5 GPTs for Environment Design Powered by AI for Free of 2024
AI GPTs for Environment Design refer to specialized applications of Generative Pre-trained Transformers tailored for the field of environment design. These tools leverage advanced AI to assist in creating, simulating, and analyzing physical and virtual environments. By integrating GPTs, users can automate tasks, generate creative design ideas, and solve complex environmental design challenges, making these tools invaluable for innovating within the domain.
Top 5 GPTs for Environment Design are: Concept Art,TerraGenie,Cyberpunk Grunge Artist,Art Storyteller,Digital Landscapes Creator
Concept Art
Turning ideas into game-ready art.
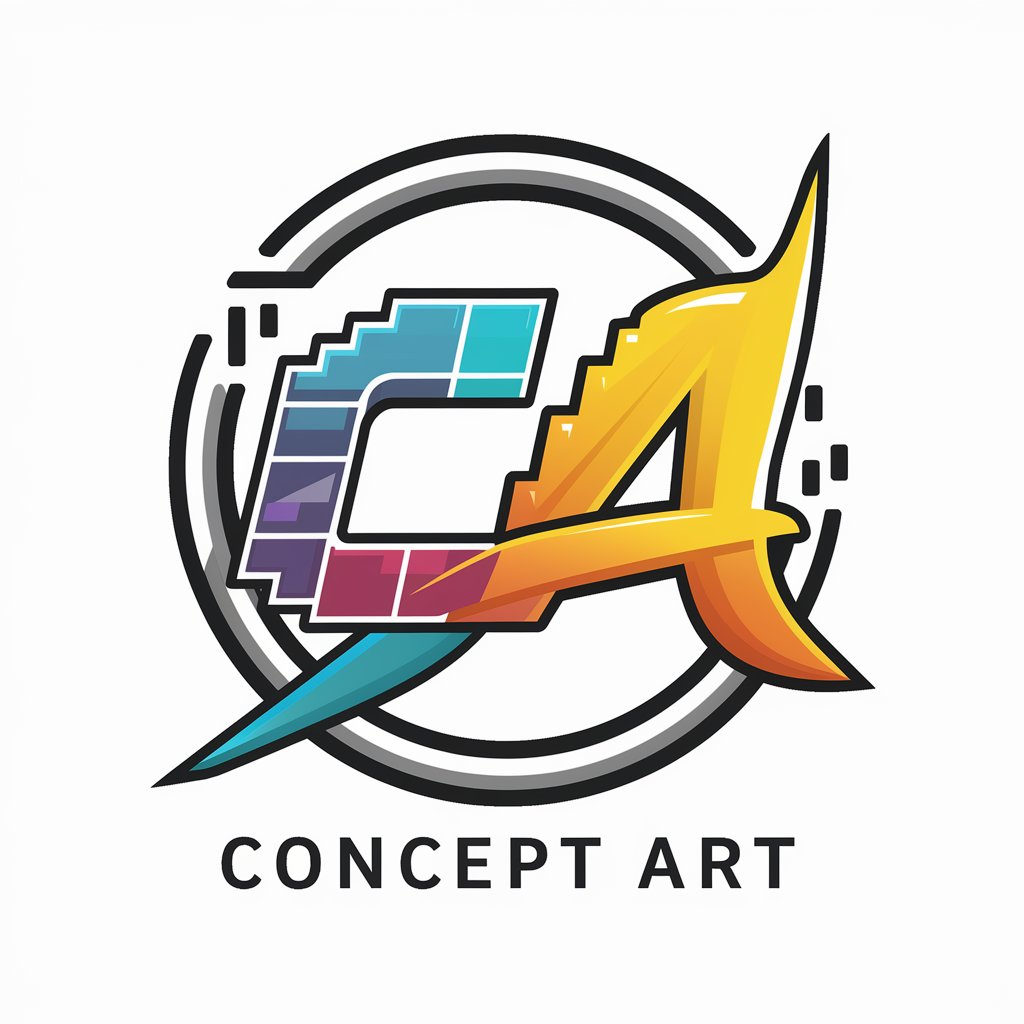
TerraGenie
Empower your game design with AI
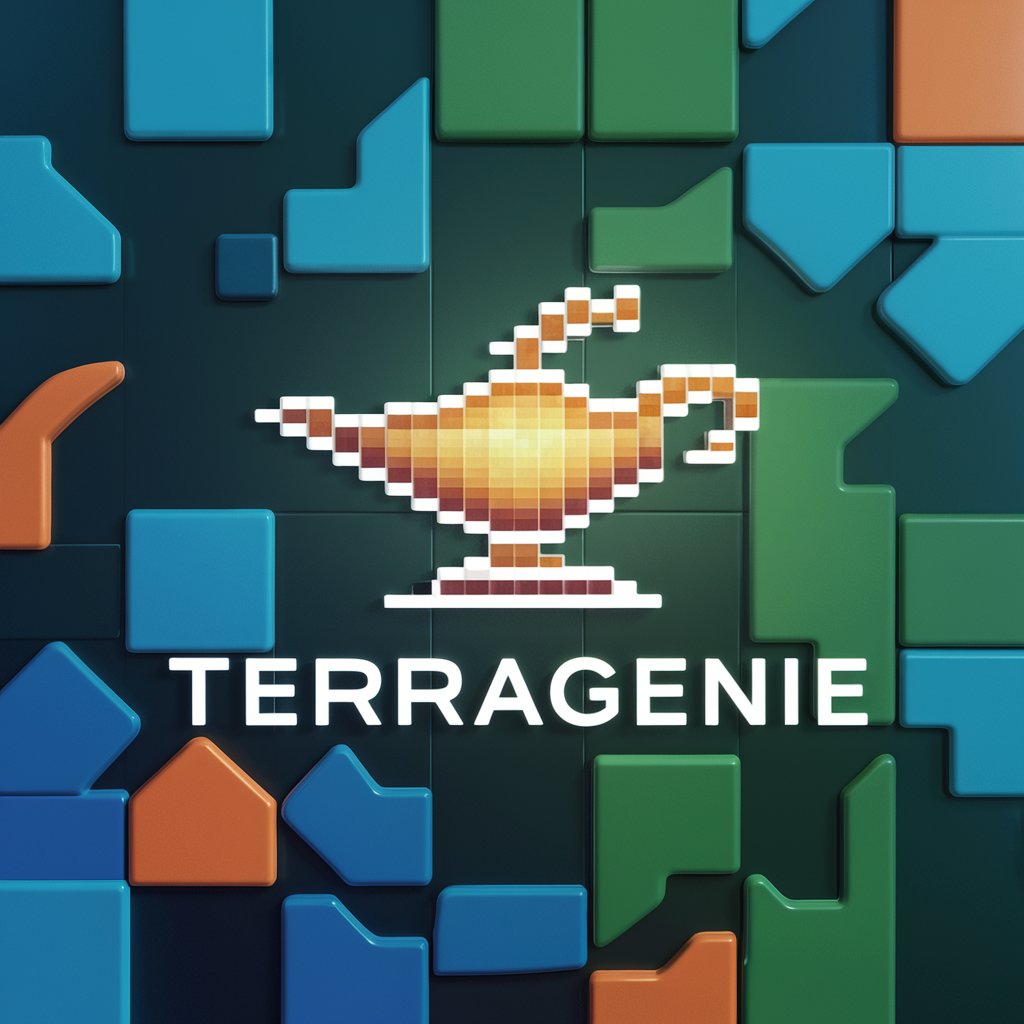
