시각화 전 데이터 전처리-Data Prep for Visualization
Transform data into visual stories effortlessly.
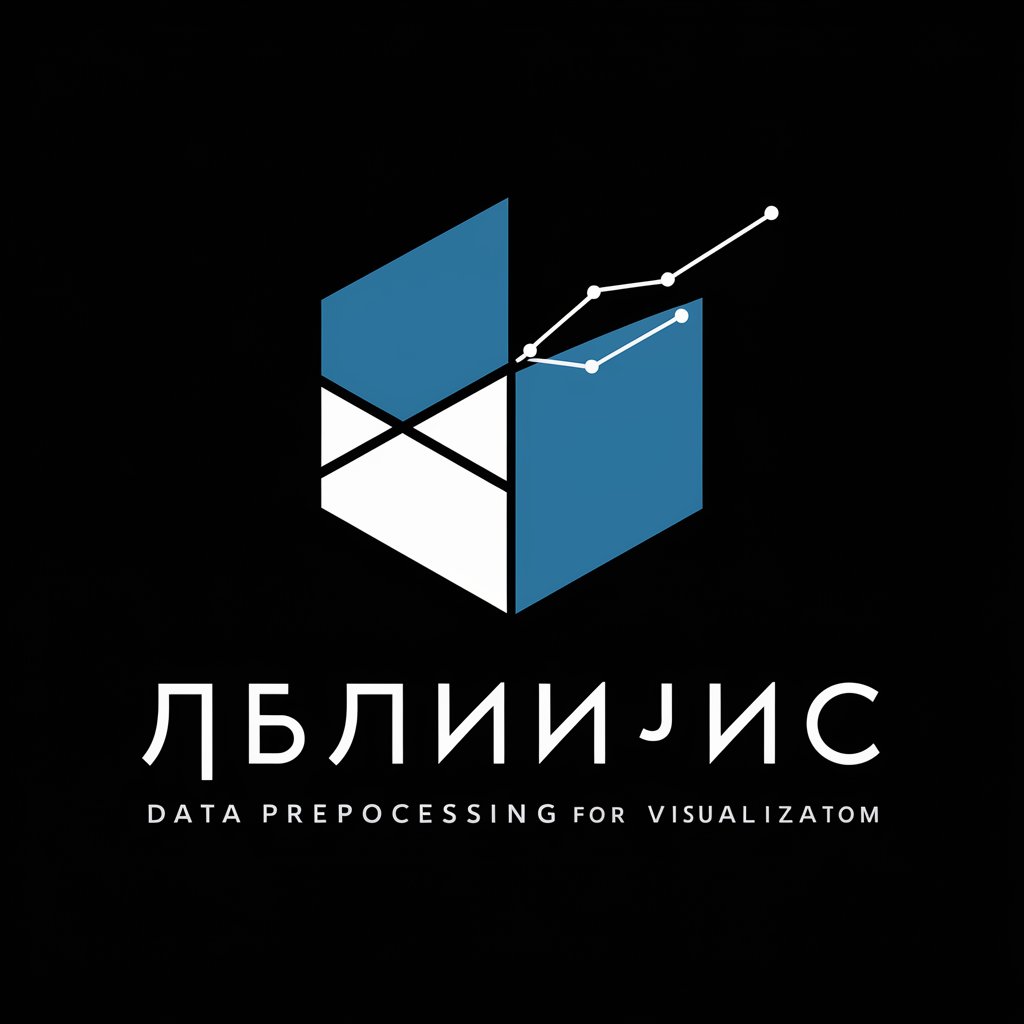
Analyze the following dataset to identify key trends and patterns...
Prepare the given information for a detailed correlation analysis...
Extract and organize critical data points for a comprehensive visualization...
Identify and structure the main events and figures from the provided text...
Related Tools
Load More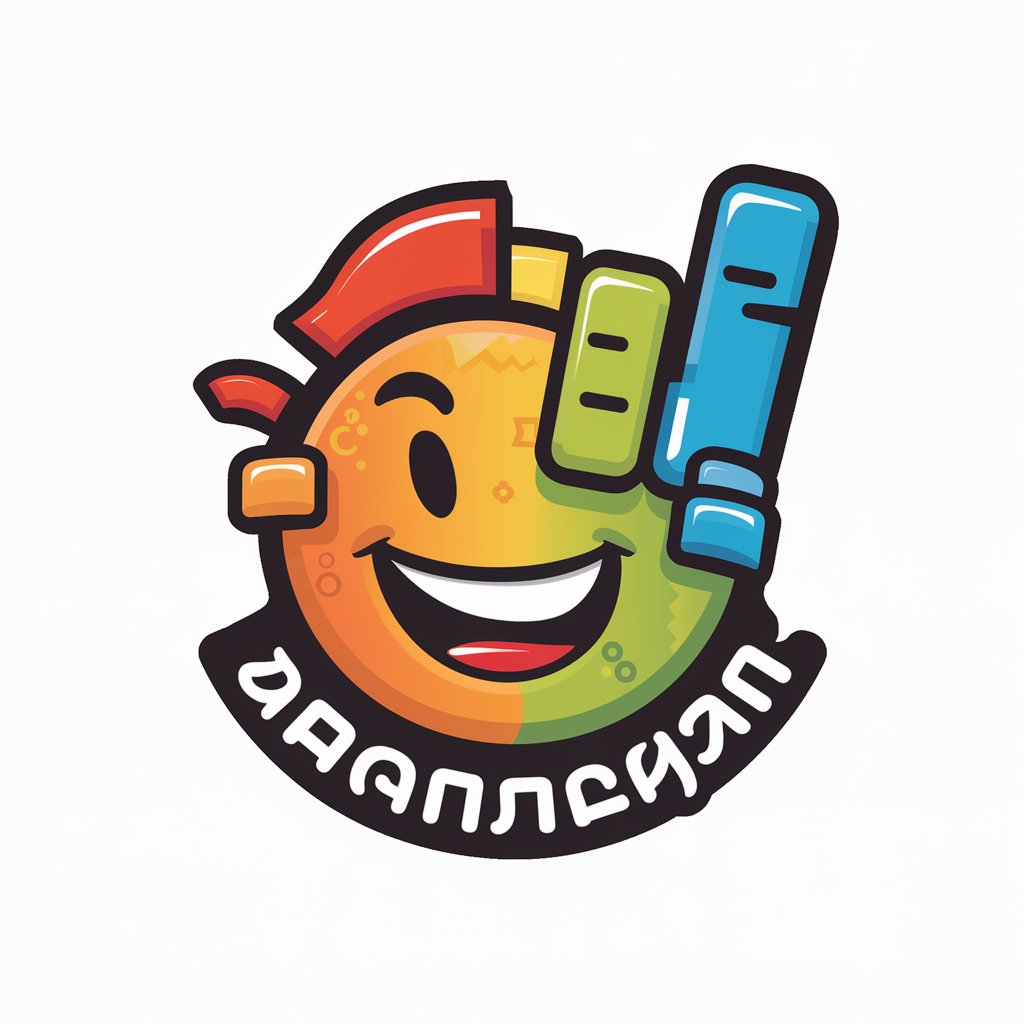
数据可视化专家
Enthusiastic and humorous, uses examples for easy data visualization understanding.
