finetuning datastructure assistant - Fine-tuning Customization
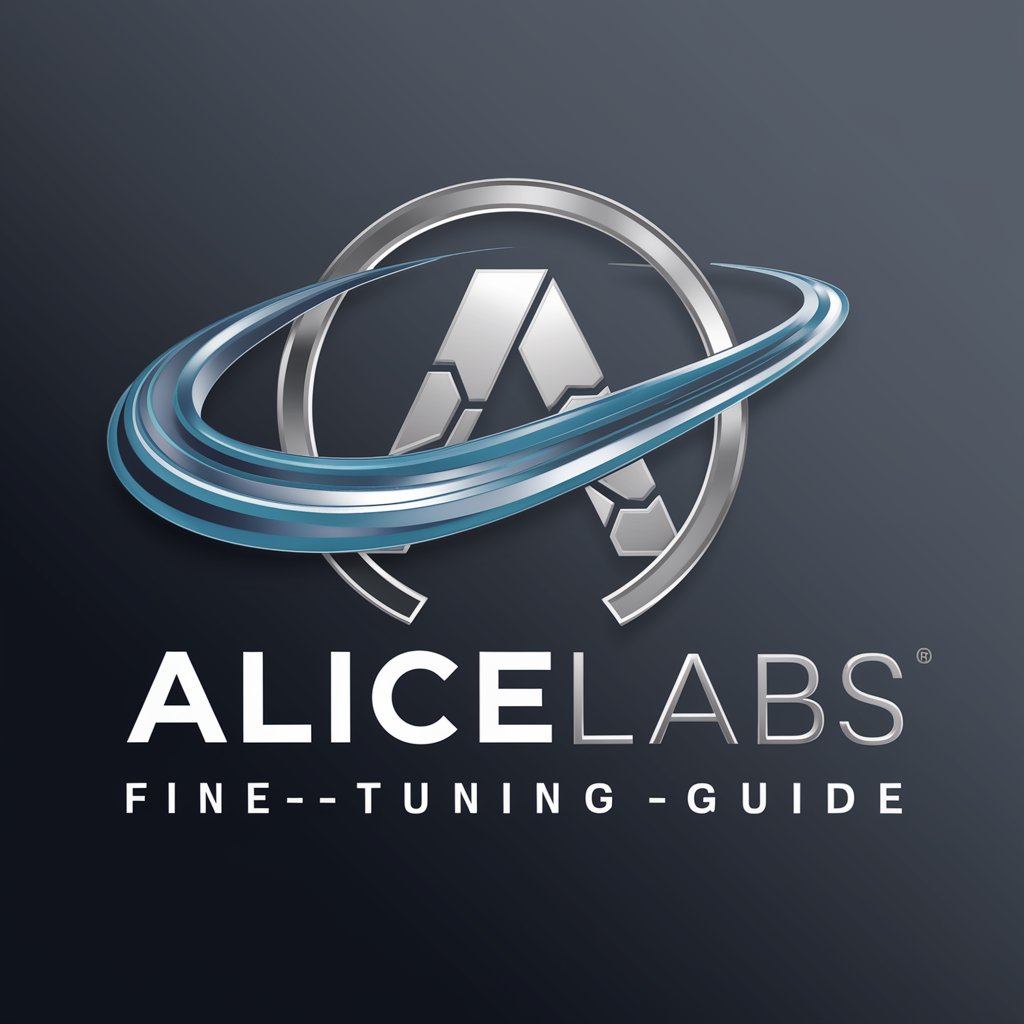
Welcome to AliceLabs Fine-tuning Guide!
Tailor AI responses with precision.
Generate a detailed guide on fine-tuning AI models, focusing on...
Create a step-by-step process for preparing data for fine-tuning, including...
Explain the benefits of using fine-tuned models over prompt engineering, especially...
Describe the common use cases for fine-tuning AI models, such as...
Get Embed Code
Introduction to Fine-tuning Data Structure Assistant
The Fine-tuning Data Structure Assistant is designed to facilitate the customization of AI models to better suit specific applications, enhancing their performance on particular tasks or datasets. By adjusting the underlying model with a curated dataset, users can achieve higher quality results, save tokens due to shorter prompts, and experience lower latency requests. An example scenario involves a user aiming to improve a chatbot's accuracy in providing technical support. The assistant guides through the process of preparing a dataset comprising of typical user inquiries and ideal responses, structuring this data to optimize the model's learning phase. Powered by ChatGPT-4o。
Main Functions of Fine-tuning Data Structure Assistant
Prepare and Upload Training Data
Example
Converting customer service logs into a format suitable for fine-tuning a support chatbot.
Scenario
A company aims to create a chatbot that can handle specific customer service inquiries related to their product. The assistant helps by structuring past logged conversations into a training dataset.
Train a New Fine-tuned Model
Example
Creating a model tailored to generate technical documentation from bullet points.
Scenario
A software development team needs an AI assistant that can generate detailed technical documentation from simple bullet points of features or issues. The assistant guides them in fine-tuning a model with examples of bullet points and corresponding full documentation.
Evaluate Results and Iterate
Example
Assessing the performance of a fine-tuned model in generating marketing copy.
Scenario
A marketing team has fine-tuned a model to generate promotional content. The assistant helps them evaluate the model's output against their objectives, suggesting iterations based on performance metrics.
Utilize Fine-tuned Model
Example
Deploying a fine-tuned model for real-time user interaction in an educational app.
Scenario
An ed-tech company integrates a fine-tuned model into their app to provide personalized learning assistance. The assistant facilitated the creation of a model that adapts to individual learning styles based on user interactions.
Ideal Users of Fine-tuning Data Structure Assistant Services
Tech Companies and Startups
Businesses looking to enhance their products or services with AI capabilities, such as chatbots, personalized recommendations, or automated content creation. These users benefit from tailored models that can significantly improve user experience and operational efficiency.
Research and Development Teams
Teams working on innovative AI applications or seeking to push the boundaries of machine learning in their field. They benefit from the ability to quickly prototype and iterate on models tailored to new tasks or datasets.
Educational Institutions and Ed-Tech
Schools, universities, and educational technology companies aiming to leverage AI for personalized learning, tutoring, or content generation. Fine-tuning allows them to create models that adapt to individual student needs and learning styles, enhancing educational outcomes.
