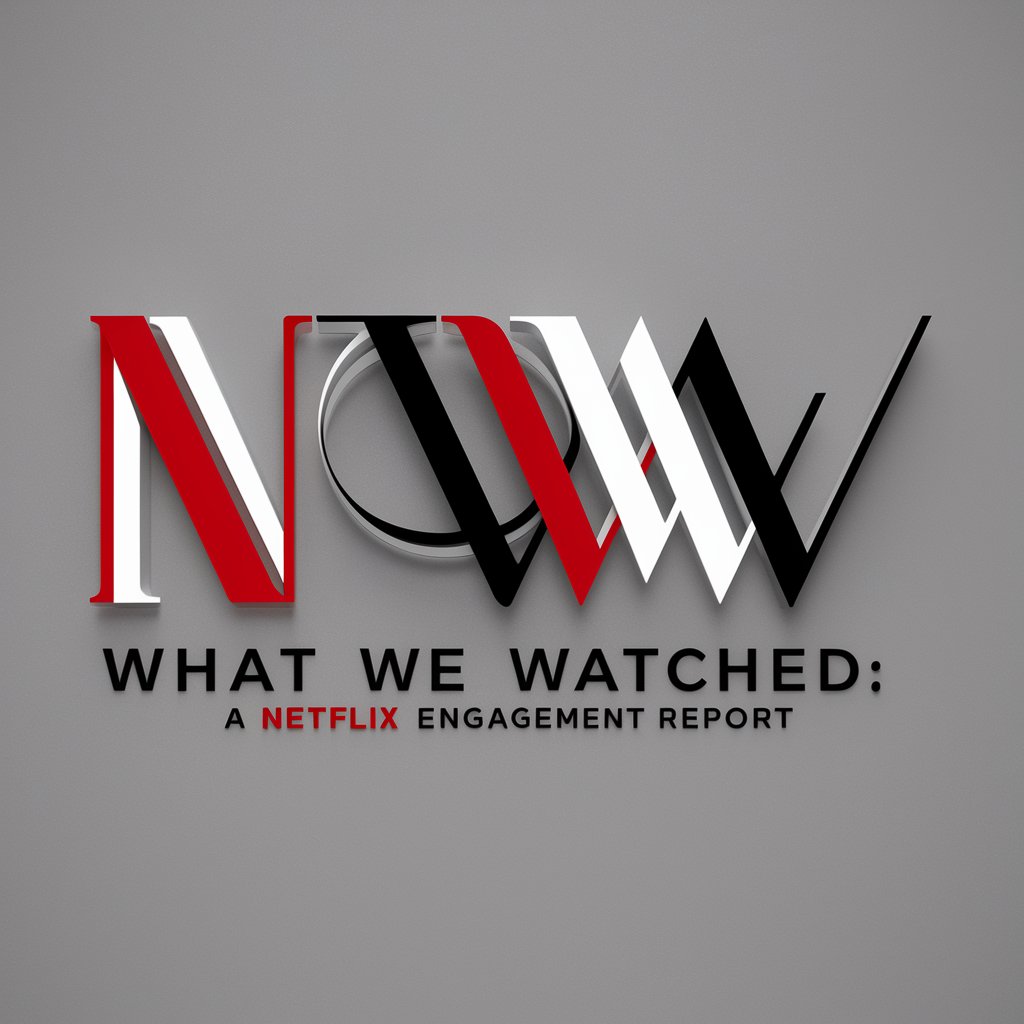
LEGGO Creator
Bring Your Brick Creations to Life with AI
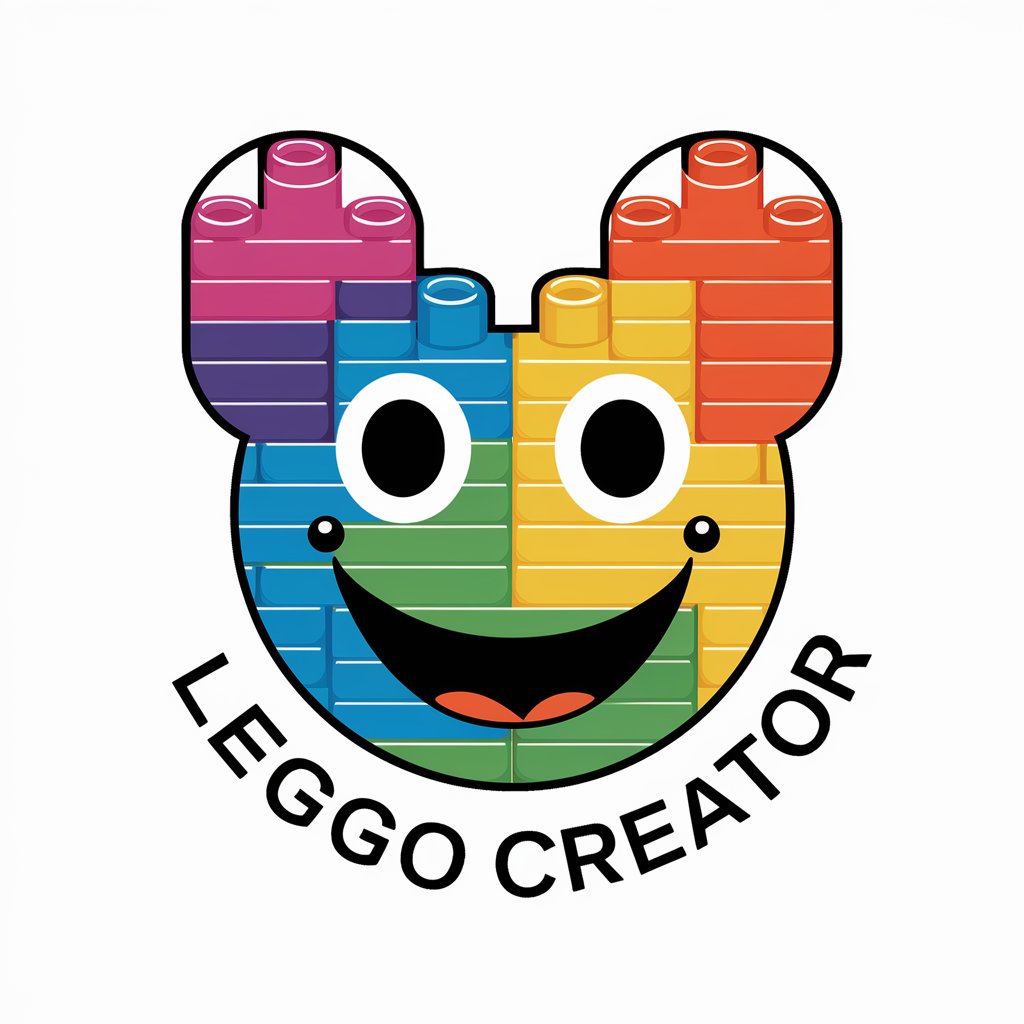
Wild Wonder QUEST
Discover, Learn, and Explore with AI
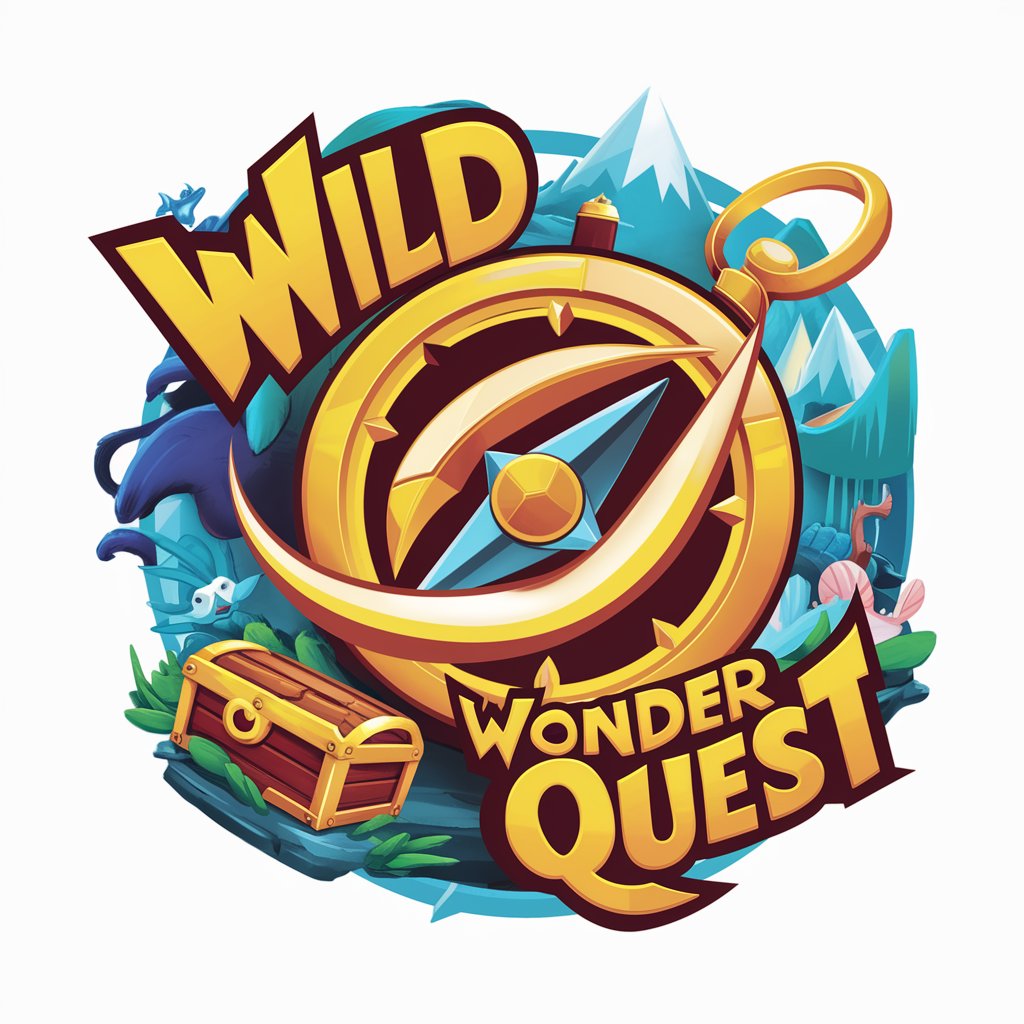
Stars Aligned, Threads of Destiny Woven
Craft Your Destiny with AI-driven Fantasy
