12 GPTs for Material Innovation Powered by AI for Free of 2025
AI GPTs for Material Innovation are advanced tools designed to leverage the power of Generative Pre-trained Transformers (GPTs) to foster innovation in materials science and engineering. These tools are tailored for analyzing, predicting, and generating insights about new materials, their properties, and their potential applications. By harnessing the vast computational capabilities of GPTs, they provide customized solutions that aid in the development of novel materials, optimize existing ones, and solve complex problems in the field of material science.
Top 10 GPTs for Material Innovation are: Industrial Design,Sustainable Plastics GPT ♻️,潮流匠心,Arquitecto Amigo,Non-Ferrous and Alloys Oracle,EcoPack Innovator,Building Materials,Global Heliogenesis University,Mr Material,Fabric Muse
Industrial Design
Empowering Design with AI
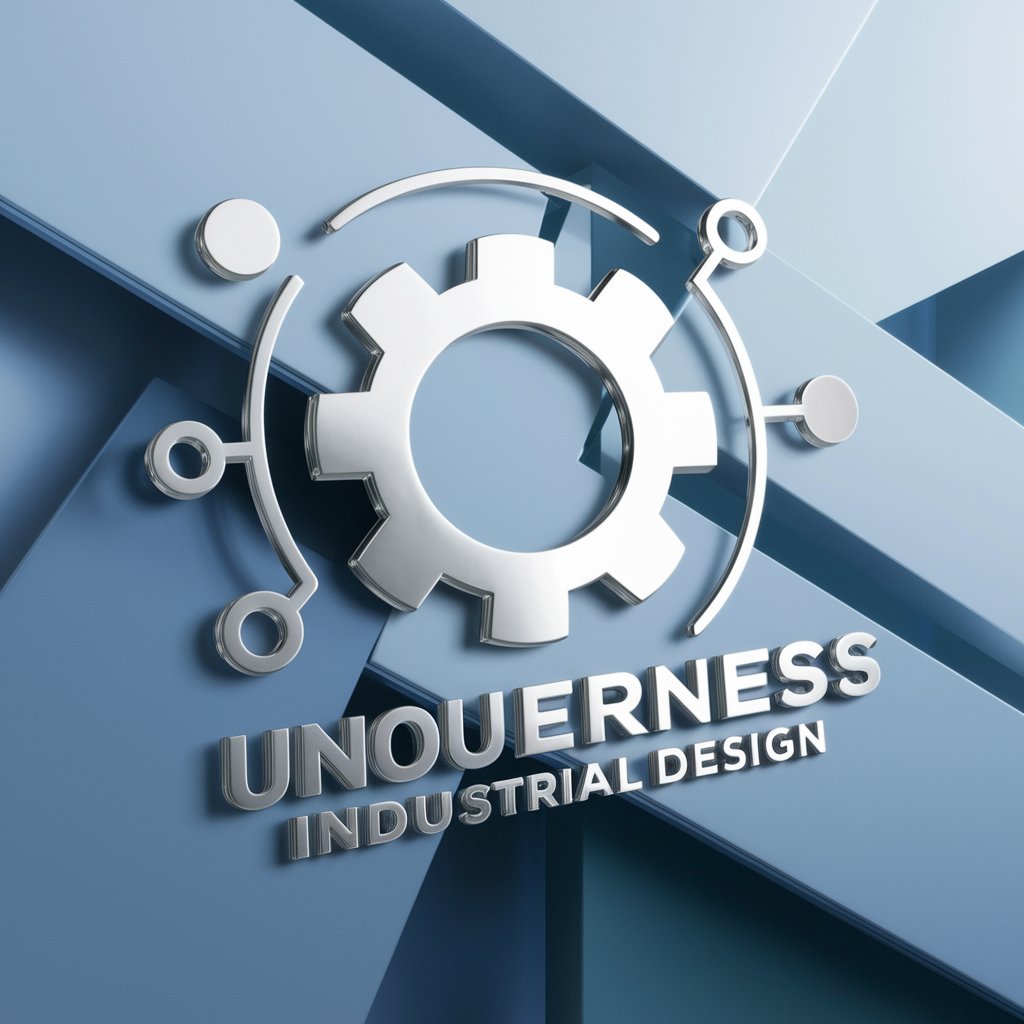
Sustainable Plastics GPT ♻️
Empowering eco-friendly innovation with AI.

潮流匠心
Empower Your Designs with AI

Arquitecto Amigo
Empowering architectural creativity with AI.