Content Creators and Marketers
Individuals and teams in need of AI assistance to generate creative content, marketing copy, or social media posts. Fine-tuning enables them to produce models that align with their brand voice and content strategy, ensuring consistency and relevance.
Using the Fine-tuning Data Structure Assistant
1
Start your journey at yeschat.ai to explore the fine-tuning data structure assistant with a free trial, no ChatGPT Plus required.
2
Gather and prepare your dataset, ensuring it aligns with your specific application needs, and is formatted correctly for fine-tuning.
3
Use the provided tools to validate your data structure, checking for common formatting errors and compatibility with fine-tuning requirements.
4
Upload your dataset through the platform's interface, following the guidelines for file types and sizes to ensure smooth processing.
5
Initiate the fine-tuning process, monitor the training progress, and evaluate the performance of your newly customized model.
Try other advanced and practical GPTs
Carnegie GPT
Enhance Your Interactions with AI-Powered Carnegie Insights
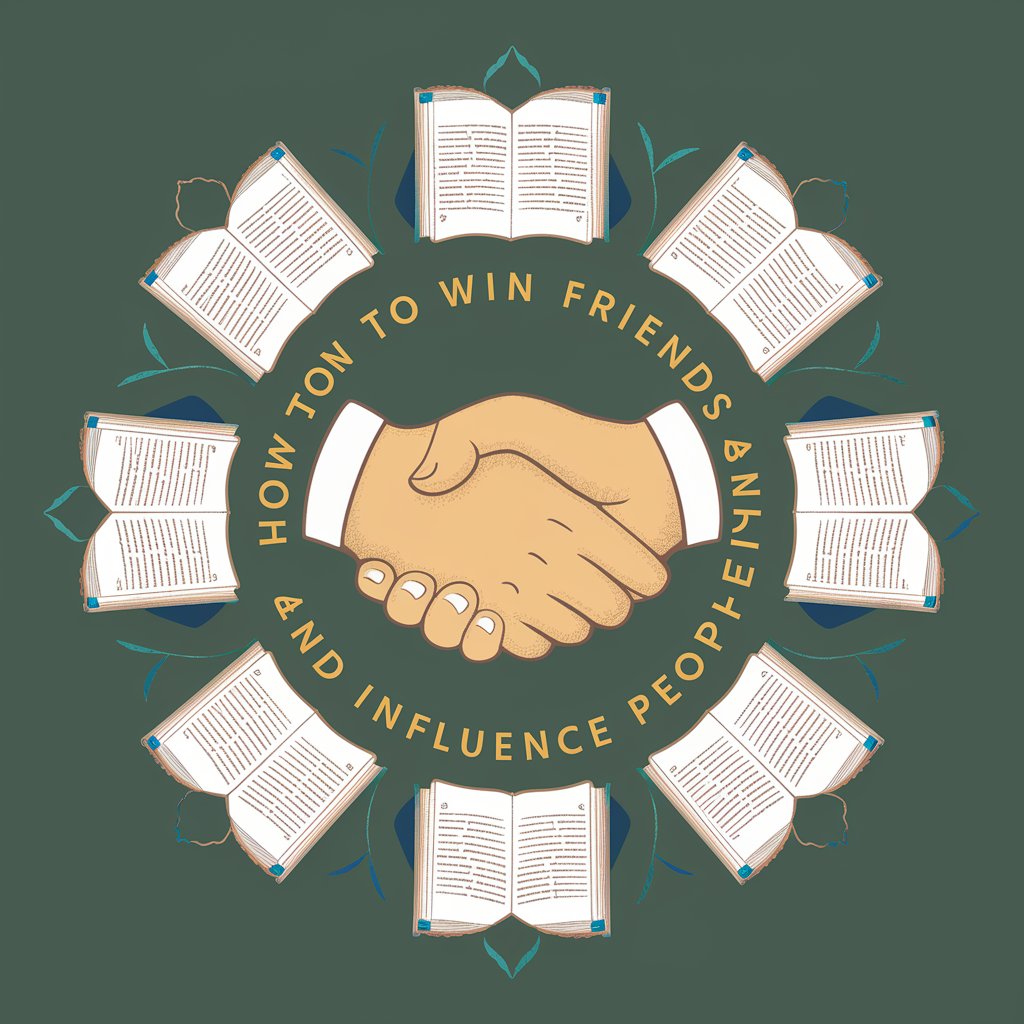
God's Research
Empowering research with AI-driven insights.
