Pattern recognition in the nucleation kinetics-AI-powered Pattern Recognition
Deciphering Nucleation with AI
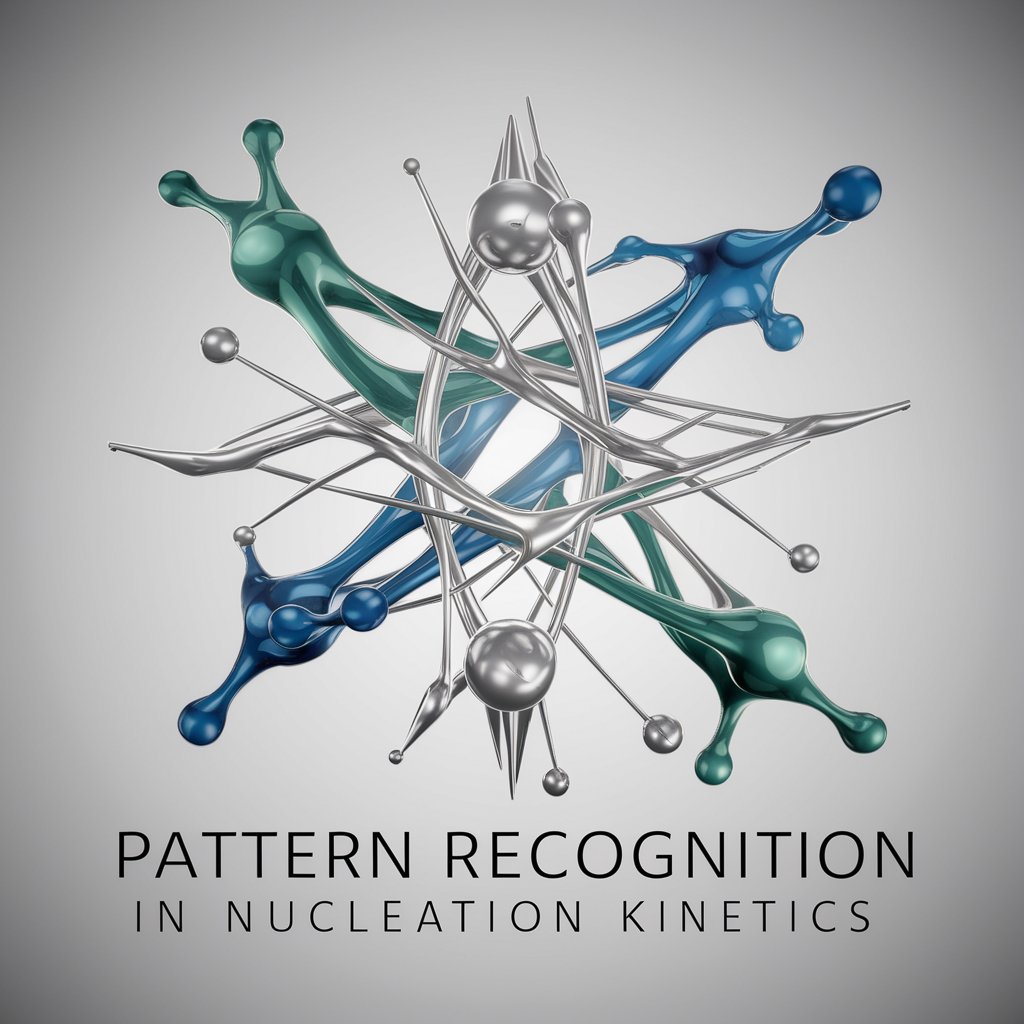
Explain the significance of pattern recognition in nucleation kinetics.
Describe the role of non-equilibrium self-assembly in biological systems.
How can AI enhance our understanding of nucleation kinetics?
What are the key challenges in studying non-equilibrium self-assembly?
Related Tools
Load More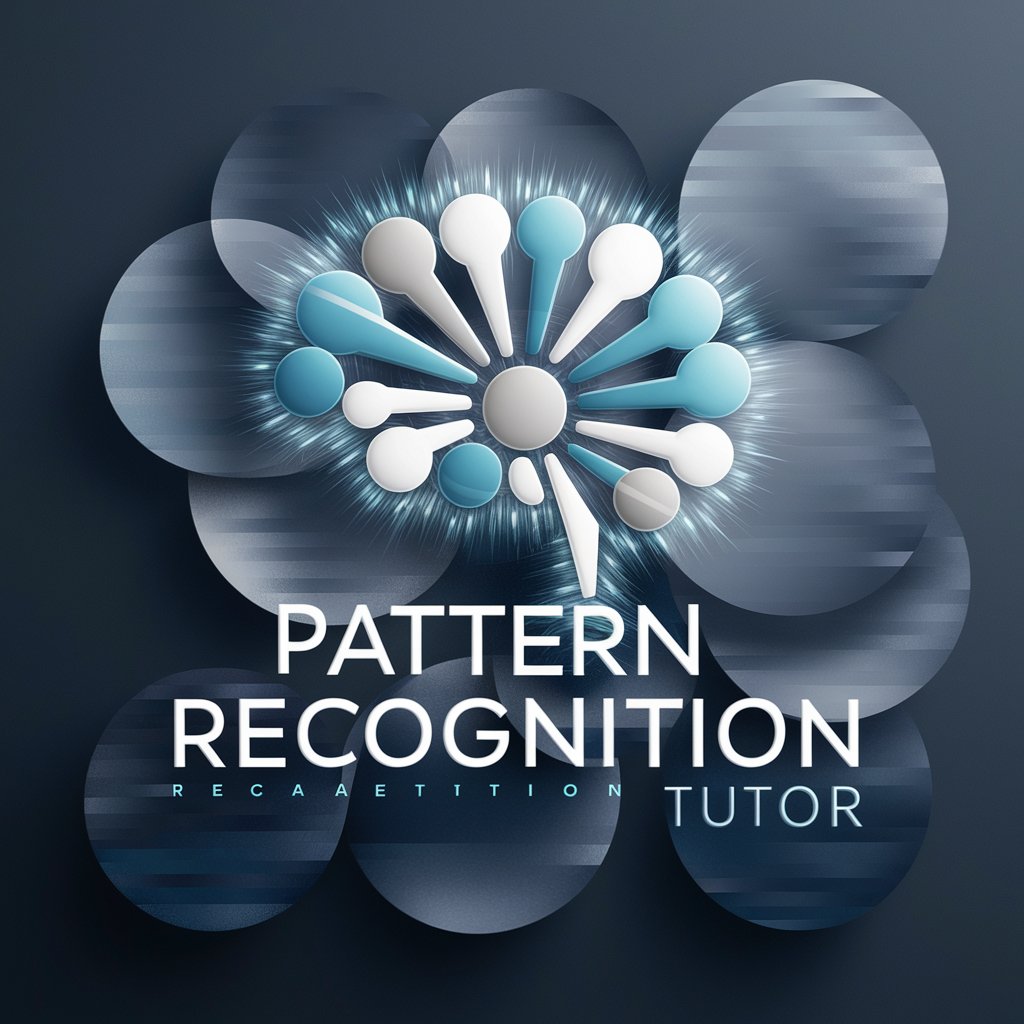
Pattern Recognition Tutor
Tutor for Pattern Recognition for graduate students, with online search.

PhotoCatalyst Tutor
Professional expert in photocatalysis, guiding in scientific analysis.