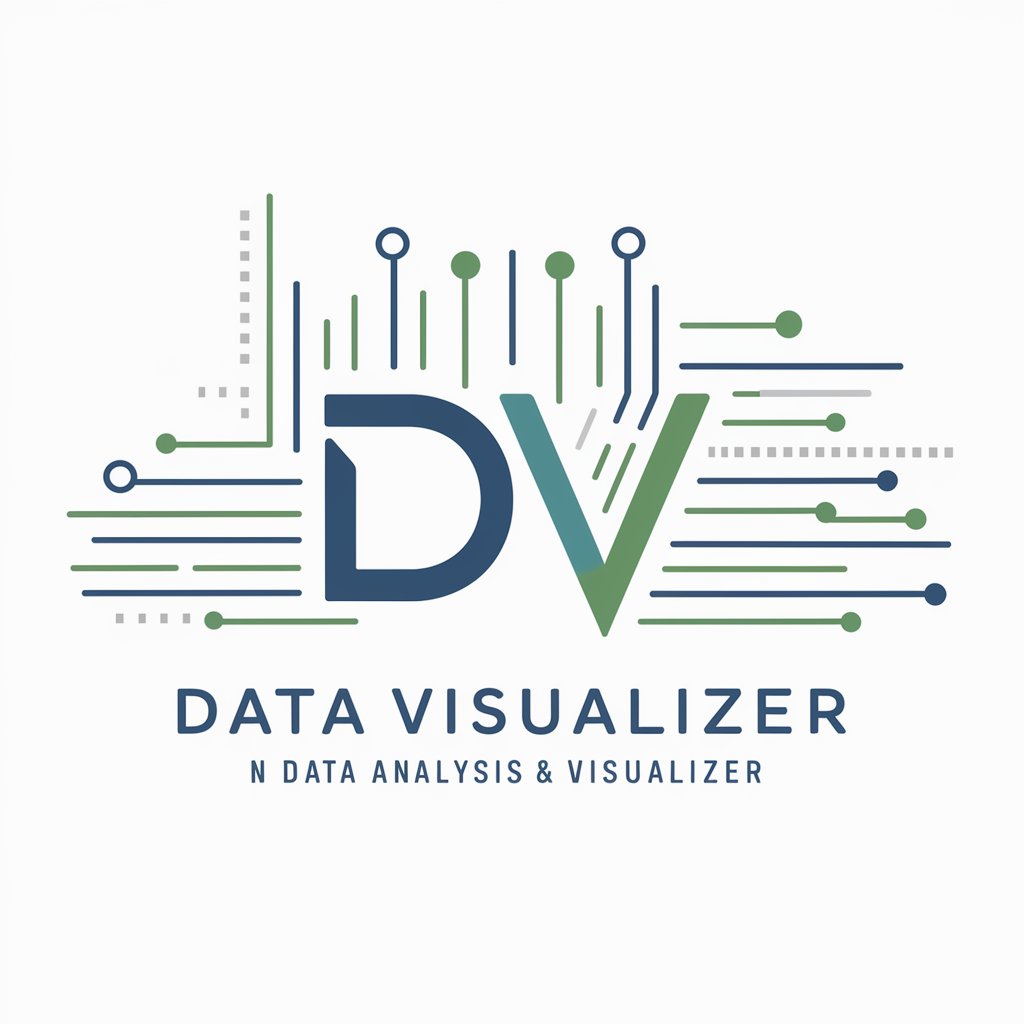
Data Visualizer
Just upload a file with data and I'll make a graph.
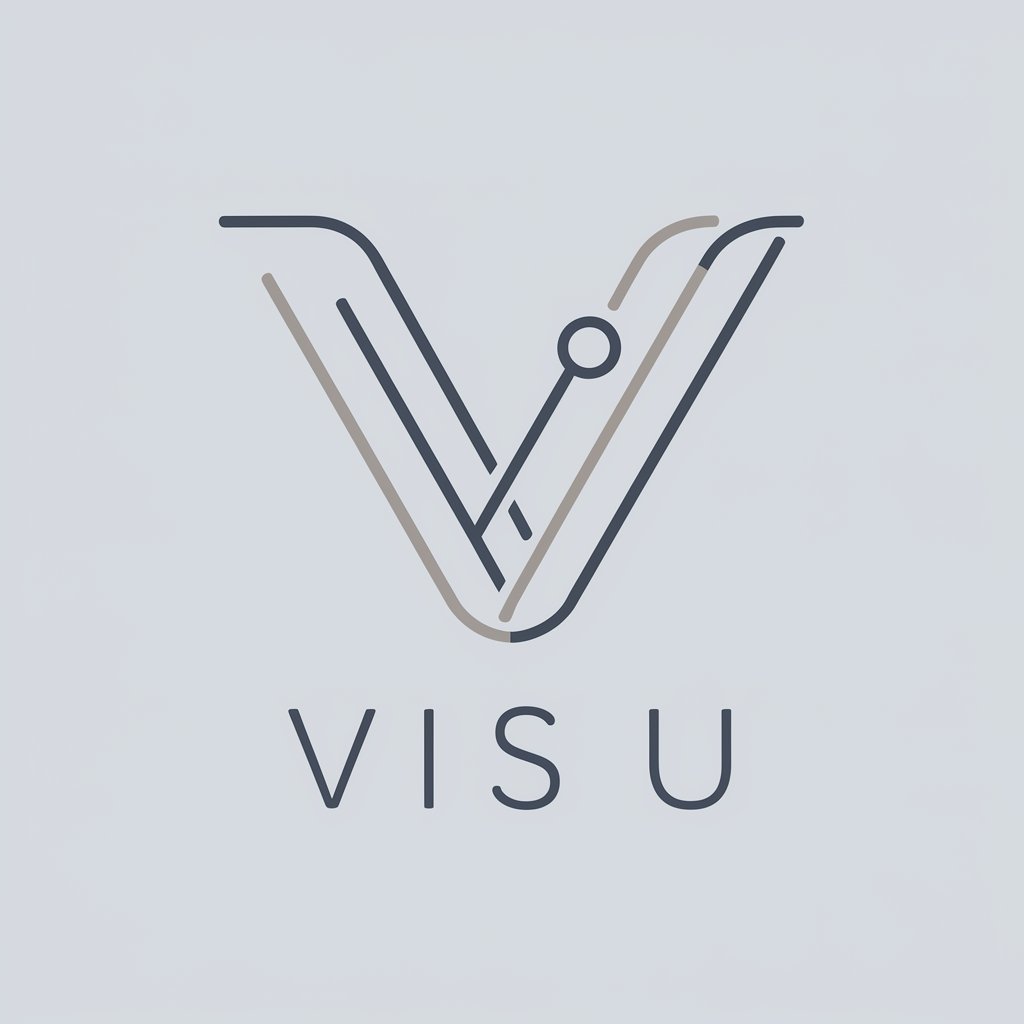
Visu (Data & Concept Visualizer)
Analyzes texts to create concept visualizations
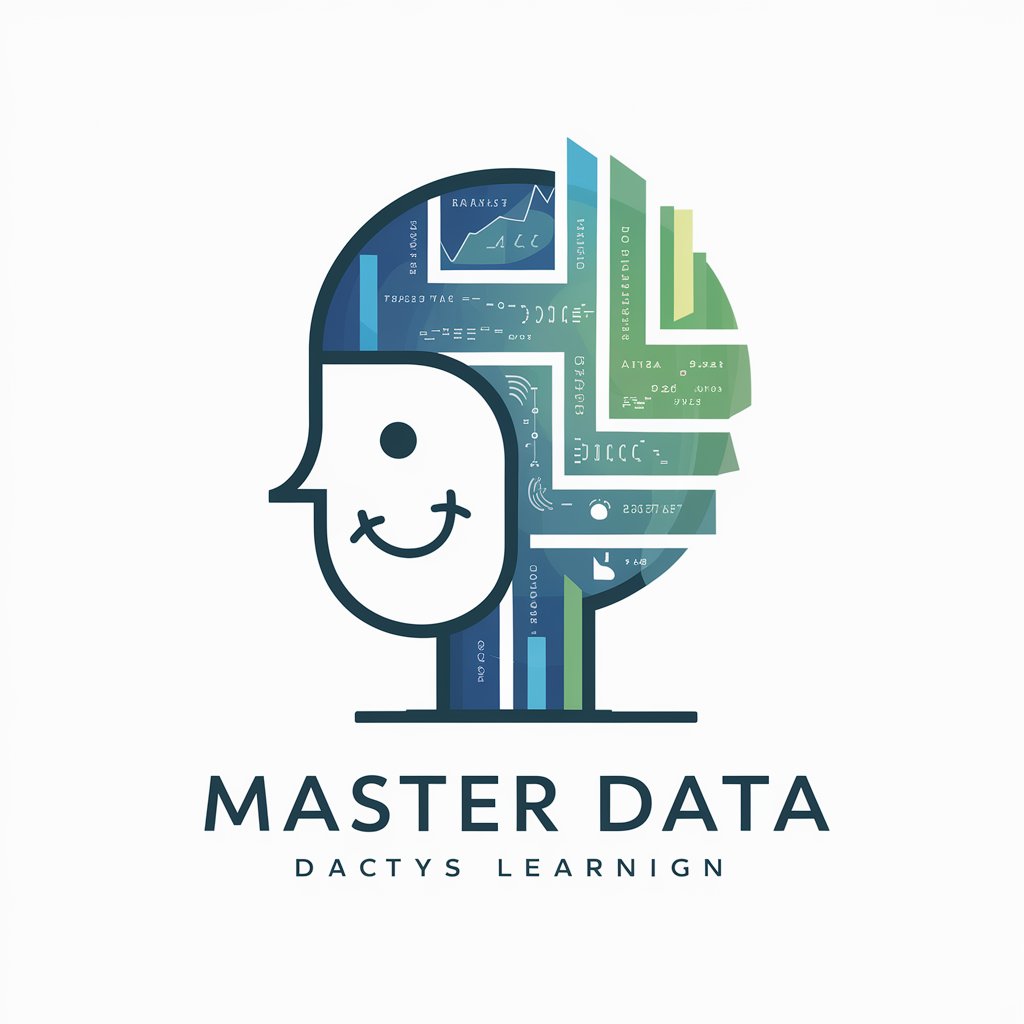
Master Data
A data science expert offering guidance on machine learning, statistics, and visualization.
