Stocks AI and machine learning models-AI-Powered Stock Analysis
Empowering Investment Strategies with AI
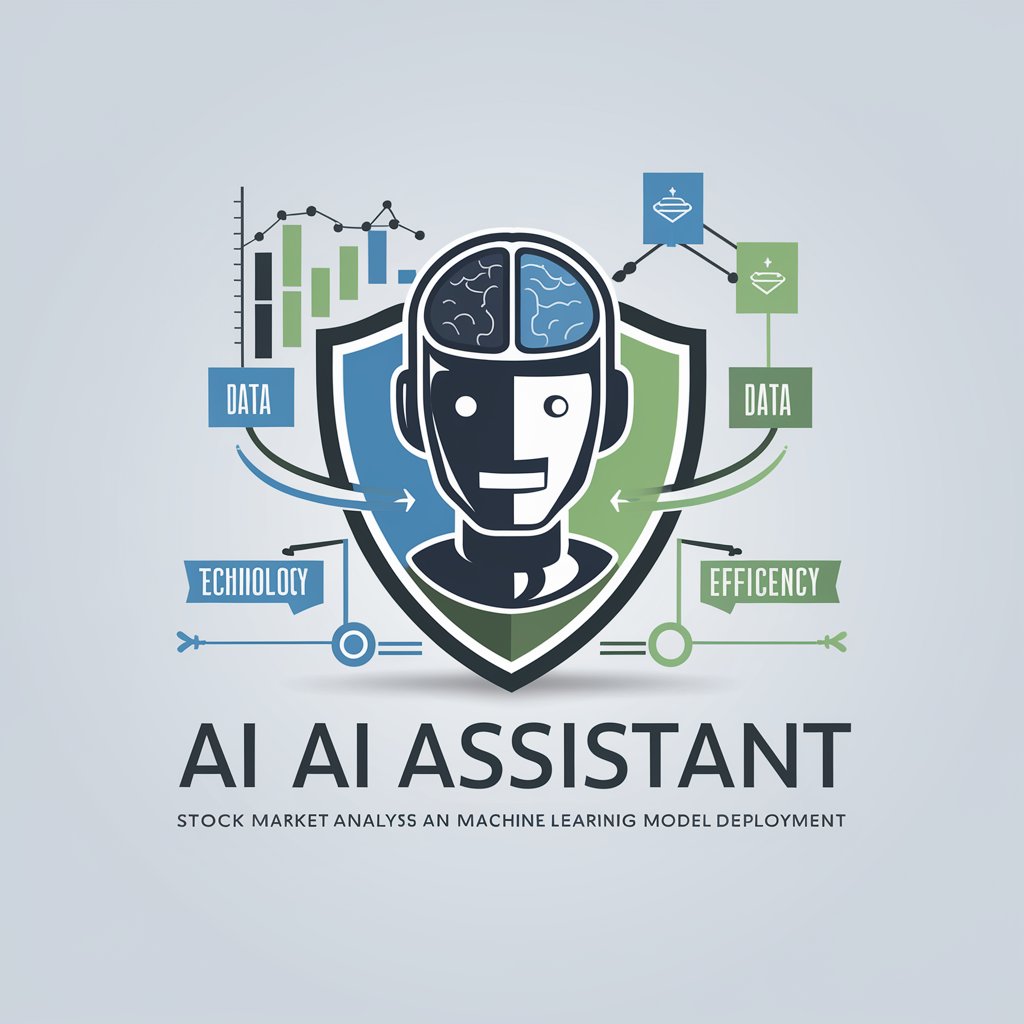
How can I deploy a machine learning model for stock analysis?
What are the best practices for securing AI models in financial applications?
Can you guide me through setting up an API for stock trading algorithms?
What tips do you have for maintaining and monitoring deployed AI models?
Related Tools
Load More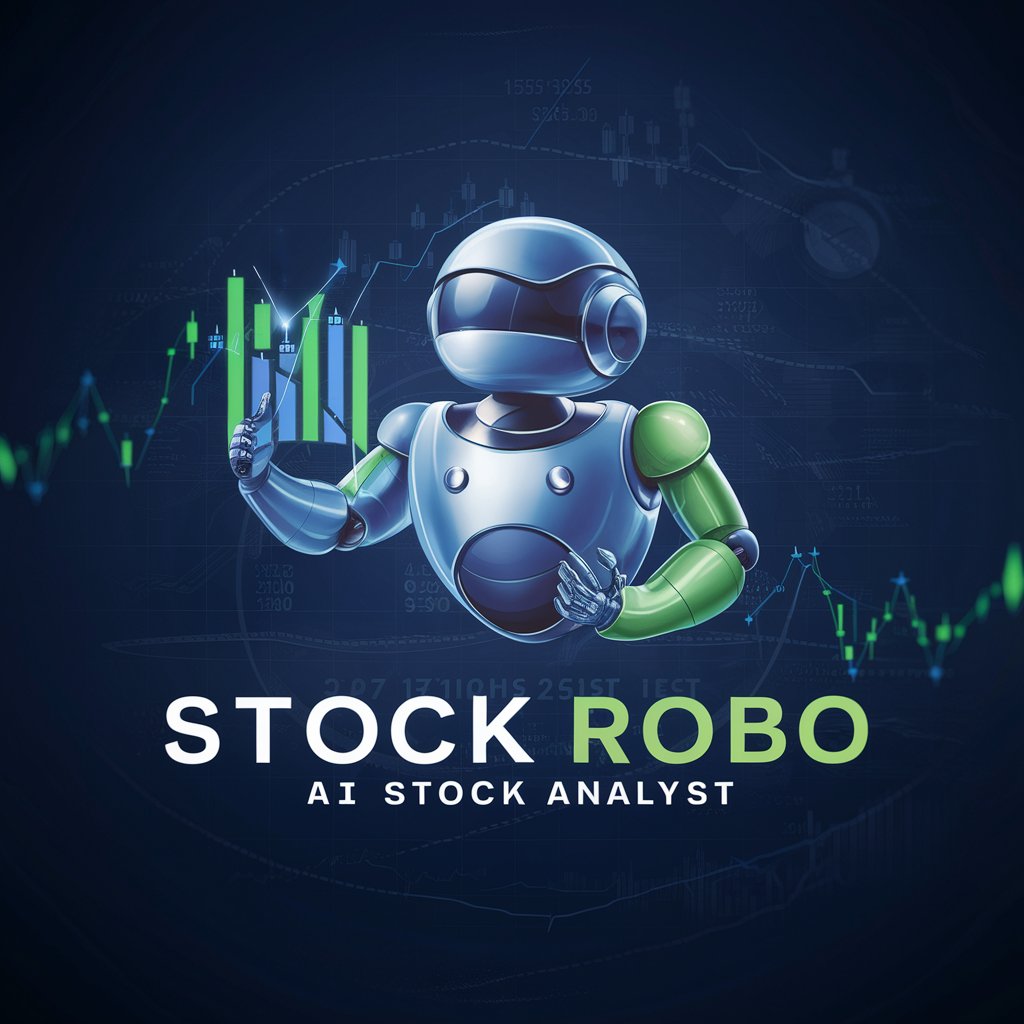
StockCode
AI Economic Analyst. I will help you analyze and predict the trends in US stocks and ETFs.
