Statistics and data analysis - AI-Powered Data Insights
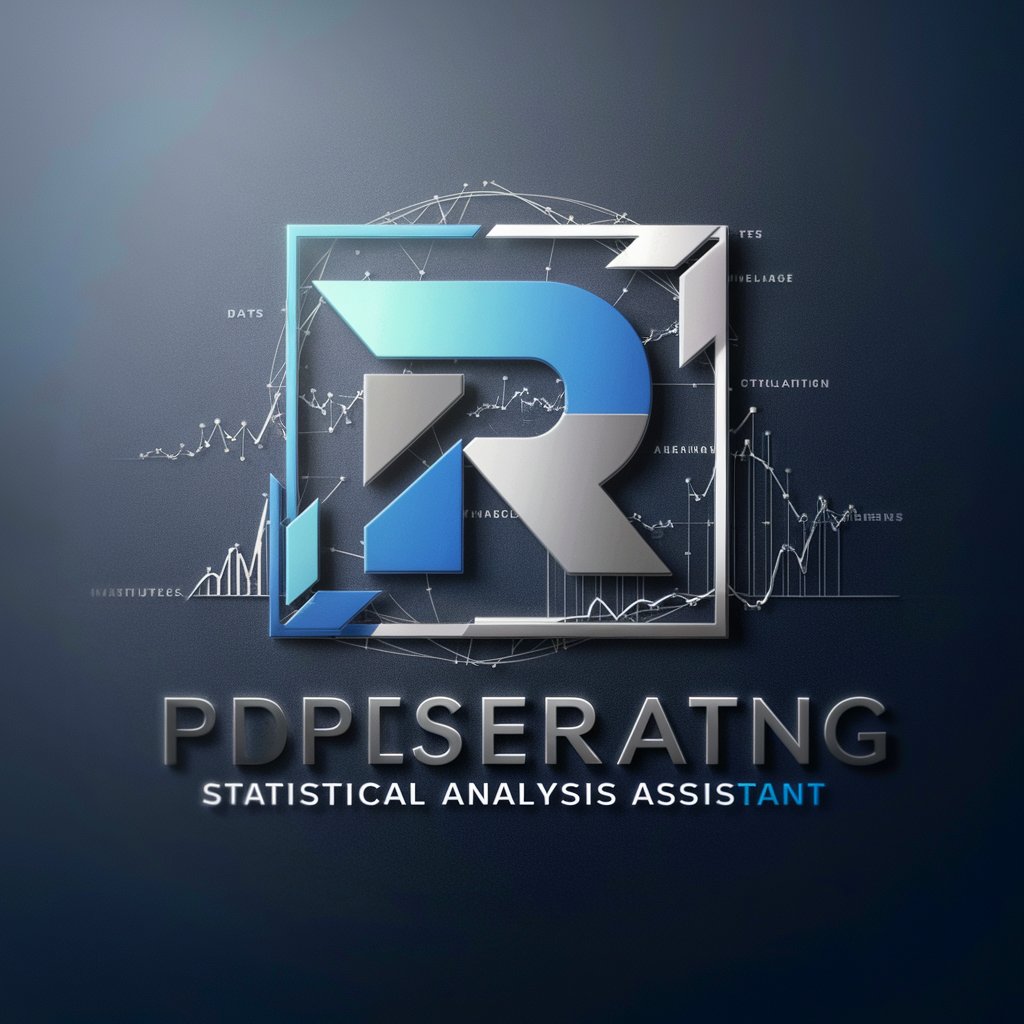
Hello! I'm here to help with your statistical and data analysis needs.
Unlock Insights with AI-Driven Analysis
Analyze a dataset to uncover trends and insights...
Write R code to perform data manipulation on...
Develop a machine learning model using R for...
Generate statistical summaries and visualizations for...
Get Embed Code
Overview of Statistics and Data Analysis
Statistics and data analysis encompass a broad range of techniques and methodologies designed for collecting, analyzing, interpreting, and presenting empirical data. This field is fundamental to empirical research across disciplines, enabling evidence-based decision-making and theoretical insights. For example, in healthcare, statistical analysis might be used to determine the effectiveness of a new treatment compared to existing ones by analyzing patient recovery rates, side effects, and other relevant metrics. In economics, it can help forecast market trends by analyzing past financial data, consumer behavior, and economic indicators. Powered by ChatGPT-4o。
Key Functions and Applications
Descriptive Statistics
Example
Calculating mean, median, mode, and standard deviation of a dataset.
Scenario
A market researcher uses descriptive statistics to summarize customer feedback scores for a new product, helping the company understand the average satisfaction level and variability in customer opinions.
Inferential Statistics
Example
Performing hypothesis testing and confidence intervals.
Scenario
A pharmaceutical company employs inferential statistics to determine whether a new drug is more effective than a placebo in clinical trials, based on a sample of trial participants.
Predictive Modeling
Example
Using linear regression, logistic regression, or machine learning models to predict future outcomes.
Scenario
A financial analyst uses predictive modeling to forecast stock prices based on historical data, economic indicators, and market sentiment, aiding investment decisions.
Data Visualization
Example
Creating charts, graphs, and interactive dashboards.
Scenario
A public health official uses data visualization to present the spread of an epidemic over time and geography, facilitating a better understanding among the public and policymakers.
Machine Learning
Example
Applying algorithms to automate data analysis, pattern recognition, and prediction.
Scenario
An e-commerce platform employs machine learning to personalize product recommendations for users based on their browsing and purchase history, enhancing customer experience and sales.
Target User Groups
Researchers and Academics
Individuals in academic and research settings who require robust analysis to validate theories, test hypotheses, and contribute new knowledge across various fields such as psychology, sociology, and natural sciences.
Business Analysts and Marketers
Professionals who rely on data to make informed decisions, understand market trends, evaluate marketing strategies, and optimize operational efficiency.
