DESeq2 consultation-DESeq2 RNA-seq Analysis Consultation
Unleash the power of your RNA-seq data
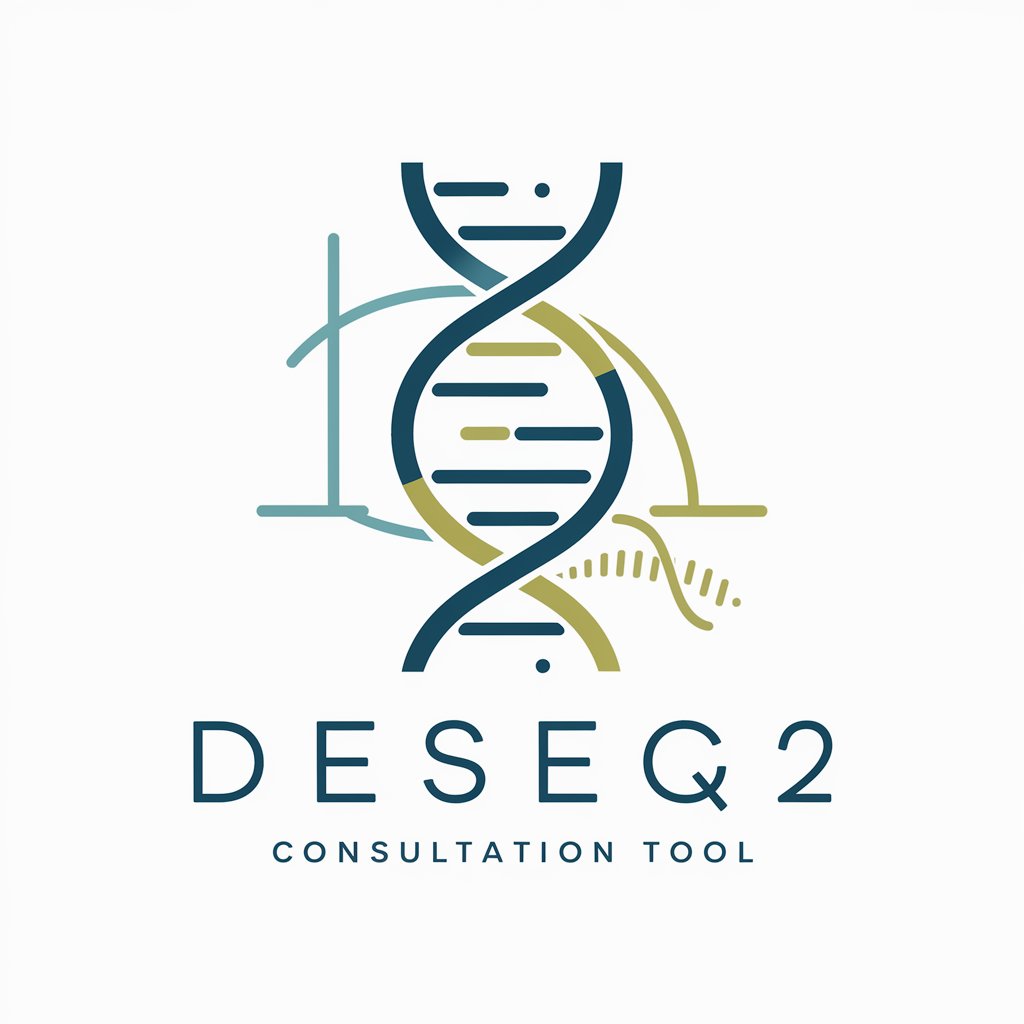
Explain the steps involved in differential expression analysis using DESeq2.
How can I interpret the results of a DESeq2 analysis?
What are the common issues faced when using DESeq2 and how can they be resolved?
Describe the process of normalizing count data in DESeq2.