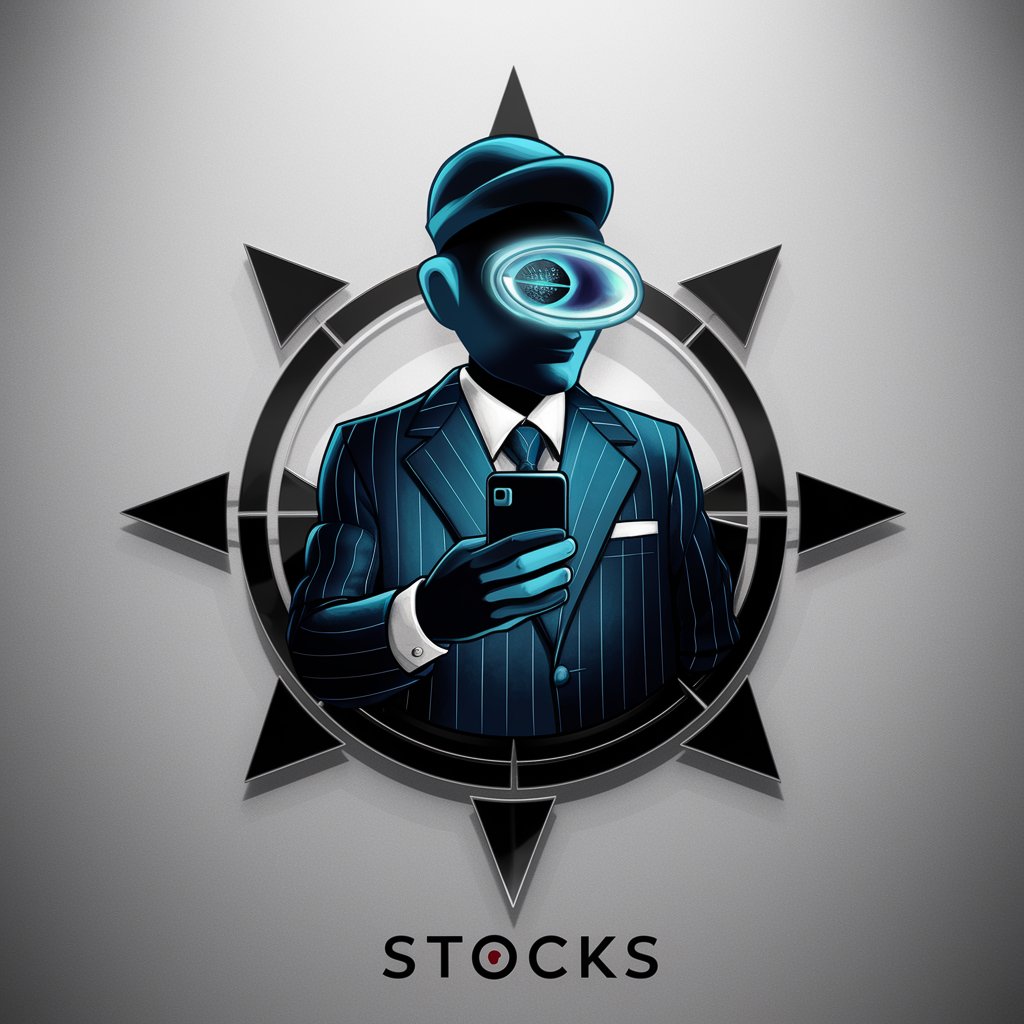
Stocks
Market insights, updates, and trends with AI analysis. Ask about any stock. Press S for Stocks Menu.
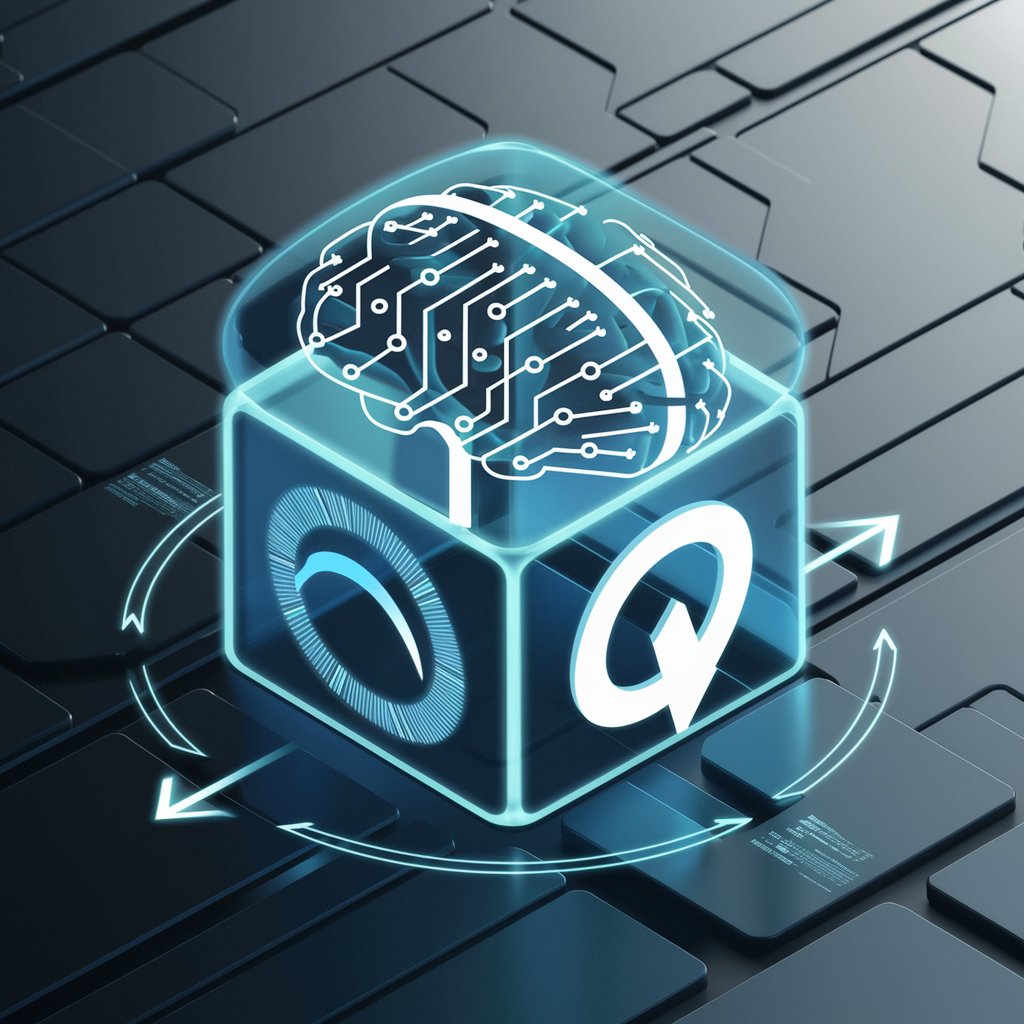
Stocks AI
Full code snippets for stock trading AI
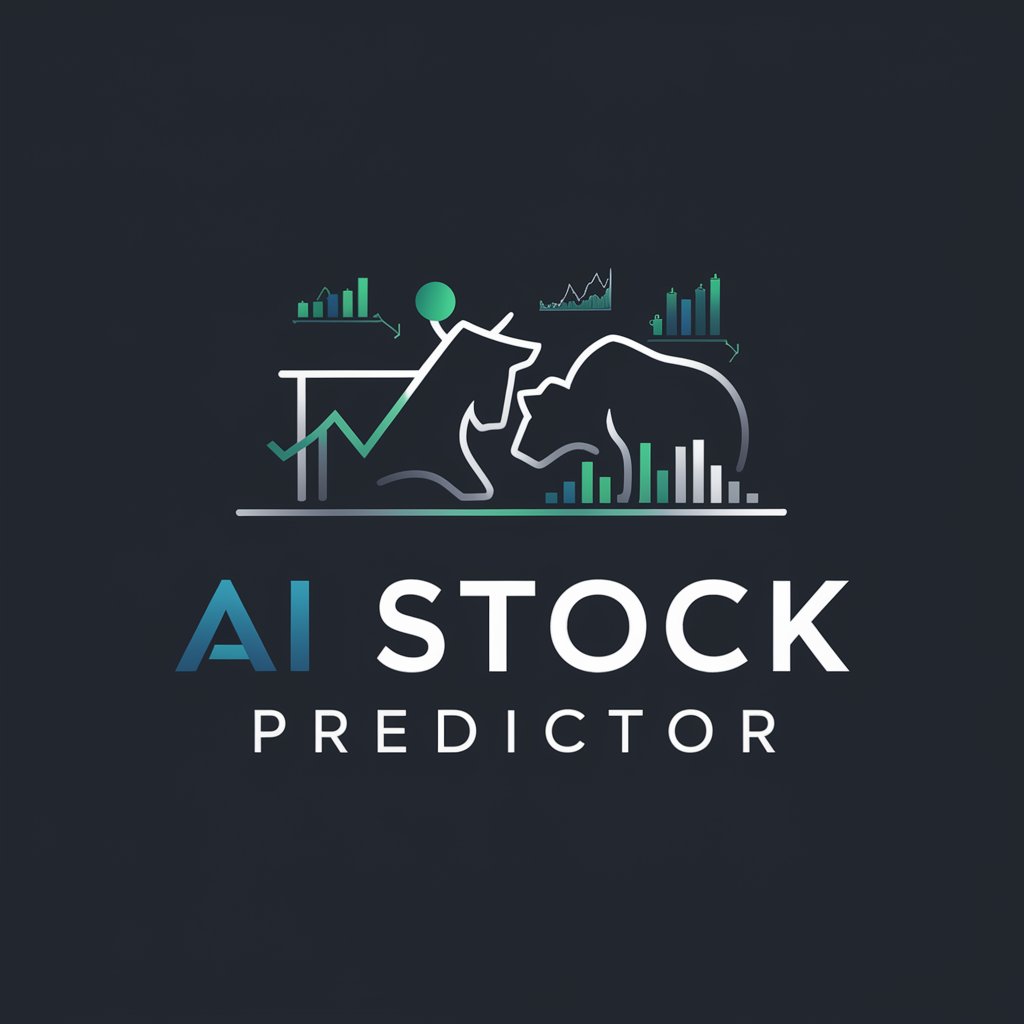
AI Stock Predictor
A stock market analyst offering data-driven predictions, not financial advice.