Healthcare Professionals
Including epidemiologists, clinical researchers, and healthcare providers who need to analyze patient data, study treatment effectiveness, and manage public health initiatives.
Finance and Investment Analysts
Individuals in the finance sector who analyze market data, forecast economic trends, and advise on investment strategies to maximize returns and minimize risks.
Data Scientists and Machine Learning Engineers
Experts who specialize in extracting insights from complex datasets using advanced statistical, computational, and machine learning techniques to drive innovation and solve complex problems.
Guidelines for Using Statistics and Data Analysis
Start with a Trial
Begin your journey by exploring yeschat.ai, where you can access a free trial without the need for login credentials or subscribing to ChatGPT Plus.
Identify Your Objectives
Clearly define your analysis goals. Whether it's for academic research, business analytics, or personal projects, understanding your objectives helps tailor your approach.
Gather and Prepare Data
Collect the necessary data relevant to your analysis. Ensure it is cleaned and formatted properly for accurate and efficient analysis.
Analyze and Interpret
Utilize statistical methods and data analysis techniques to uncover patterns, trends, and insights. Tools and software like R or Python can be particularly helpful.
Apply Findings
Use the insights gained from your analysis to inform decisions, support hypotheses, or contribute to further research. Documenting and sharing your findings can also be beneficial.
Try other advanced and practical GPTs
Python Data Analysis
AI-powered insights from your data
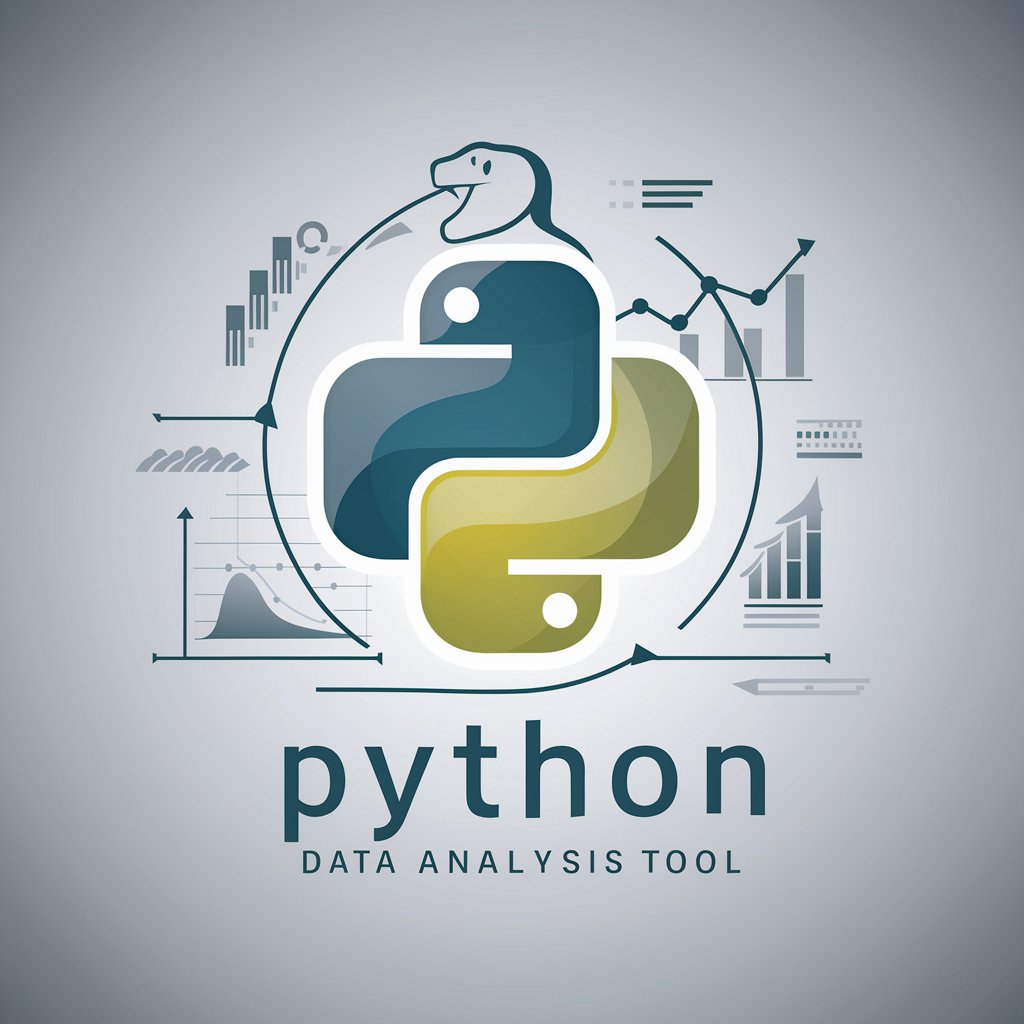
Exporitory Data Analysis (EDA)
Empower your data with AI-driven analysis