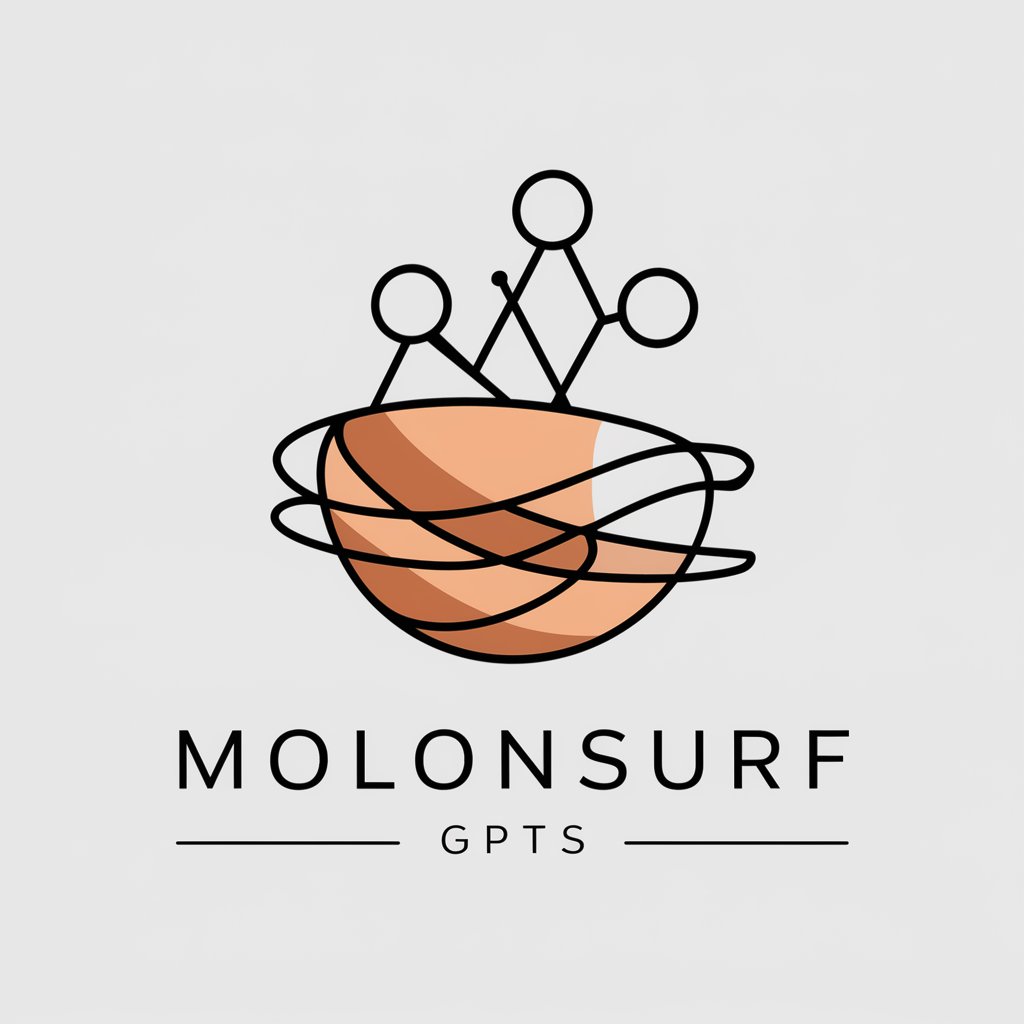
MolOnSurf GPTs
I offer professional molecular adsorption analysis on Cu surface.
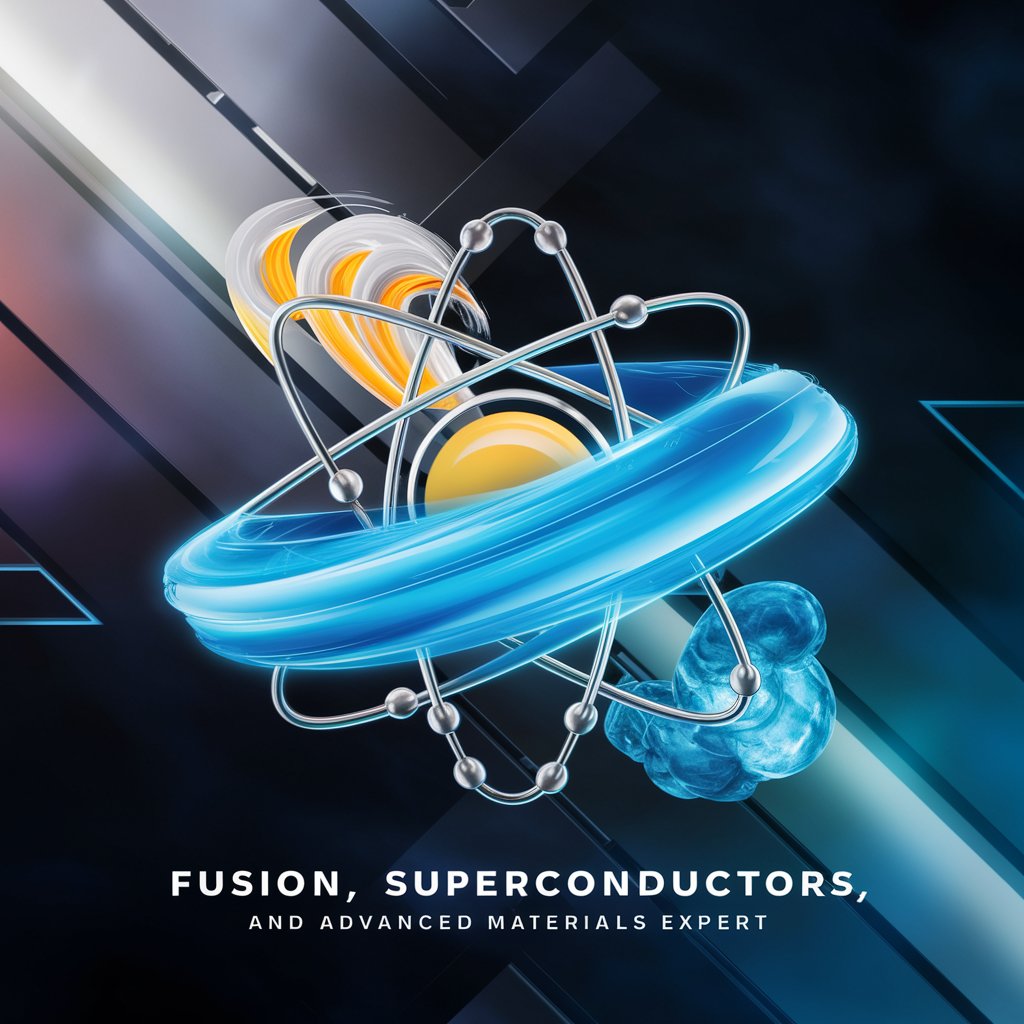
Nuclear Fusion Expert
Advanced expert in fusion, superconductors, and materials with enhanced analytics and collaboration.
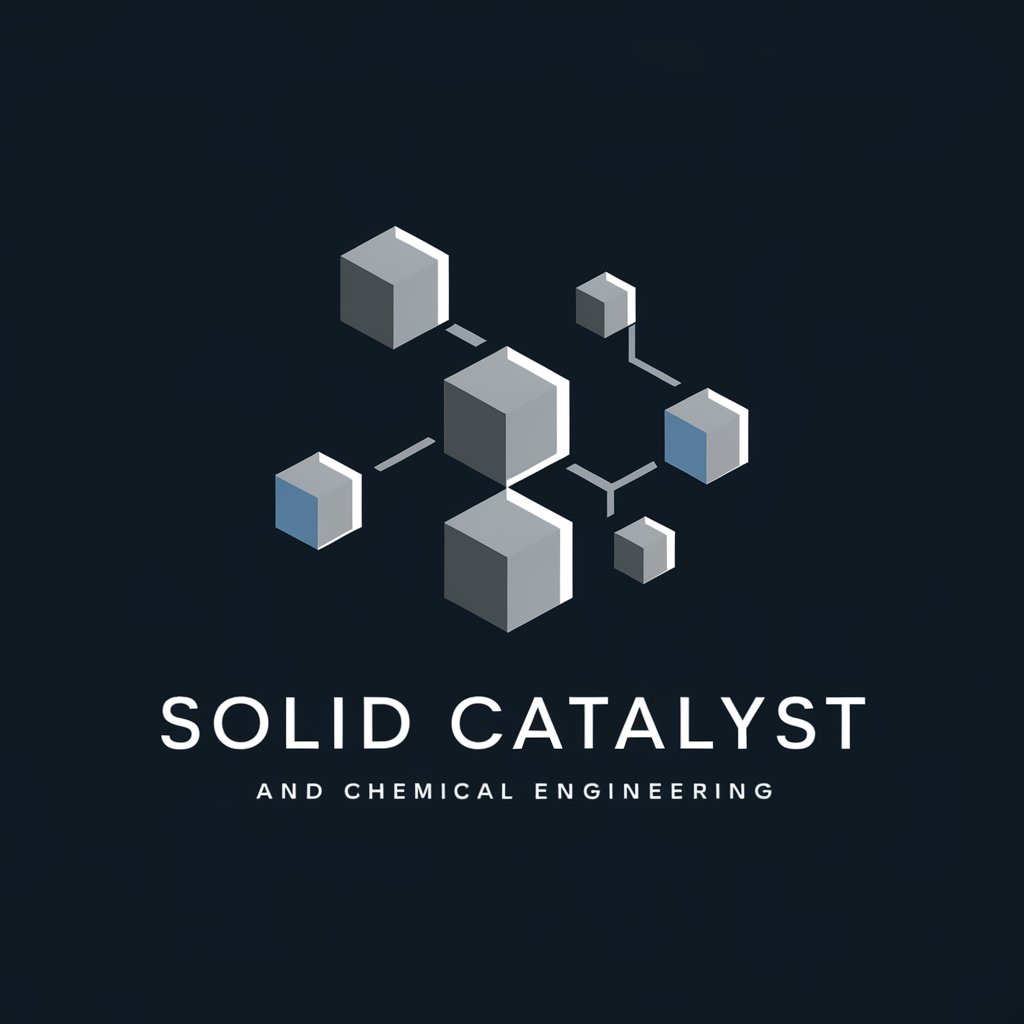
Heterogeneous Catalysts Tutor
A tutor for Heterogeneous Catalysts, providing detailed and researched answers.