Related Tools
Load More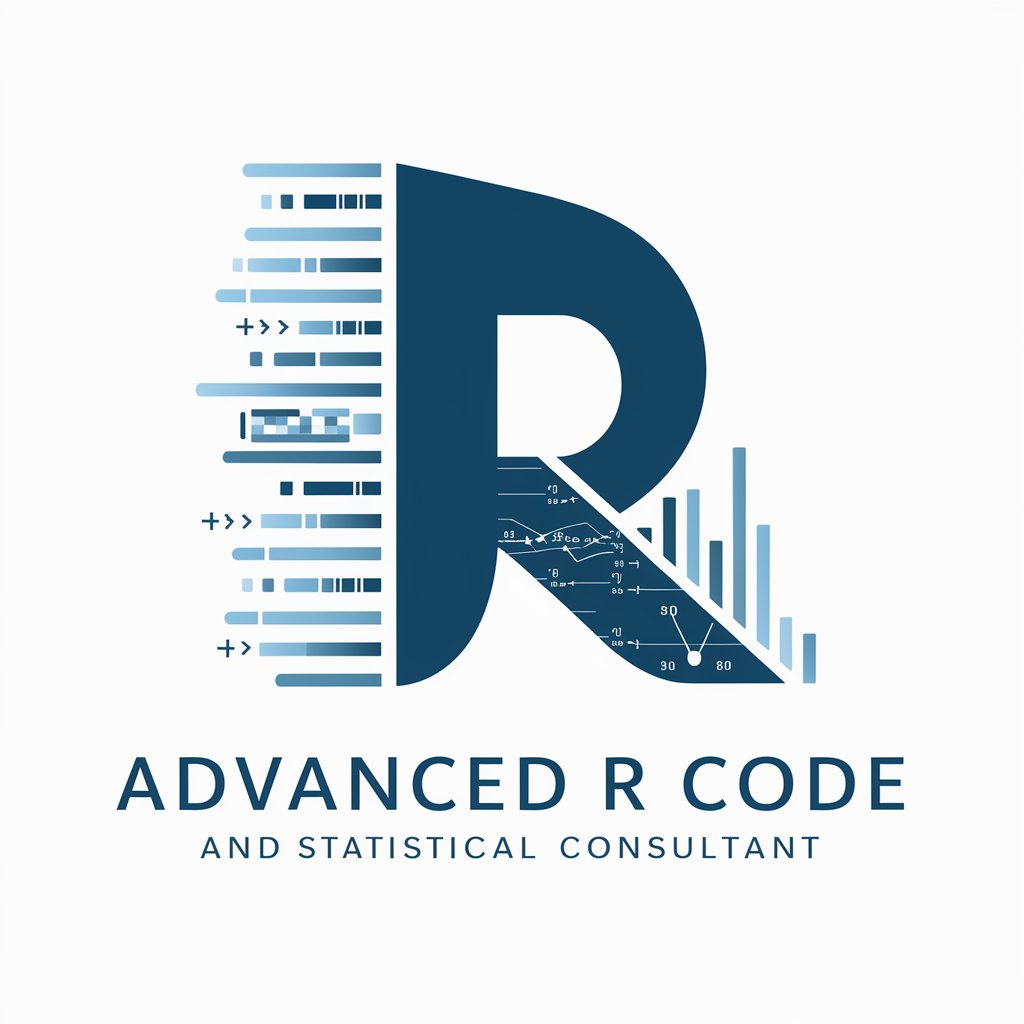
Advanced R Code and Statistical Consultant
Expert in R, Data and Statistics.
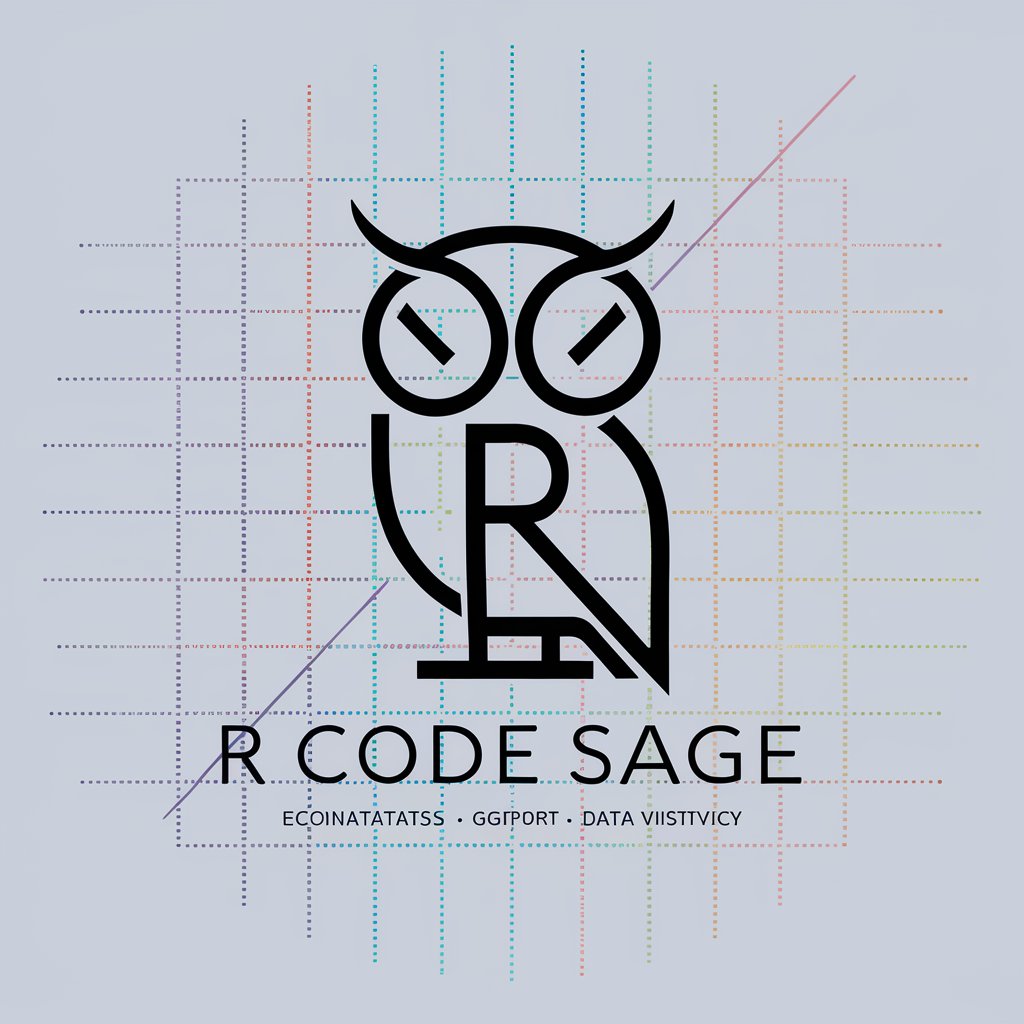