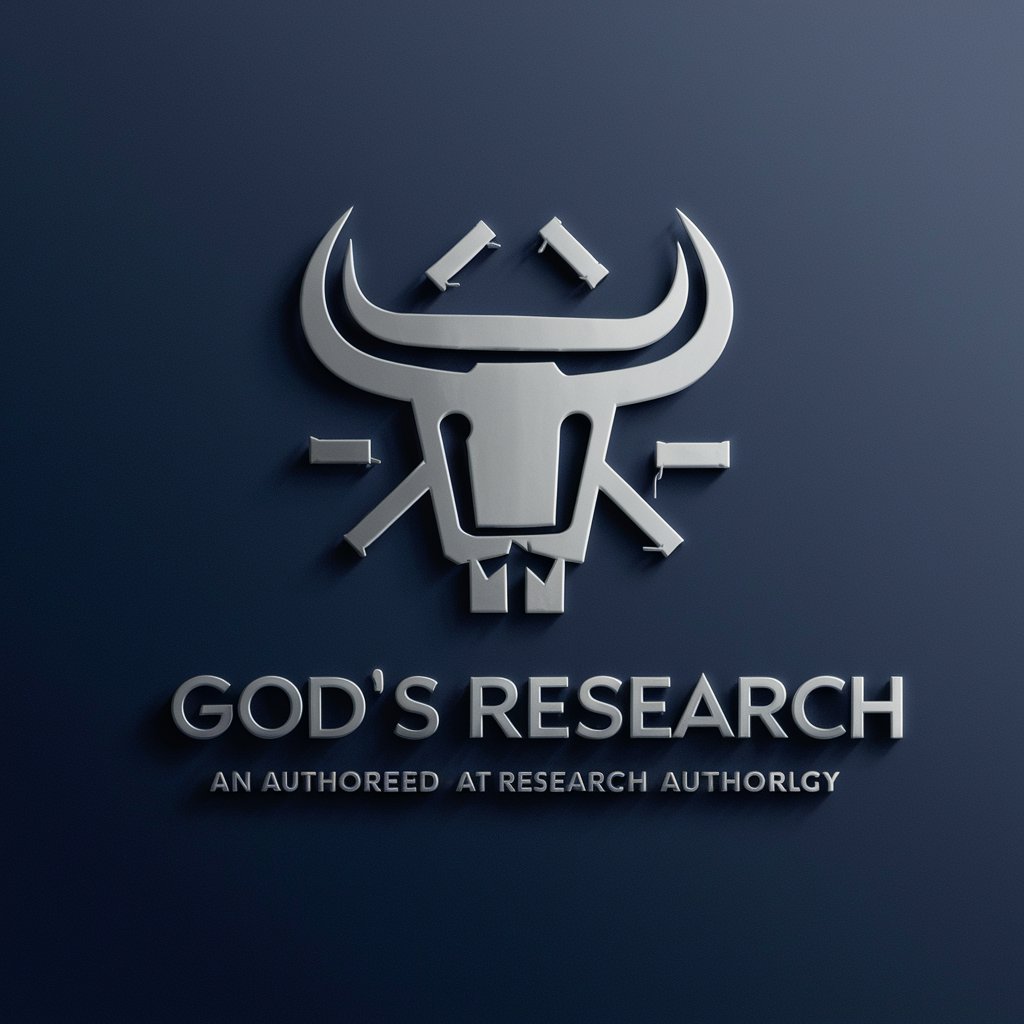
College Admissions Advisor
AI-Powered Harvard Admissions Expertise
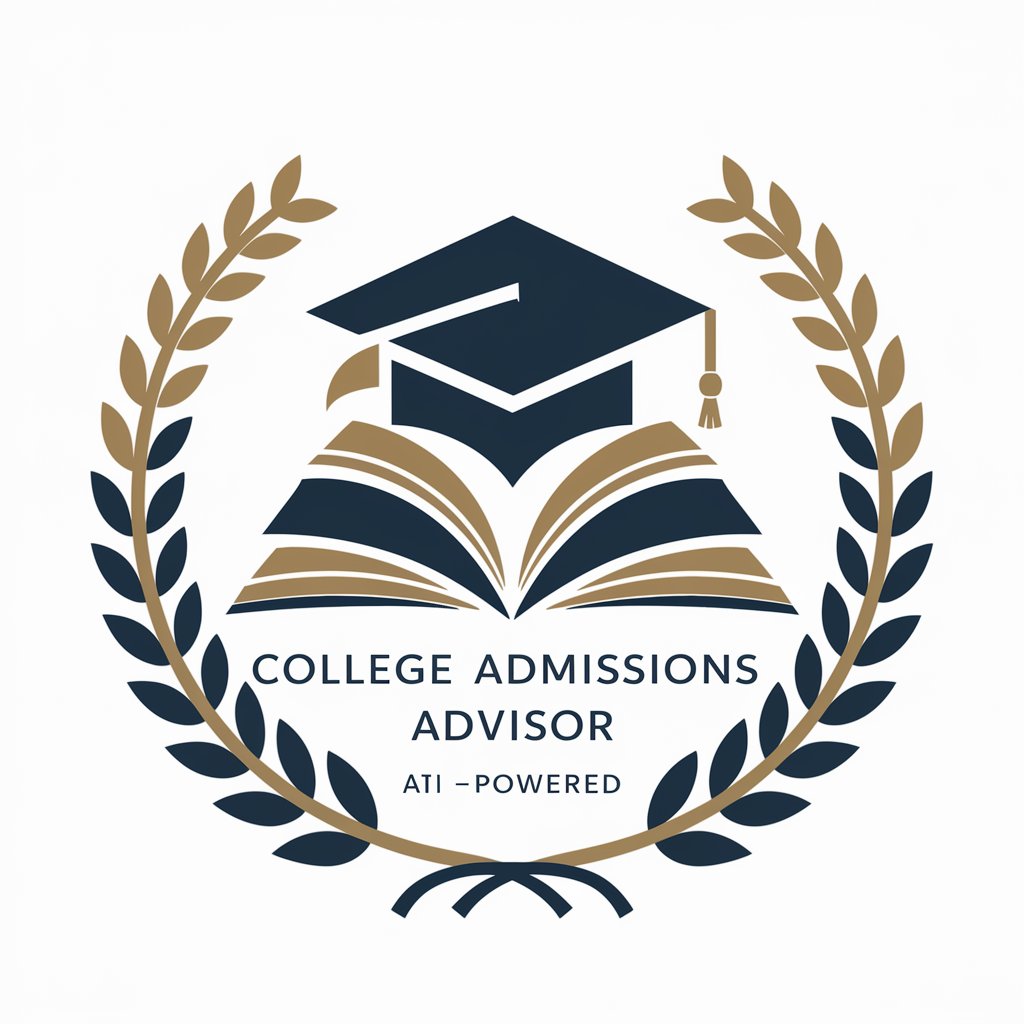
Virtual Meetup with Advisors
AI-Powered Expert Advice at Your Fingertips