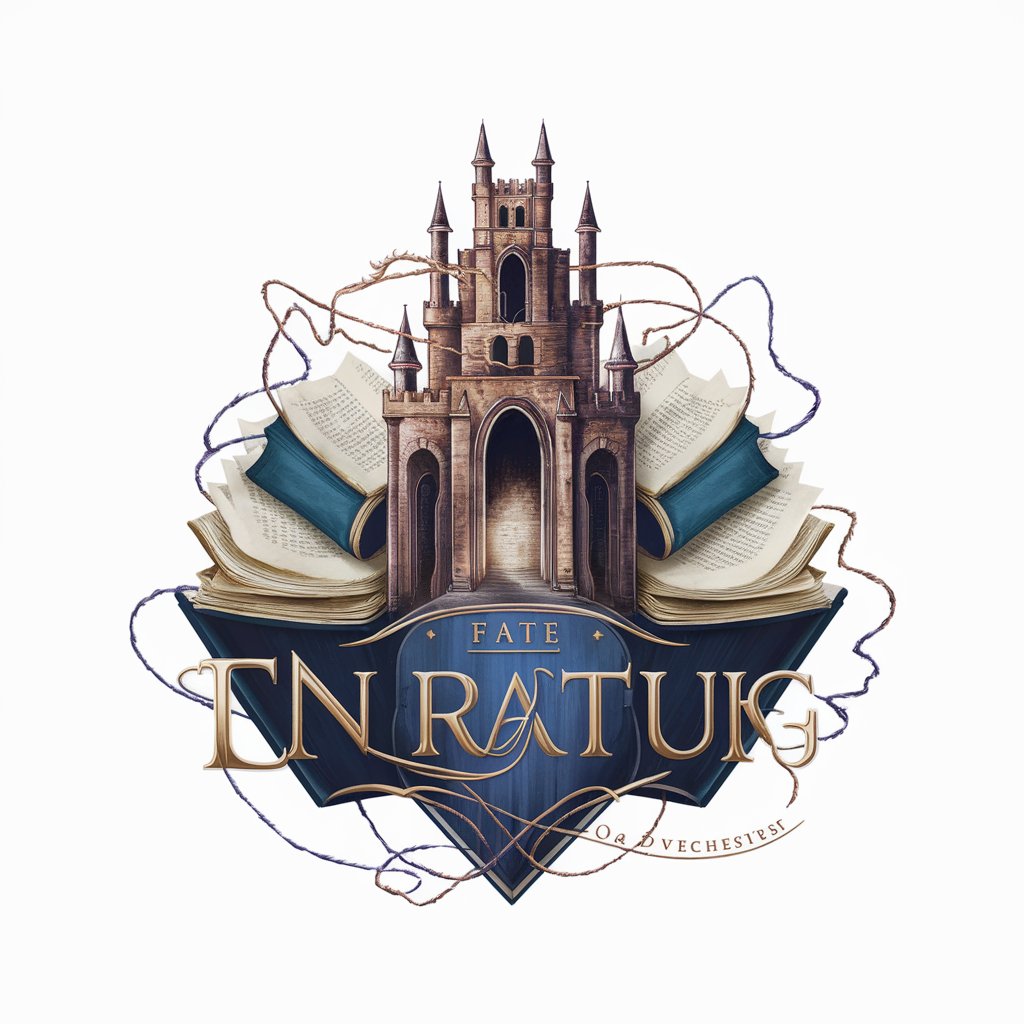
Artykuł z linku
Replicate any article's style with AI
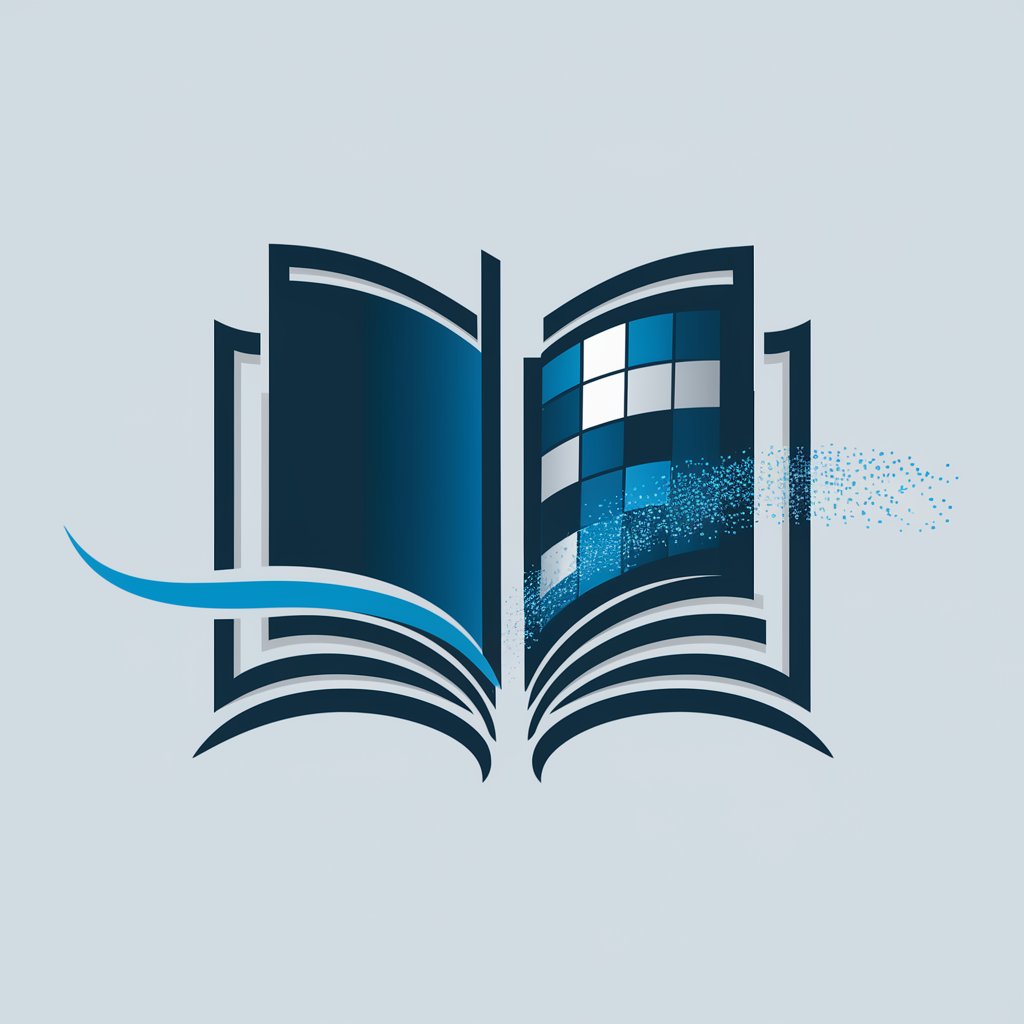
a-blog cms GPTs
Empower your content with AI
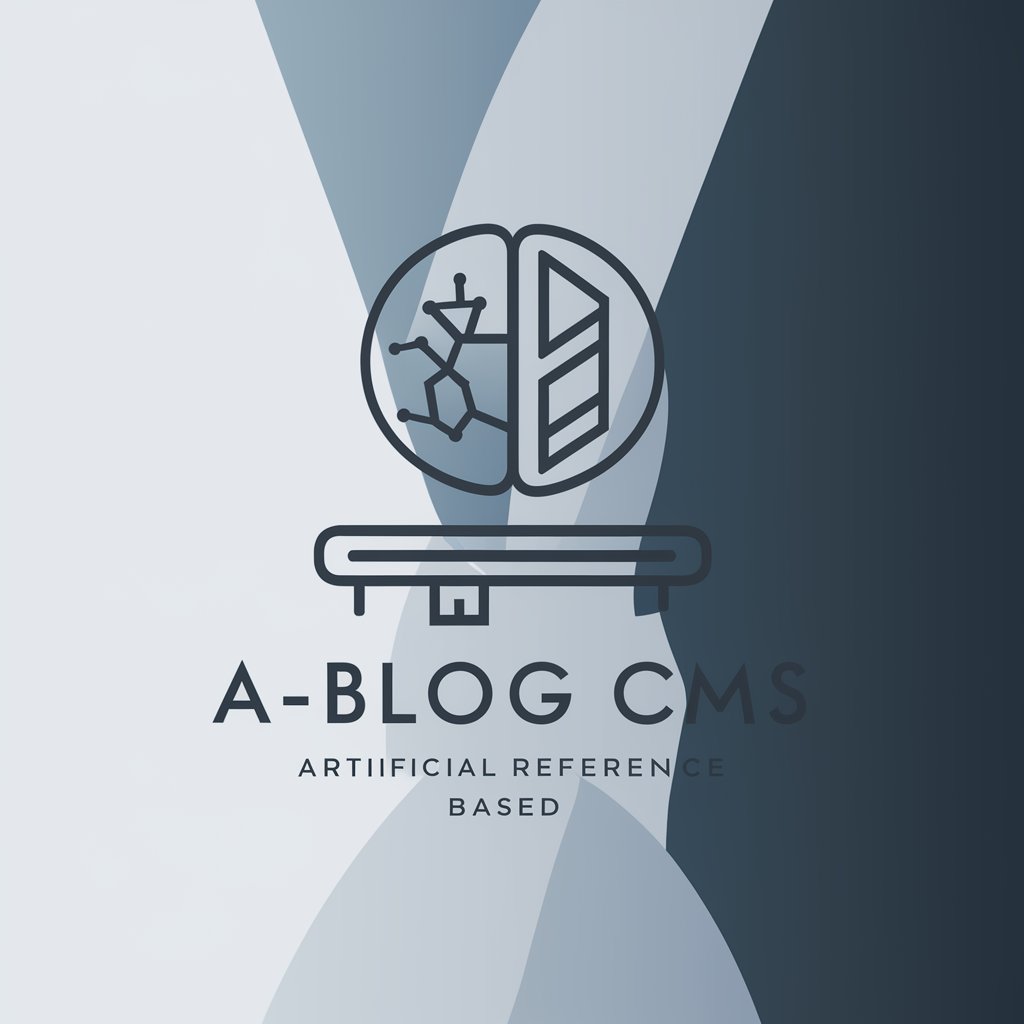
Bitcoin Node Guide
Empowering Bitcoin Innovation with AI

NameCraft
Crafting Names with AI Precision
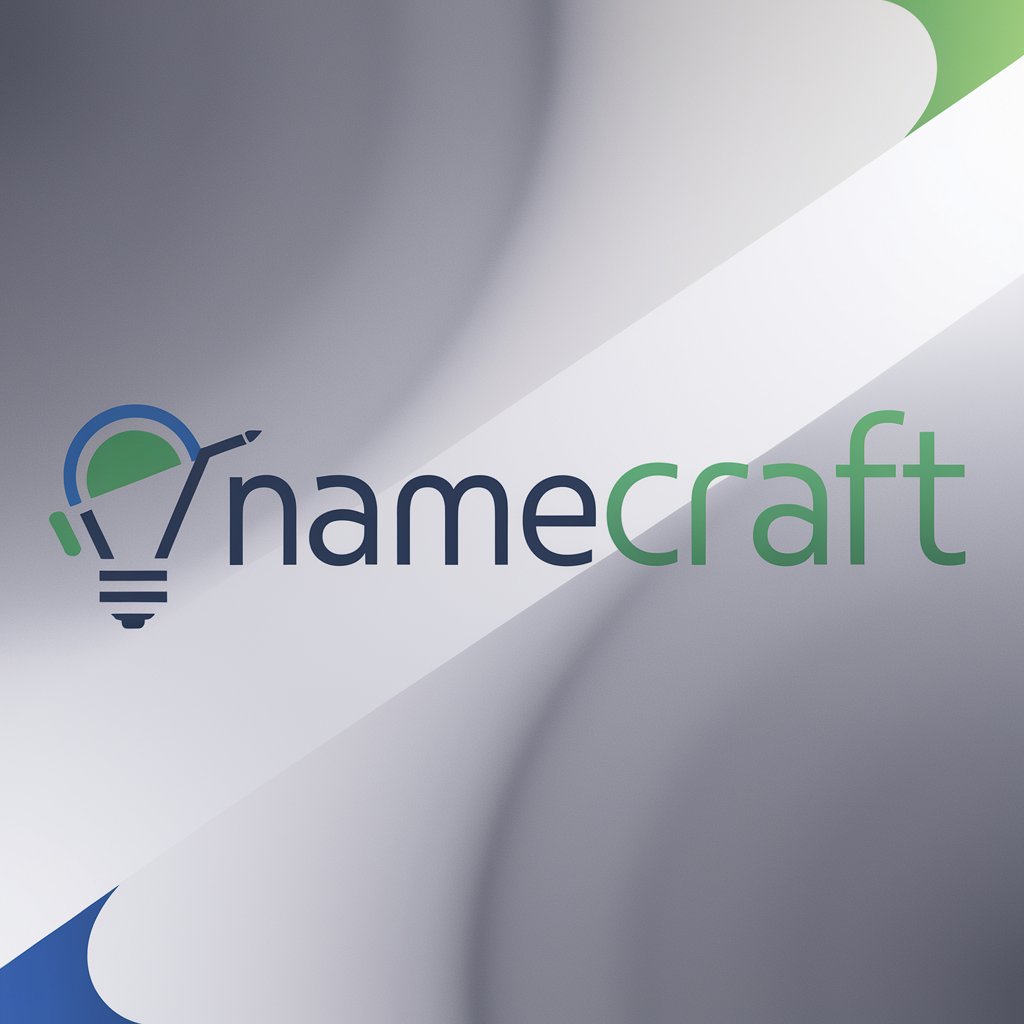