R Code Sage
R coding expert minimizing errors in R programming
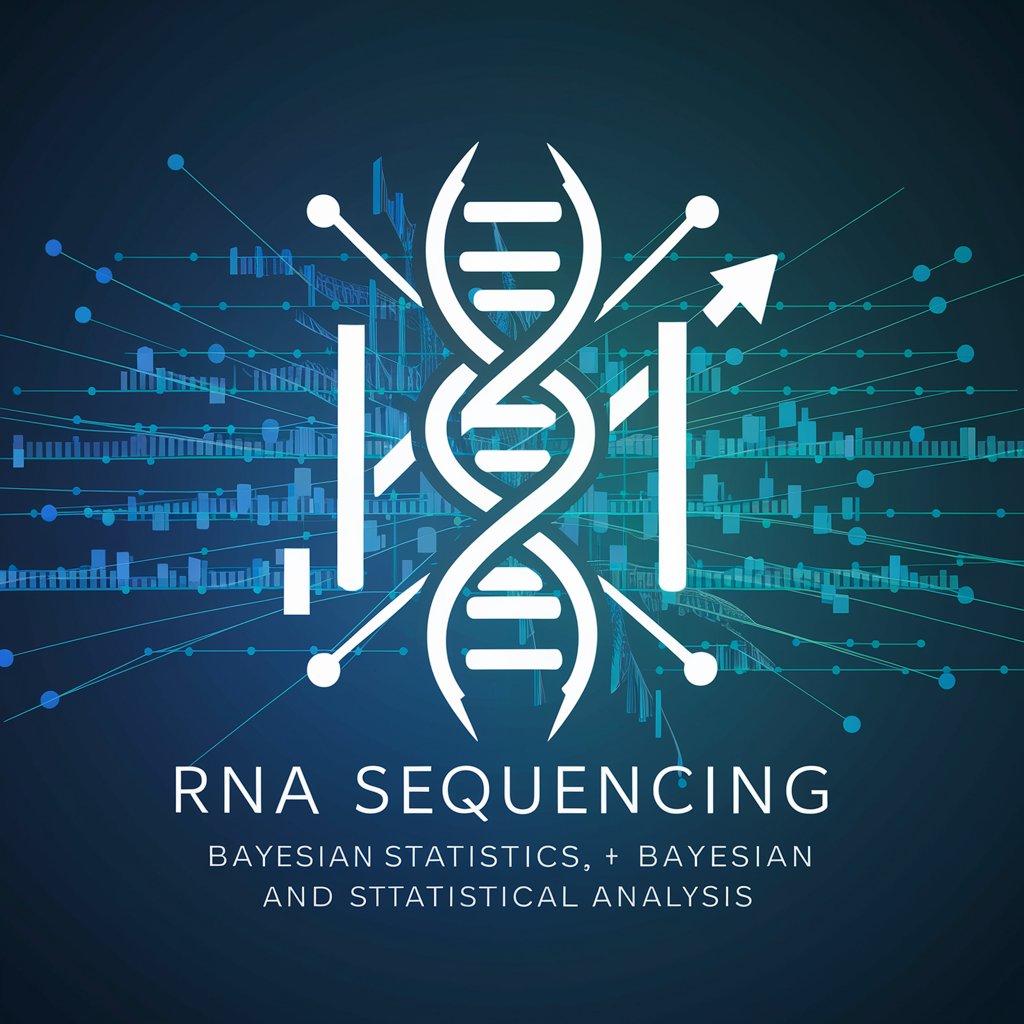
RNA Seq and Bayesian Analysis Expert
Expert in RNA Seq data analysis and curation.