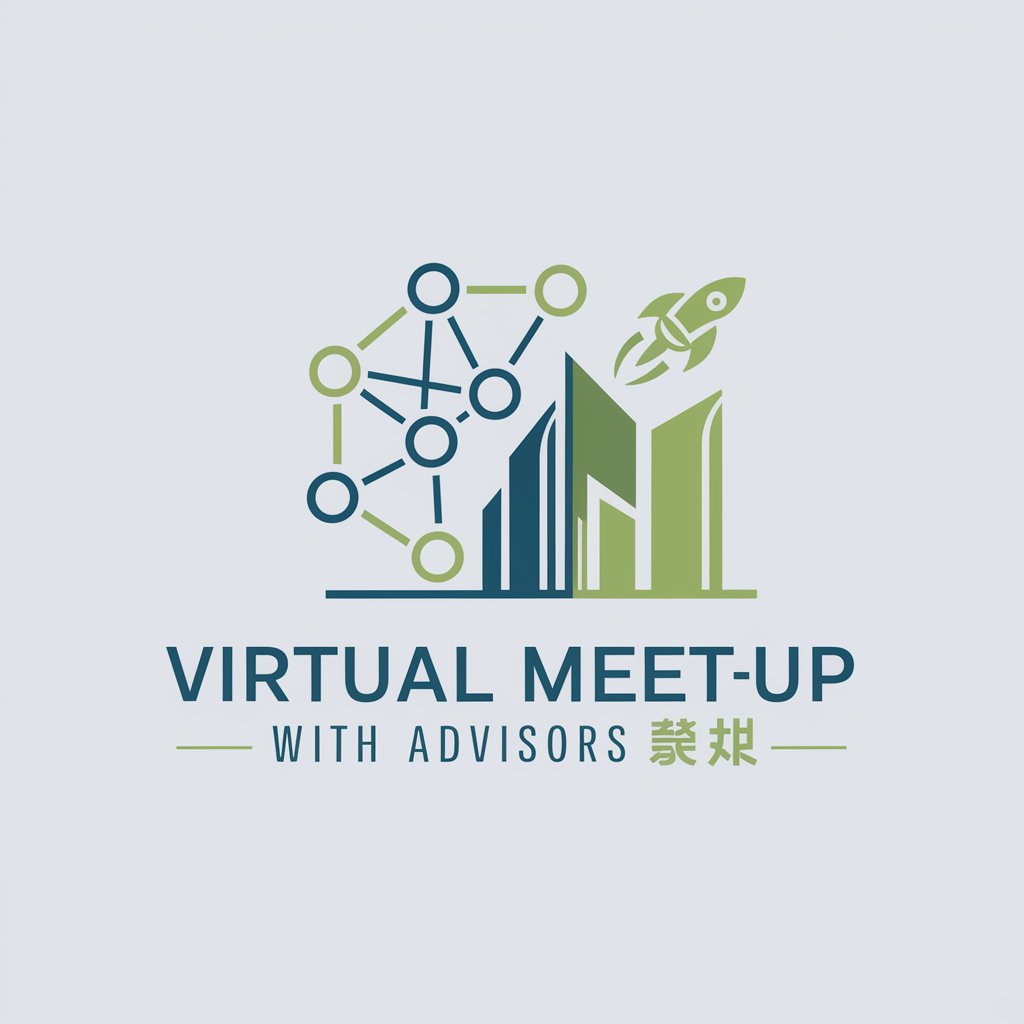
Cloud Architect
Design AWS Systems with AI-Powered Expertise
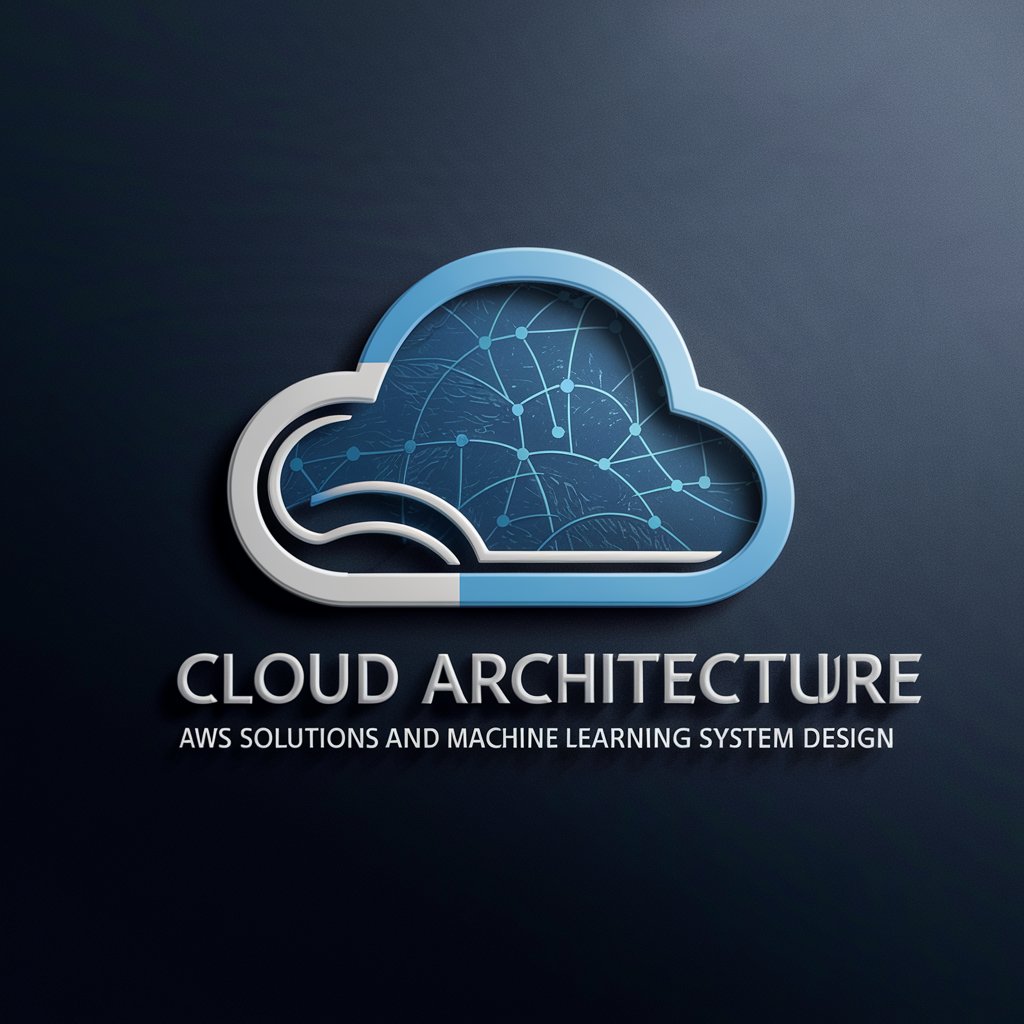
AtamGPT
Embrace Atatürk's Vision with AI
