5 GPTs for Material Optimization Powered by AI for Free of 2024
AI GPTs for Material Optimization refers to the specialized use of Generative Pre-trained Transformers (GPTs) in the field of materials science and engineering. These advanced AI tools are designed to assist in the optimization of materials for various applications, leveraging vast datasets to predict material properties, identify novel materials, and suggest improvements for existing materials. By incorporating GPTs, stakeholders can harness the power of artificial intelligence to accelerate material discovery and development, enhance performance, reduce costs, and minimize environmental impact.
Top 5 GPTs for Material Optimization are: Architecture,2D Cutting Stock,Craft Buddy,SprayTechGPT,Steel Structure Worker
Architecture
Design Smarter with AI-Powered Architecture
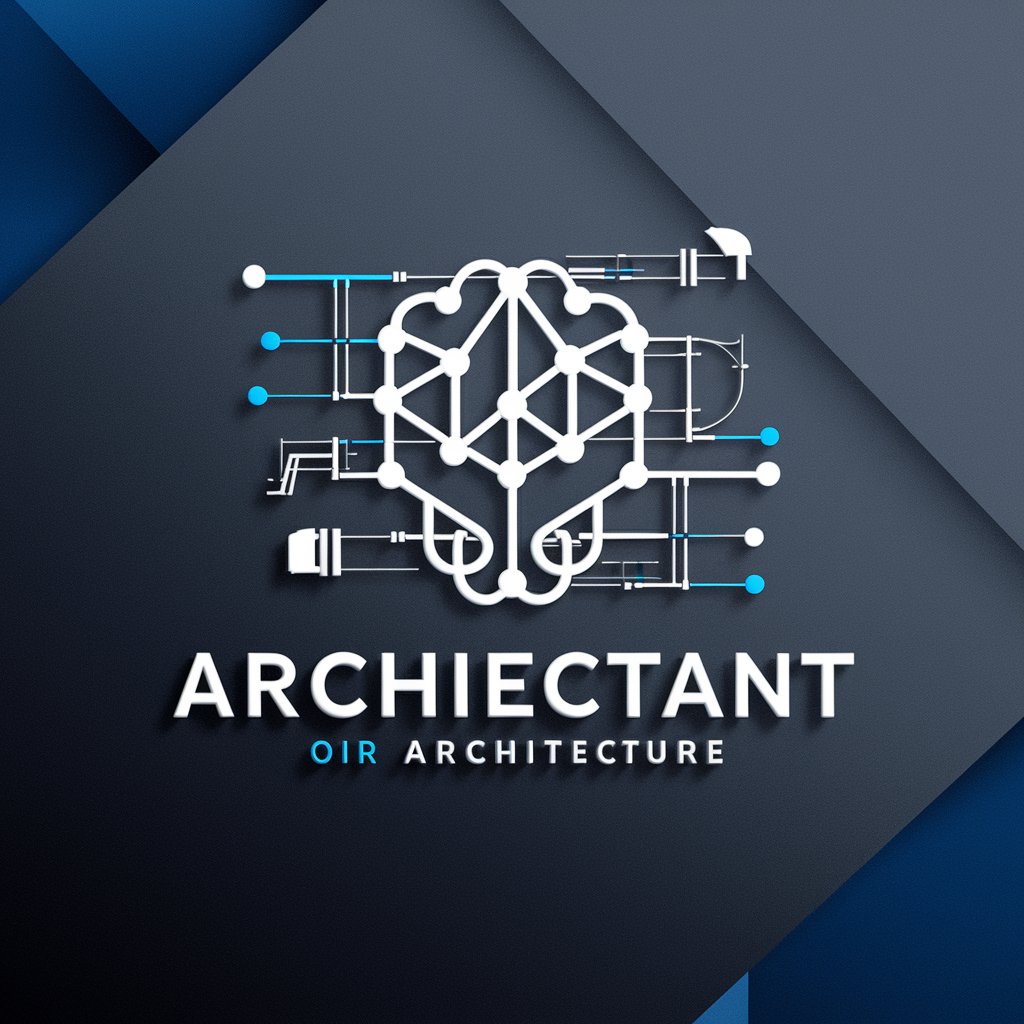
2D Cutting Stock
Optimize, Cut, Save - Powered by AI

Craft Buddy
Empowering Creativity with AI
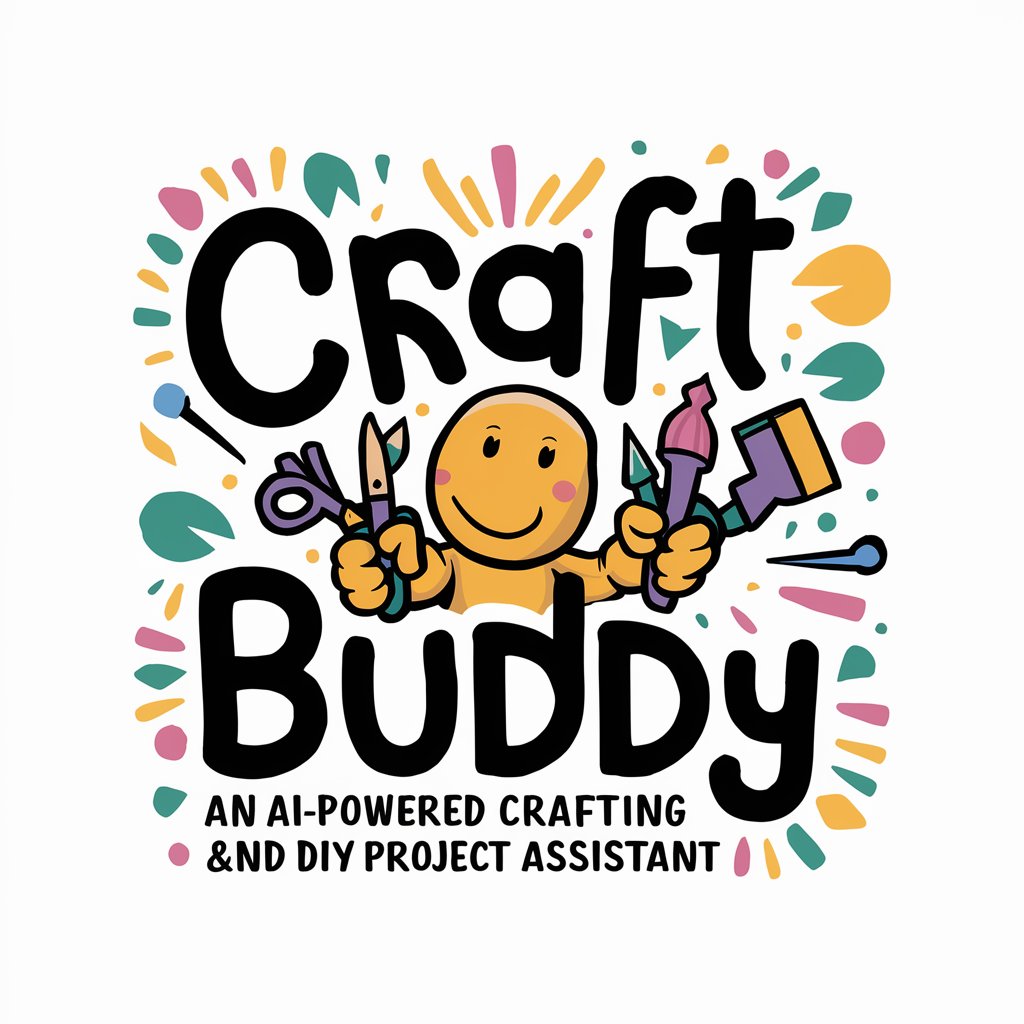
SprayTechGPT
Optimize industrial spraying with AI precision.