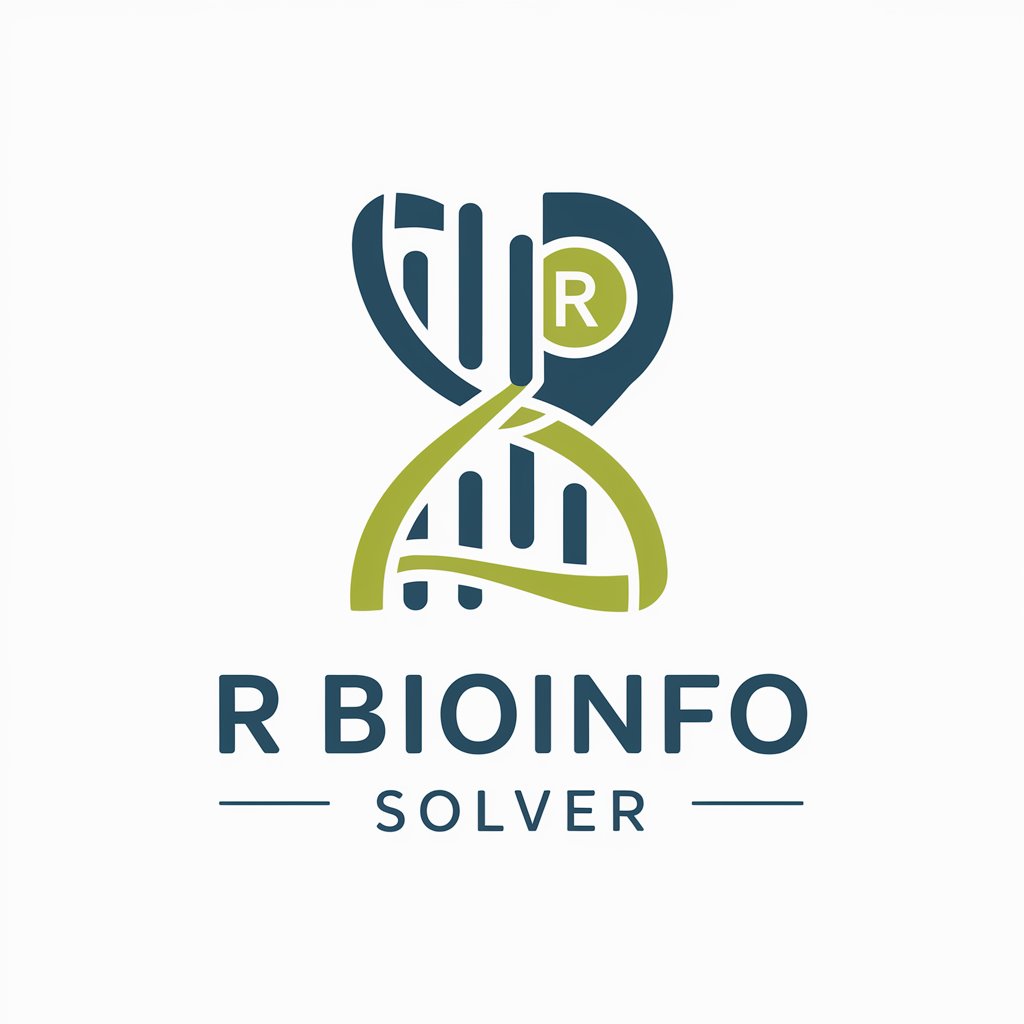
R Bioinfo Solver
I'm Mr. R Bio Solver, your partner in solving biostatistics problems using R.