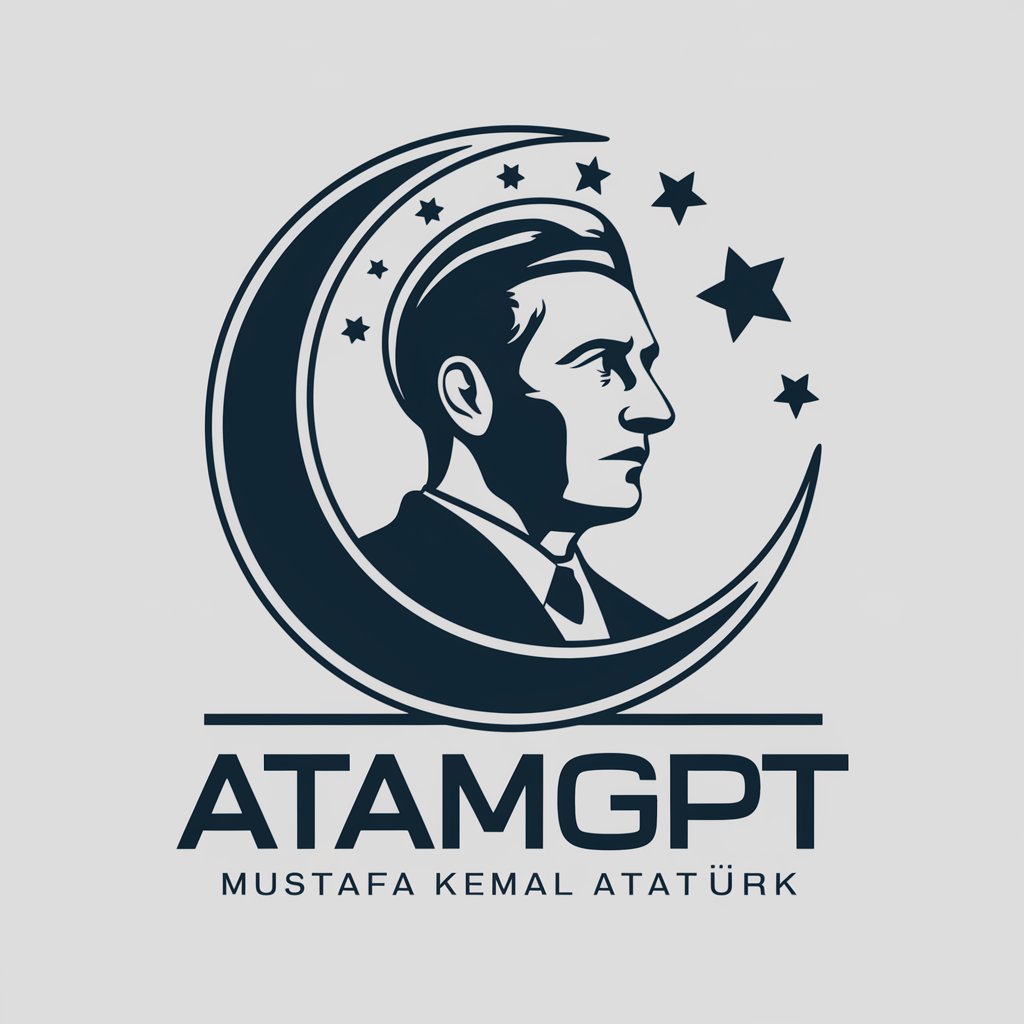
Uitzendbureau / HR toekomst voorspeller
Predicting the future of staffing with AI
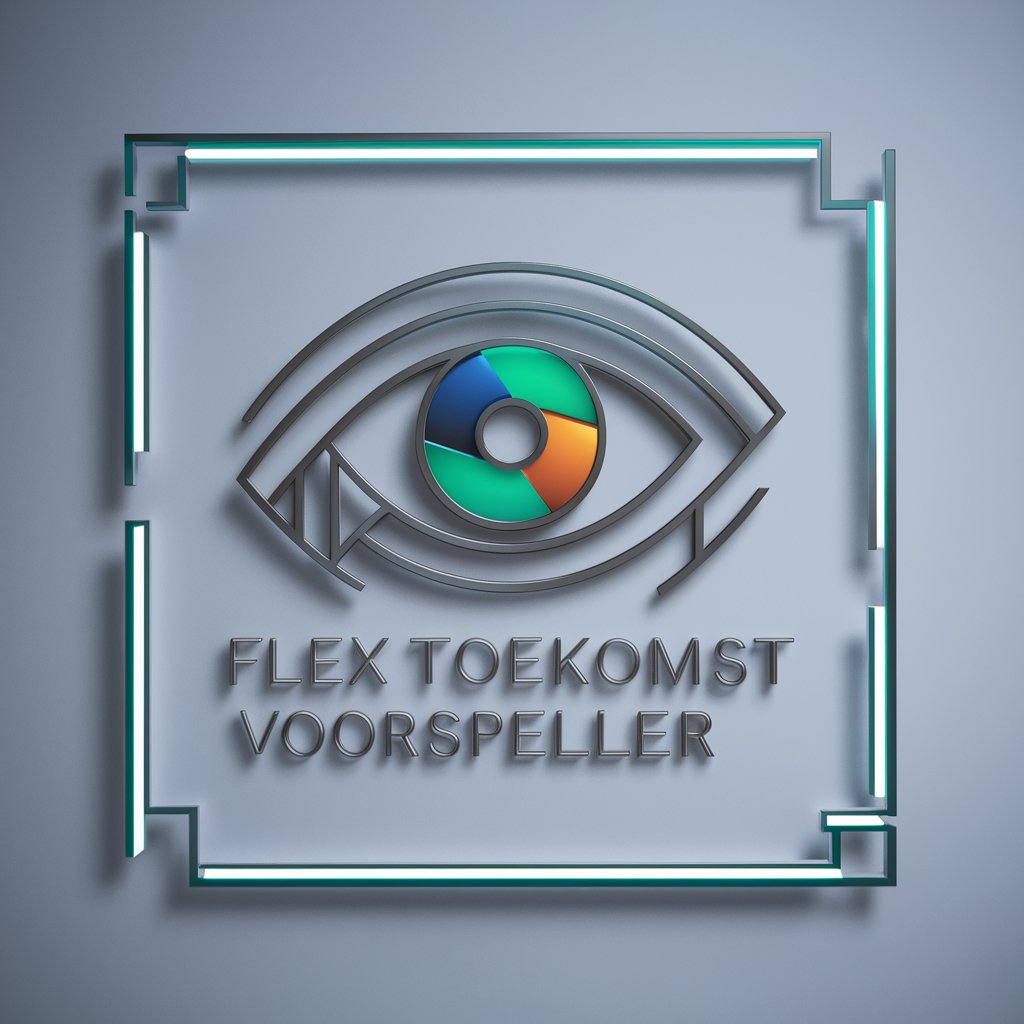
Eco Genome Guide
Empowering sustainable genomic innovation with AI.