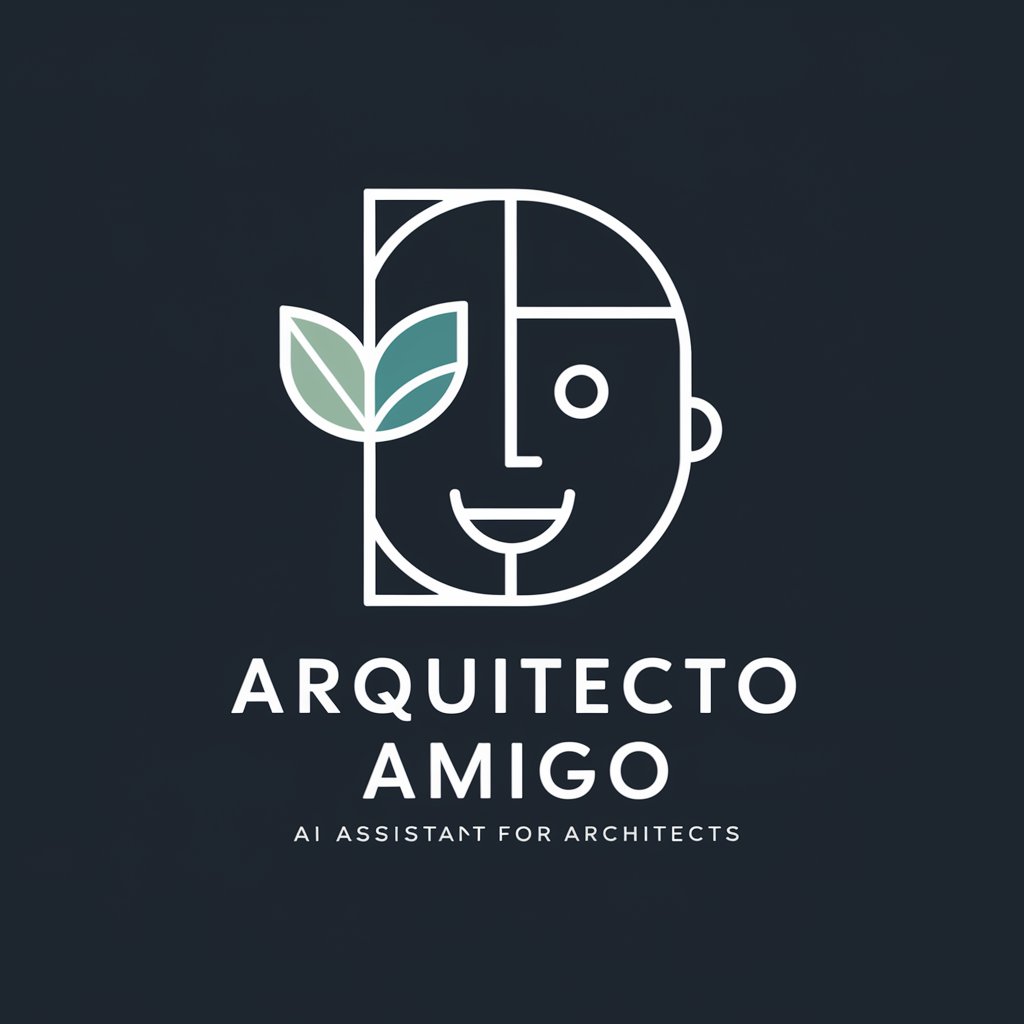
Non-Ferrous and Alloys Oracle
Empowering metallurgy with AI insights
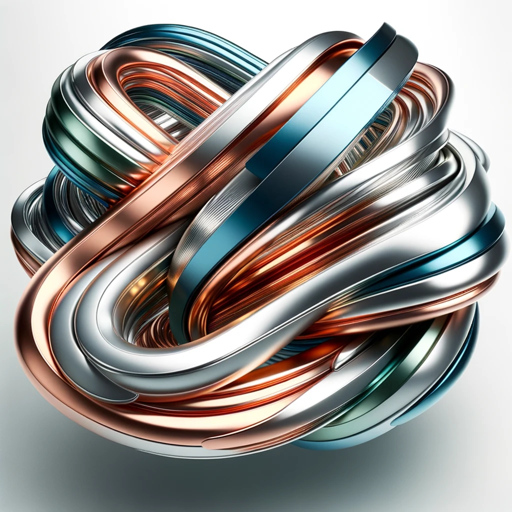
EcoPack Innovator
Innovate Sustainably with AI
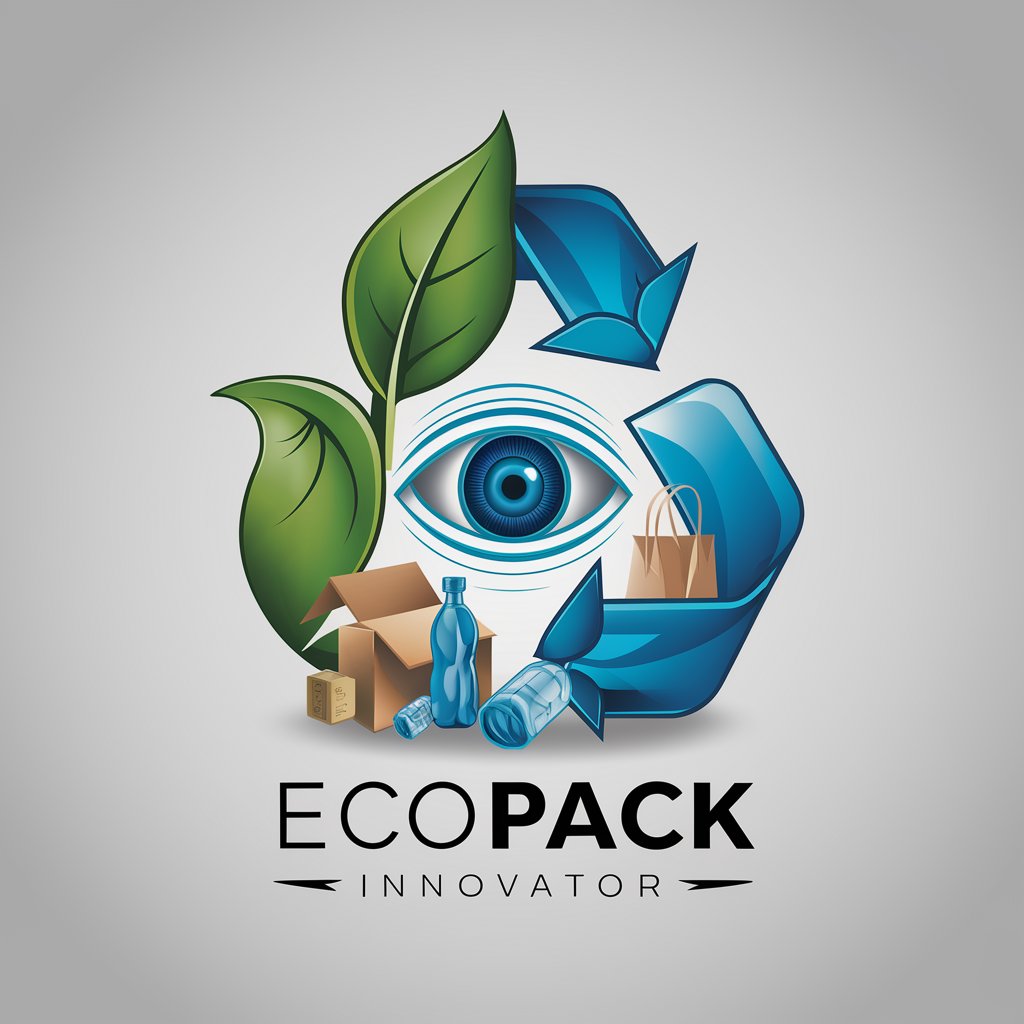
Building Materials
Empowering construction with AI-driven material insights
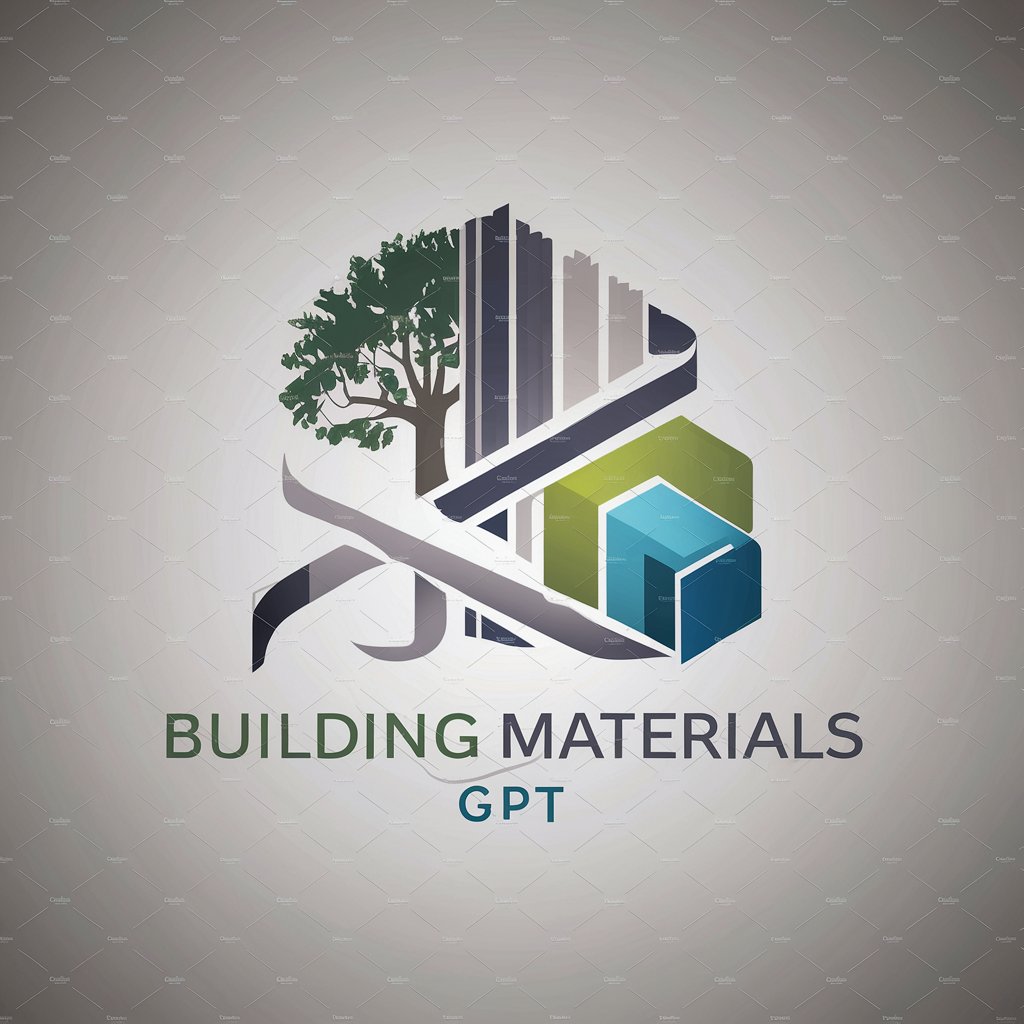
Global Heliogenesis University
Empowering sustainable construction with AI-driven insights.
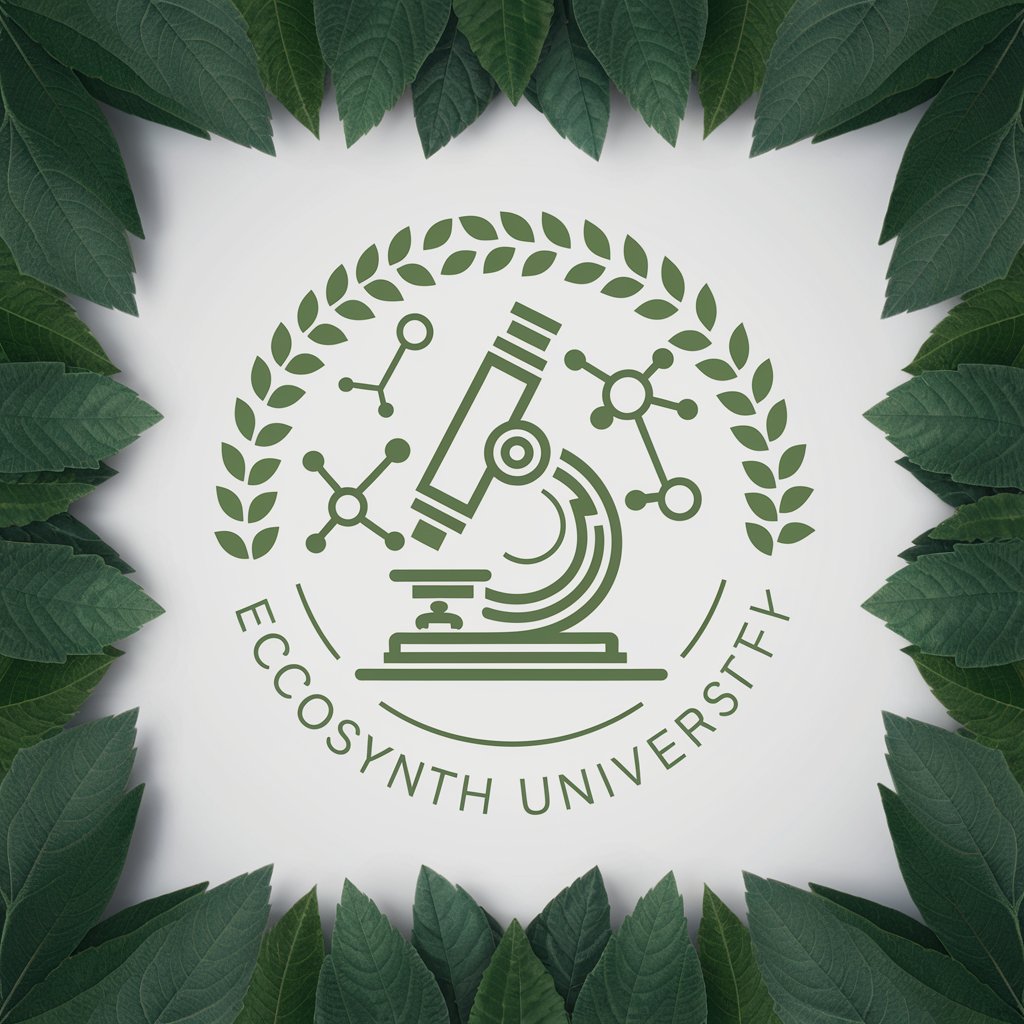
Mr Material
Empowering Material Decisions with AI
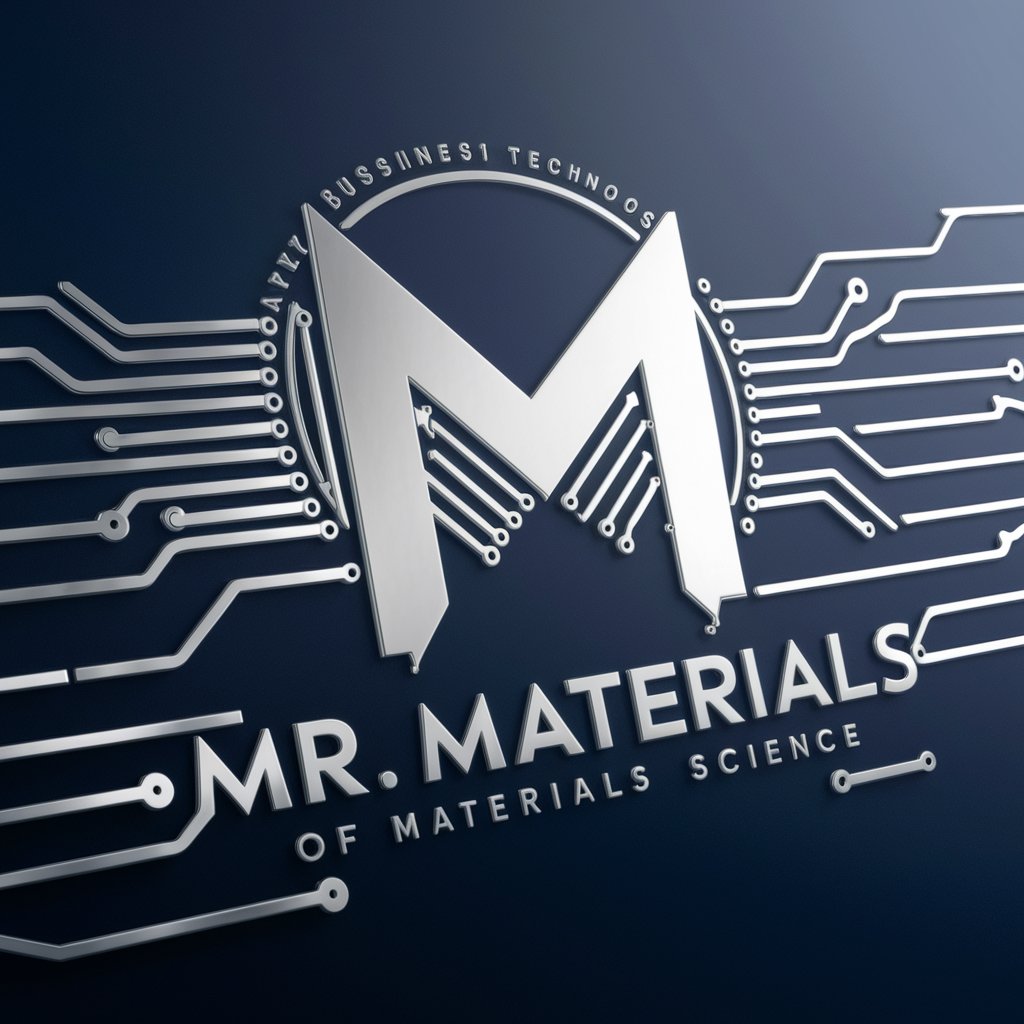
Fabric Muse
Crafting Creativity with AI
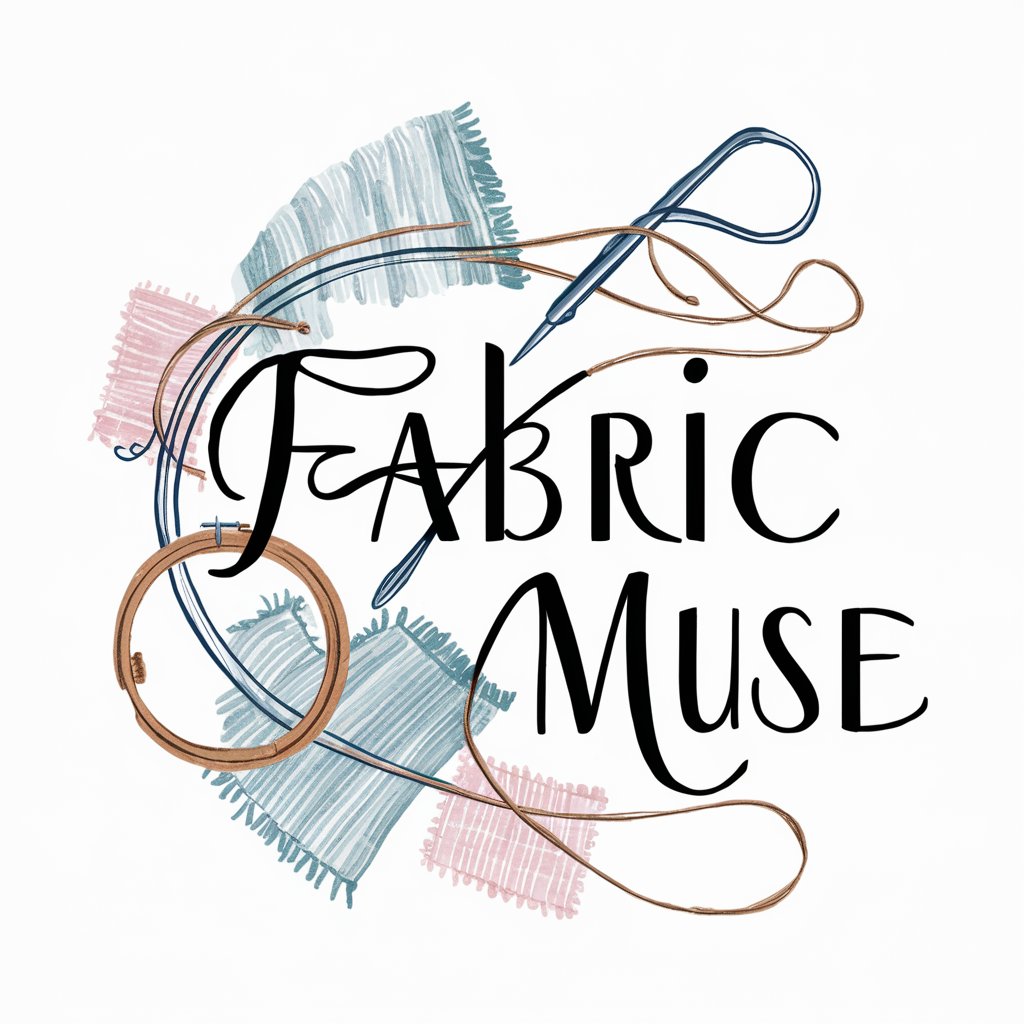
Insect-Based Biomimicry for Technology Innovator
Harnessing nature's genius with AI
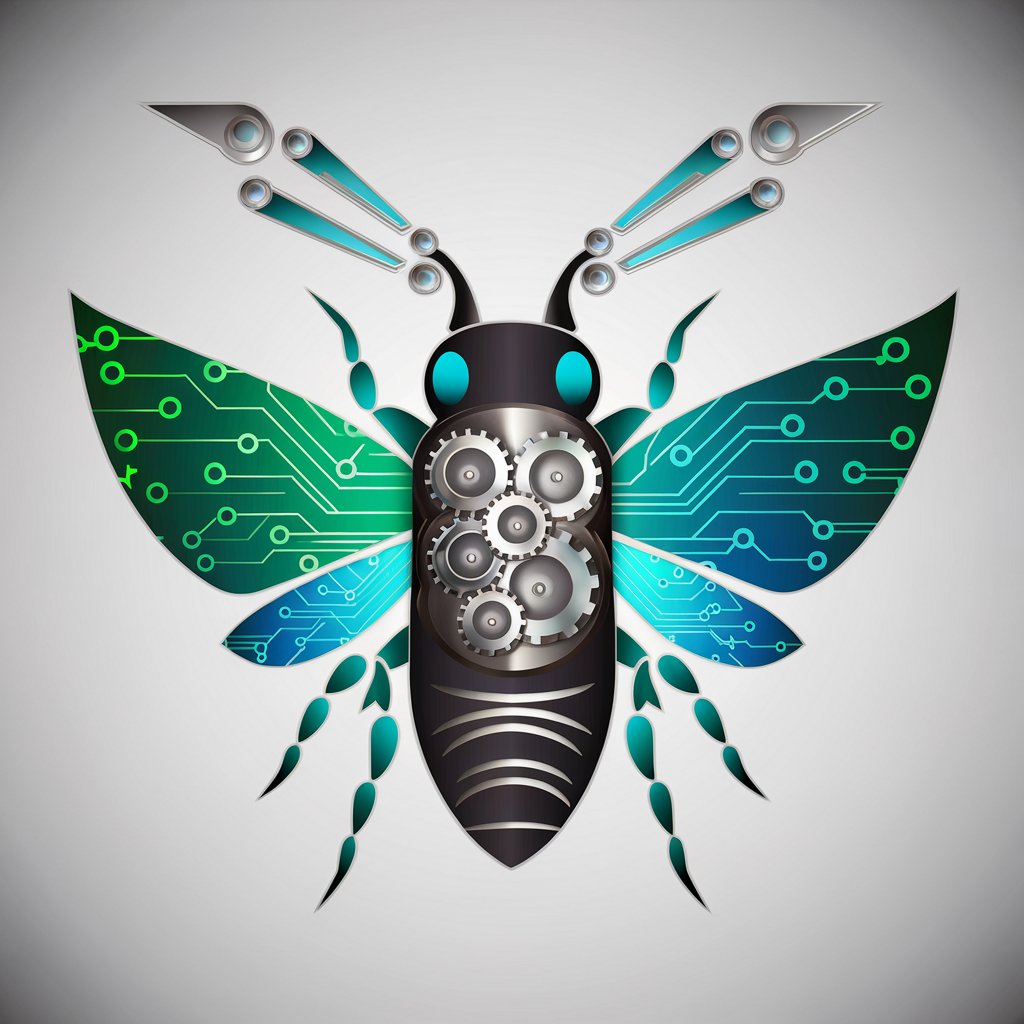