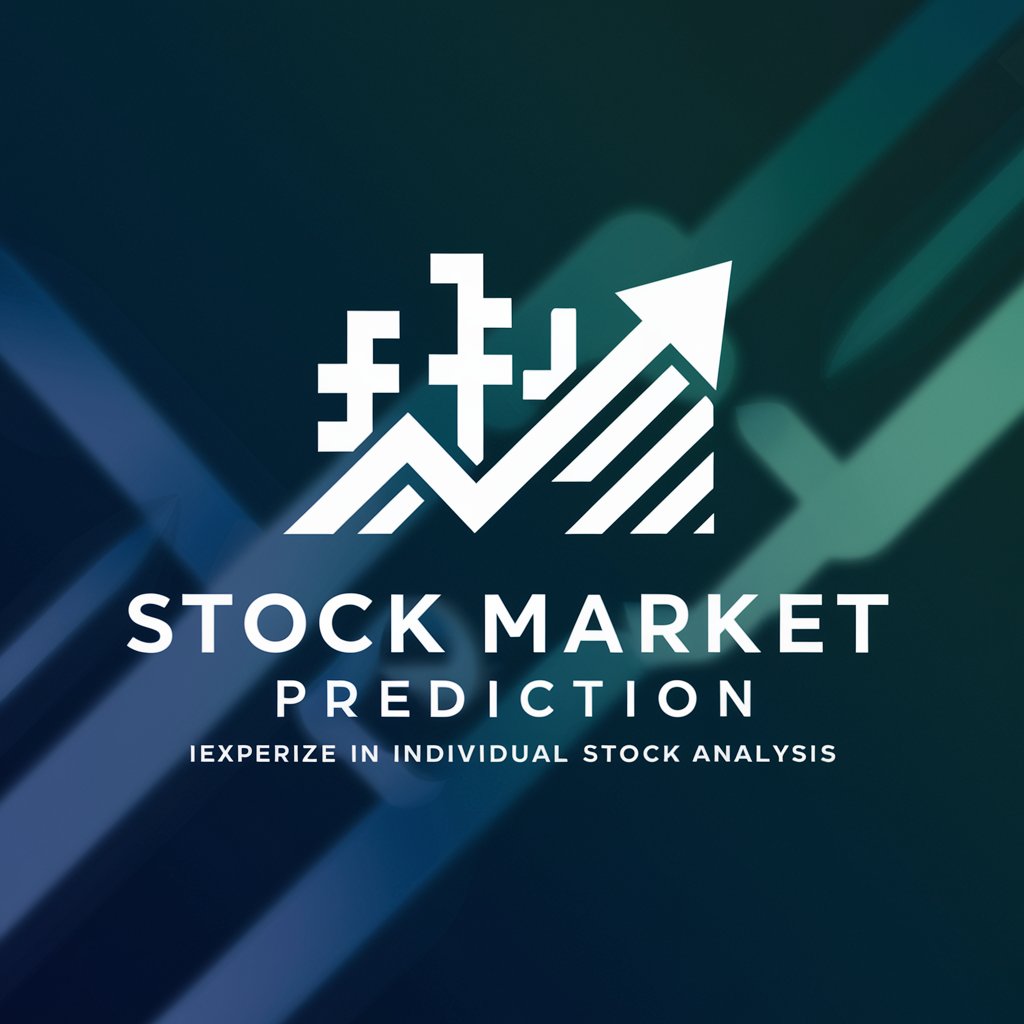
Stock Market Prediction
Professional and factual economics and finance expert for stock market analysis and predictions.
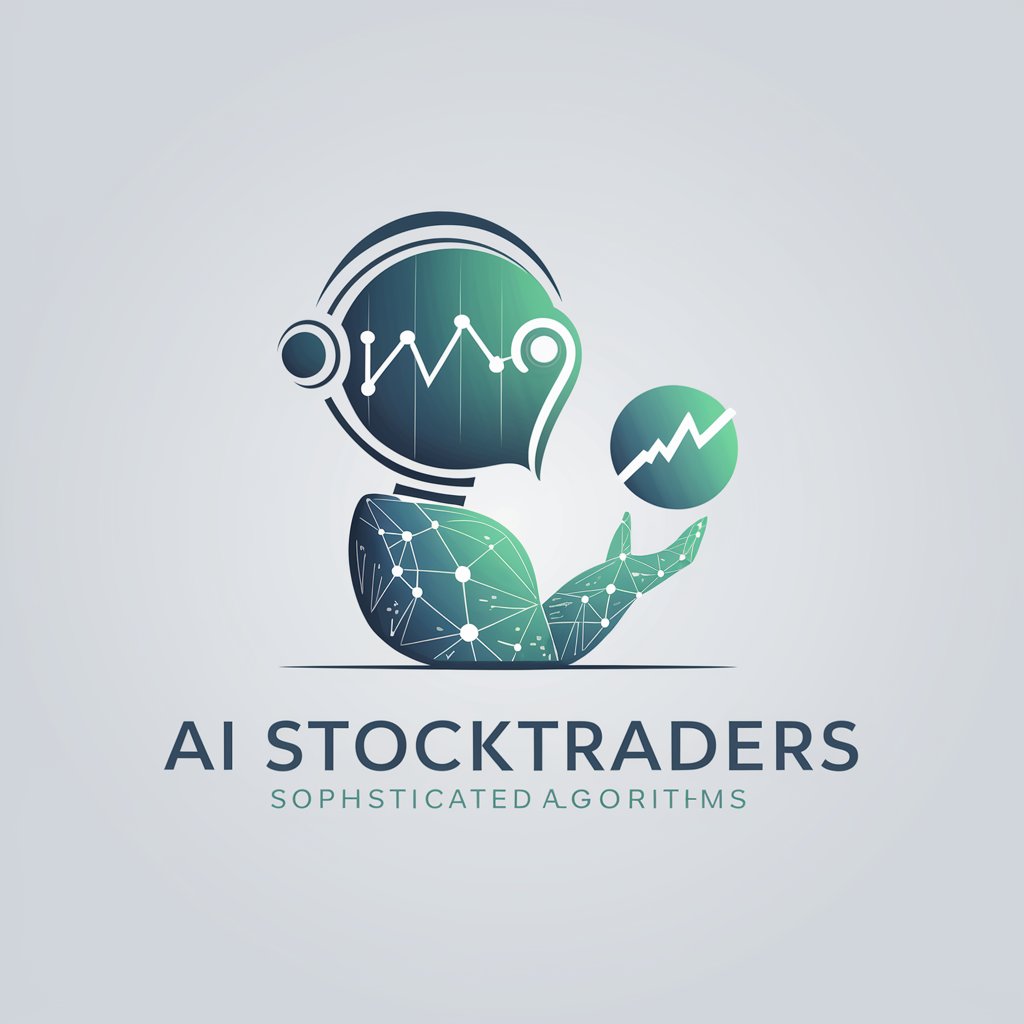
AI StockTraders
Provides AI-driven stock market analysis and insights
Overview of Stocks AI and Machine Learning Models
Stocks AI and machine learning models are designed to provide insights, predictions, and analytics for the stock market using advanced algorithms and data analysis techniques. These models analyze historical data, financial statements, market trends, and real-time information to identify patterns, predict future stock prices, and generate trading signals. By leveraging machine learning, these models continuously learn and adapt to new data, improving their accuracy over time. Examples include predictive analytics models for forecasting stock prices, sentiment analysis models to gauge market sentiment from news and social media, and portfolio optimization models to suggest the best allocation of assets for risk-adjusted returns. Powered by ChatGPT-4o。
Core Functions and Use Cases
Predictive Analytics
Example
Forecasting future stock prices based on historical data and trends
Scenario
A financial analyst uses a predictive model to identify potential investment opportunities by forecasting the future prices of stocks, helping to make informed decisions about when to buy or sell.