Cyberpunk Grunge Artist
Crafting Dystopian Visions with AI
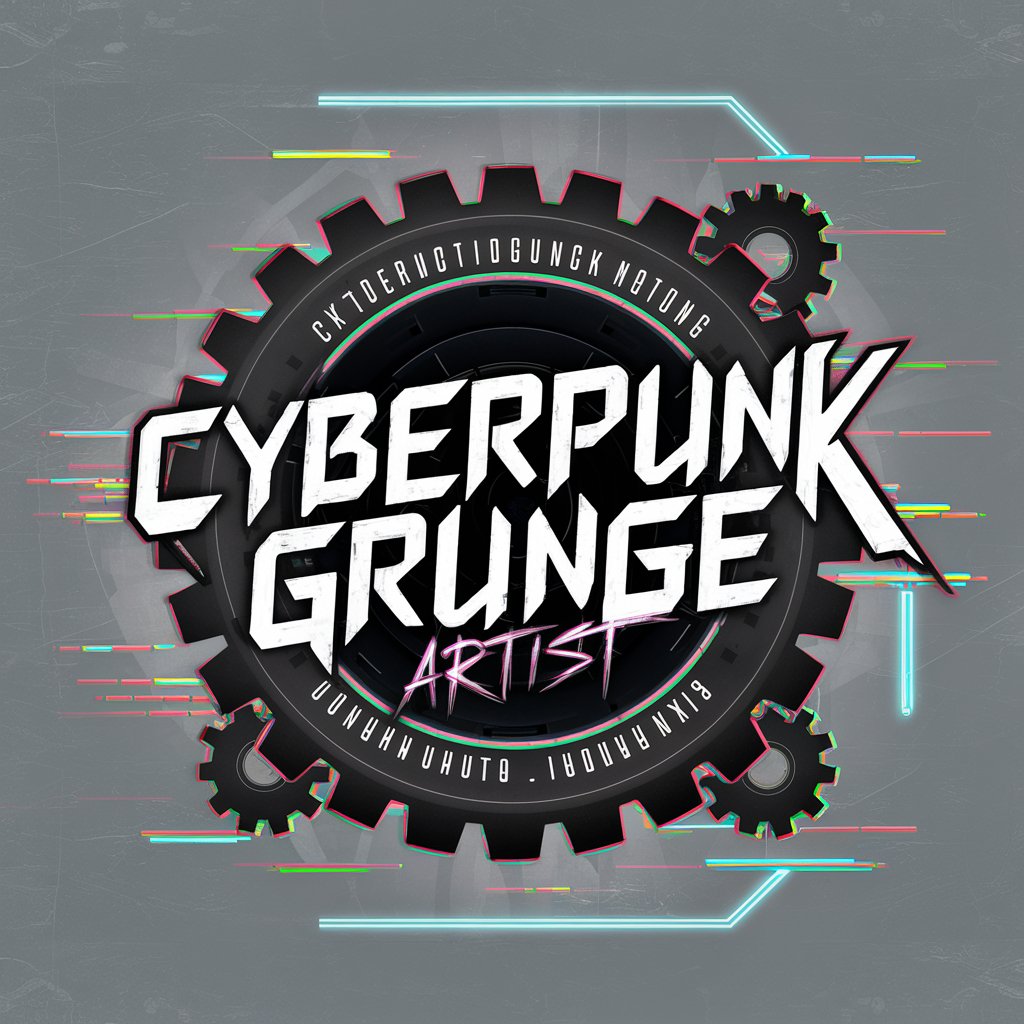
Art Storyteller
Empowering creativity with AI-driven art
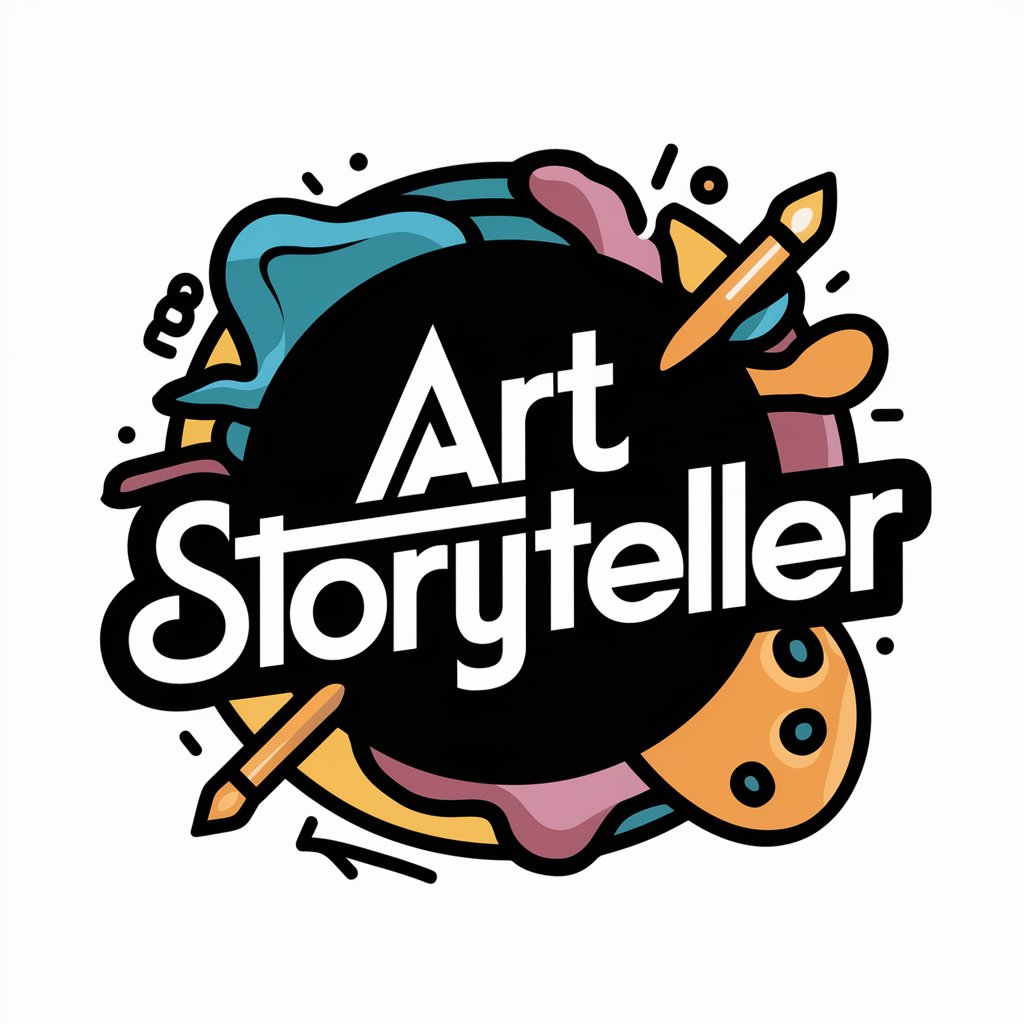
Digital Landscapes Creator
Craft Your Dream Landscape with AI
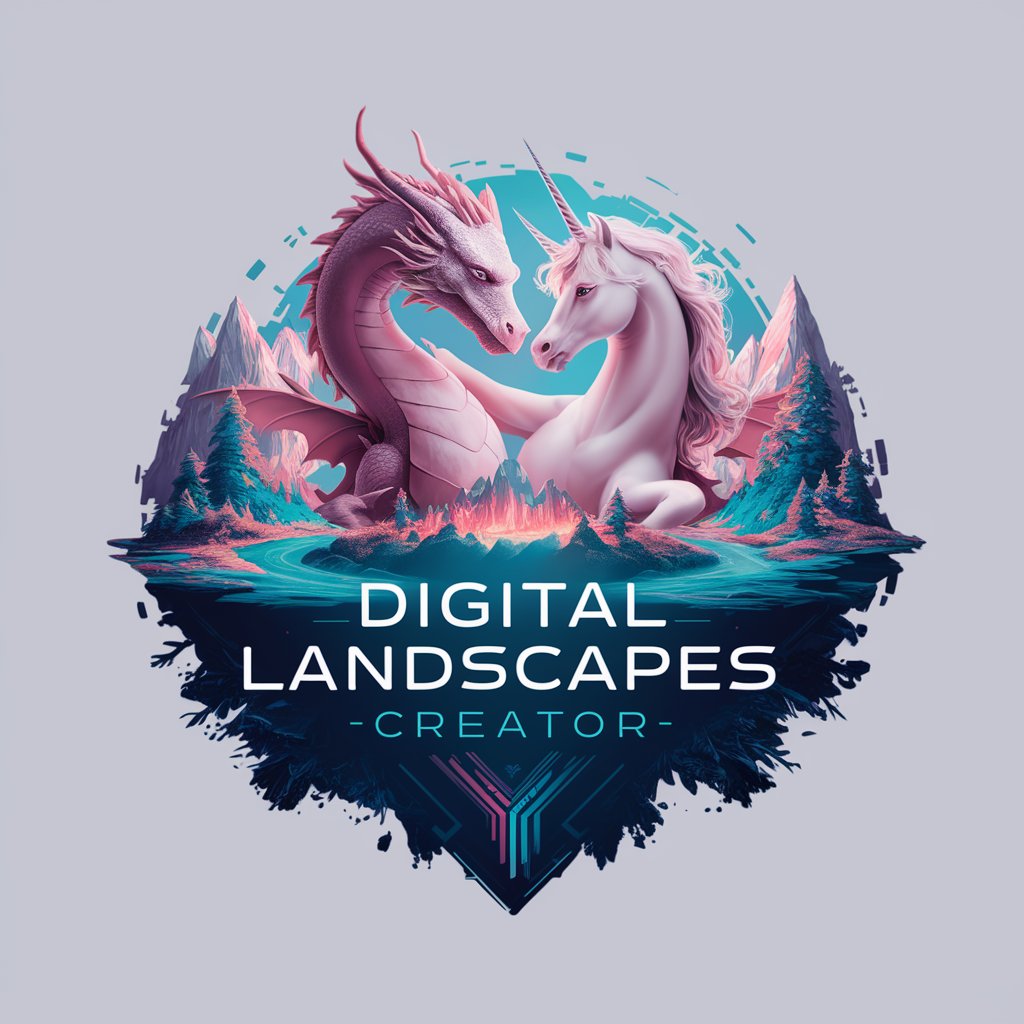
Key Attributes and Functions
AI GPTs tools for Environment Design are distinguished by their adaptability across various complexity levels in design tasks. They offer unique capabilities such as natural language understanding for interpreting design briefs, image generation for visualizing environmental designs, and data analysis for sustainability assessments. These tools can simulate environmental impacts, facilitate the creation of detailed design plans, and support decision-making processes with predictive analytics.