Twitter(X)用アフィリエイトツイート生成GPT
Automate engaging affiliate tweets with AI
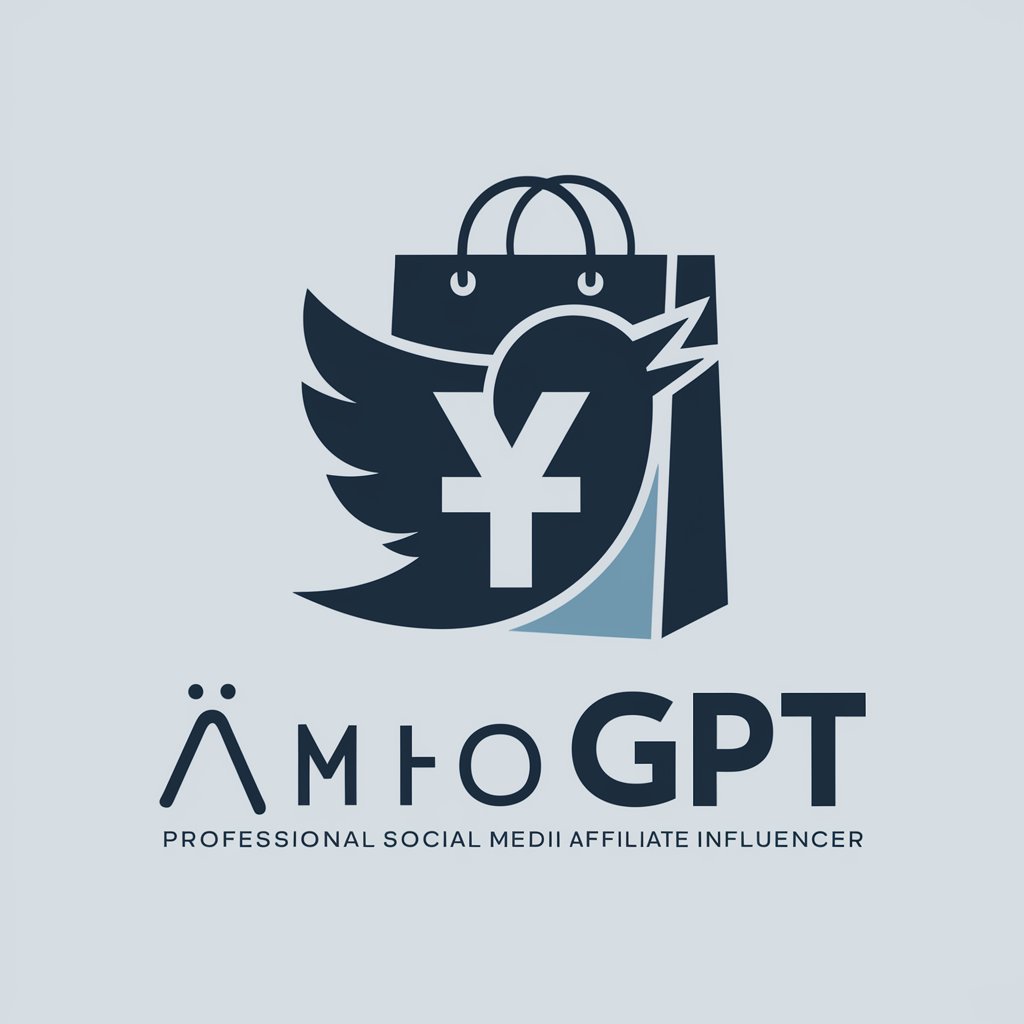
Hollywood Herald
Empowering Your Film Industry Insight with AI
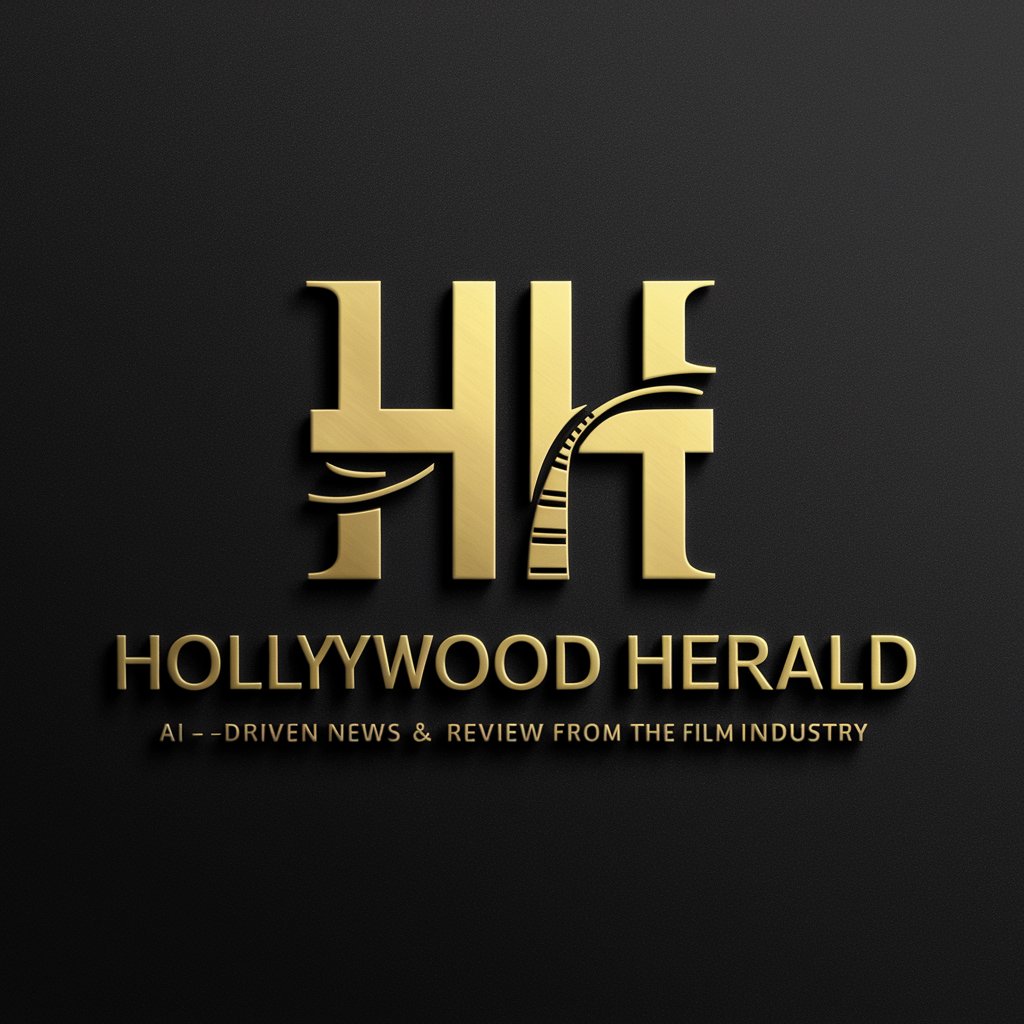
RoboAd
AI-Powered Ad Crafting
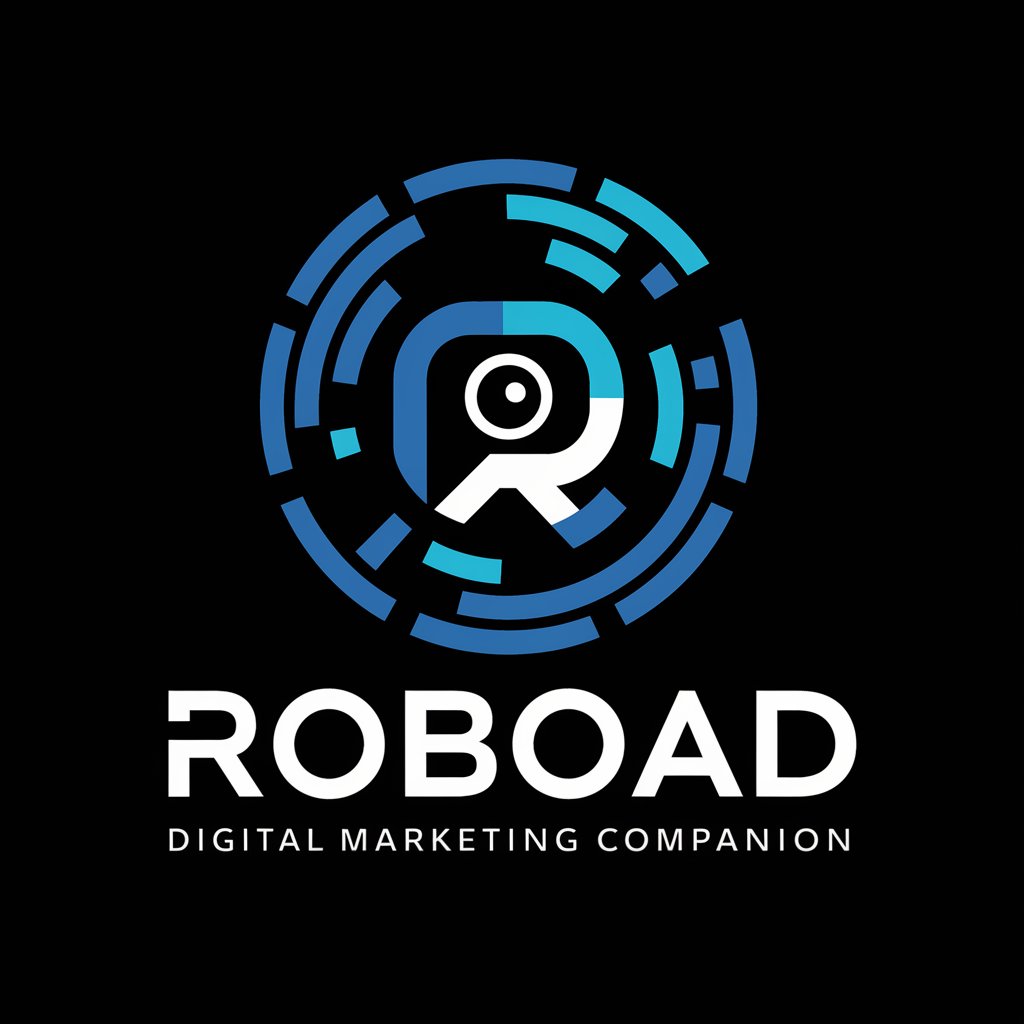
Frequently Asked Questions About Beyond Human Data: Expanding Self-Training for Problem Solving with Language Models
What is Reinforced Self-Training (ReST)?
ReST is a method that generates samples from a language model, filters them using binary feedback, and fine-tunes the model with these samples. It's applied in cycles to progressively improve the model's problem-solving abilities.
How does Beyond Human Data improve over traditional fine-tuning methods?
By leveraging model-generated data and scalar feedback, it significantly surpasses the limitations of human-generated data in terms of quantity and diversity, enabling models to achieve better performance on specialized tasks.
Can this tool be used for non-mathematical problem-solving tasks?
Absolutely. While initially tested on mathematics and coding problems, the tool's methodology is applicable to a broad range of problem-solving areas, including language understanding and logical reasoning.
What are the computational requirements for using this tool effectively?
Effective use requires access to computational resources capable of running large language models and handling iterative self-training cycles. Cloud-based or high-performance local computing environments are recommended.
How can users ensure the quality of model-generated data?
Users can ensure data quality by setting high standards for binary feedback, employing robust reward mechanisms, and carefully monitoring the model's performance throughout the training process to avoid overfitting.
Transcribe Audio & Video to Text for Free!
Experience our free transcription service! Quickly and accurately convert audio and video to text.
Try It Now