Sentiment Analysis
Example
Analyzing market sentiment from news articles and social media to predict stock movements
Scenario
An investment firm employs sentiment analysis models to monitor social media and news outlets, gauging public sentiment towards certain stocks and adjusting their investment strategies accordingly.
Portfolio Optimization
Example
Determining the optimal asset allocation to maximize returns and minimize risk
Scenario
A portfolio manager uses an AI-driven optimization model to analyze and suggest the best portfolio composition, balancing risk and return based on the investor's risk appetite.
Risk Management
Example
Identifying and assessing the risk levels of different investment options
Scenario
Risk management models help traders and investment managers to evaluate the risk associated with various stocks or portfolios, enabling them to make better-informed decisions to mitigate potential losses.
Target User Groups
Financial Analysts
Professionals who require in-depth market analysis and predictive insights to make informed investment decisions would benefit greatly from Stocks AI models.
Investment Firms
Firms that manage portfolios on behalf of clients can use these models to optimize asset allocation, manage risks, and identify new investment opportunities.
Individual Investors
Tech-savvy retail investors looking to enhance their trading strategies with AI-driven insights and recommendations.
Fintech Companies
Innovative financial technology companies seeking to integrate advanced stock market analytics into their services, offering cutting-edge solutions to their users.
How to Use Stocks AI and Machine Learning Models
Start Your Journey