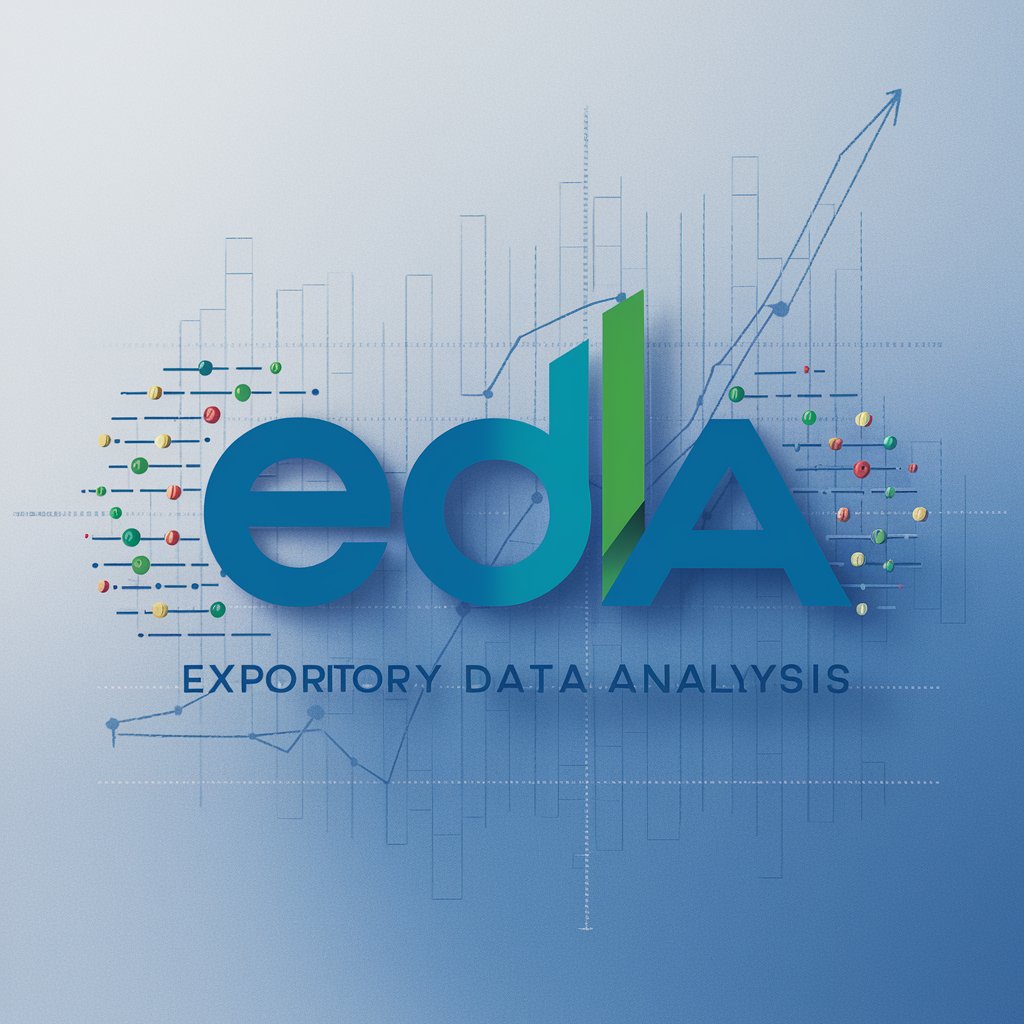
Jarvis
Empowering efficiency with AI
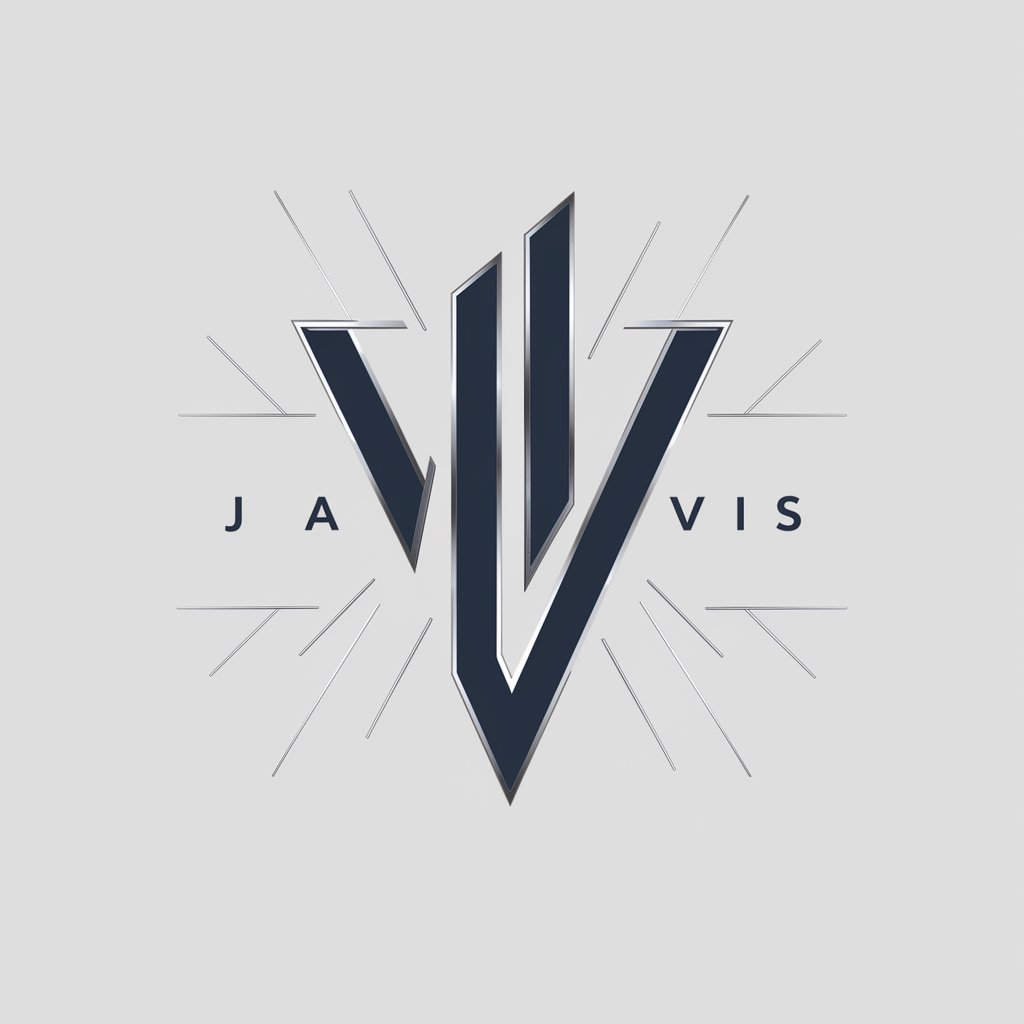
Technology Stack
Empowering Industries with AI
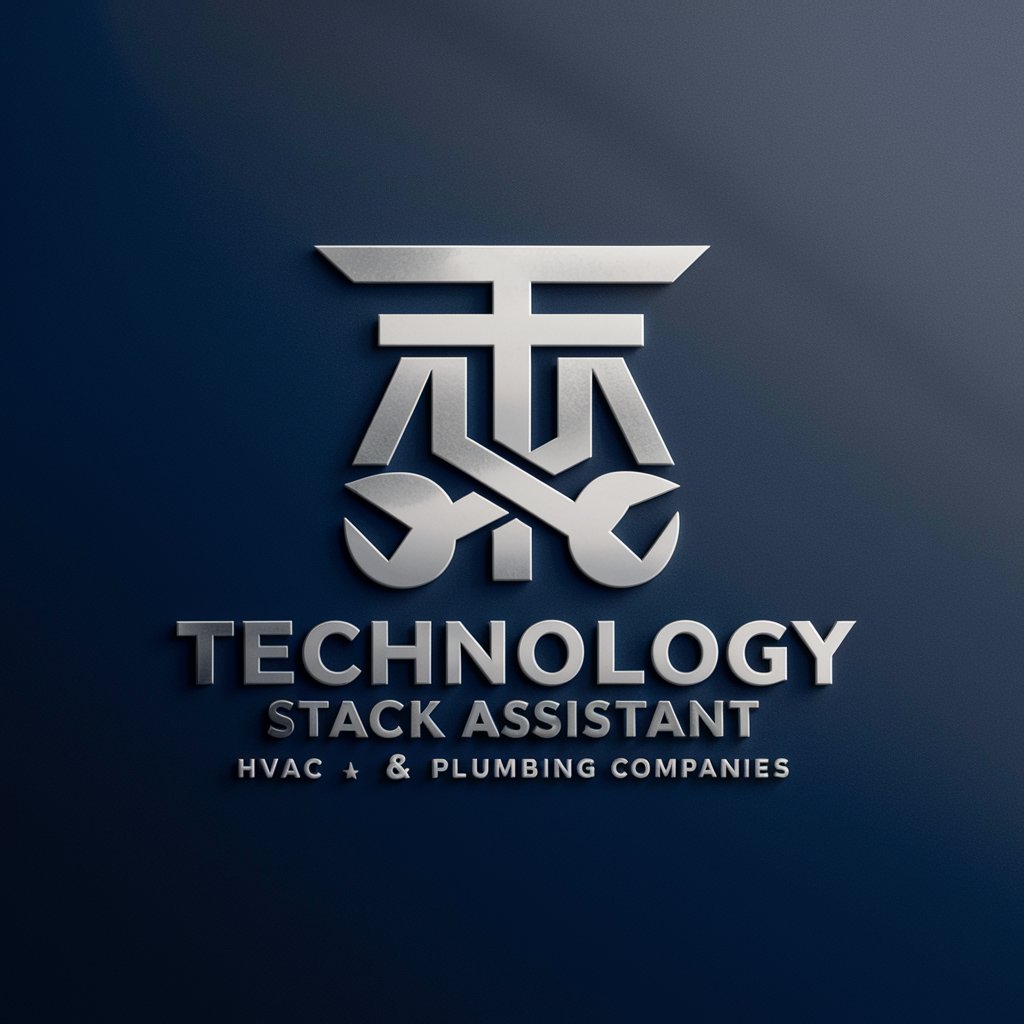
KANJI NAME CONVERTER
Transform Your Name into Art with AI
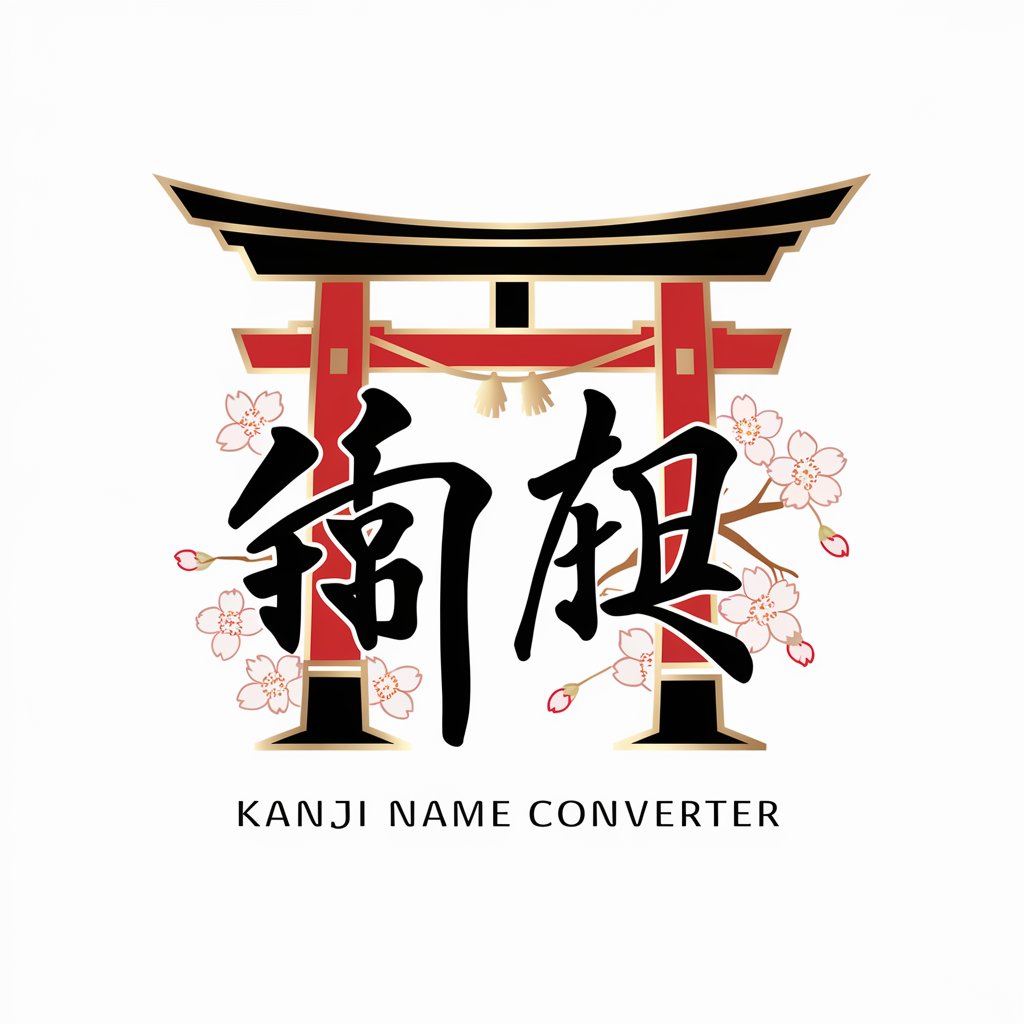
Book Summary
Unlock Knowledge with AI-Powered Summaries
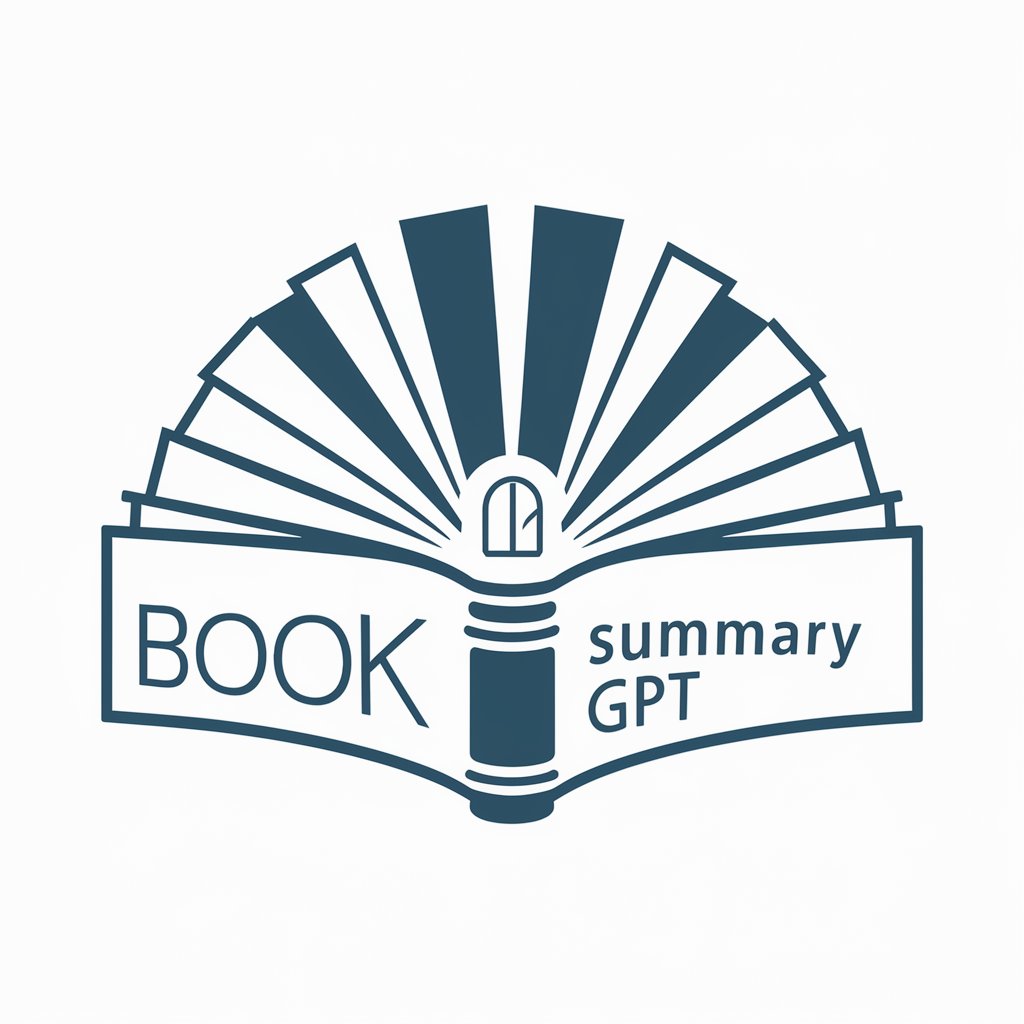
Data Analysis With Excel GPT