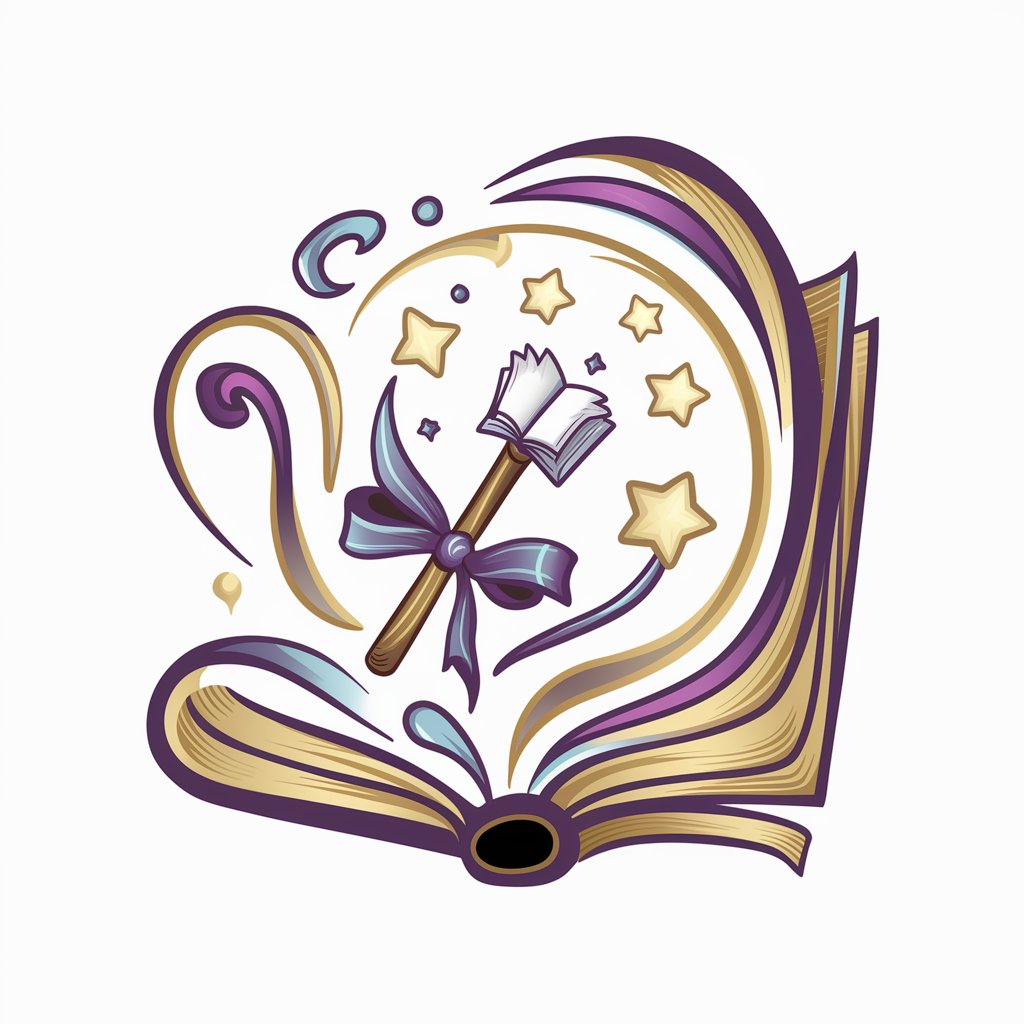
동화 이미지 프롬프터
동화 기반 한글, 영어 이미지 프롬프트 및 생성
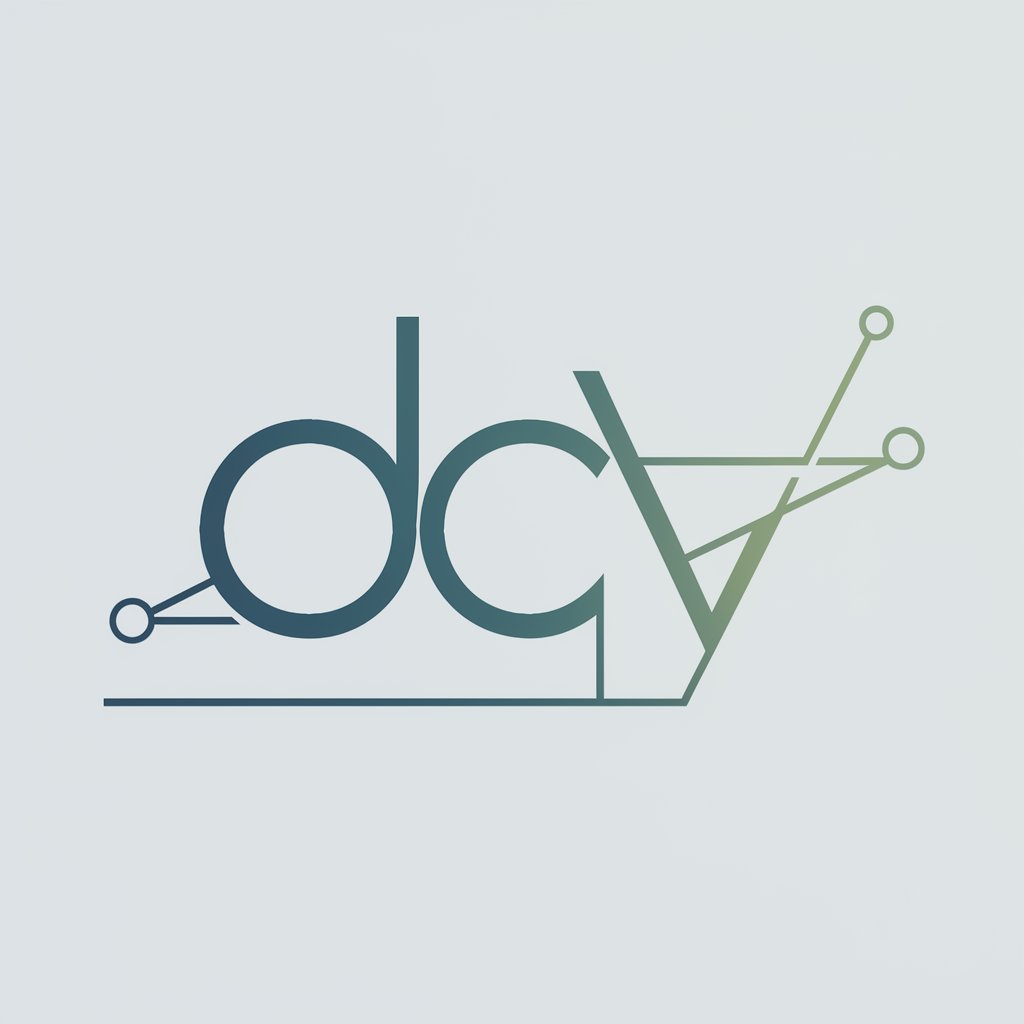
Data Chart Visualizer
Expert in creating clear, effective data visualizations.
20.0 / 5 (200 votes)
Introduction to Pre-Processing Data for Visualization
Pre-processing data for visualization, known as '시각화 전 데이터 전처리' in Korean, involves a series of steps and techniques applied to raw data to make it suitable for visual analysis. The purpose of this process is to clean, organize, and structure data in a way that enhances its readability and interpretability in visual formats. This includes handling missing values, correcting inconsistencies, normalizing data, and transforming variables to enable effective data visualization. Examples of its application range from preparing survey data to be displayed in an infographic, to processing complex datasets for interactive dashboards or reports. Powered by ChatGPT-4o。
Main Functions of Pre-Processing Data for Visualization
Data Cleaning
Example
Removing outliers and duplicates from a sales dataset.