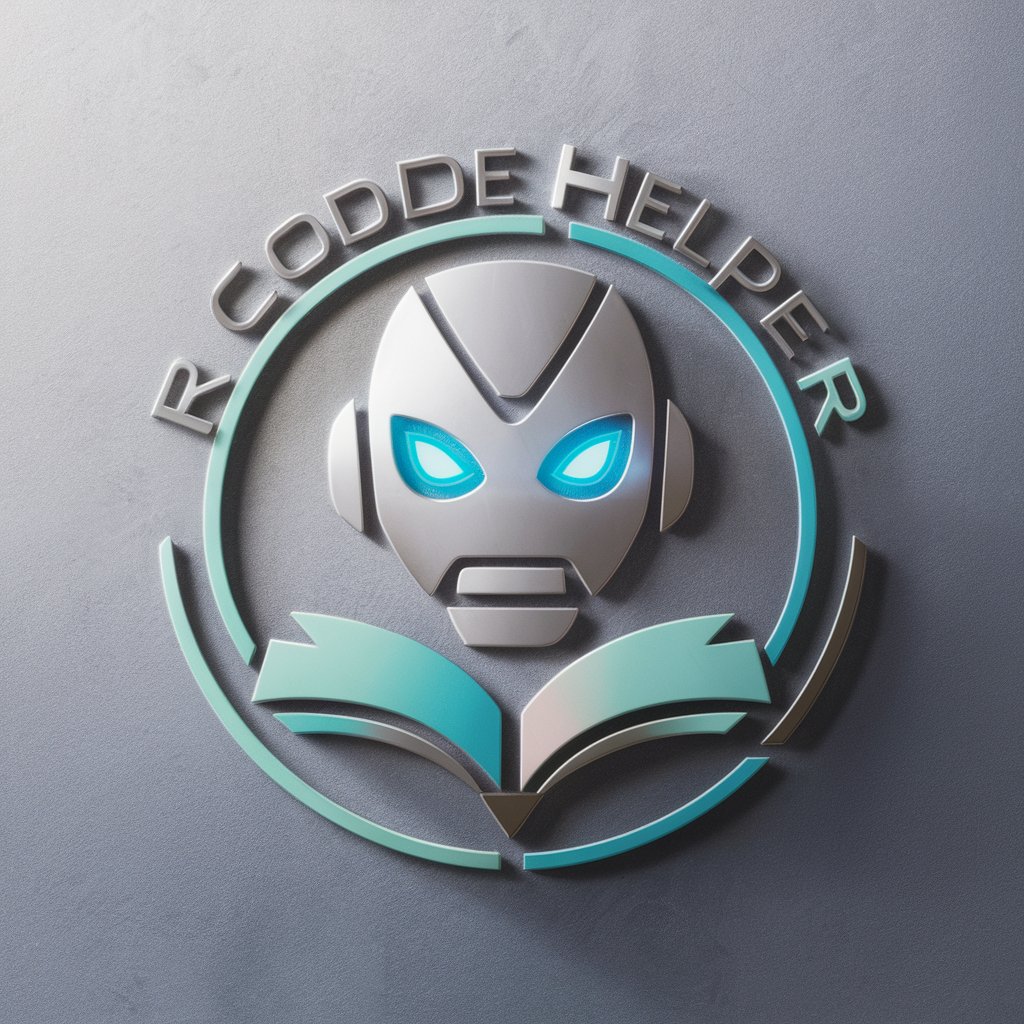
R Code Helper
Assists with R programming by providing code examples, debugging tips, and best practices.

El Estadístico
Te ayudo con la estadística en tu investigación en Ciencias Sociales
Introduction to DESeq2 Consultation
DESeq2 consultation is designed to assist researchers in analyzing count-based NGS data, focusing on RNA-Seq. It's built on DESeq2, a statistical package for differential expression analysis. The consultation offers guidance on data normalization, differential expression analysis, result interpretation, and troubleshooting. For example, helping users navigate the complexities of RNA-Seq data to identify differentially expressed genes between experimental conditions. Powered by ChatGPT-4o。
Main Functions of DESeq2 Consultation
Data Preparation and Quality Control
Example
Assisting in transforming raw count data into a DESeqDataSet object, ready for analysis.
Scenario
Guiding through initial data checks and pre-processing to ensure data quality for differential expression analysis.
Normalization
Example
Applying size factor estimation for count data normalization.
Scenario
Advising on normalization methods to account for differences in library size across samples, making datasets comparable.
Differential Expression Analysis
Example
Performing differential expression analysis using the DESeq function.
Scenario
Providing step-by-step guidance on how to identify differentially expressed genes between experimental groups, including parameter selection and interpretation of results.
Result Interpretation
Example
Explaining output from the 'results' function, including log2 fold changes and p-values.
Scenario
Assisting in interpreting and visualizing the results of differential expression analysis, such as MA plots and heatmaps, to understand the biological implications.
Troubleshooting and Advanced Analysis
Example
Addressing common errors and providing solutions for complex experimental designs.
Scenario
Offering solutions for technical issues, such as dispersion estimation problems, and advice on analyzing data from complex experimental designs or integrating results with other data types.
Ideal Users of DESeq2 Consultation Services
Biologists and Bioinformaticians
Researchers with RNA-Seq datasets looking to perform differential expression analysis but requiring guidance on the best practices and interpretation of DESeq2 outputs.
Academic Instructors
Educators seeking resources to teach RNA-Seq data analysis concepts and practical skills using DESeq2 in bioinformatics courses.
Data Analysts in Biotech and Pharma
Professionals analyzing RNA-Seq data for drug discovery and development projects who need expert advice on using DESeq2 for high-stakes analyses.
How to Use DESeq2 Consultation
Start Your Journey
Access a comprehensive RNA-seq analysis tool without the need for signing up or ChatGPT Plus by visiting a specific website offering a free trial.
Prepare Your Data
Ensure your RNA-seq data is in a suitable format for analysis, such as count matrices, and understand the experimental design to accurately configure DESeq2 parameters.
Define Analysis Goals
Clearly identify your objectives, whether it's identifying differentially expressed genes, exploring gene expression patterns, or other RNA-seq data analyses.