Empowering Excel analysis with AI
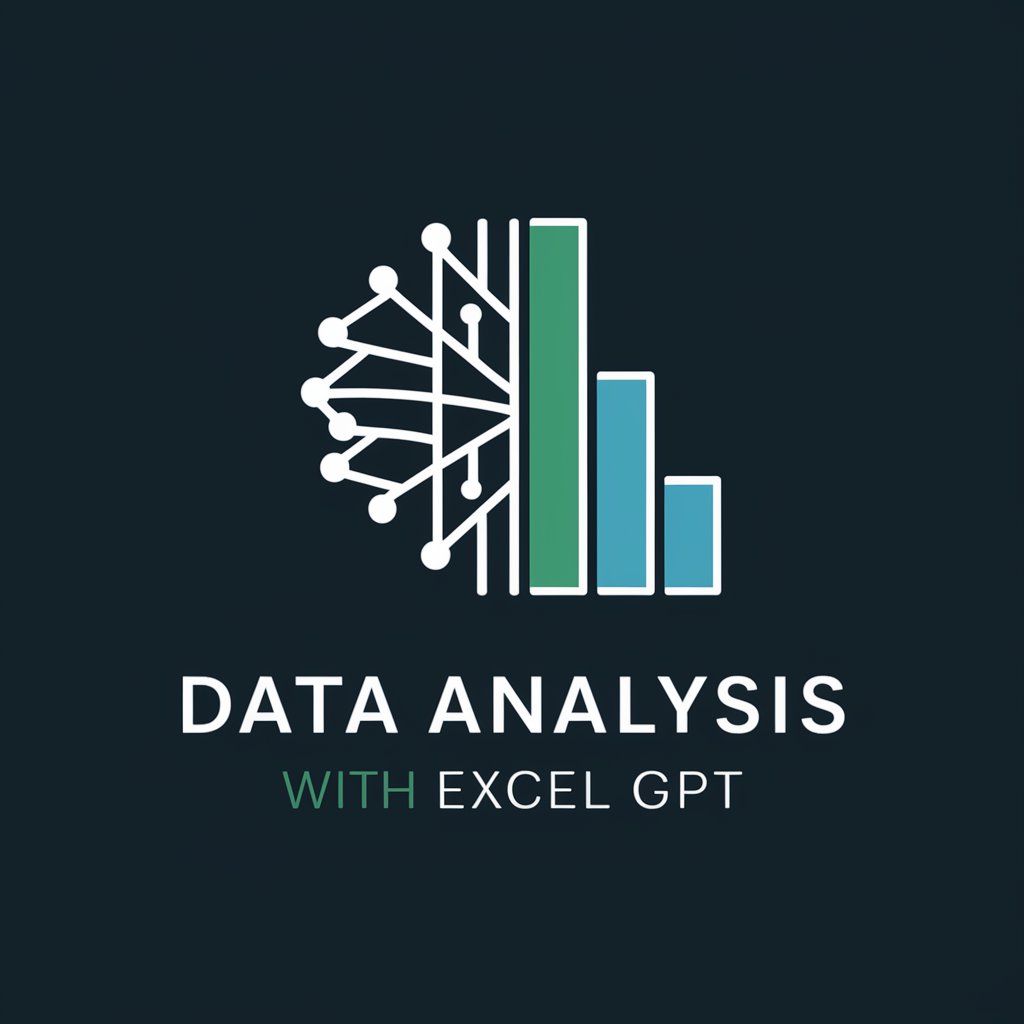
Advanced Data Analysis & Guiderails
AI-powered insights at your fingertips
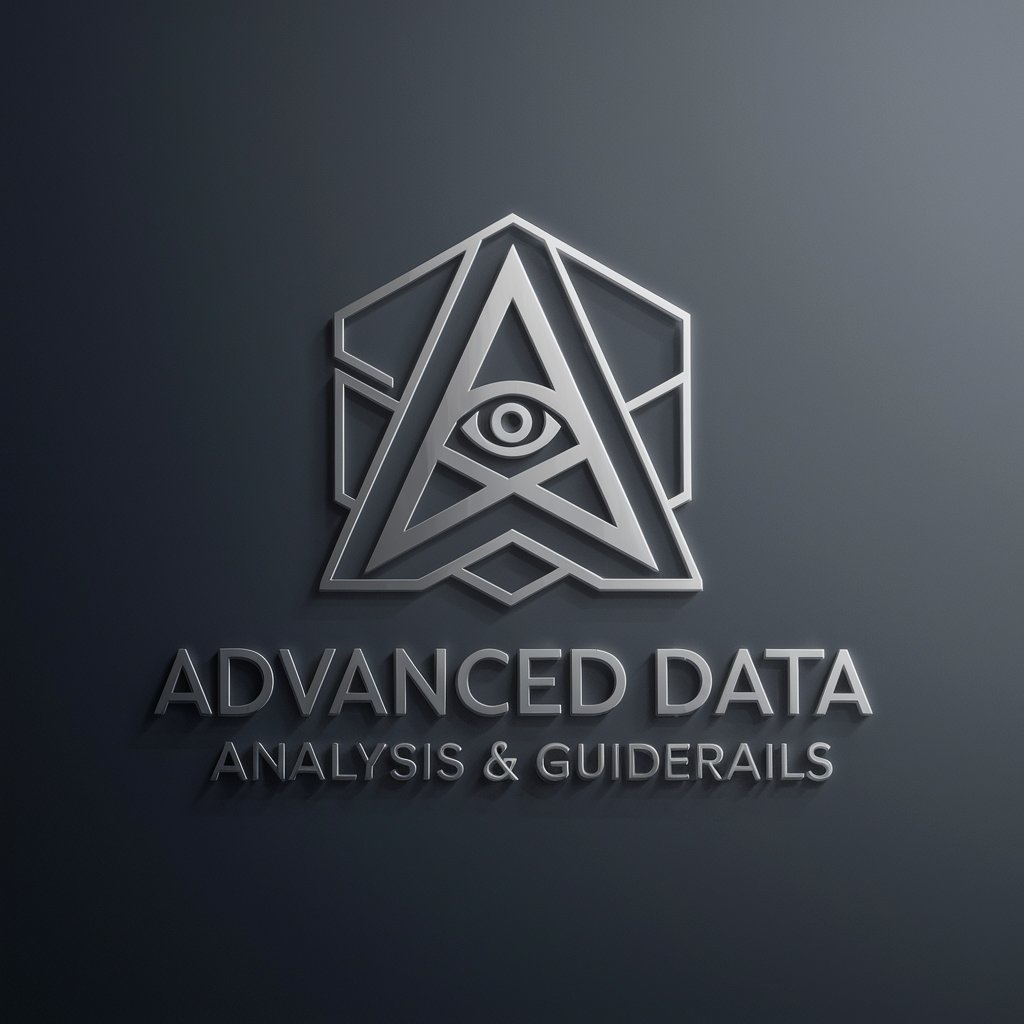
Data Analysis GPT
Empowering Data Decisions with AI
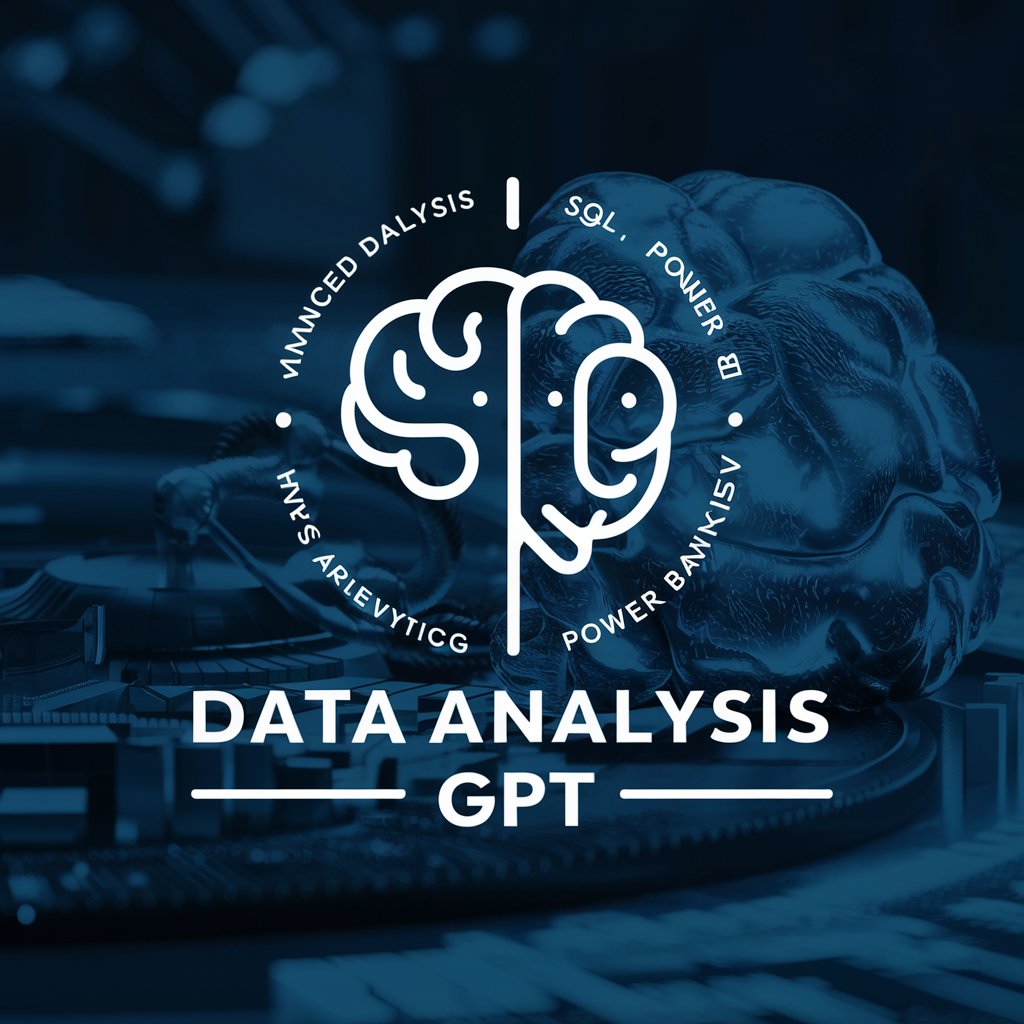
Qualitative Data Analysis
AI-powered thematic insight discovery
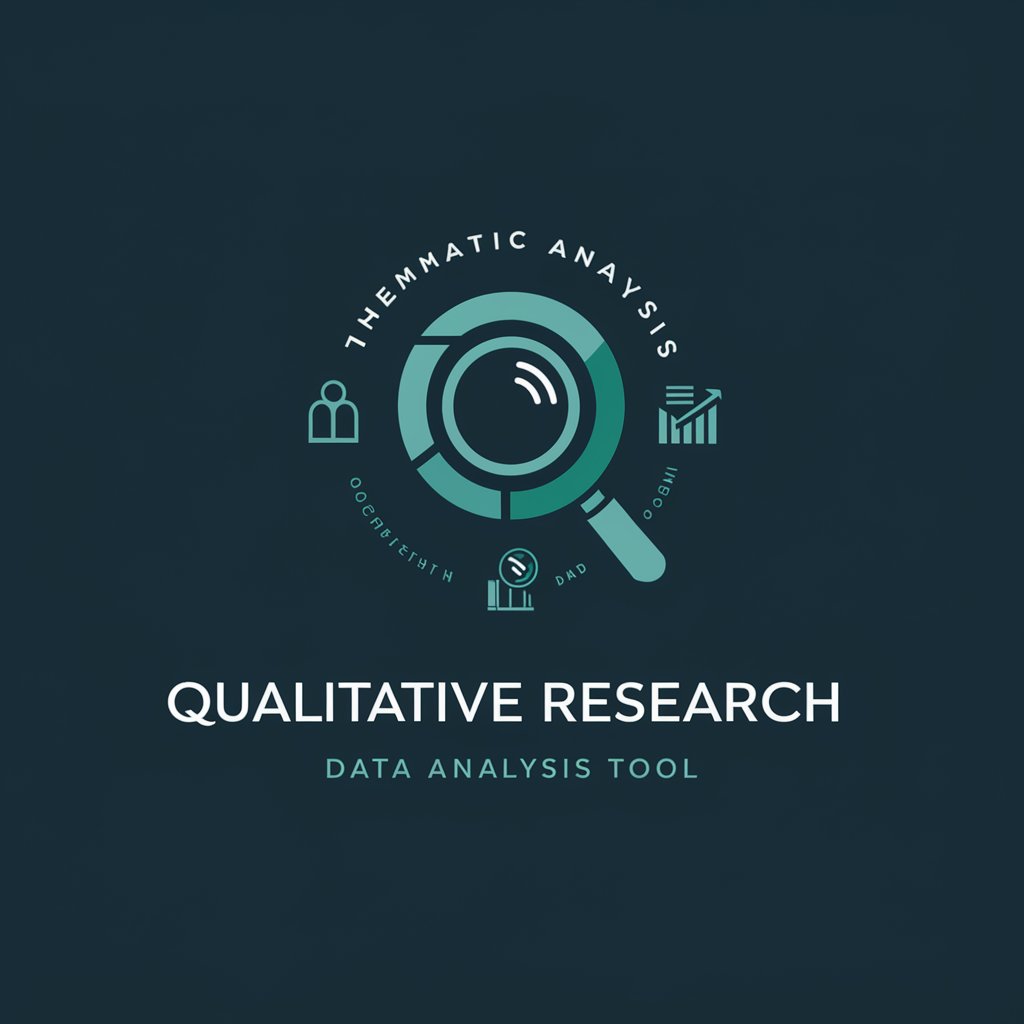
Data Analysis - SPSS
Empowering research with AI-driven SPSS analysis.