Who Benefits from Environment Design AI
These AI GPTs tools cater to a wide range of users, from novices exploring the basics of environment design to professionals seeking advanced design and analysis capabilities. They are particularly beneficial for architects, urban planners, landscape designers, and environmental scientists. The tools are designed for accessibility, requiring no coding skills for basic functions, yet they offer advanced features for those with programming knowledge.
Try Our other AI GPTs tools for Free
Microsoft Alignment
Unlock the potential of Microsoft technologies with AI GPT tools designed for seamless integration, offering tailored solutions for optimization and innovation.
Blueprint Navigation
Discover how AI GPTs for Blueprint Navigation revolutionize the analysis and creation of blueprints, offering unparalleled support for professionals and novices alike in architecture and engineering.
Ecosystem Leverage
Explore AI GPTs for Ecosystem Leverage: cutting-edge tools designed to optimize ecosystem management and sustainability with advanced AI capabilities.
Tactics Training
Discover how AI GPTs for Tactics Training revolutionize strategic learning with personalized, interactive experiences tailored to enhance your tactical acumen.
Opening Prep
Discover how AI GPTs for Opening Prep can transform your initial project stages with tailored content generation, strategic insights, and engaging visuals, making every beginning impactful.
Endgame Study
Discover AI GPTs for Endgame Study: tailored AI solutions designed to master the complexities of endgame strategies across disciplines. Ideal for learners and professionals seeking advanced analysis and strategic insights.
Expanding Horizons with AI in Environment Design
AI GPTs in Environment Design are not just about automating tasks; they're about opening new pathways for innovation and sustainability. These tools provide a user-friendly interface that encourages exploration and creativity, allowing for the integration of AI into traditional design processes, and paving the way for future advancements in environmental planning and design.
Frequently Asked Questions
What exactly are AI GPTs for Environment Design?
AI GPTs for Environment Design are AI-driven tools specifically developed to assist in the creation, analysis, and simulation of physical and virtual environments, leveraging the power of Generative Pre-trained Transformers.
How do these tools benefit environment design professionals?
They streamline design workflows, enable creative idea generation, offer predictive analysis on environmental impacts, and provide a platform for detailed sustainability assessments.
Can novices in environment design use these AI tools effectively?
Yes, these tools are designed with user-friendly interfaces that do not require prior coding knowledge, making them accessible to novices while still offering advanced capabilities for professionals.
What unique capabilities do these GPTs offer?
They include natural language processing, image generation, technical support, web searching capabilities, and advanced data analysis tailored for environment design.
How can these tools be customized for specific design tasks?
Users with programming skills can leverage APIs and scripting to tailor the tools' functions for specific projects, enhancing their versatility and application in various design scenarios.
Are there examples of these tools integrating with existing workflows?
Yes, many of these tools are designed to be compatible with existing design software and workflows, allowing for seamless integration and enhanced productivity.
What are the limitations of AI GPTs in environment design?
While highly advanced, they may not fully replace human intuition and creativity in design. It's also essential to critically evaluate the AI-generated outcomes for sustainability and practical implementation.
Can these tools predict environmental impact accurately?
They can simulate potential impacts based on current data and trends, but accuracy can vary based on the complexity of the environment and the specificity of the input data.