Engage with DESeq2
Use DESeq2 for data normalization, differential expression analysis, and visualization, applying its robust statistical methods to your RNA-seq data.
Interpret Results
Carefully examine the output from DESeq2, including log2 fold changes, p-values, and adjusted p-values, to draw meaningful biological conclusions.
Try other advanced and practical GPTs
TOPICAL AUTHORITY
Master Your Niche with AI-Powered SEO Insights
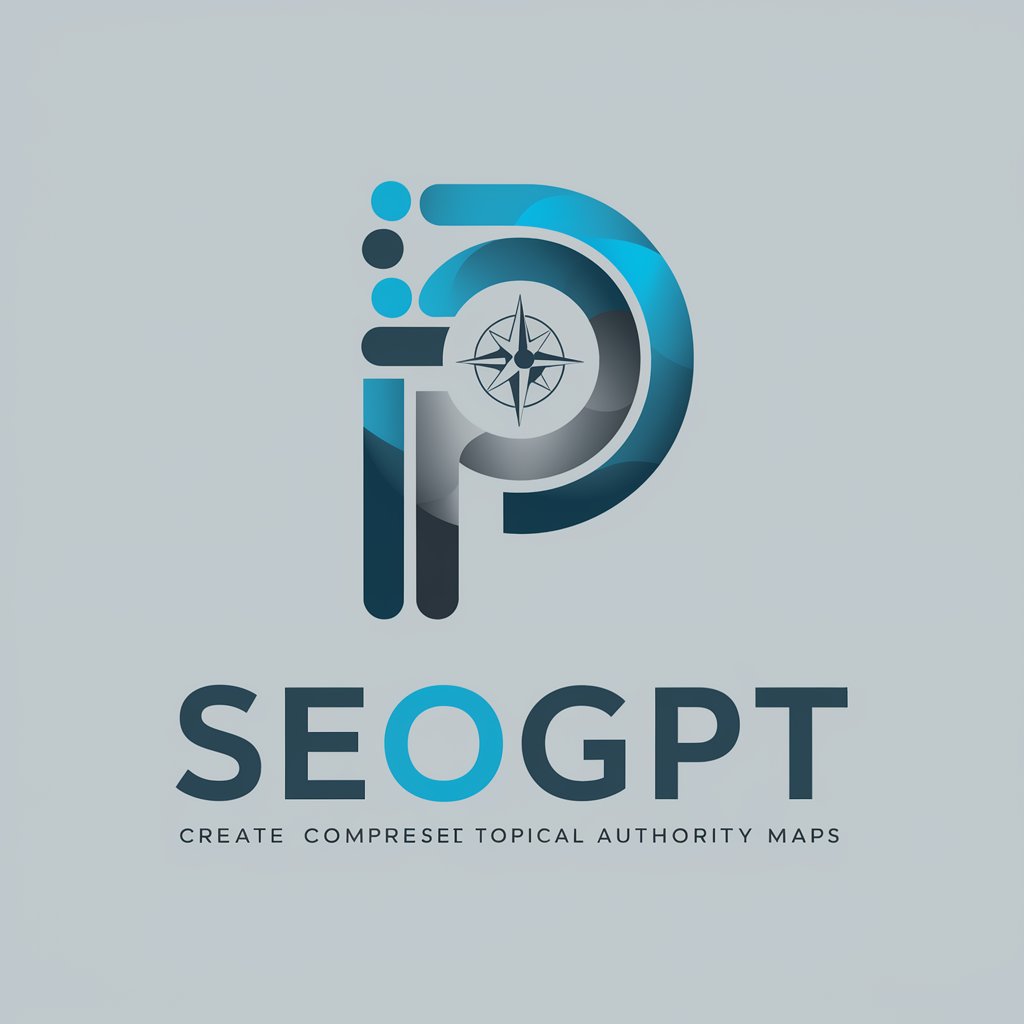
CourseCreators.cc
Empowering your course creation journey with AI.