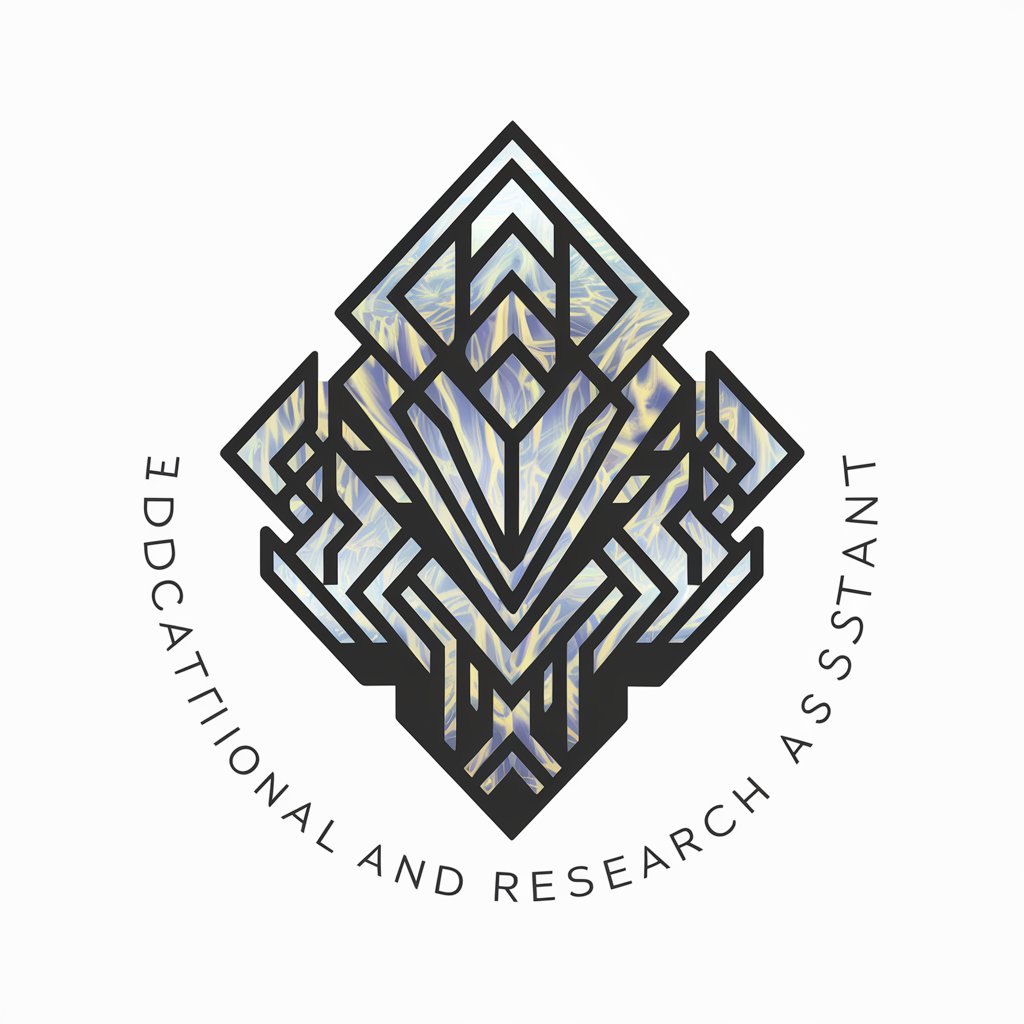
Quasicrystals and Understanding Crystallography
Expert in quasicrystals and crystallography with adaptable humor, trust, and language settings.
20.0 / 5 (200 votes)
Understanding Pattern Recognition in the Nucleation Kinetics
Pattern recognition in the nucleation kinetics focuses on identifying and analyzing patterns within the process of nucleation in non-equilibrium self-assembly systems. This involves the use of advanced algorithms and artificial intelligence (AI) techniques to understand how particles come together to form larger structures under conditions far from equilibrium. The primary goal is to decipher the complex mechanisms driving nucleation and to predict the outcomes of self-assembly processes under various conditions. An example scenario is in the formation of crystalline structures from a supersaturated solution, where recognizing patterns in nucleation kinetics can help in predicting the size, shape, and distribution of crystals formed. This insight is invaluable in fields such as materials science, pharmaceuticals, and nanotechnology, where controlled self-assembly processes are critical. Powered by ChatGPT-4o。
Core Functions of Pattern Recognition in Nucleation Kinetics
Prediction of Nucleation Outcomes
Example
Predicting the crystallization process of proteins which is crucial in drug development and materials engineering.
Scenario
Researchers can input environmental conditions into a model trained on nucleation kinetics data to predict how proteins will crystallize, aiding in the design of more effective drugs.
Analysis of Self-Assembly Mechanisms
Example
Understanding the self-assembly of nanoparticles into complex structures.
Scenario
By analyzing the patterns in how nanoparticles aggregate under different conditions, scientists can design nanoparticles that self-assemble into desired configurations for use in targeted drug delivery systems.
Optimization of Material Properties
Example
Enhancing the performance of photovoltaic cells through controlled nucleation of perovskite materials.
Scenario
Engineers can use pattern recognition in nucleation kinetics to optimize the nucleation process of perovskite materials, improving the efficiency and stability of solar cells.
Who Benefits from Pattern Recognition in Nucleation Kinetics?
Materials Scientists
These professionals can leverage pattern recognition in nucleation kinetics to design new materials with desired properties by controlling the self-assembly processes at the molecular or nanoscale level.
Pharmaceutical Researchers
In drug formulation and development, understanding and controlling the crystallization process is crucial. Pattern recognition in nucleation kinetics can provide insights into optimal conditions for drug synthesis and formulation, ensuring efficacy and stability.
Nanotechnology Engineers
For those designing devices and systems at the nanoscale, controlling the assembly of nanoparticles is key. Pattern recognition techniques can aid in the design of nanodevices with precise functionalities by predicting and guiding the self-assembly processes.
Guidelines for Using Pattern Recognition in Nucleation Kinetics
Start your journey
Begin by accessing a specialized platform offering a trial, such as visiting a site like yeschat.ai, where you can engage with the tool without the need for signing up or subscribing to premium services.
Understand the basics
Familiarize yourself with the fundamental concepts of nucleation kinetics and pattern recognition. This might involve reviewing academic literature or online resources that explain the principles of non-equilibrium self-assembly processes.
Collect and prepare your data
Gather experimental or simulated data related to your study of nucleation processes. Ensure your data is clean, well-organized, and formatted in a way that is compatible with the pattern recognition tool you plan to use.
Engage with the tool
Use the tool to analyze your data, applying pattern recognition algorithms to identify and characterize nucleation events. Take advantage of any customization options to tailor the analysis to your specific needs.
Interpret and apply findings
Review the results provided by the tool, interpreting the identified patterns in the context of your research. Apply these insights to inform further experiments, simulations, or theoretical models.