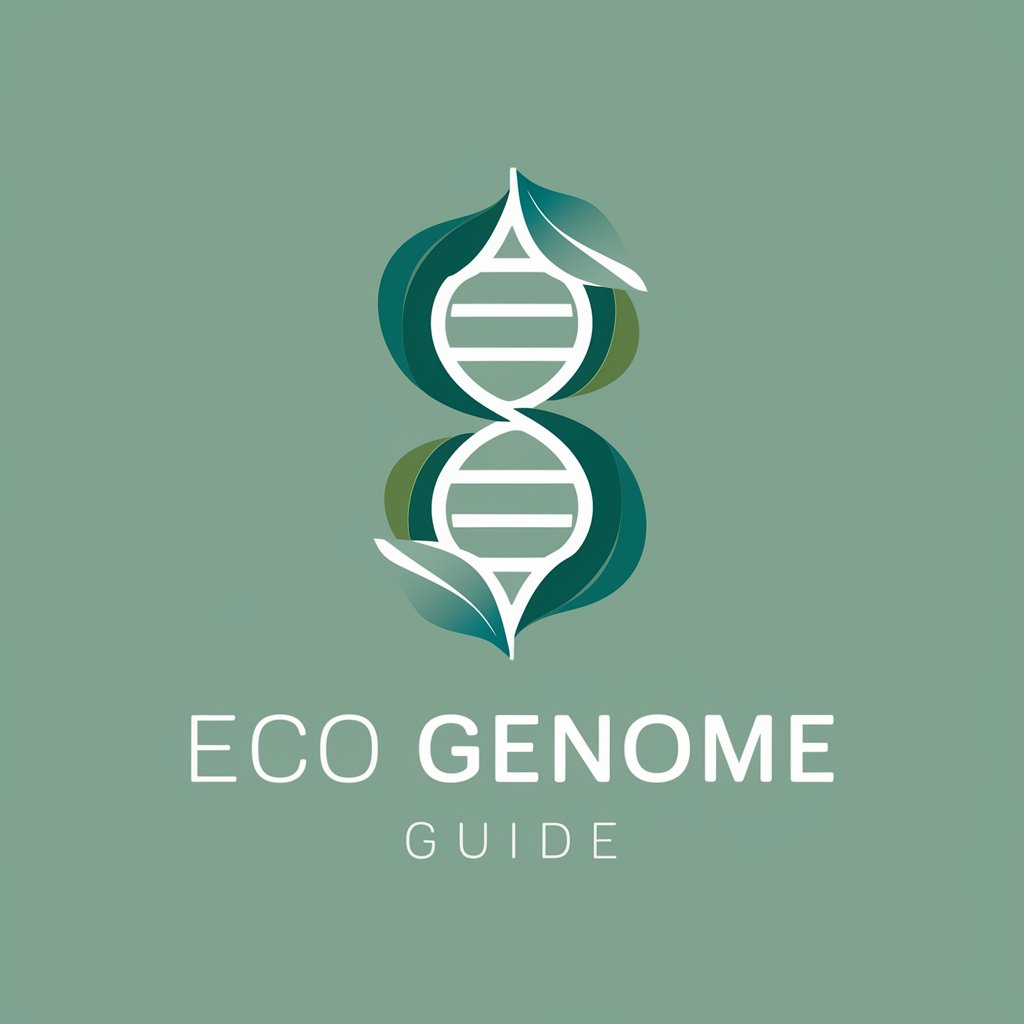
High Engagement Content Creator
Empower Creativity with AI