Universal Chemist (UCH)
Revolutionizing Chemistry with AI
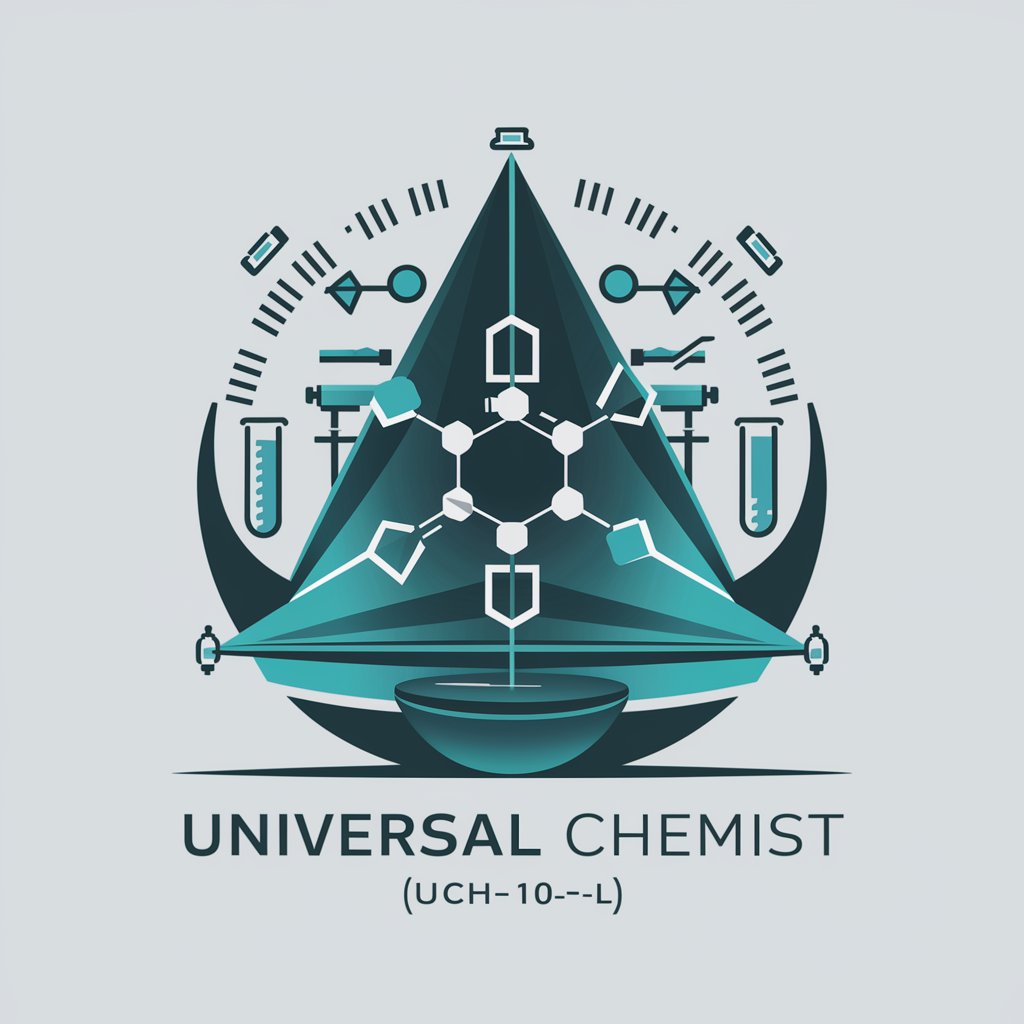
Key Attributes of AI GPTs in Material Innovation
These GPTs tools stand out due to their adaptability across various complexities within the material innovation domain, from straightforward property prediction to complex material behavior analysis under different conditions. Special features include advanced language understanding for technical documentation, web searching for the latest research, image generation for material structure visualization, and data analysis capabilities for identifying trends and patterns in material science data.
Who Benefits from Material Innovation AI Tools