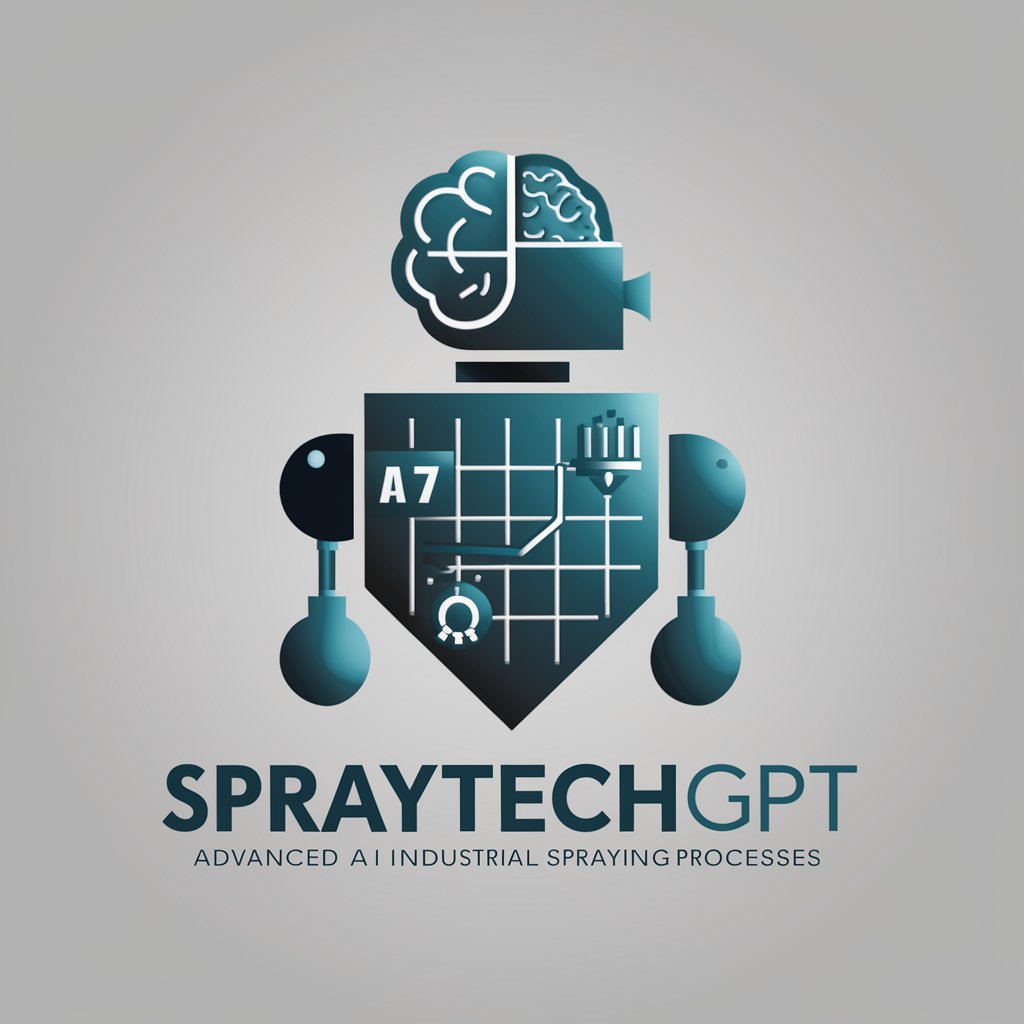
Steel Structure Worker
Revolutionizing steel fabrication with AI
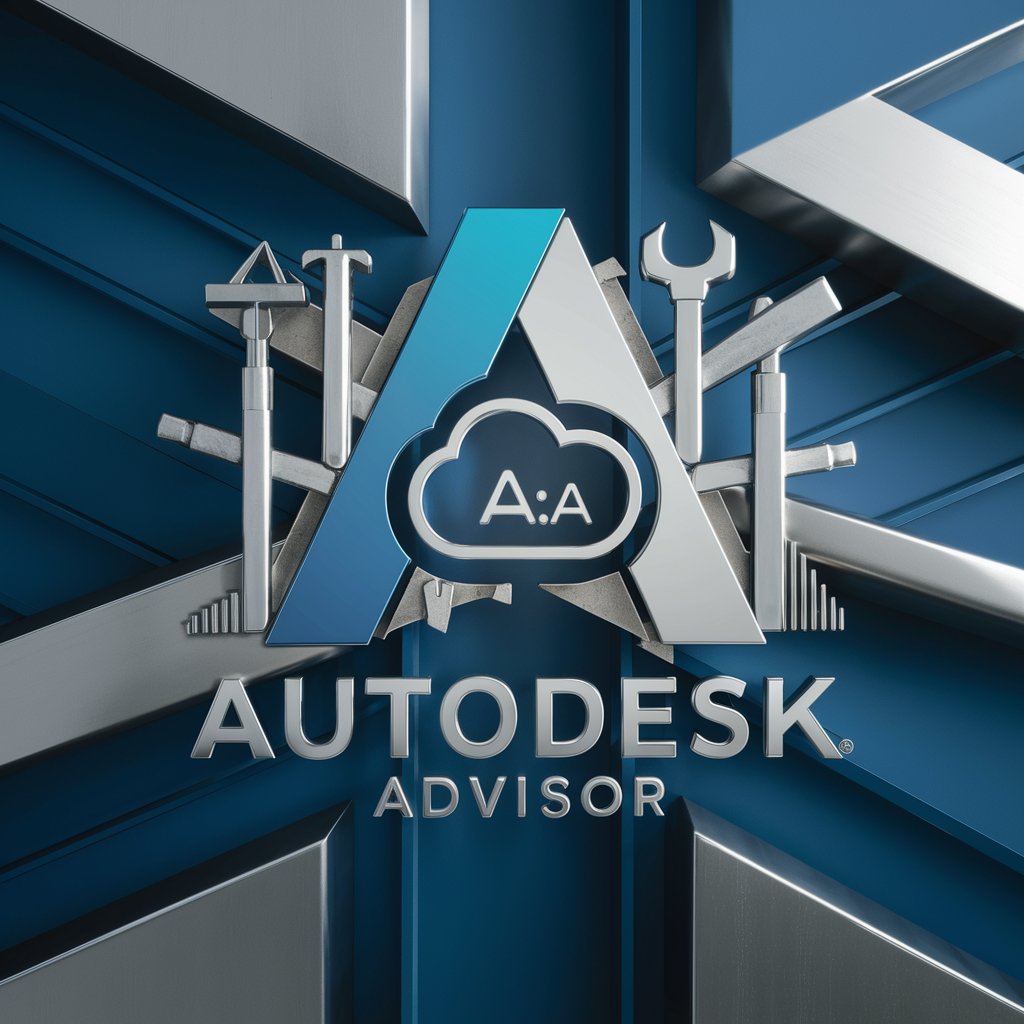
Key Attributes of Material Optimization GPTs
The core features of AI GPTs for Material Optimization include their adaptability to a wide range of material science tasks, from predicting material properties to generating novel material compositions. These tools can analyze complex datasets, recognize patterns, and provide insights that would be difficult or time-consuming to obtain manually. Special features may include natural language processing for interpreting technical documents, web searching for the latest research, image creation for visualizing molecular structures, and data analysis capabilities for identifying trends and optimizing material formulations.
Who Benefits from Material Optimization GPTs?
The primary users of AI GPTs for Material Optimization include researchers, engineers, and professionals working in material science, chemistry, and related fields. These tools are also invaluable for educators, students, and novices seeking to deepen their understanding of material properties and design principles. With user-friendly interfaces, they cater to individuals without programming expertise, while offering advanced customization options for developers and technical users to tailor solutions to specific research needs.
Try Our other AI GPTs tools for Free
Feature Requests
Discover how AI GPTs for Feature Requests can revolutionize your product development process with advanced AI capabilities, making innovation faster and more efficient.
Code Scanning
Discover how AI GPTs for Code Scanning revolutionize code quality and security with real-time analysis, automation, and adaptability across programming languages.
Threat Monitoring
Discover how AI GPTs revolutionize Threat Monitoring with real-time detection, analysis, and response to cyber threats. Tailored for both novices and professionals, these tools integrate seamlessly into existing systems, offering advanced protection.
VR Exploration
Discover how AI GPTs are revolutionizing VR Exploration, providing immersive, interactive experiences with real-time content creation and personalized narratives.
AR Navigation
Discover how AI GPTs revolutionize AR Navigation with real-time, personalized guidance. Enhancing your journey with interactive routes and points of interest.