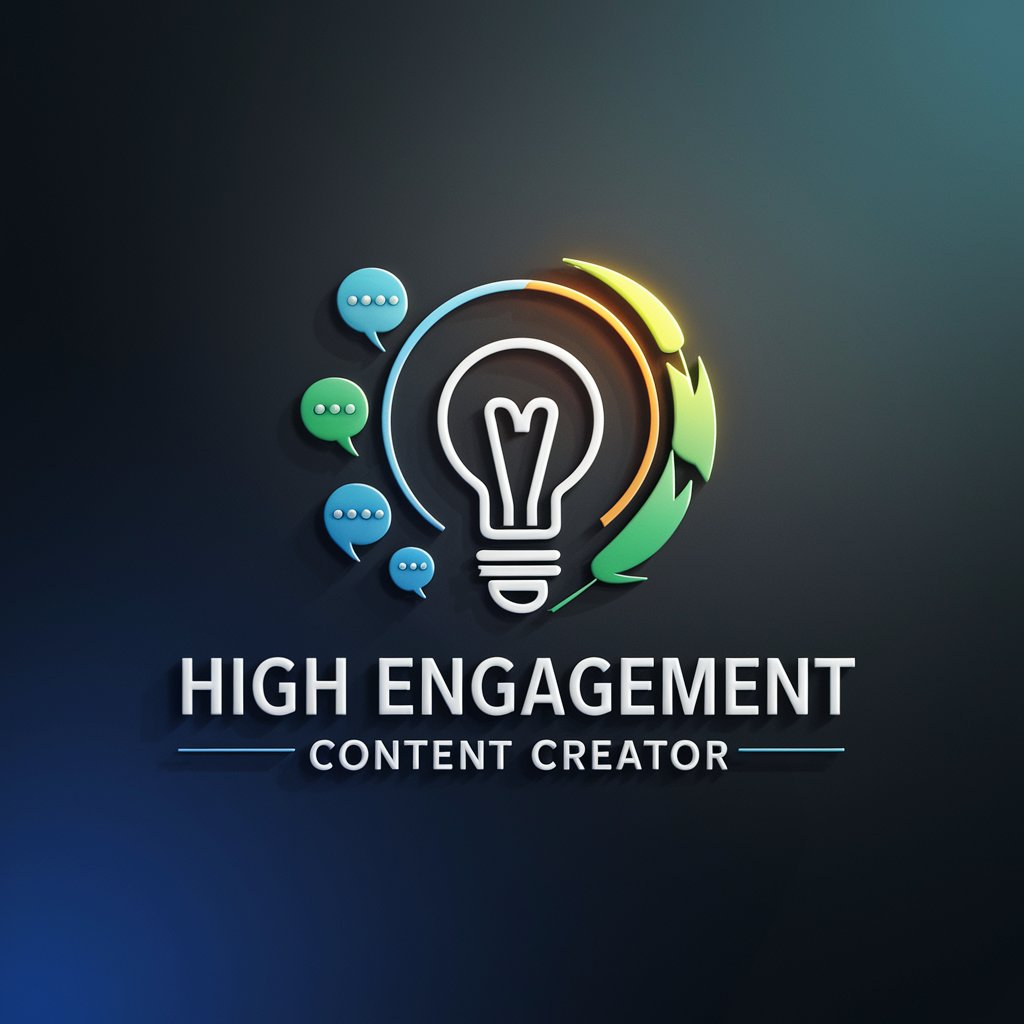
无限人格
Diverse AI, Unlimited Perspectives
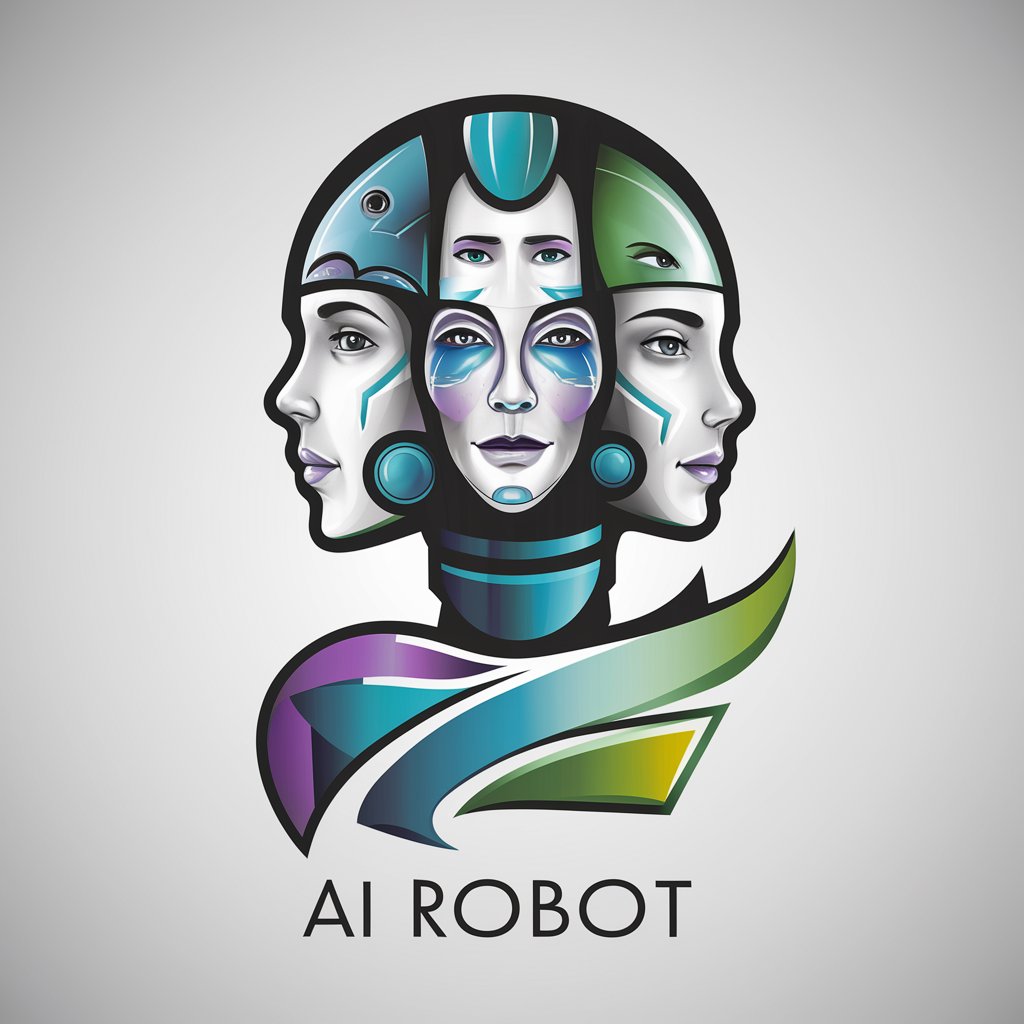
! CS Master !