The primary users of AI GPTs for Material Innovation range from novices with a budding interest in materials science to experienced professionals and developers in the field. These tools are designed to be accessible to users without programming skills, offering user-friendly interfaces and guided processes, while also providing extensive customization options for those with a deeper technical background.
Try Our other AI GPTs tools for Free
Legal Concepts
Revolutionize your legal practice with AI GPTs for Legal Concepts: automated research, document drafting, and insightful analytics tailored for the legal field.
Insurance Handling
Discover how AI GPTs transform insurance handling with automation, improved efficiency, and personalized services, making insurance processes seamless for companies and policyholders alike.
Project Strategizing
Discover how AI GPTs revolutionize project strategizing with tailored insights and strategic planning tools, designed for project managers and strategists seeking to optimize outcomes.
Conceptual Simplification
Discover how AI GPTs for Conceptual Simplification transform complex concepts into easy-to-understand language, making knowledge accessible to all.
Daily Decisions
Discover how AI GPTs for Daily Decisions can transform your decision-making with intelligent, personalized solutions tailored to your needs. Make informed choices effortlessly, every day.
Random Selection
Discover AI GPT tools for Random Selection, offering advanced solutions for generating random choices with fairness and flexibility. Ideal for professionals and novices alike.
Further Perspectives on AI-Driven Material Innovation
AI GPTs for Material Innovation stand at the forefront of technological advancement, offering user-friendly interfaces that simplify complex material science concepts. Their ability to integrate with existing systems enables a streamlined approach to material research and development, making them invaluable tools for accelerating innovation in various sectors.
Frequently Asked Questions
What exactly are AI GPTs for Material Innovation?
AI GPTs for Material Innovation are specialized versions of Generative Pre-trained Transformers adapted for tasks in materials science, aiding in discovery, analysis, and prediction of material properties.
Who can use these AI GPTs tools?
They are designed for a wide range of users, from students and educators in materials science to research scientists and industrial professionals looking for innovative material solutions.
Do I need coding skills to use these tools?
No, these tools are developed with interfaces that are accessible to users without coding expertise, though programming skills can enhance customization and functionality.
How can AI GPTs contribute to material innovation?
They accelerate the research and development process by providing quick access to material data, predicting material properties, generating innovative material combinations, and offering insights into new material applications.
Can these tools predict material properties?
Yes, one of the core capabilities includes the prediction of physical, chemical, and mechanical properties of materials using advanced algorithms and data analysis.
Are these tools capable of visualizing material structures?
Yes, they incorporate image generation features that can visualize molecular structures, material compositions, and potential defects within materials.
How do these tools stay updated with the latest research?
AI GPTs for Material Innovation often include web searching capabilities that scour scientific databases and journals for the latest findings and integrate them into their knowledge base.
Can these tools integrate with existing workflows?
Yes, they are designed for easy integration with existing material science research and development workflows, allowing for seamless collaboration and data exchange.