Home Financing
Discover how AI GPTs for Home Financing simplify the mortgage process with personalized advice, loan comparisons, and financial planning, making home buying accessible to all.
Expanding Horizons with GPTs in Material Science
AI GPTs for Material Optimization represent a paradigm shift in materials science, offering customized solutions across sectors. They enhance the ability to discover and design advanced materials, with interfaces that cater to both novice and expert users. Their integration into existing workflows can significantly accelerate research and development cycles, making them a cornerstone of innovative material science research.
Frequently Asked Questions
What exactly are AI GPTs for Material Optimization?
They are AI tools designed to assist in the selection, design, and optimization of materials using data-driven insights and predictions.
How do these GPTs improve material development processes?
By analyzing vast amounts of data, they can identify optimal material compositions, predict properties, and suggest enhancements, speeding up innovation and reducing costs.
Can non-technical users operate these AI tools effectively?
Yes, these tools are designed with intuitive interfaces, making them accessible to non-technical users while still offering depth for experts.
Are there customization options for researchers with coding skills?
Absolutely, many GPTs offer APIs and programming interfaces that allow users to tailor functionalities to specific research questions.
What types of material optimization can these GPTs perform?
They can assist with a variety of tasks, including property prediction, material selection, and formulation optimization, across numerous industries.
How do these tools integrate with existing research workflows?
They are designed to be flexible and can be integrated into current systems through APIs or as standalone tools, complementing traditional research methods.
Can AI GPTs for Material Optimization predict new materials?
Yes, by leveraging machine learning algorithms and vast datasets, they can suggest novel material compositions that haven't been previously considered.
What are the limitations of these AI tools?
While powerful, they rely on the quality and quantity of available data and may not always capture the complexity of material interactions in novel environments.