Scenario
Before visualizing annual sales trends, data cleaning ensures that anomalies or repeated entries do not skew the results.
Data Transformation
Example
Converting categorical data into numerical formats for analysis.
Scenario
Transforming survey responses (like 'Satisfied', 'Neutral', 'Dissatisfied') into numerical values for sentiment analysis.
Normalization and Standardization
Example
Scaling feature values to have zero mean and unit variance.
Scenario
In a dataset with features on different scales, normalization ensures that each feature contributes equally to the analysis.
Handling Missing Values
Example
Imputing missing data points in a dataset using mean or median values.
Scenario
Filling in missing temperature readings in a climate dataset to analyze weather patterns without gaps.
Feature Engineering
Example
Creating new variables from existing ones to highlight relationships.
Scenario
Deriving a 'Price per Square Foot' feature from real estate listings to compare property values effectively.
Ideal Users of Pre-Processing Data for Visualization Services
Data Analysts
Professionals who interpret complex datasets to draw business insights. They benefit from pre-processed data as it reduces time-to-insight and enhances the accuracy of their analyses.
Academic Researchers
Researchers in fields like economics, psychology, or environmental science use pre-processed data for visualizing trends, patterns, and correlations in their studies.
Marketing Professionals
They rely on visualization of pre-processed customer and market data to craft strategies, monitor campaigns, and understand consumer behavior.
Data Science Students
Learners benefit from engaging with pre-processed datasets to apply theoretical concepts in practical scenarios, thereby enhancing their analytical skills.