Try other advanced and practical GPTs
DadGokePT
Crafting Humor with AI Precision
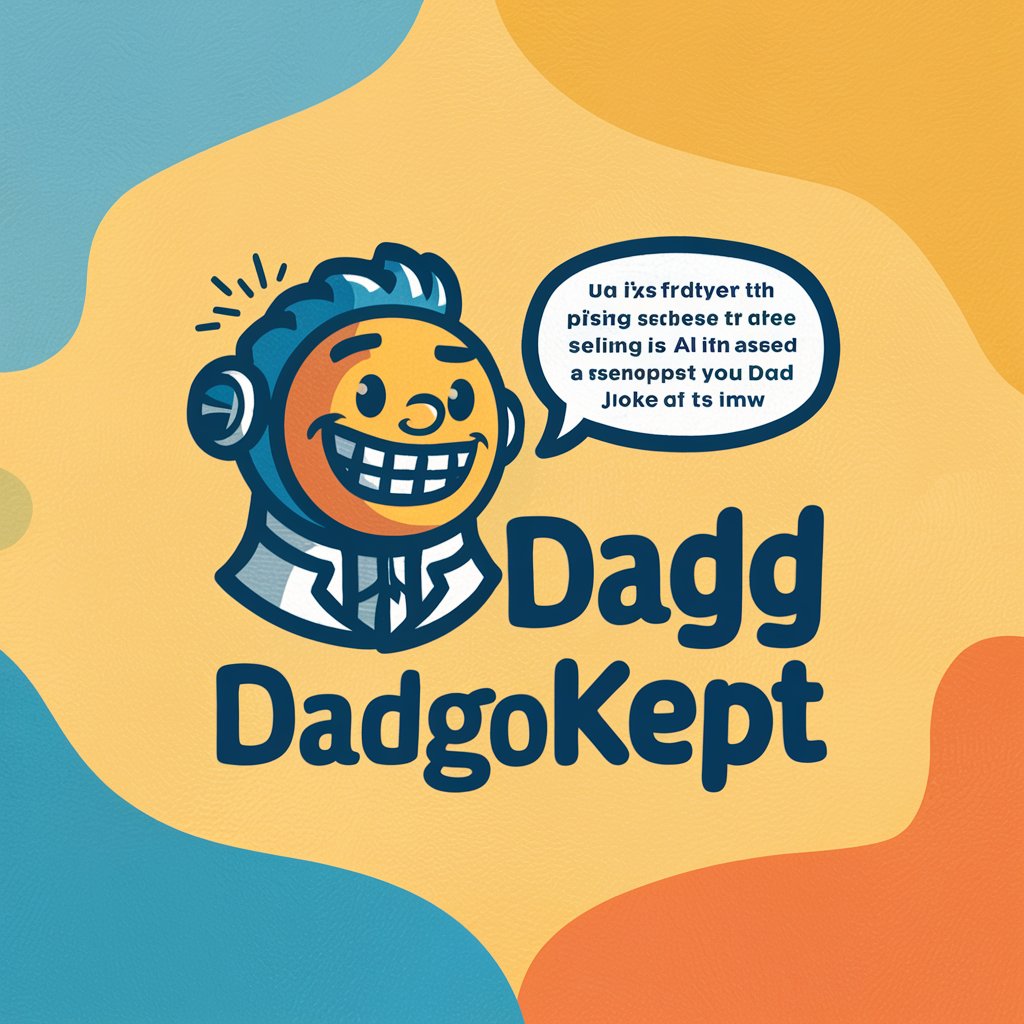
Kerbside Collection Guide
AI-powered Recycling Simplified
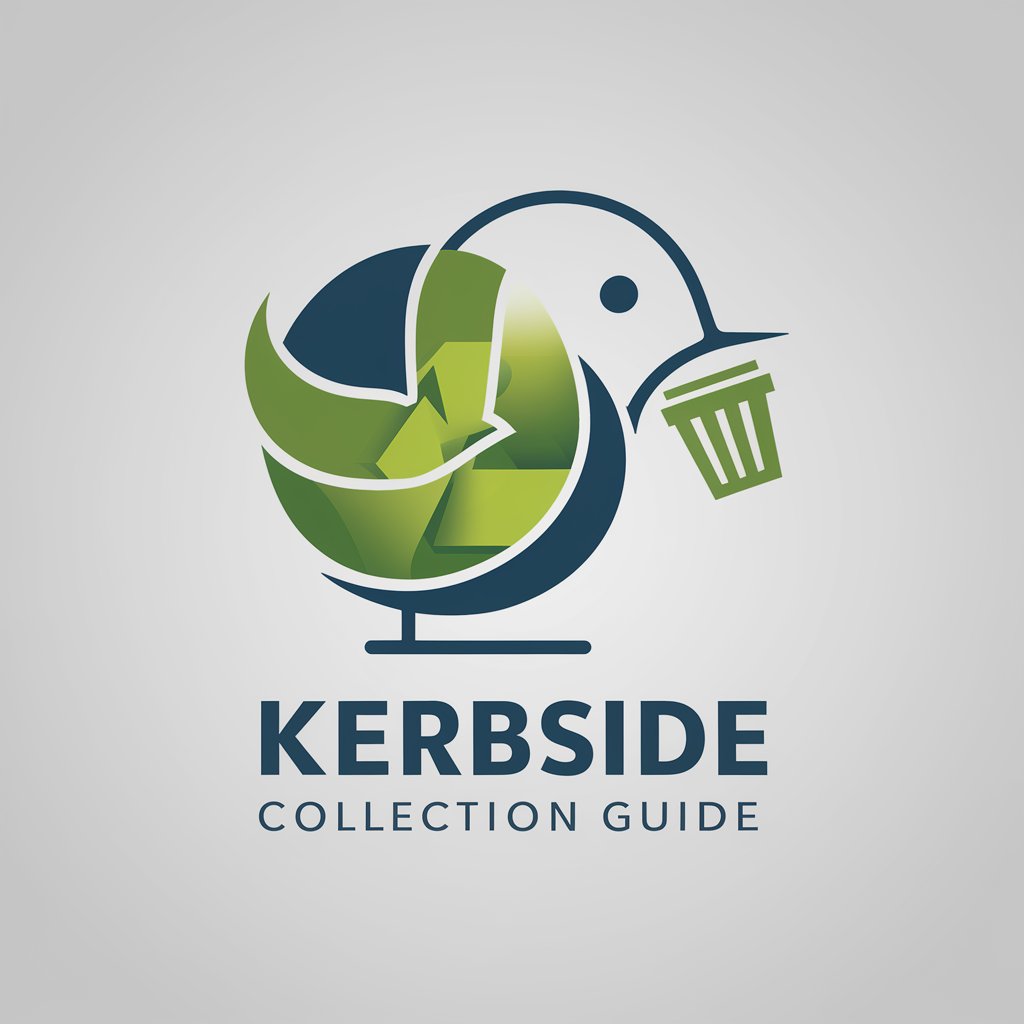
Kpop Story ai
Your AI-powered K-pop Companion
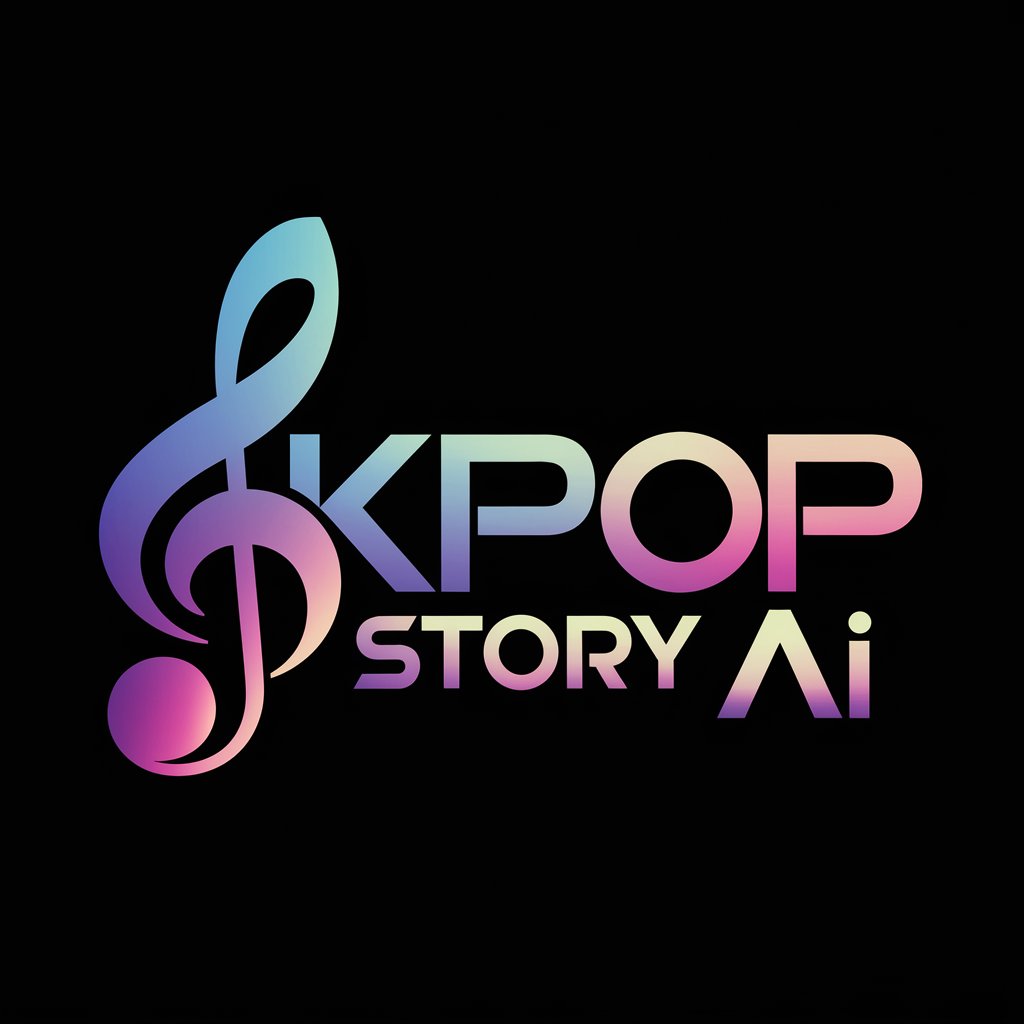
ストーリーをイラスト君
Bringing stories to life with AI-powered illustrations.