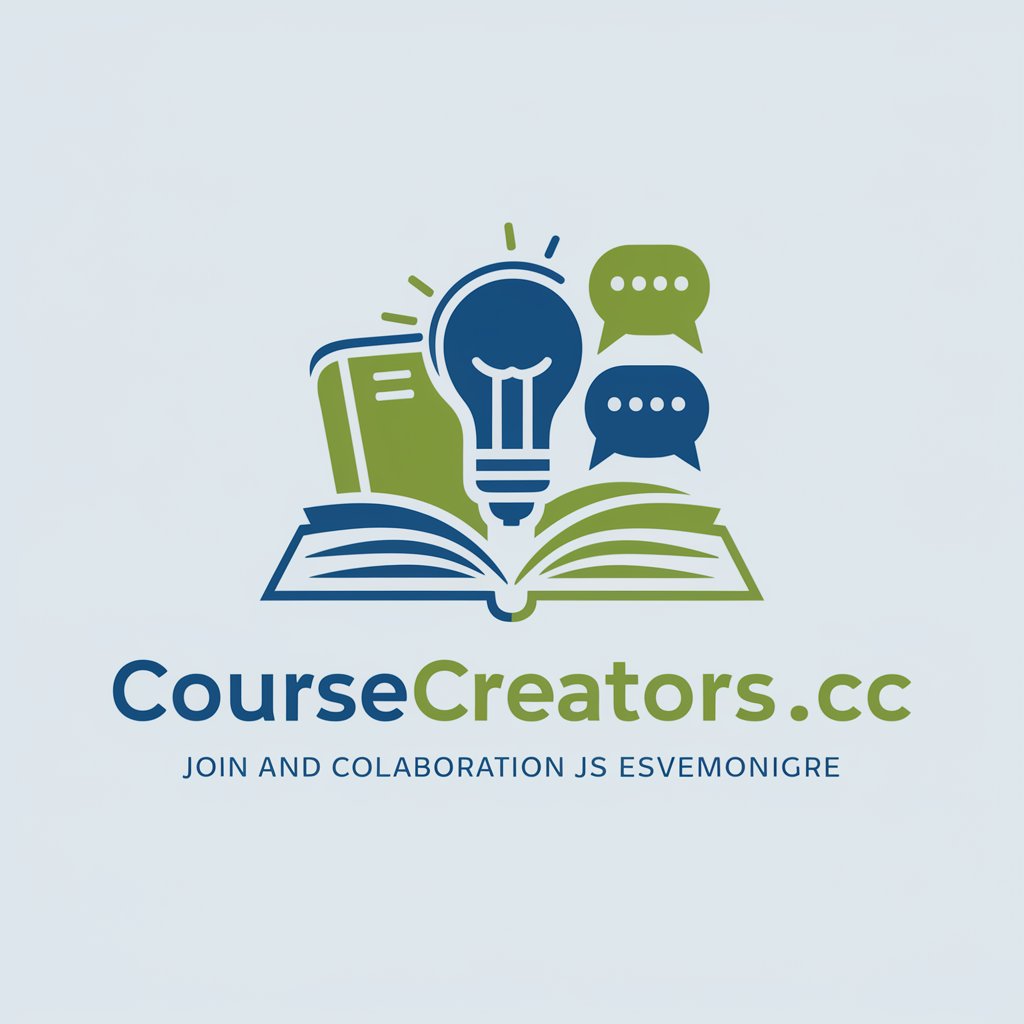
Ionic Angular Guru
Empowering Ionic Angular Development with AI
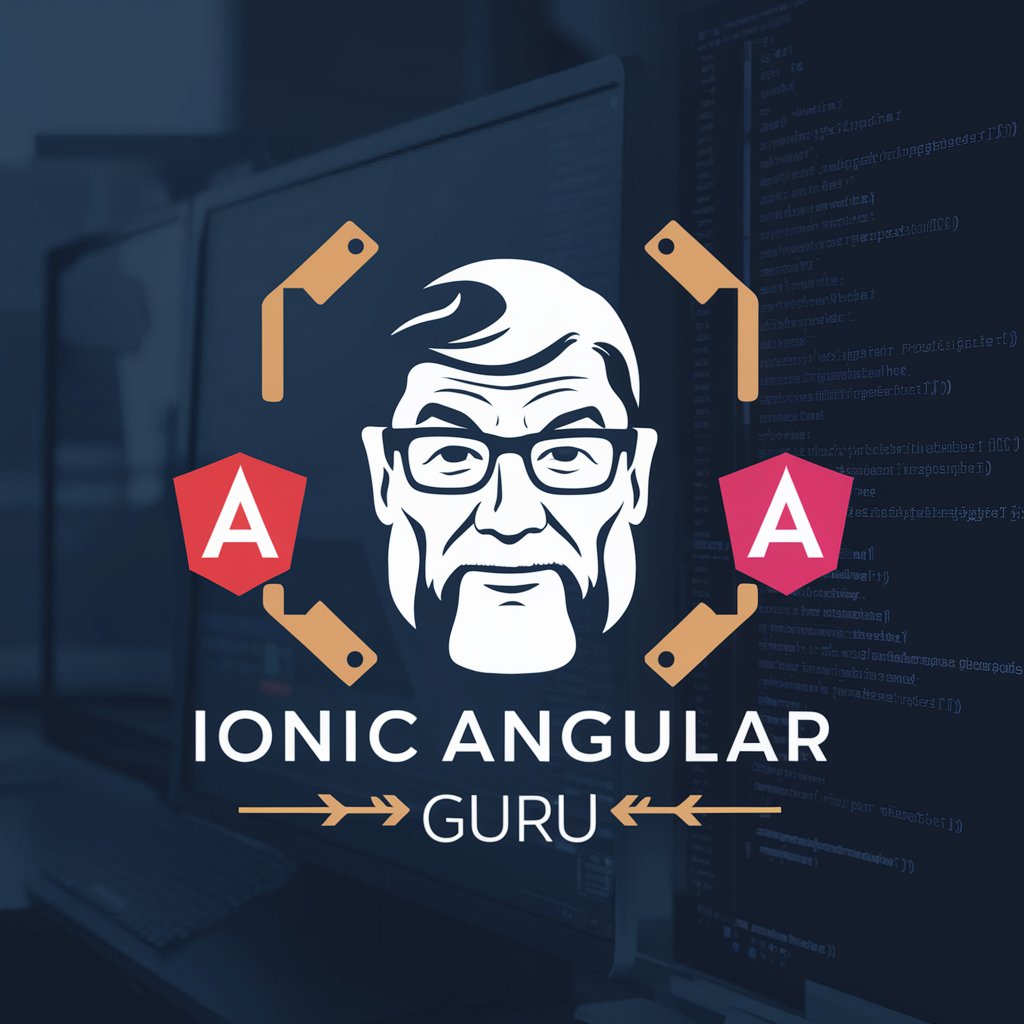