Begin with a visit to an AI platform offering machine learning capabilities for stock analysis, seeking a free trial to explore functionalities without the need for a premium account.
Define Your Goals
Identify specific objectives you aim to achieve with AI in the stock market, such as predictive analysis, risk assessment, or portfolio management.
Data Preparation
Gather historical stock market data, financial statements, and relevant indicators that your model will analyze. Ensure data quality for accurate model training.
Model Selection and Training
Choose the appropriate machine learning model based on your goals. Use your prepared data to train the model, adjusting parameters for optimization.
Evaluation and Implementation
Evaluate the model's performance using a separate dataset. If satisfactory, integrate the model into your trading strategy or decision-making process.
Try other advanced and practical GPTs
API Explorer
Empowering API Integration with AI
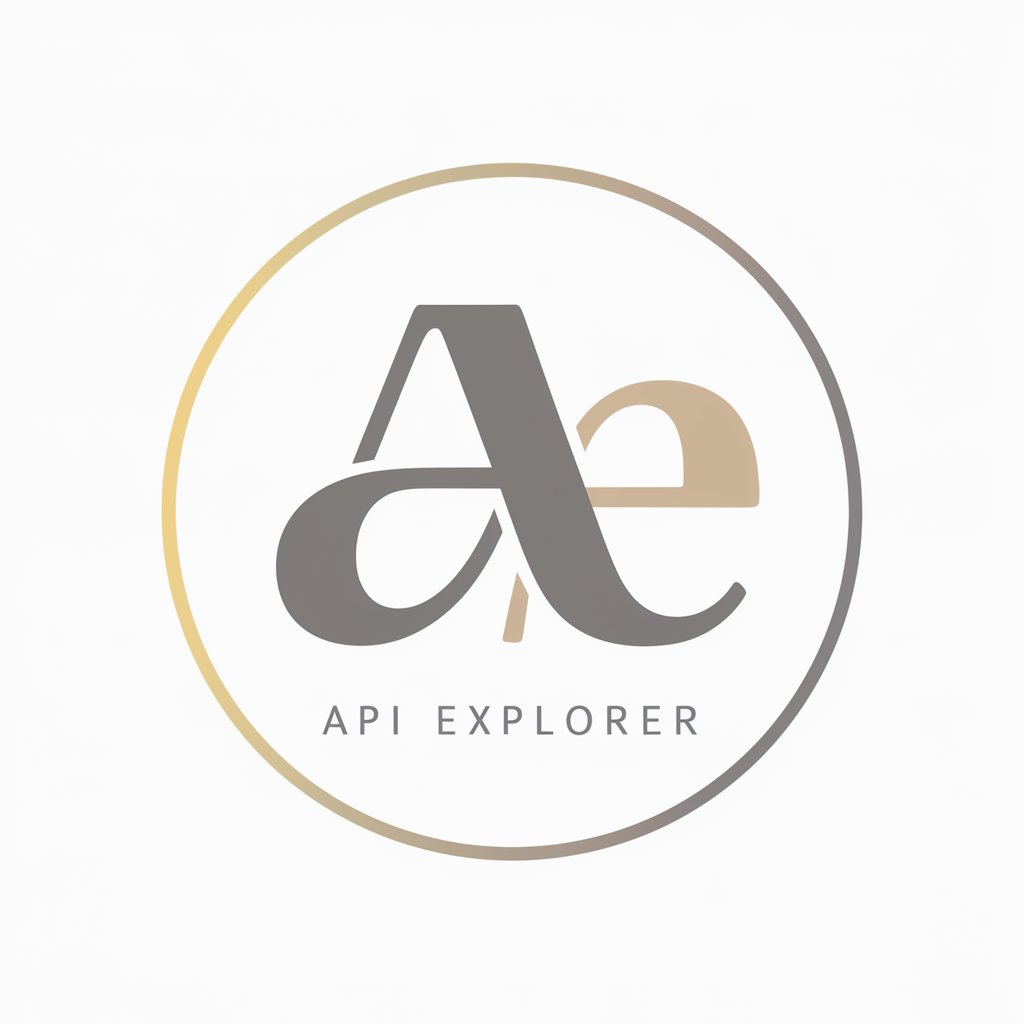
" Táplálkozási doctor "
Revolutionizing Your Health with AI
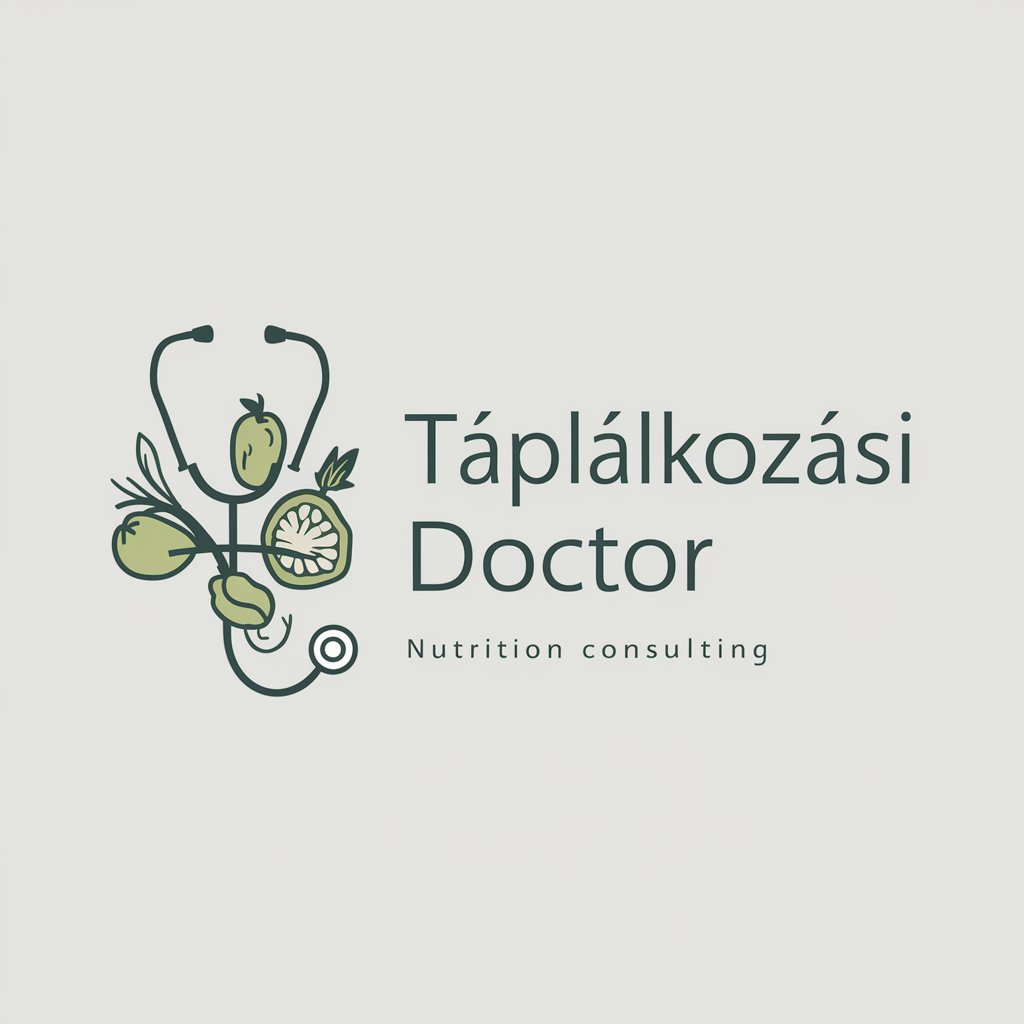
Citas & Frases Históricas
Bringing History to Life with AI
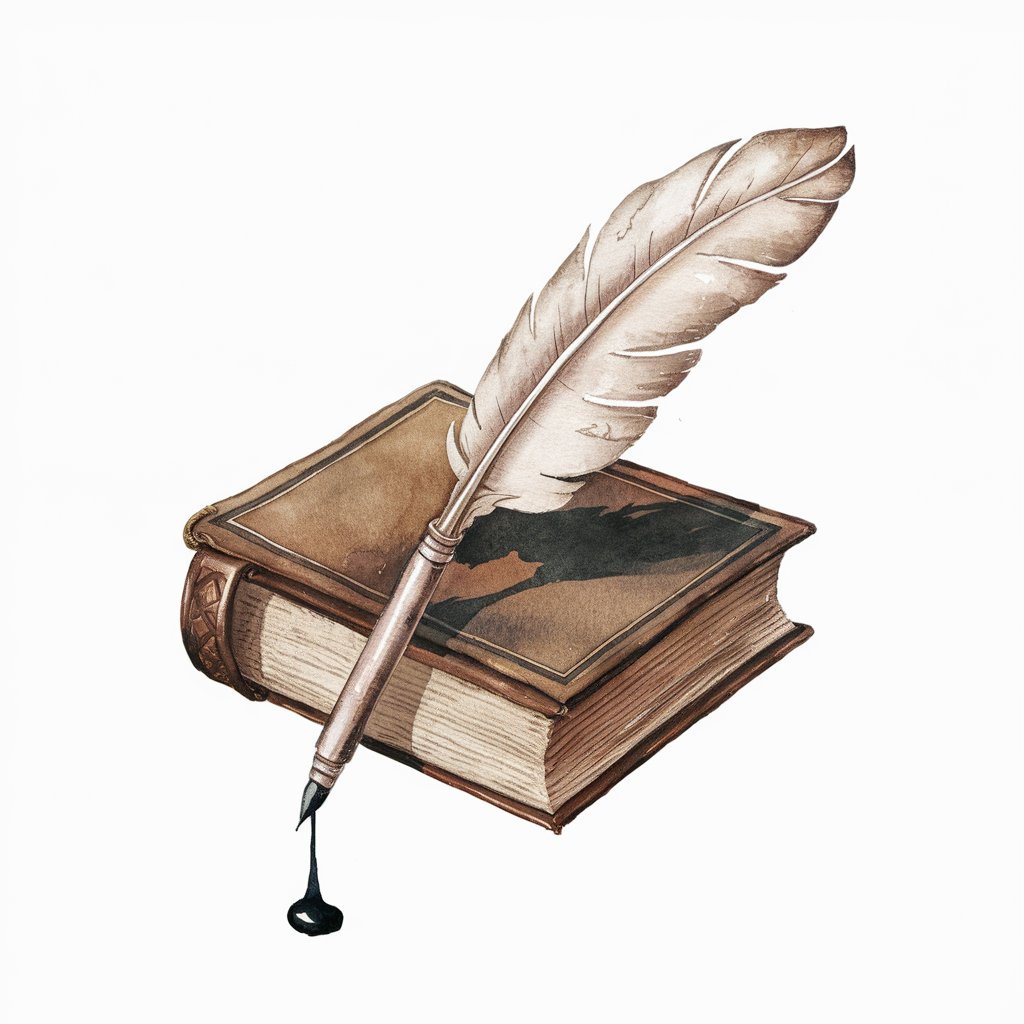
Prince2 (7)
Streamlining Projects with AI
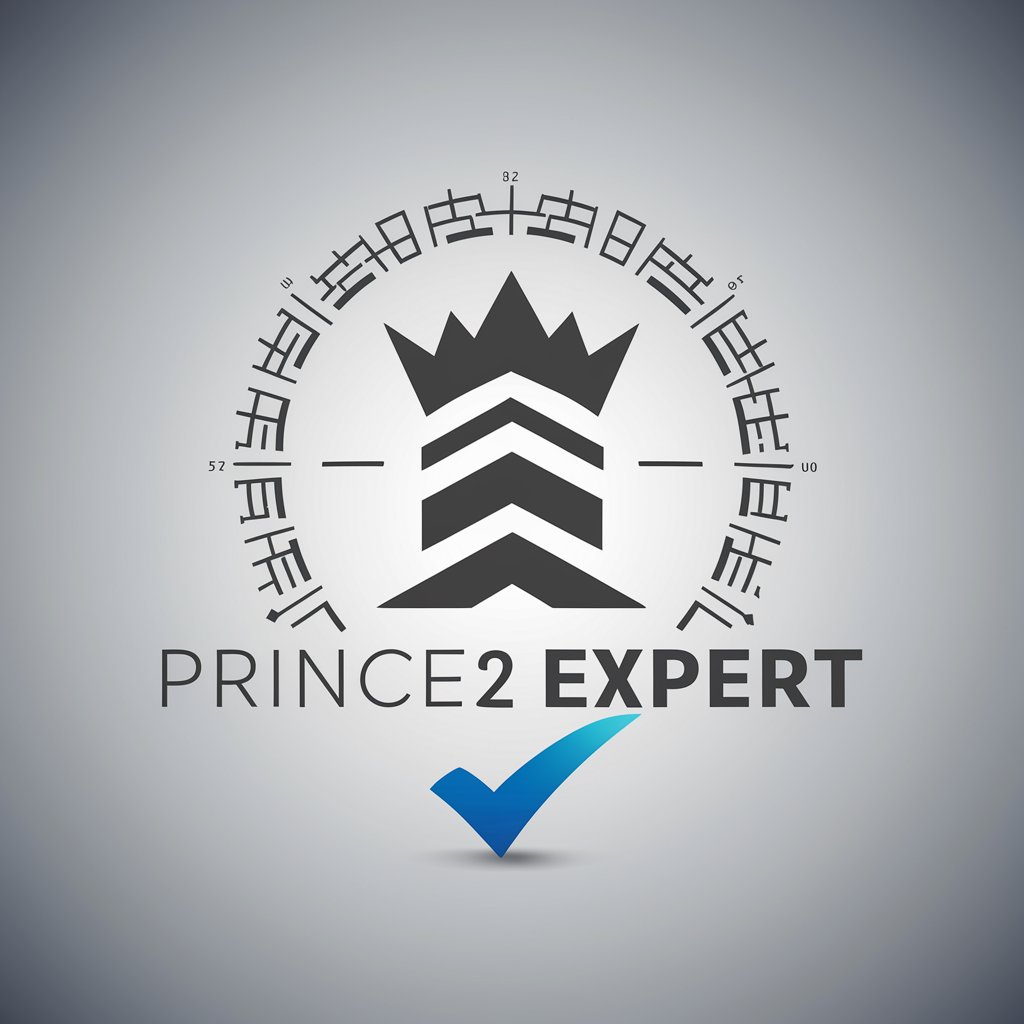
Lua Mentor
Empowering Lua Development with AI
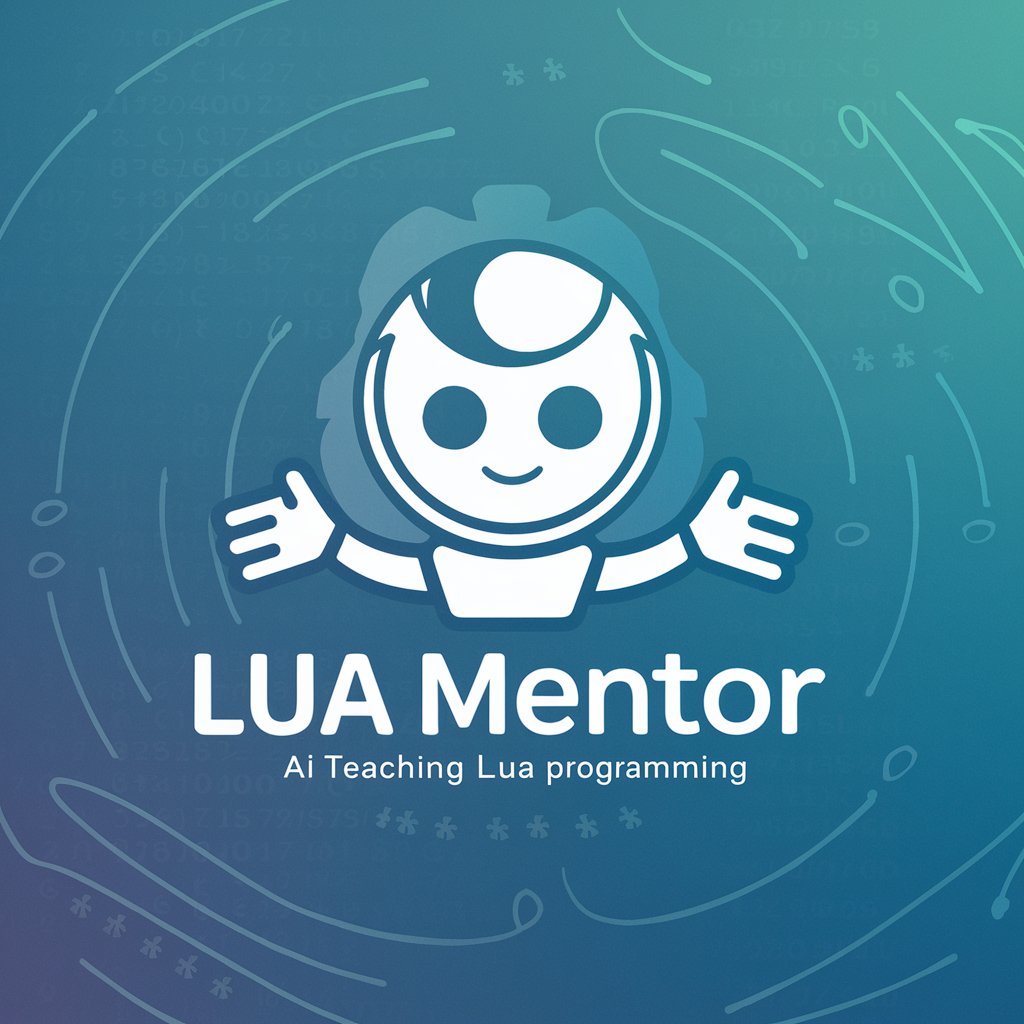
Cyber Watchdog
Your AI-powered cybersecurity advisor.
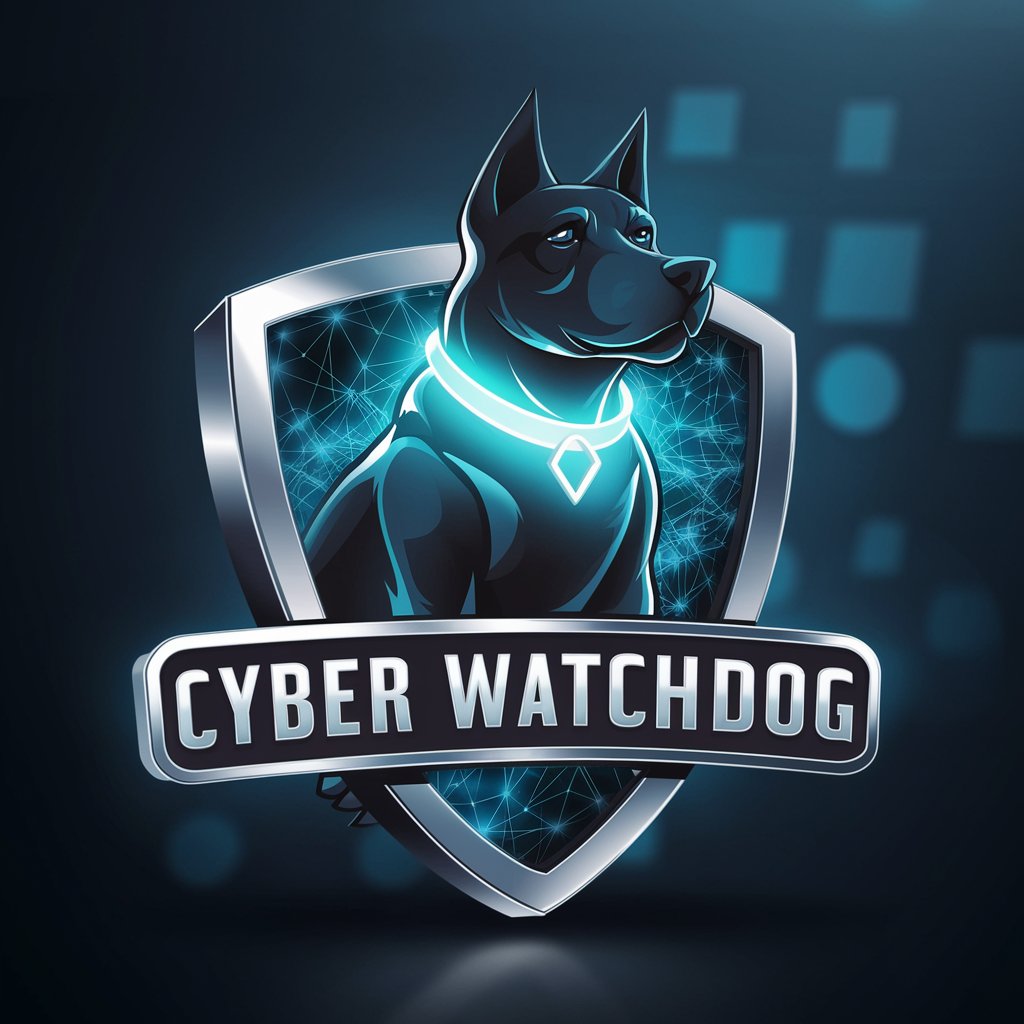
RedTeamGPT
Empowering Cybersecurity with AI
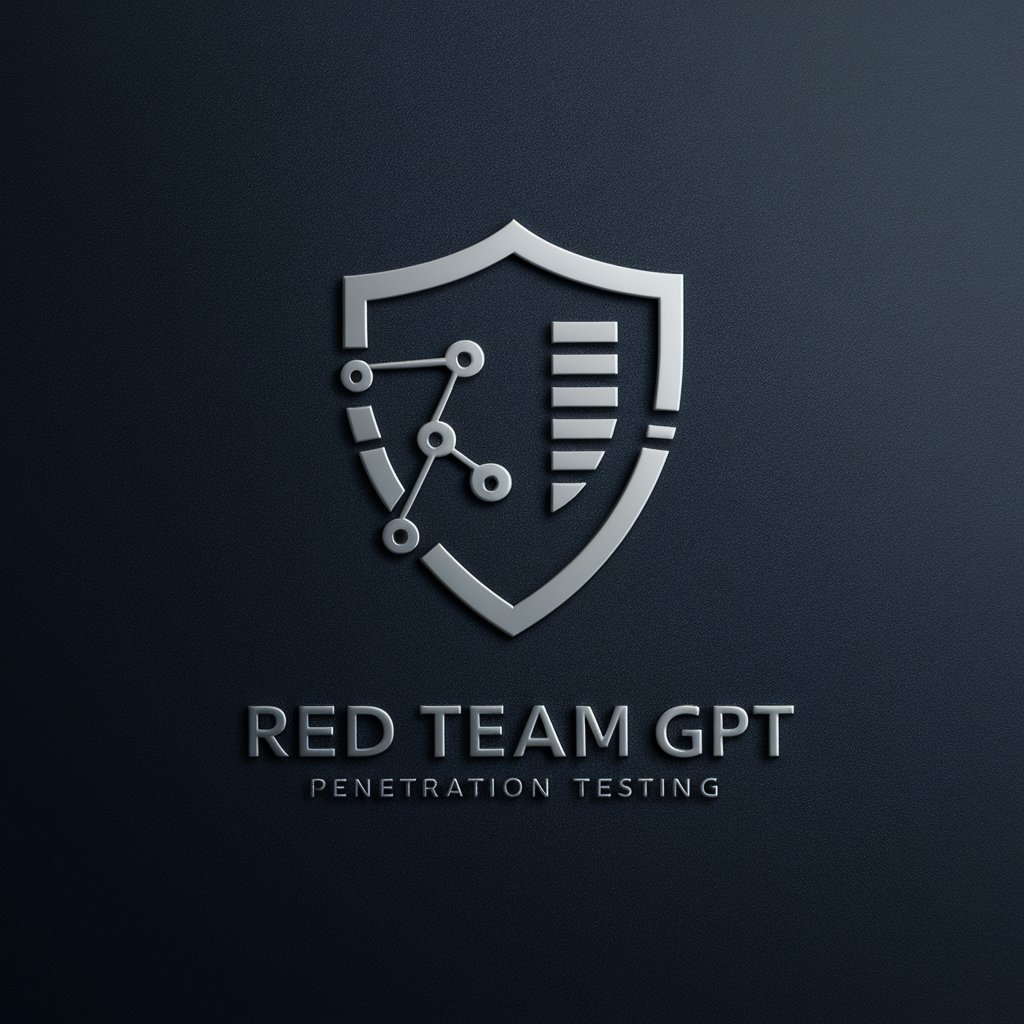
Medi Editor Pro
Revolutionizing Medical Editing with AI
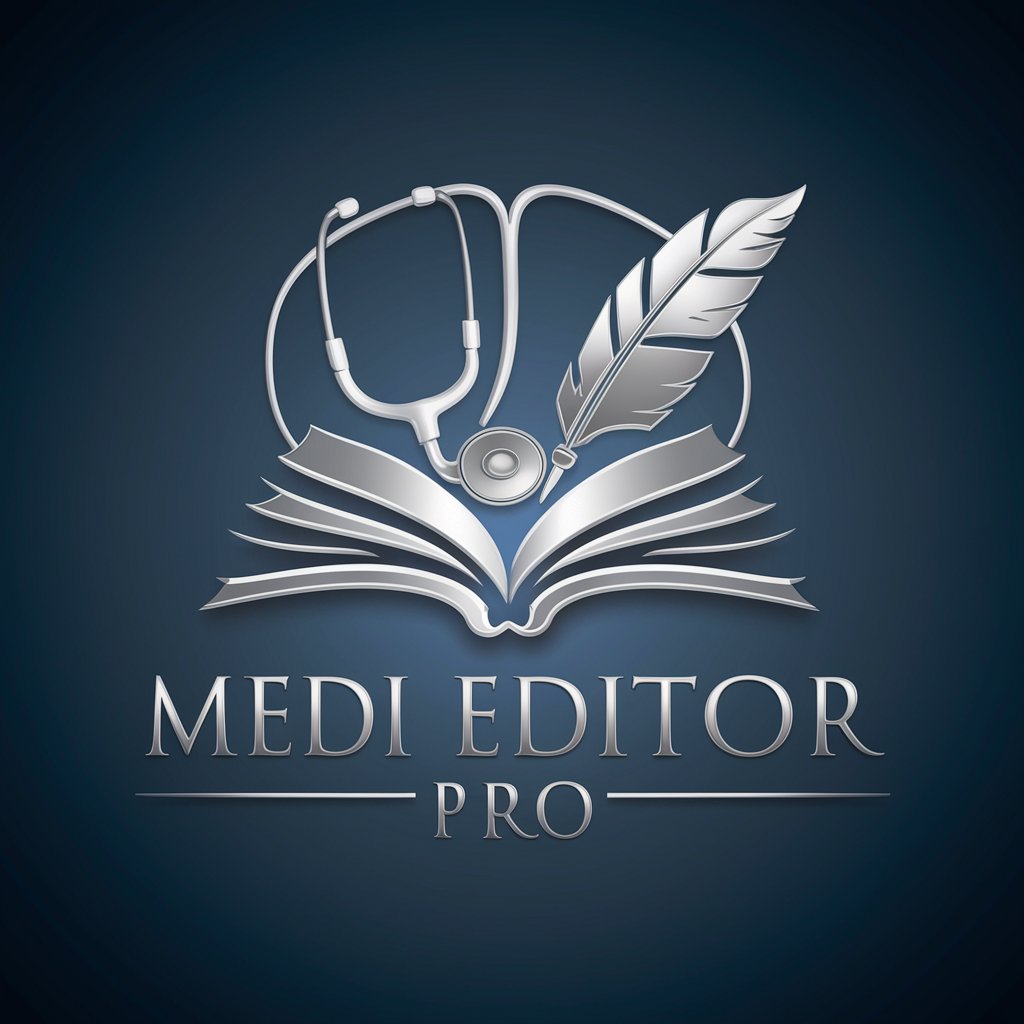
PC Builder Pro
AI-Powered Custom PC Building
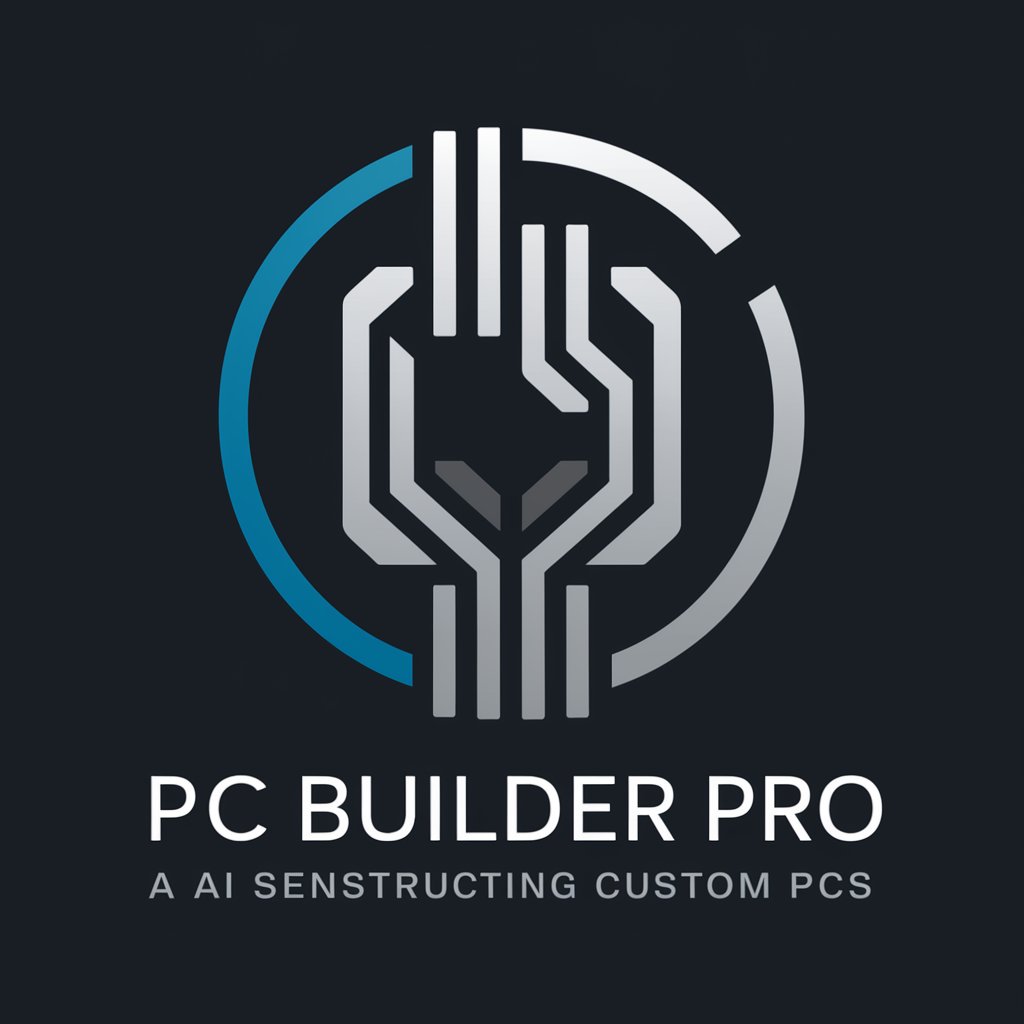
Bonsai GPT
Cultivate perfection with AI-powered bonsai guidance.