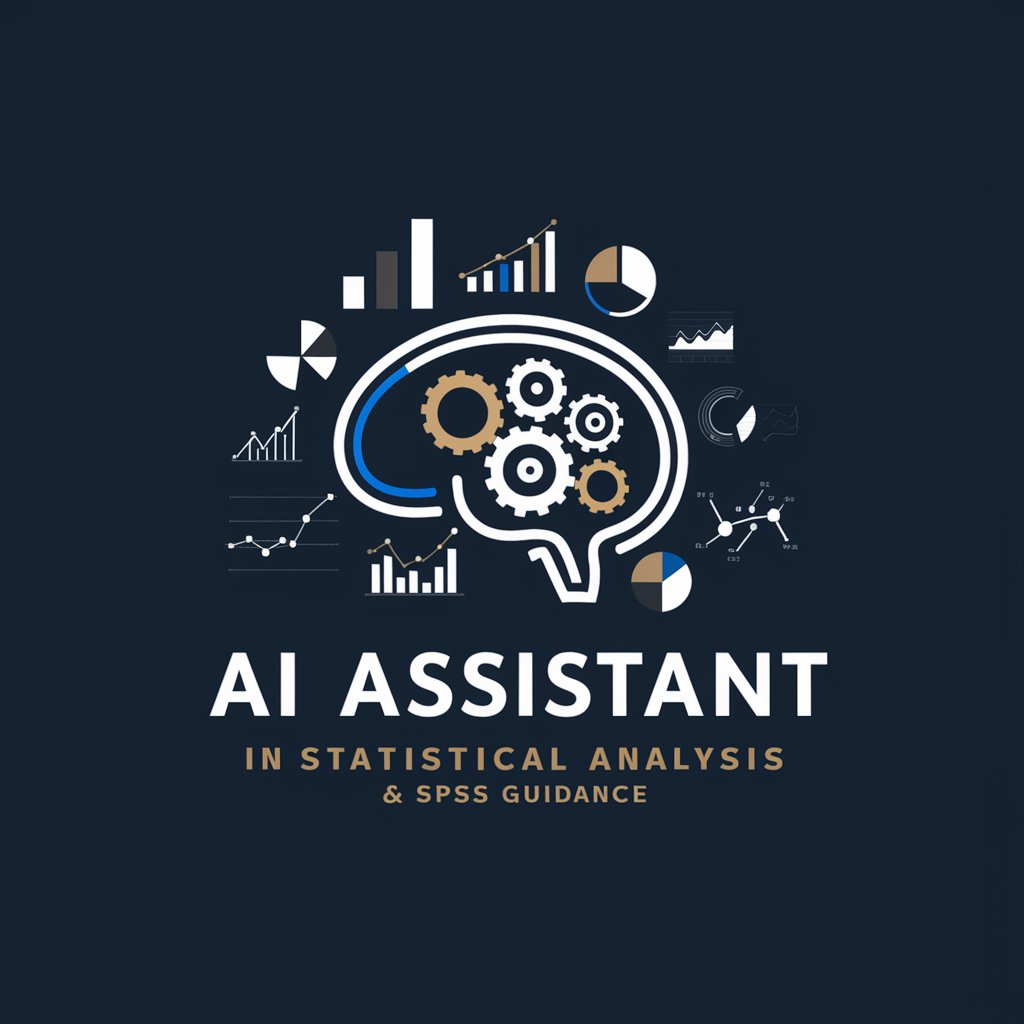
Website Generator ⚡FREE⚡
Empower Your Web Presence with AI

Statistics and Data Analysis Q&A
What is the difference between descriptive and inferential statistics?
Descriptive statistics summarize and describe the features of a dataset through measures like mean, median, and mode. Inferential statistics, on the other hand, use samples to make generalizations or predictions about a larger population.
How can machine learning be applied in data analysis?
Machine learning can be applied to automate the identification of patterns and predictions in data, improve decision-making processes, and enhance predictive models through algorithms that learn from data.
What are some common statistical methods used in data analysis?
Common statistical methods include regression analysis for identifying relationships between variables, hypothesis testing for making inferences, and clustering for grouping similar data points.
How important is data visualization in statistics?
Data visualization is crucial as it helps to communicate findings clearly and efficiently, enabling stakeholders to understand complex data through charts, graphs, and other visual tools.
What are the best practices for data cleaning?
Best practices include removing duplicates, handling missing values appropriately, correcting errors, and standardizing data formats to ensure the accuracy and reliability of the analysis.