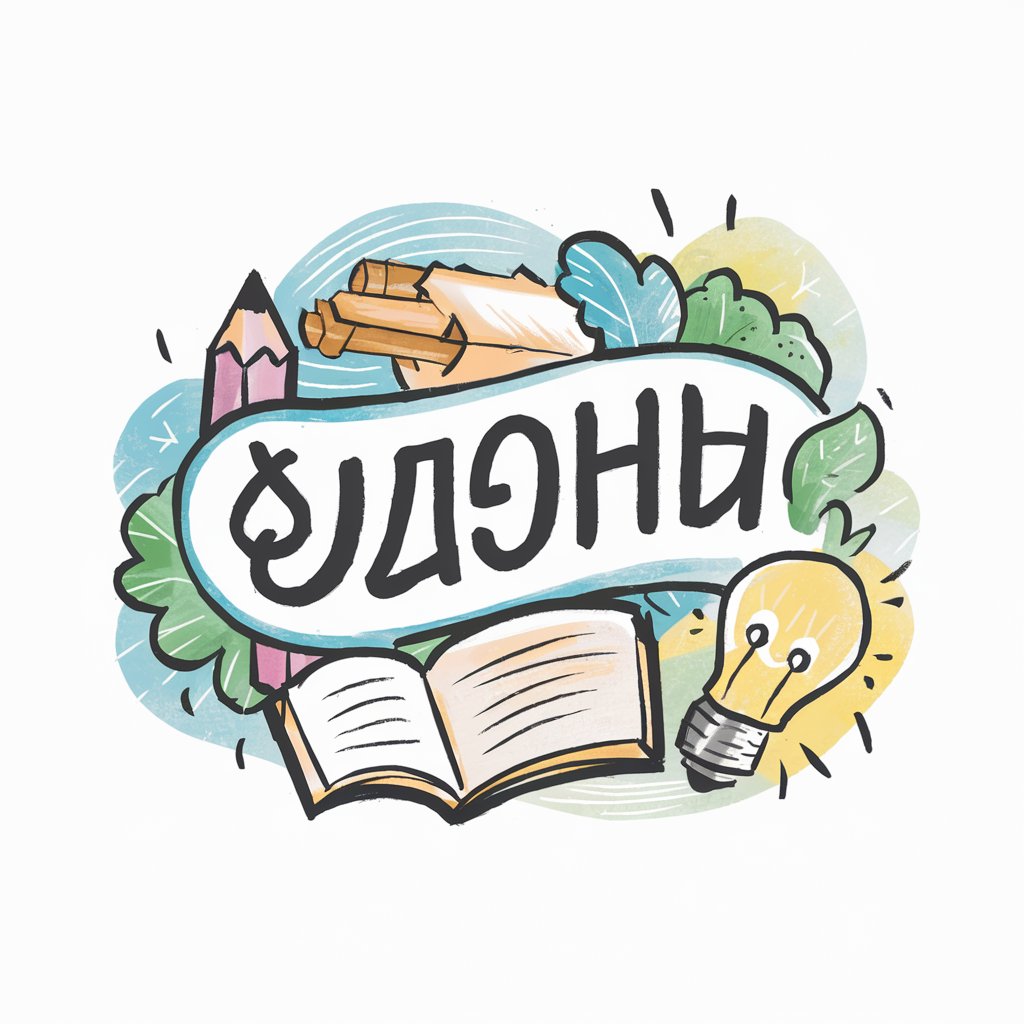
Writer Hivemind by MetaMinds.App
Empower Your Writing with AI Diversity
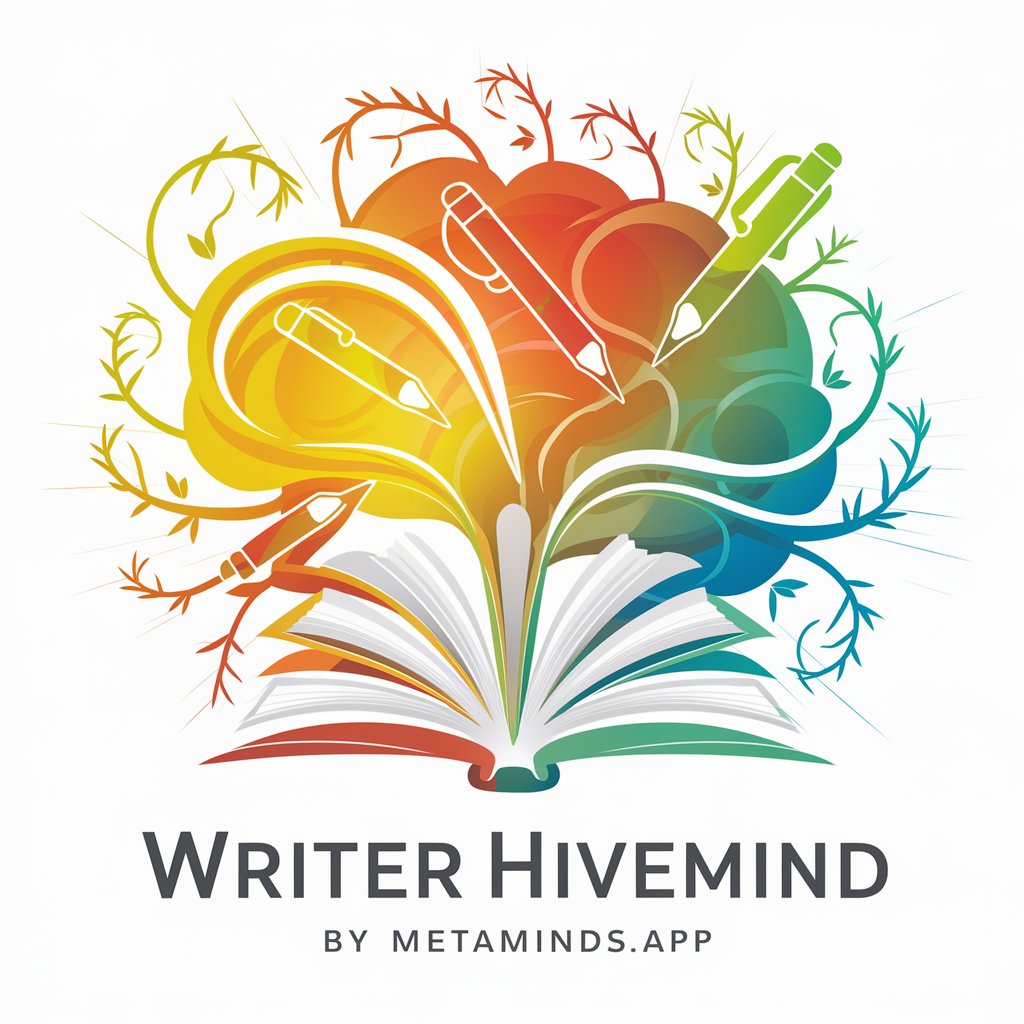
Global Tech Navigator
Empowering Your Google Experience with AI