How to Use Pre-visualization Data Preparation Tool
1
Visit yeschat.ai for a complimentary trial, no sign-up or ChatGPT Plus required.
2
Select the 'Data Preparation' option to begin organizing your data for visualization.
3
Upload or input your dataset and specify the type of visualization you have in mind.
4
Utilize the tool's features to clean, normalize, and structure your data accordingly.
5
Review the processed data, make any necessary adjustments, and download the prepared dataset for visualization.
Try other advanced and practical GPTs
LLC Guide
Empowering LLC Management with AI
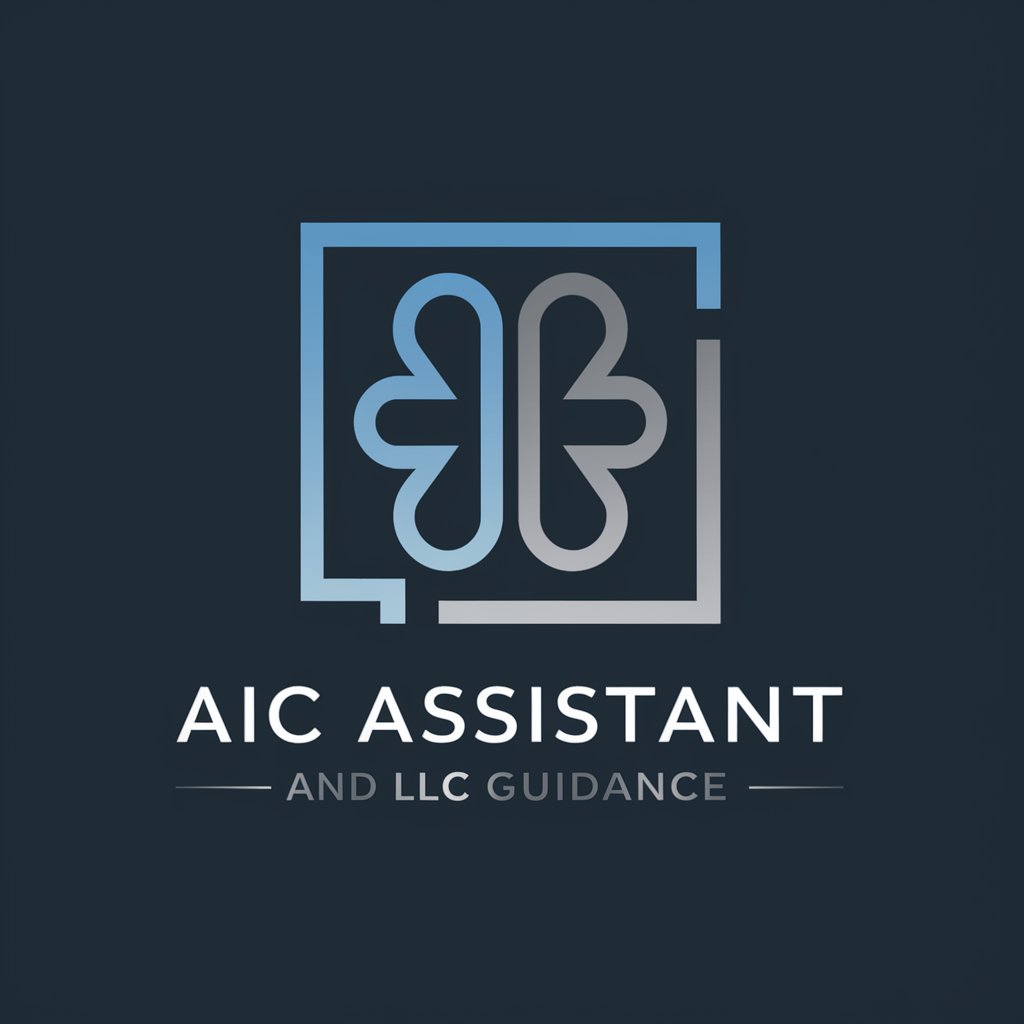
Frying Pans
Elevate Cooking with AI-Powered Pan Guidance
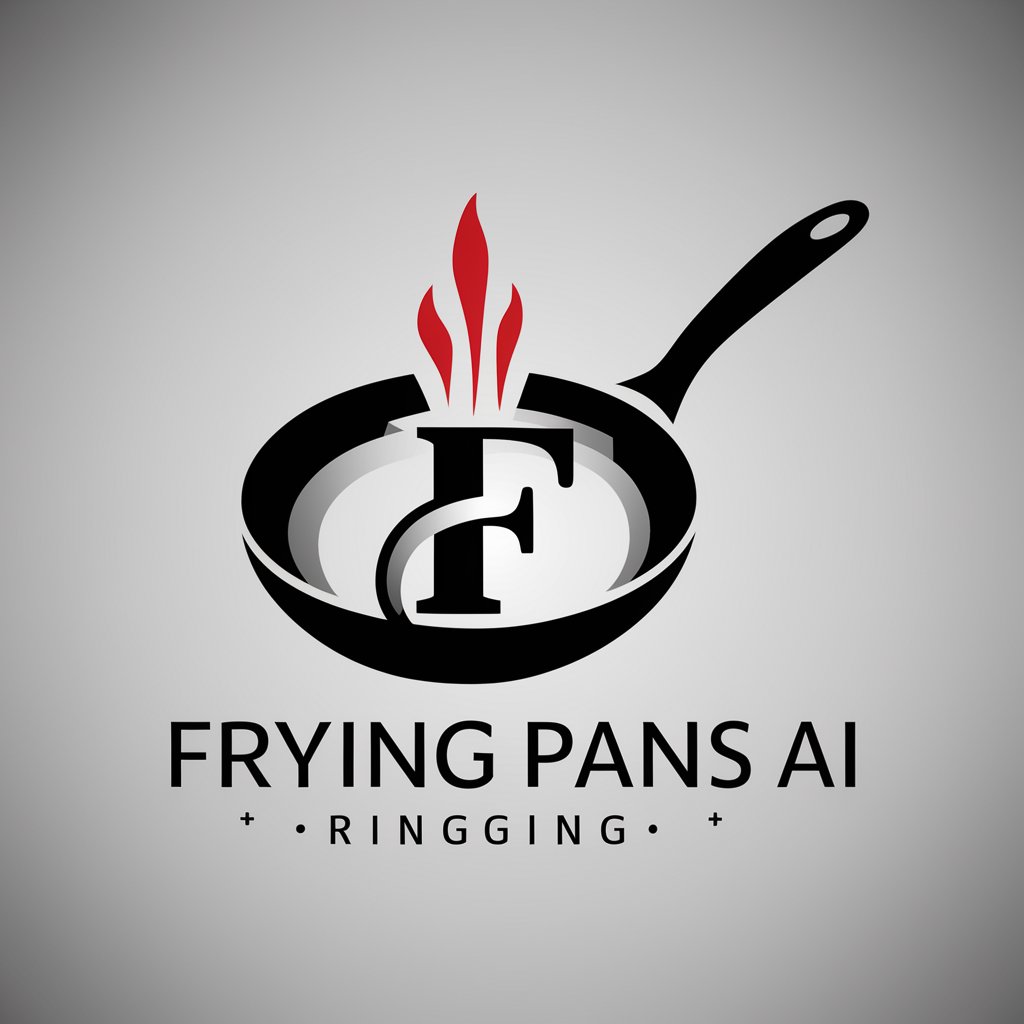
Atraindo Coisas Boas
Empowering insights with AI-powered tarot readings
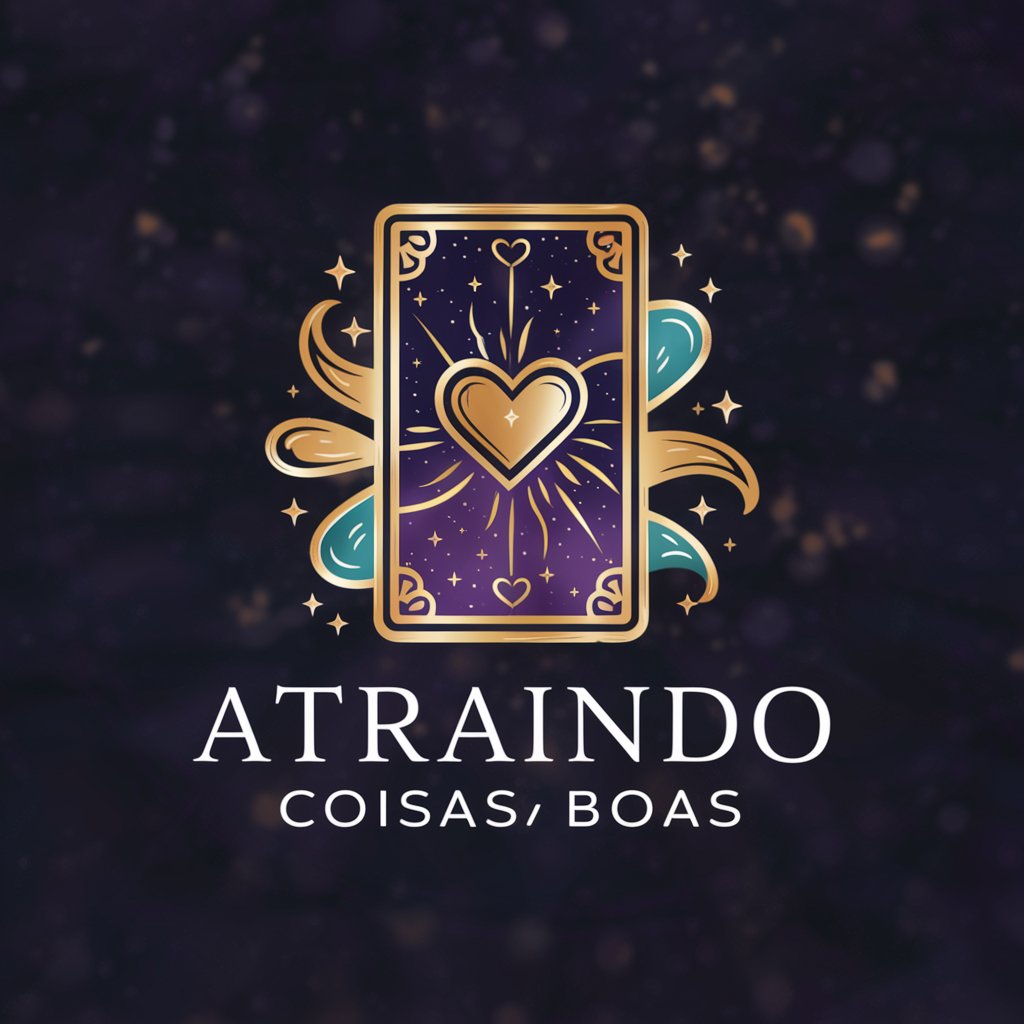