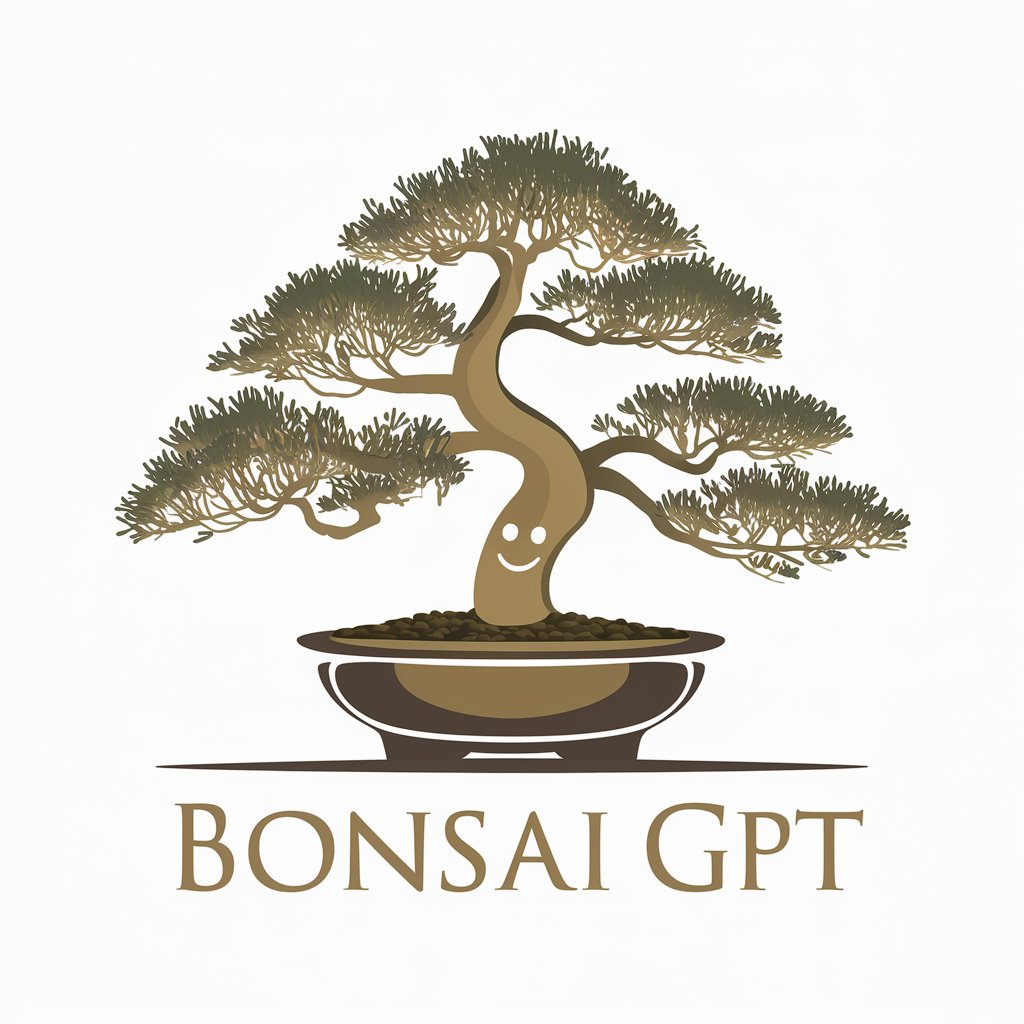
BioInfo Analyst
Empowering Bioinformatics with AI

Instrutivos Procedimentos CAPS RJ
Streamlining CAPS Operations with AI
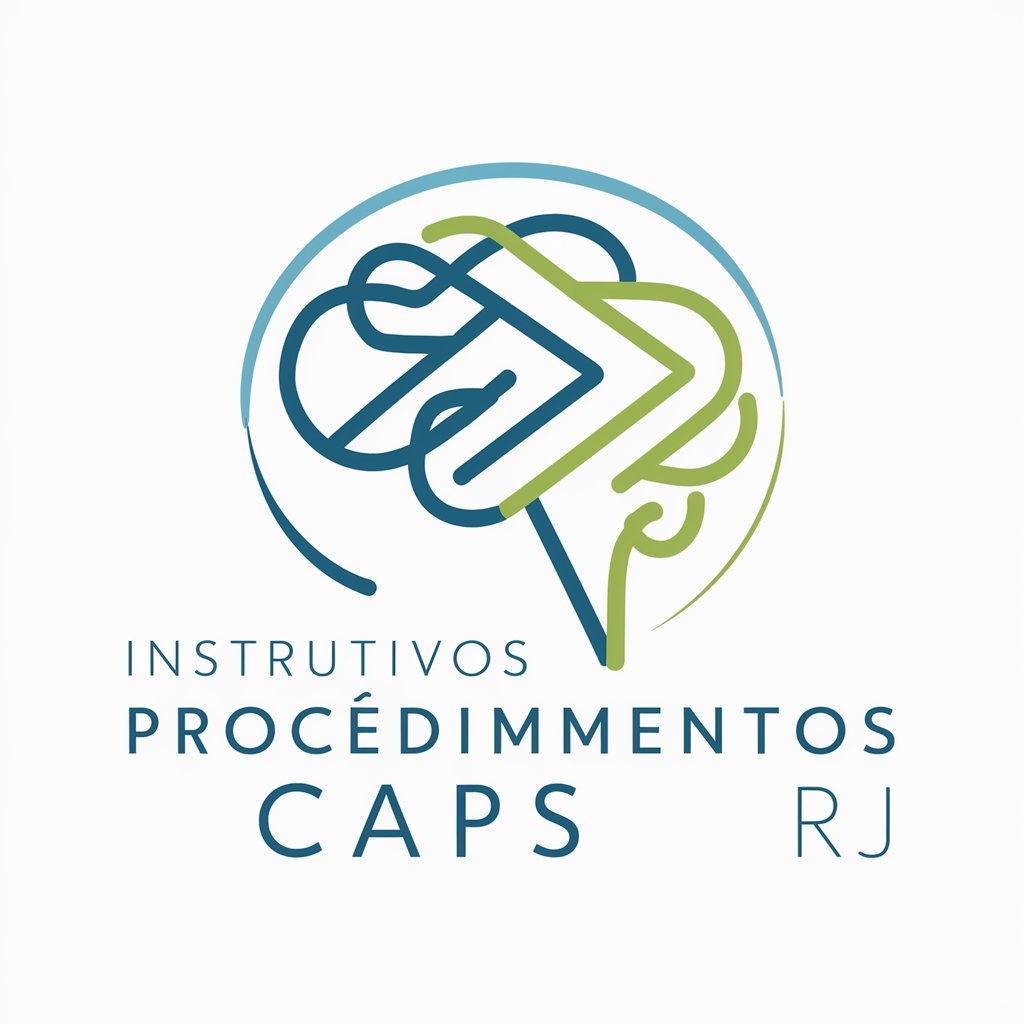
FAQs on Stocks AI and Machine Learning Models
What data is essential for training Stocks AI models?
Key data includes historical stock prices, trading volumes, financial statements, market indicators, and economic factors. Quality and granularity of data significantly impact model accuracy.
Can AI models predict stock market movements accurately?
While AI models can identify patterns and trends not easily observable, predicting market movements with absolute accuracy is challenging due to market volatility and unforeseen events.
How do machine learning models differ in stock analysis?
Models vary in complexity, from linear regression for trends analysis to neural networks for deep learning tasks. The choice depends on the specific analysis goal and data complexity.
What are common challenges in deploying Stocks AI models?
Challenges include overfitting, data over-reliance, regulatory compliance, and ensuring models remain adaptive to market changes without manual intervention.
How can one enhance the performance of a Stocks AI model?
Enhancements can be achieved through continuous training with updated data, feature engineering to identify new predictive signals, and ensemble methods to combine multiple models for improved accuracy.