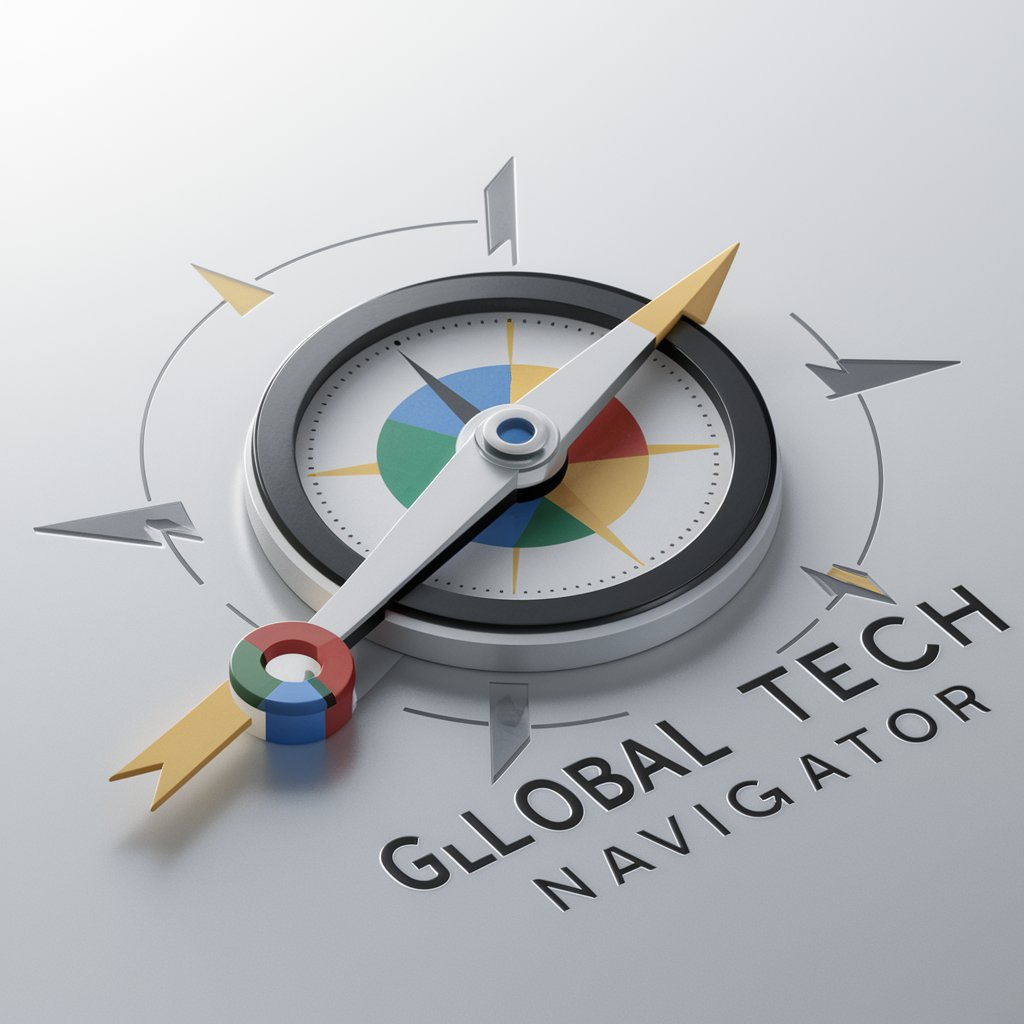
Video Title Generator
Crafting Your Story's Headline with AI
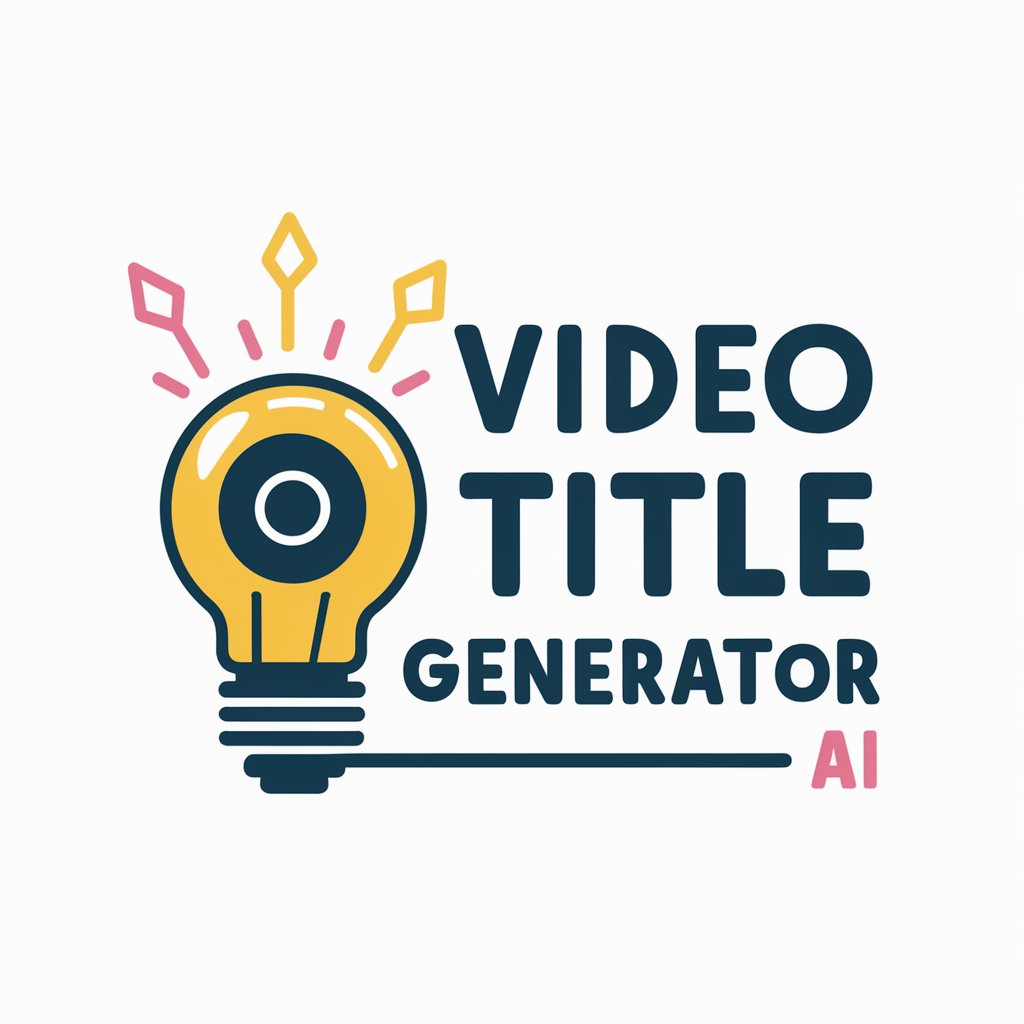
Wing Whiz
Identify birds with AI-powered precision.