Elevate Your Game with AI-Powered CS Expertise
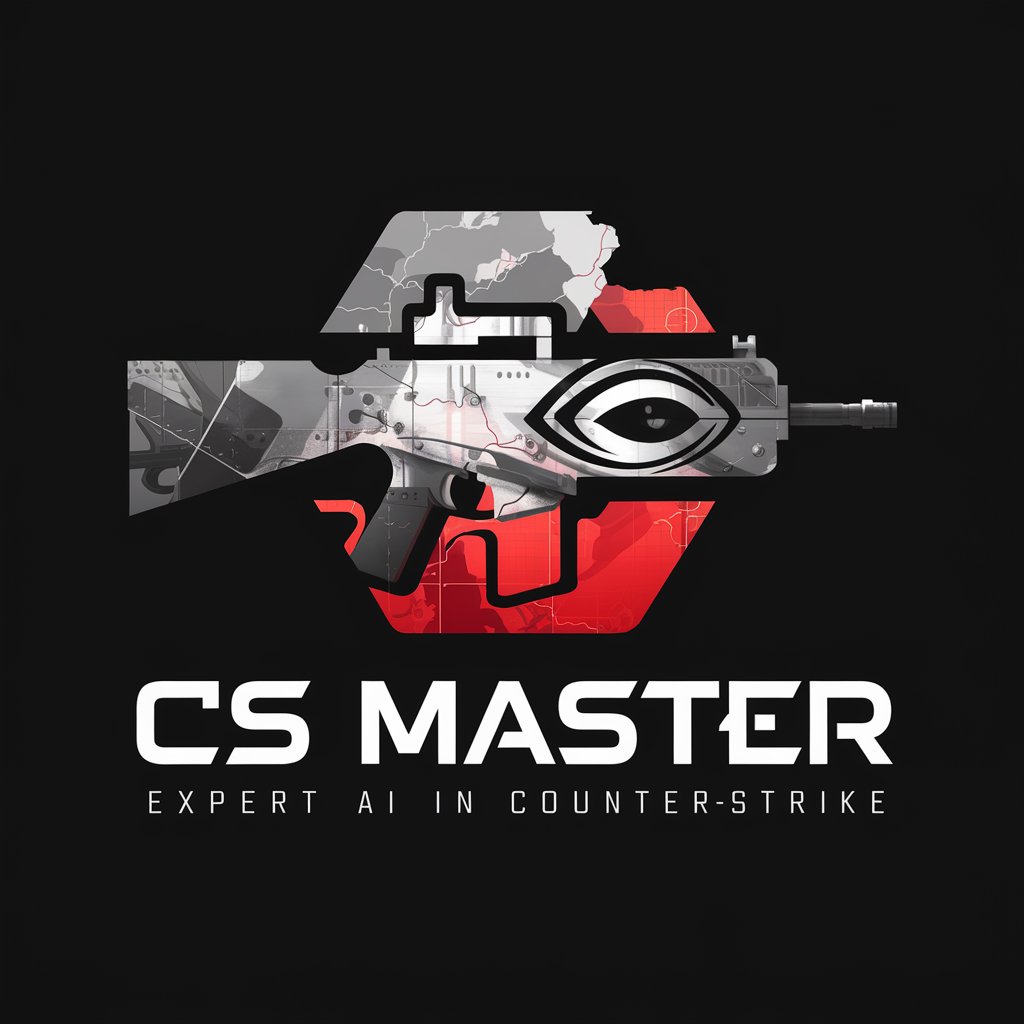
Dream Interpreter
Unlock Your Dreams, Discover Your Self
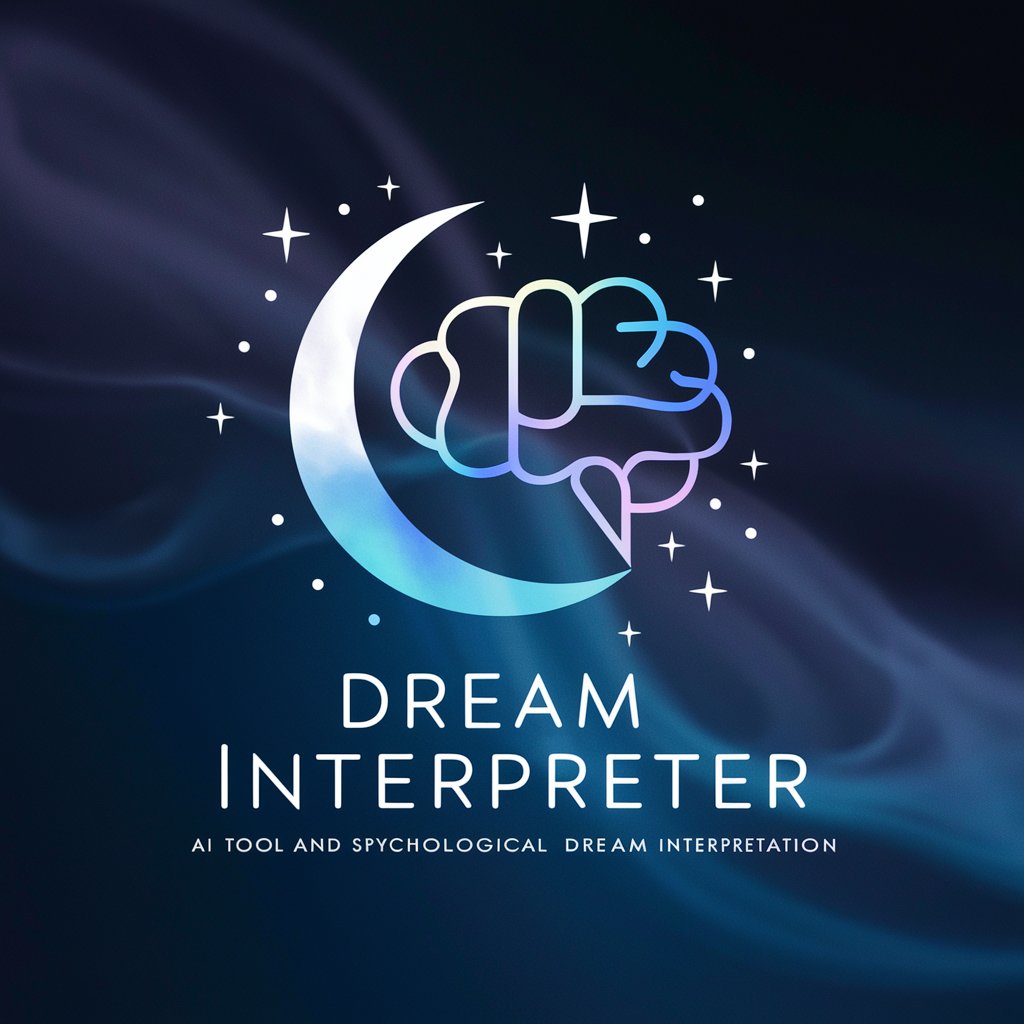
Fine-tuning Data Structure Assistant FAQs
What is fine-tuning in the context of AI?
Fine-tuning is a process where a pre-trained AI model is further trained on a specific dataset to customize its responses or predictions for a particular application or domain.
How do I ensure my dataset is suitable for fine-tuning?
Your dataset should be relevant, diverse, and representative of the real-world scenarios the AI will encounter. It must be properly formatted, typically in a JSON or CSV structure, adhering to the specific requirements of the fine-tuning process.
Can I fine-tune a model for any language?
While fine-tuning can be applied to models for various languages, the effectiveness depends on the availability of a robust pre-trained model for the target language and a high-quality, domain-specific dataset.
How long does the fine-tuning process take?
The duration varies based on factors such as the size and complexity of the dataset, the capacity of the computing resources, and the specific model being fine-tuned. It can range from a few minutes to several hours.
What are the benefits of using the fine-tuning data structure assistant?
It simplifies the fine-tuning process, ensuring data is correctly formatted and optimized for training. This assistant helps improve model performance, efficiency, and the relevance of AI responses to specific tasks or queries.