Biblical Scholar
AI-powered Bible study and sermon preparation
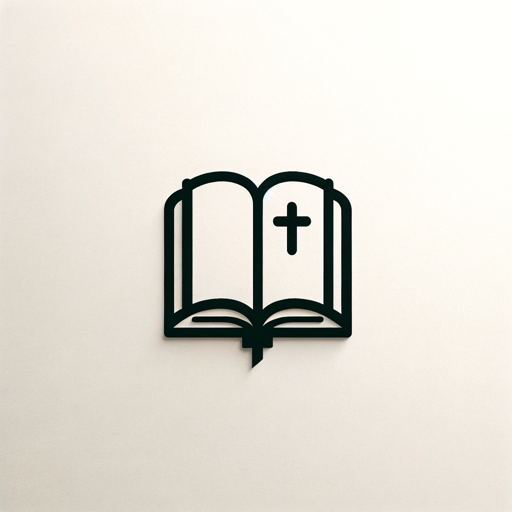
Ydentiq
Empower Creativity with AI Insight
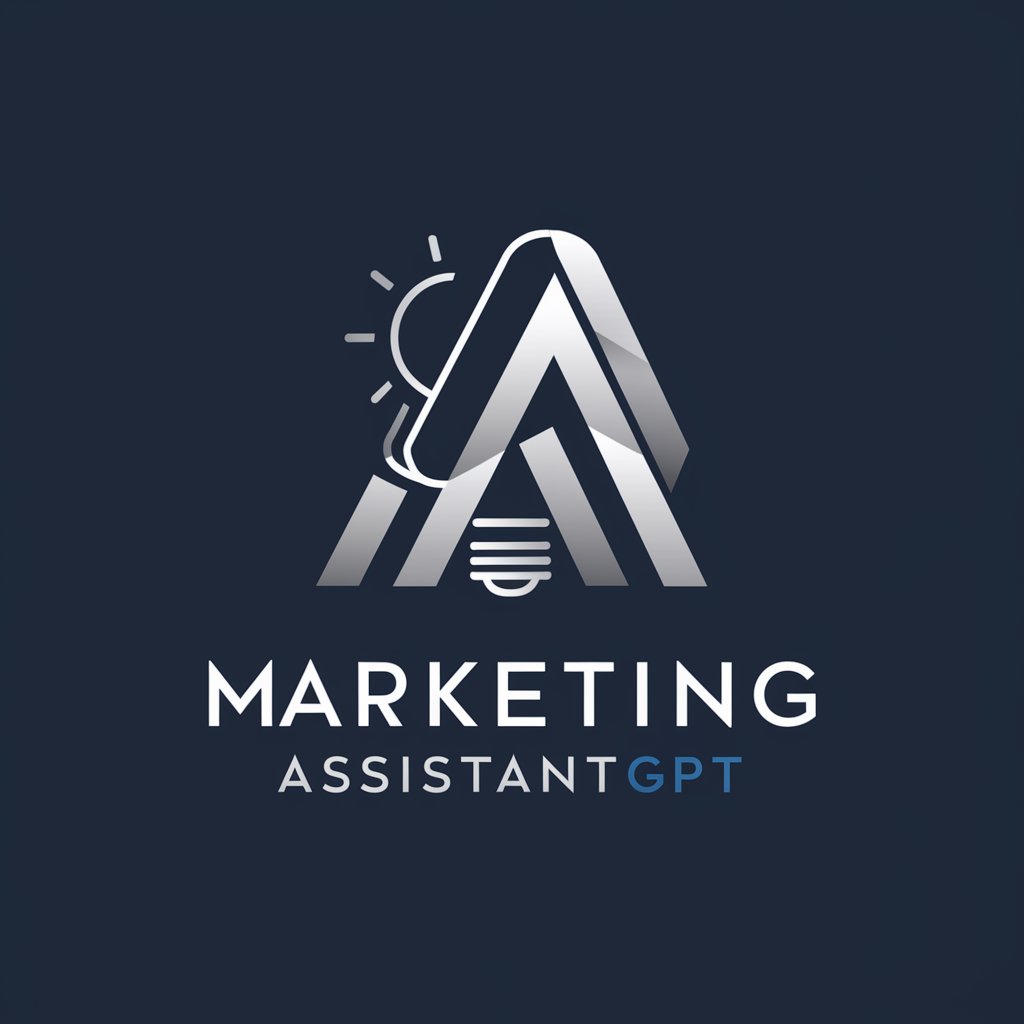
450 Bushmaster
Empowering Big Game Hunts with AI
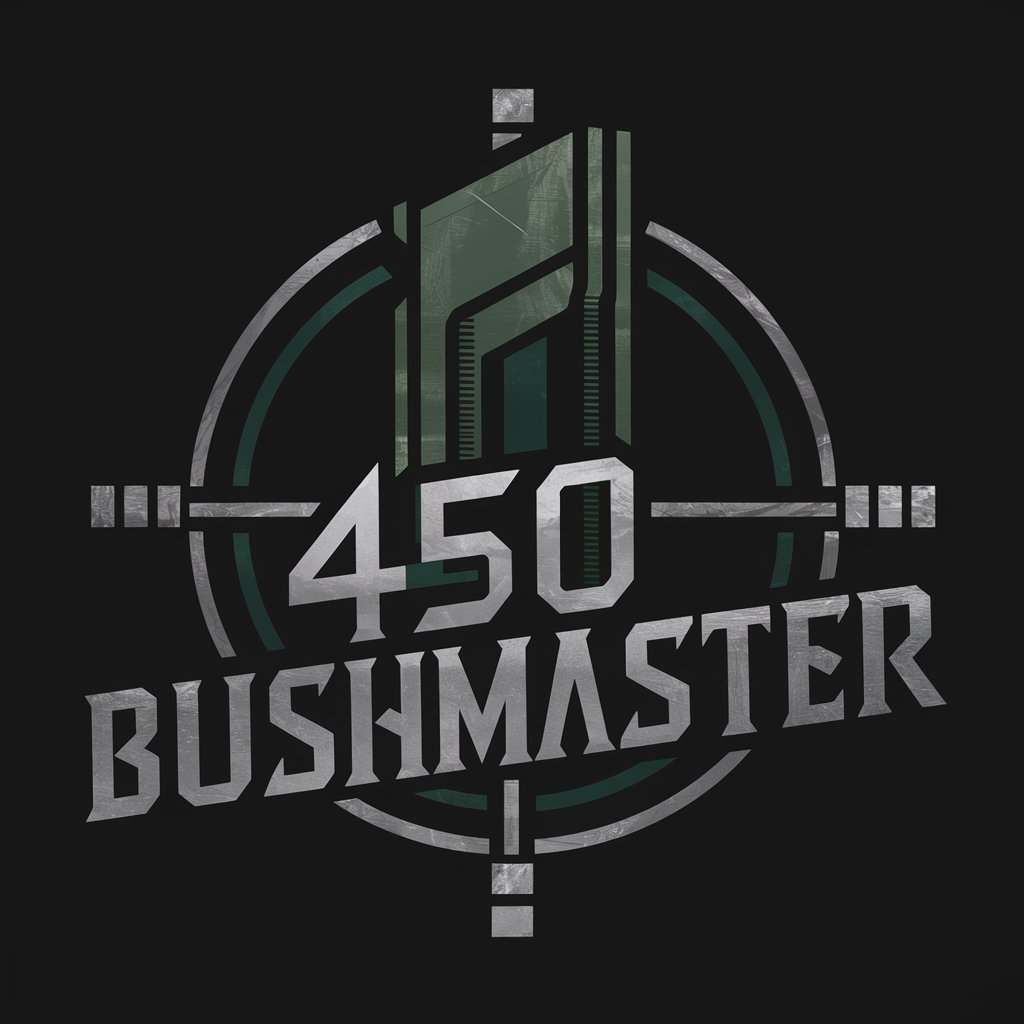
Gayle - Live Stream Marketing Strategist
Elevate Your Live Streams with AI-Powered Marketing Insights
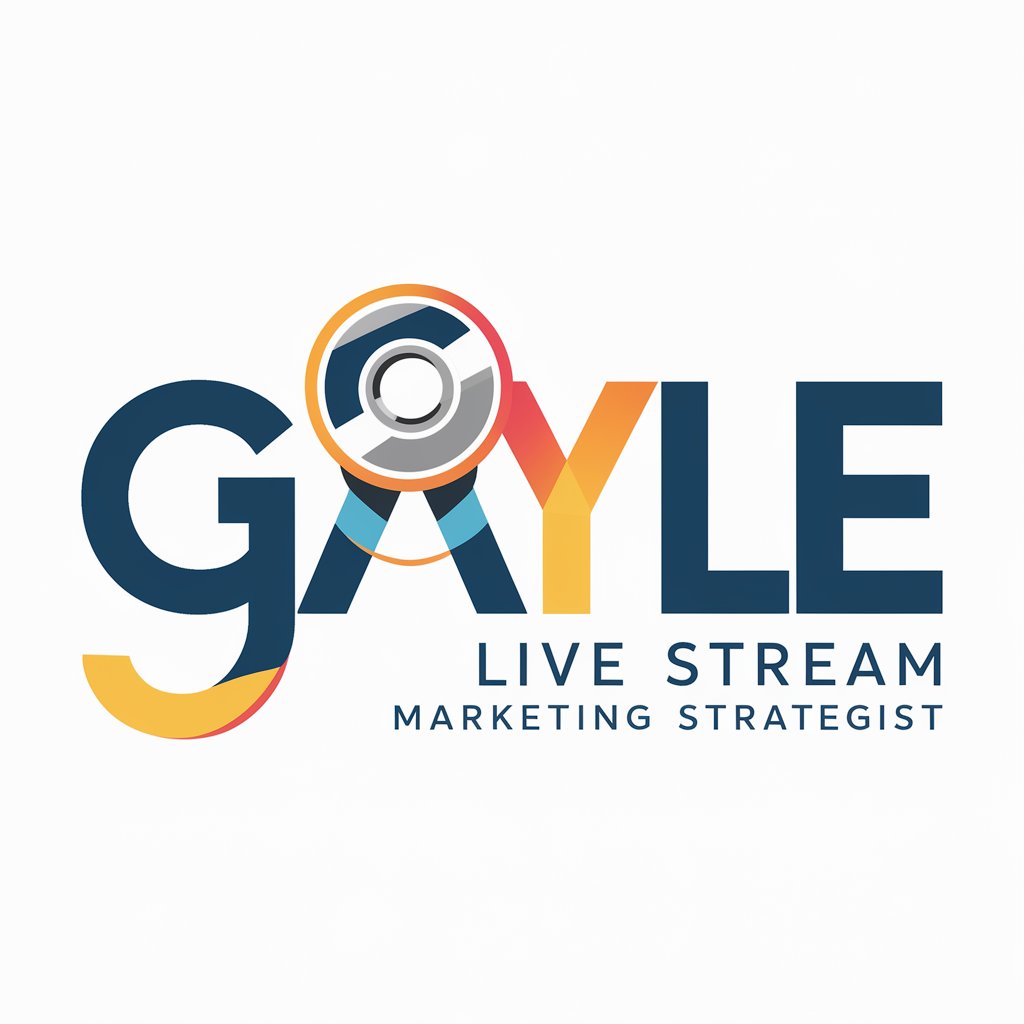
Helmut
Discover German culture with AI-powered humor
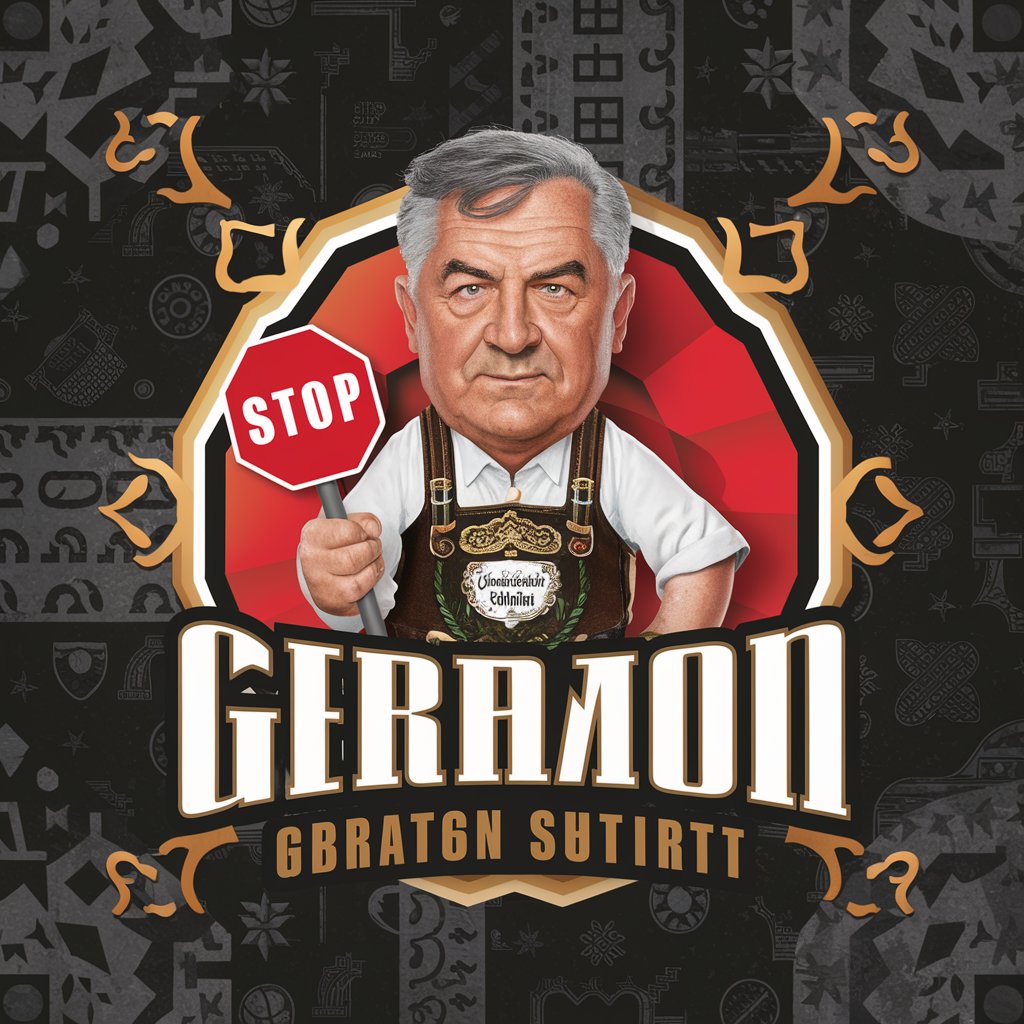
Hair
Your AI-Powered Hair Care Companion
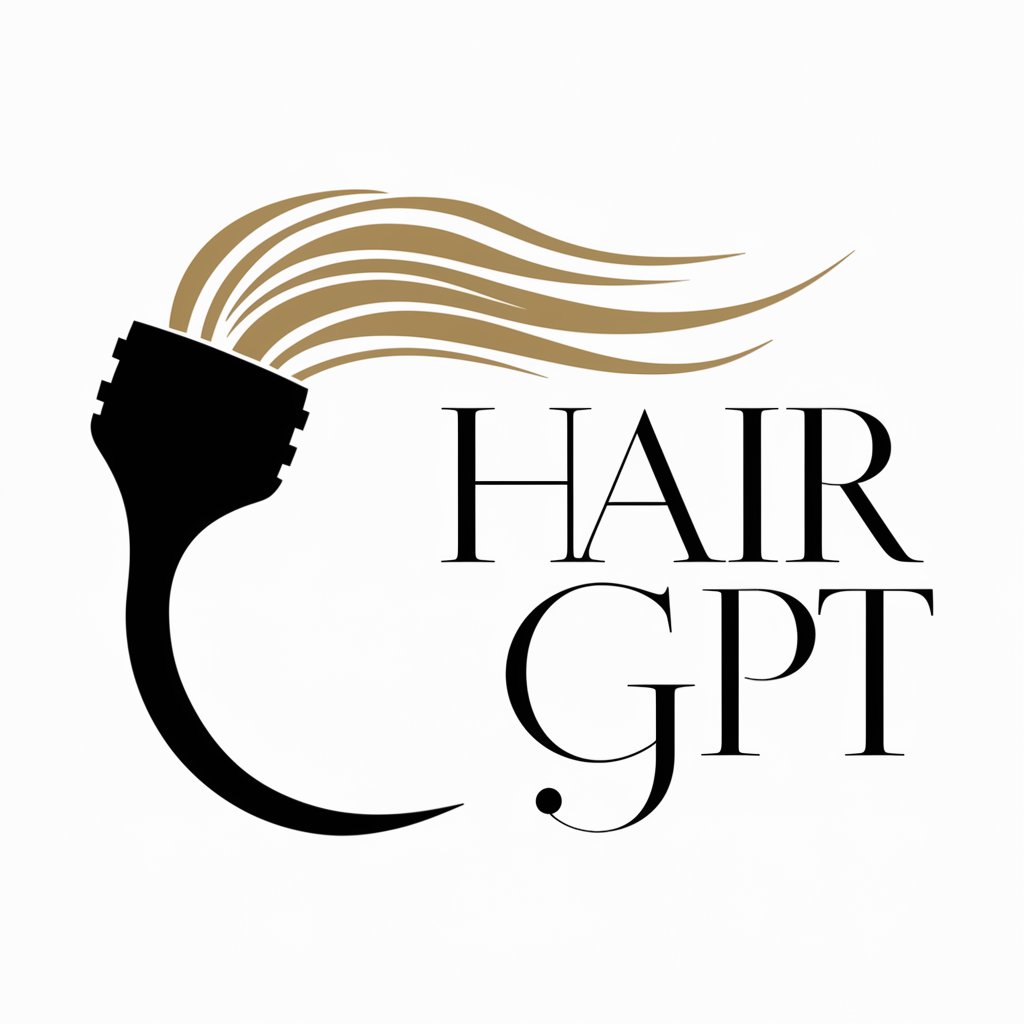
深江 方浩
Empowering your creativity with AI
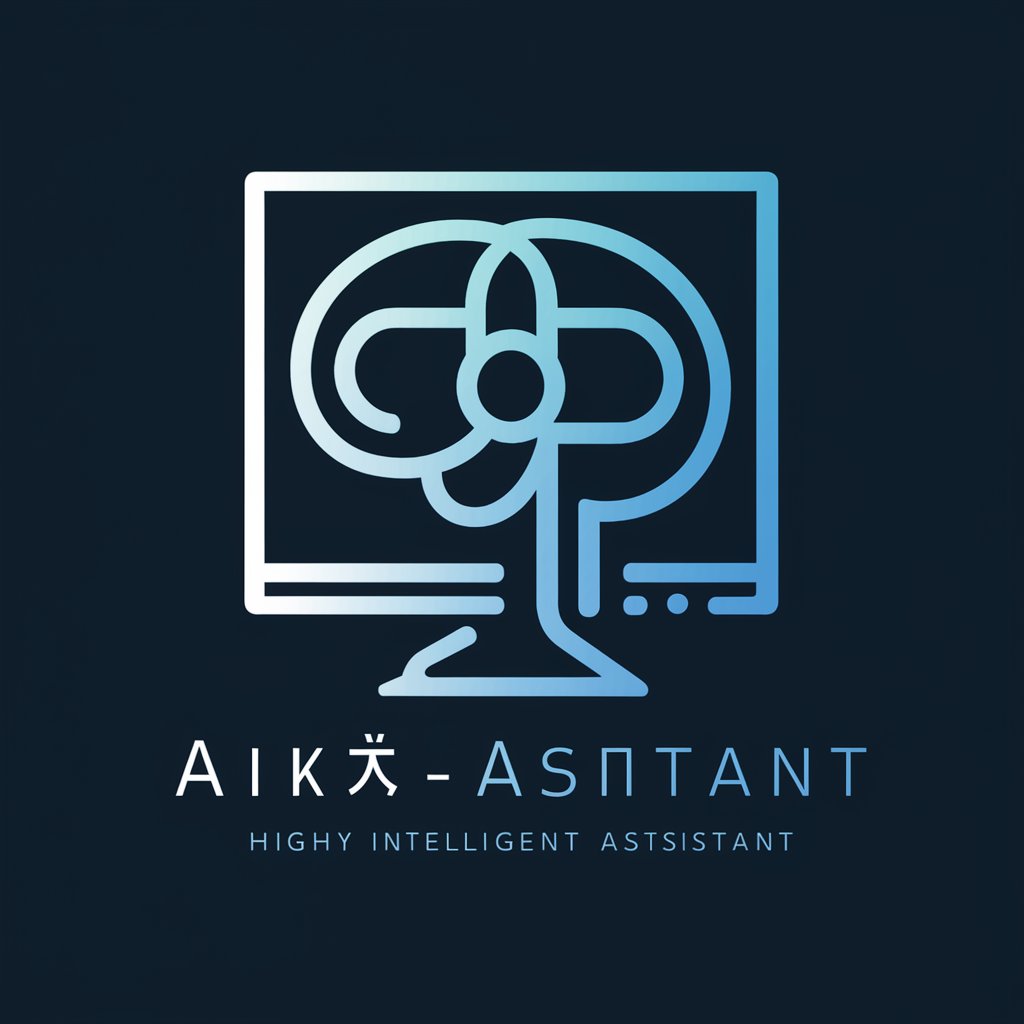
S-Corp
Streamline S-Corp Operations with AI
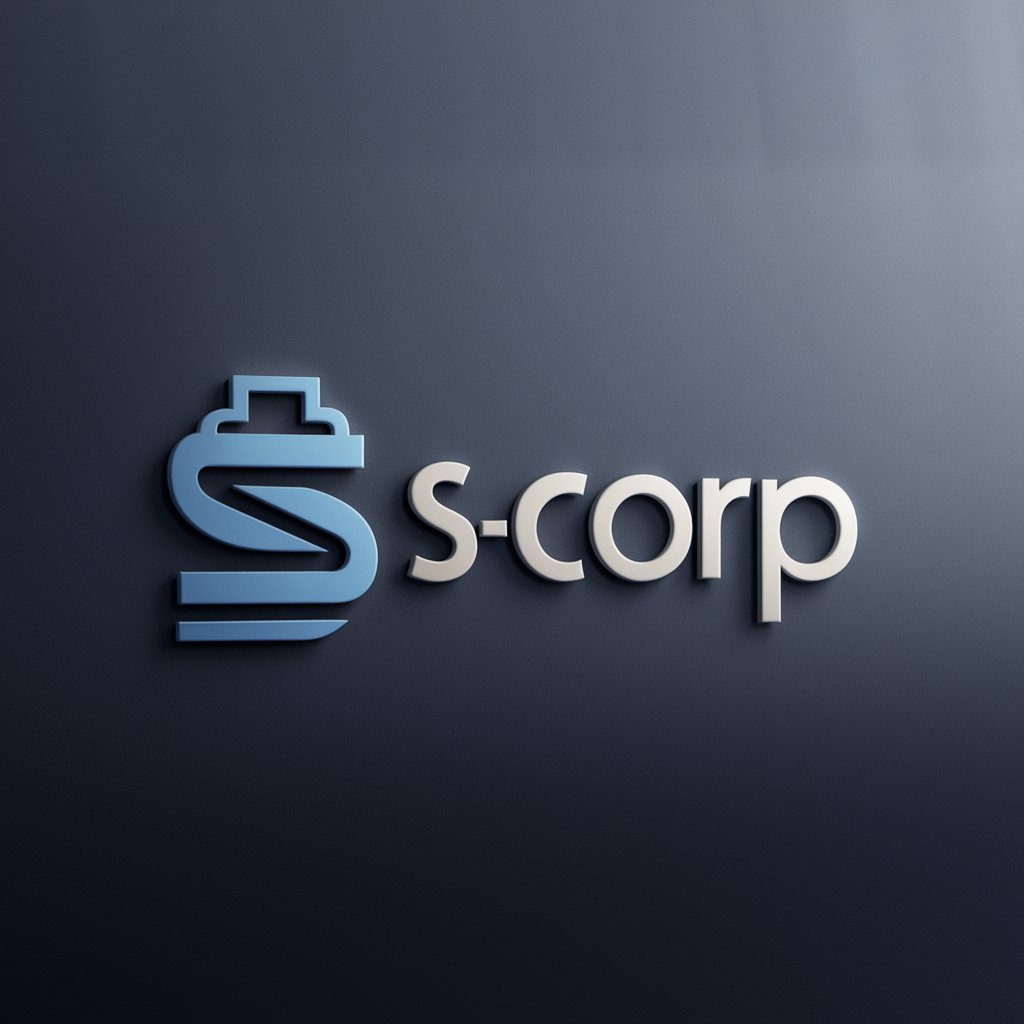
test gpt
Empowering creativity and productivity with AI
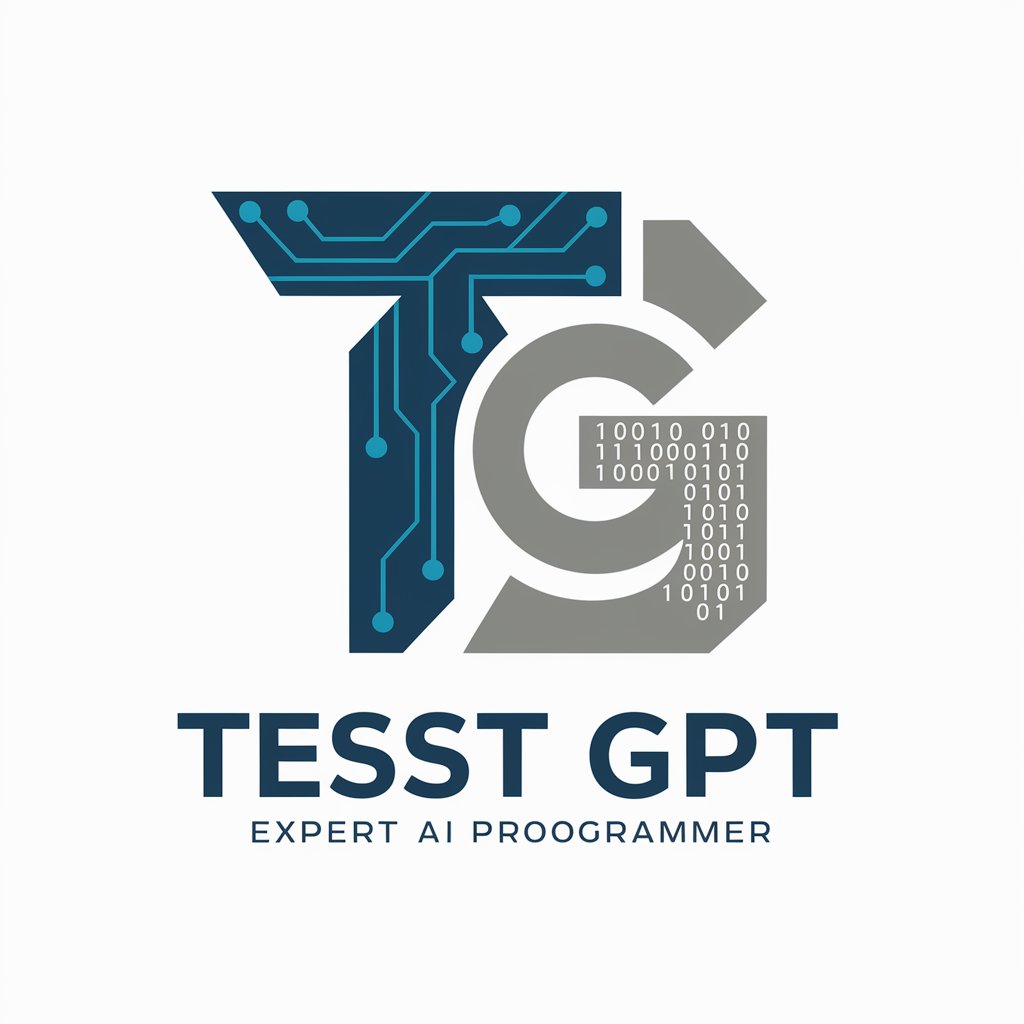
Q&A on Pre-visualization Data Preparation
What is pre-visualization data preparation?
It involves cleaning, normalizing, and structuring data to make it suitable for visualization.
Can I use this tool for large datasets?
Yes, it's designed to efficiently handle and process large volumes of data.
Does it support all data formats?
It supports a wide range of data formats, including CSV, JSON, and Excel, among others.
How does this tool help in improving data visualization?
By preparing data thoroughly, it ensures that visualizations are accurate, insightful, and easy to interpret.
Is any prior knowledge required to use this tool?
Basic understanding of data formats and visualization goals is helpful but not mandatory due to the tool's intuitive interface.
Create Stunning Music from Text with Brev.ai!
Turn your text into beautiful music in 30 seconds. Customize styles, instrumentals, and lyrics.
Try It Now