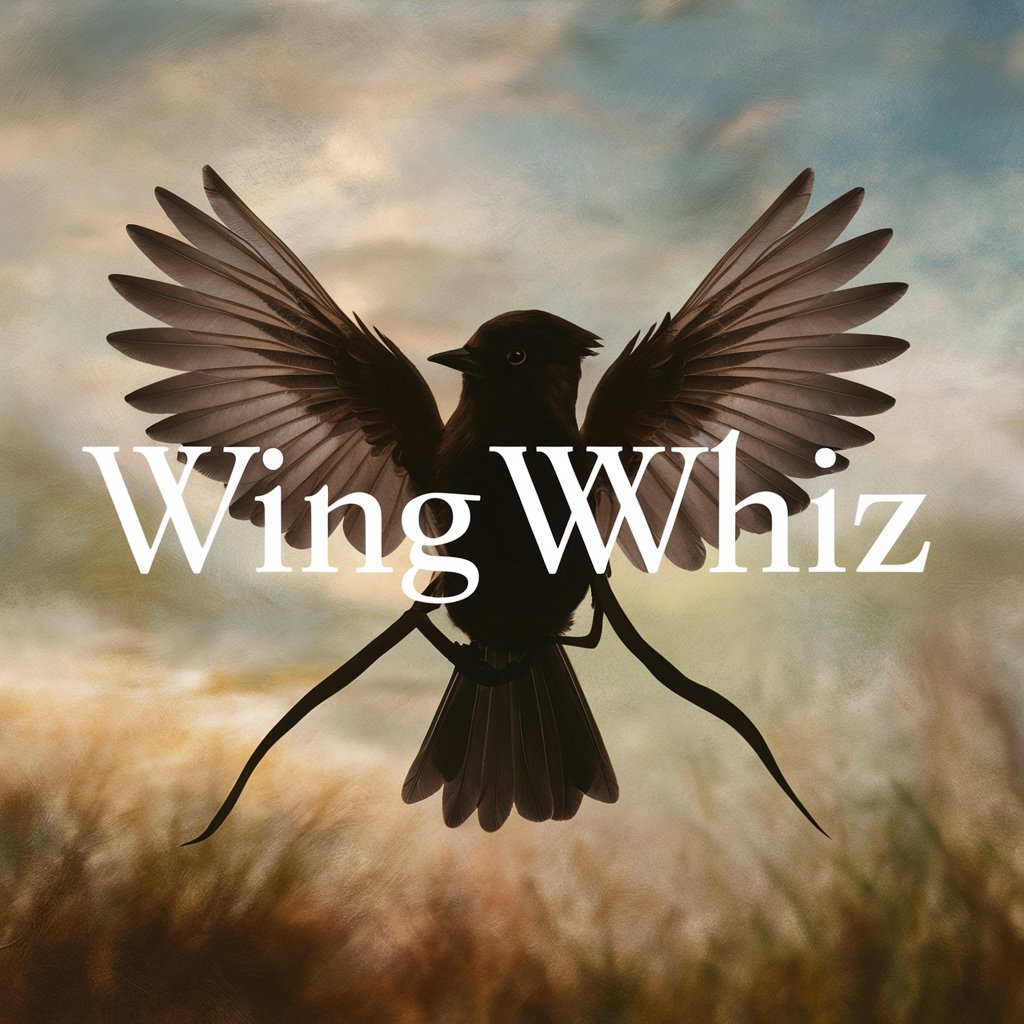
How To
Empowering Decisions with AI
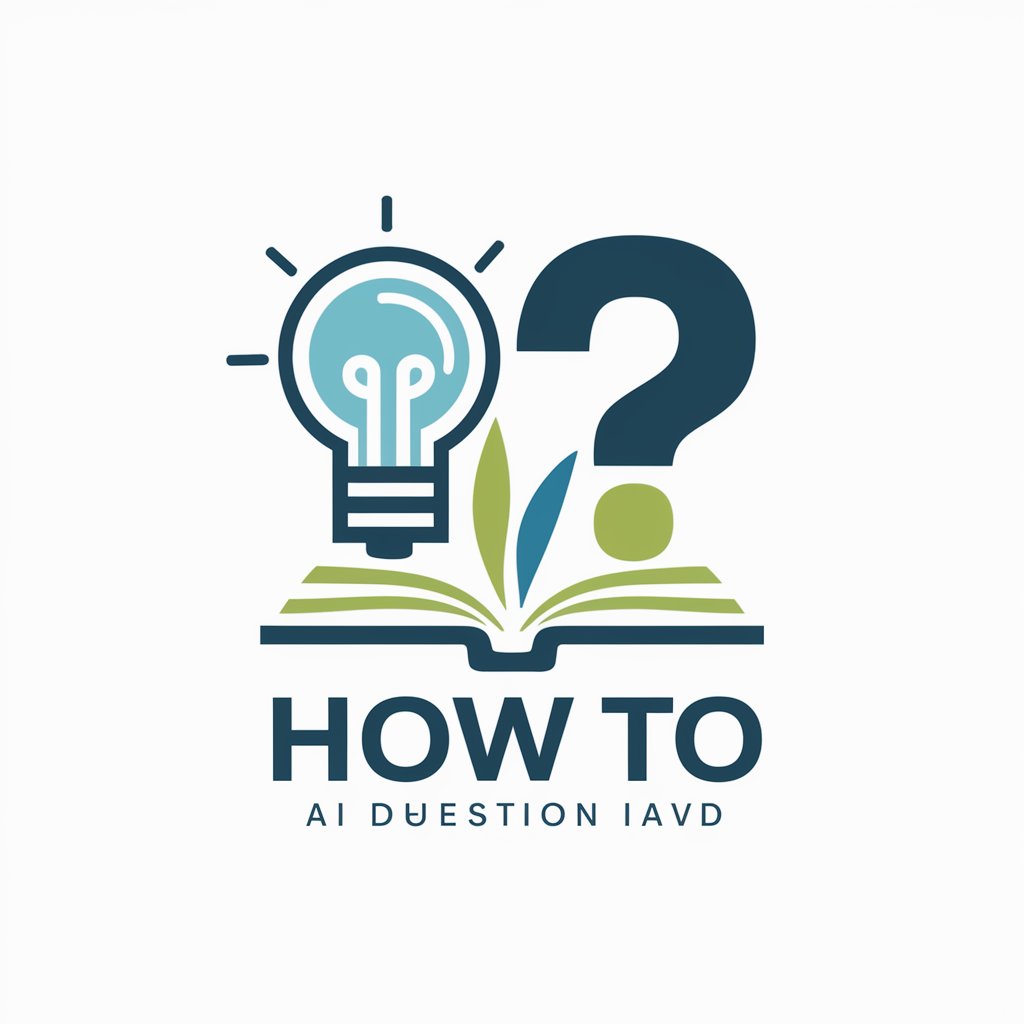
Horoscope GPT
Unlock Your Cosmic Potential with AI
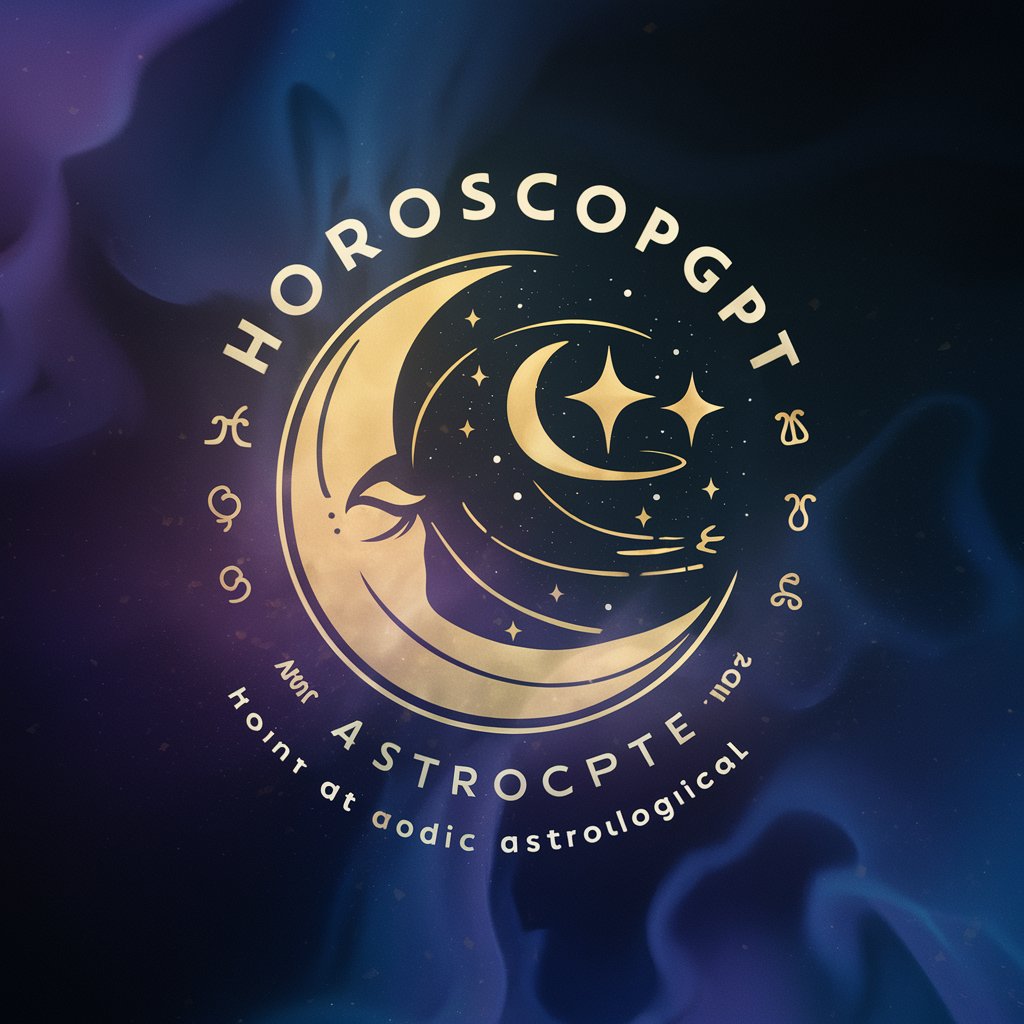
September In Miami meaning?
Unlock Insights with AI
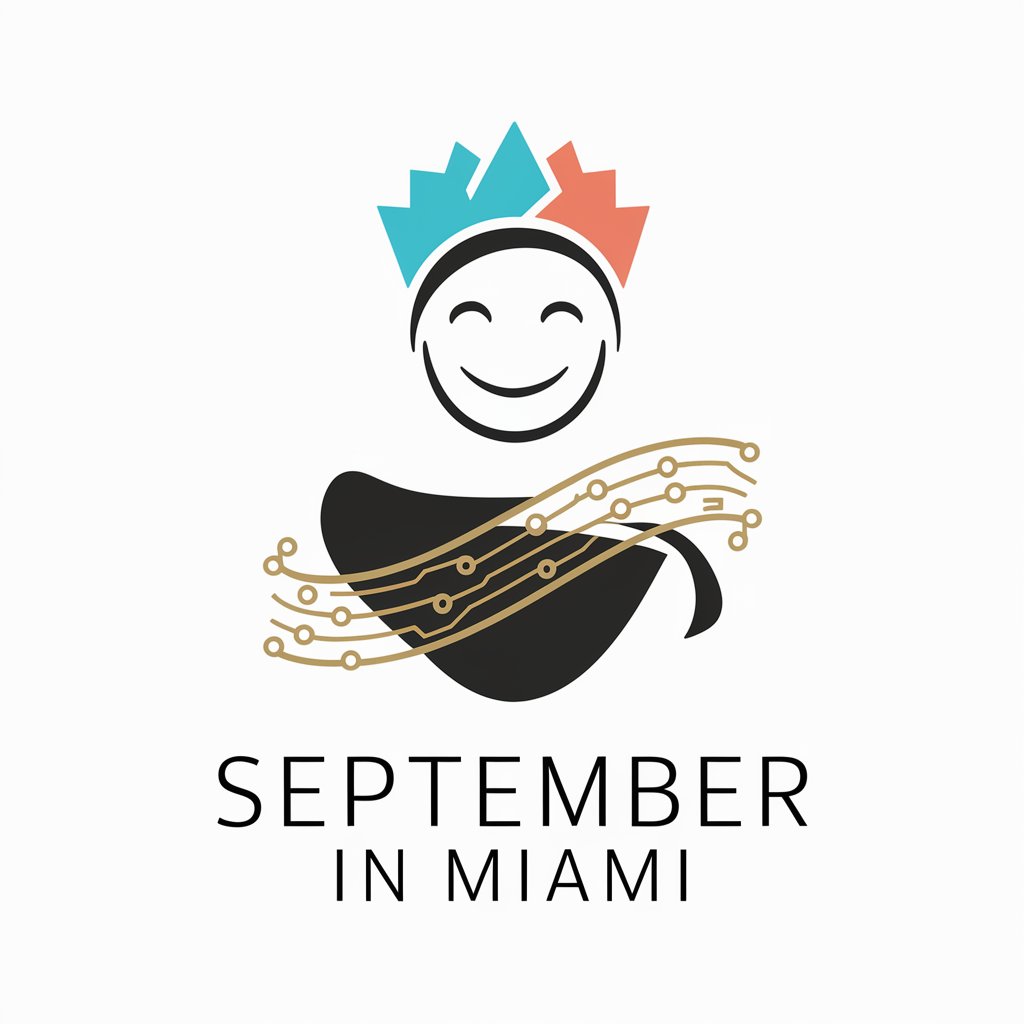
DEFCON.CONSULTING:TRIZ
Innovate smarter, faster with AI-powered TRIZ
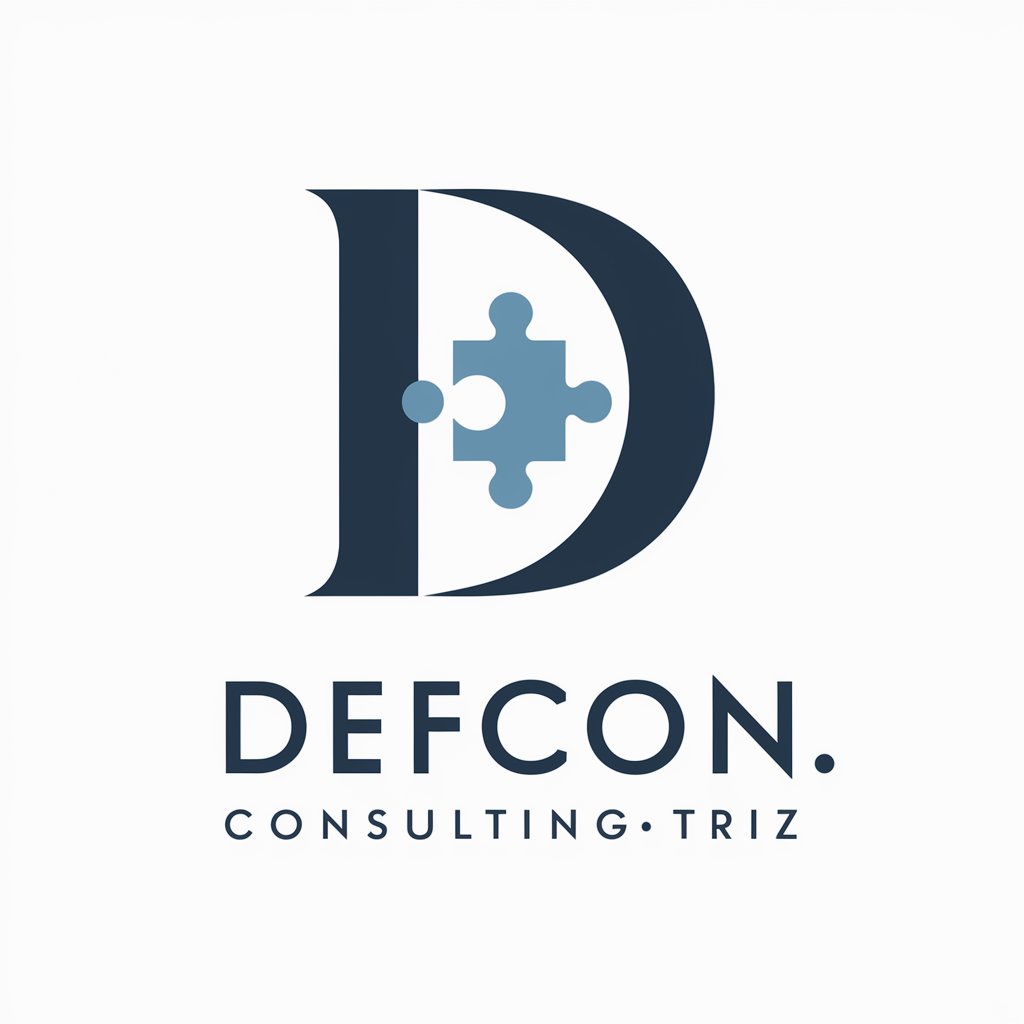
Q&A on Pattern Recognition in Nucleation Kinetics
What is pattern recognition in nucleation kinetics?
Pattern recognition in nucleation kinetics involves the use of algorithms to analyze data from non-equilibrium self-assembly processes, identifying patterns that represent nucleation events. This approach facilitates the understanding of how nucleation occurs and progresses under various conditions.
Why is pattern recognition important for studying nucleation kinetics?
Pattern recognition enables researchers to detect and analyze complex nucleation events that may be difficult to identify through traditional methods. It provides a quantitative basis for understanding the kinetics of nucleation, supporting the development of models and theories in material science and beyond.
What types of data are needed for pattern recognition in nucleation kinetics?
The data typically includes time-lapse images, spectroscopy data, or simulations that capture the dynamic process of nucleation. This data should be detailed enough to allow for the identification of specific patterns or changes indicating nucleation events.
How can AI improve the analysis of nucleation kinetics?
AI, through machine learning and pattern recognition, can process and analyze large datasets more efficiently than traditional methods, uncovering hidden patterns and correlations. It can also predict nucleation behavior under untested conditions, significantly advancing our understanding of these processes.
What are the practical applications of understanding nucleation kinetics through pattern recognition?
Insights gained from such analyses can inform the design of materials with desired properties, improve industrial crystallization processes, and contribute to advancements in pharmaceuticals, nanotechnology, and materials engineering by optimizing conditions for desired nucleation outcomes.
Create Stunning Music from Text with Brev.ai!
Turn your text into beautiful music in 30 seconds. Customize styles, instrumentals, and lyrics.
Try It Now