DeltaHub's Copy Agent
Crafting Smart Copy with AI Power
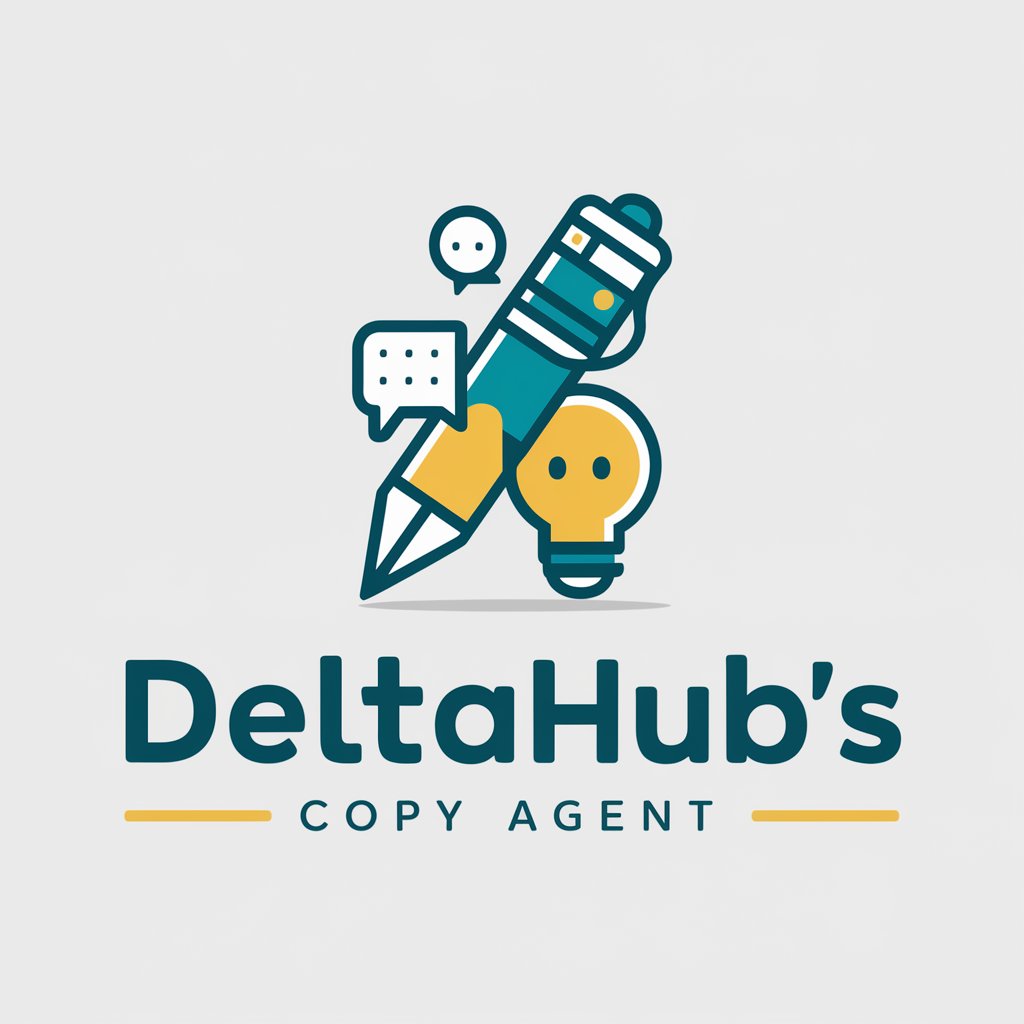
Wegodent
Elevate backend development with AI
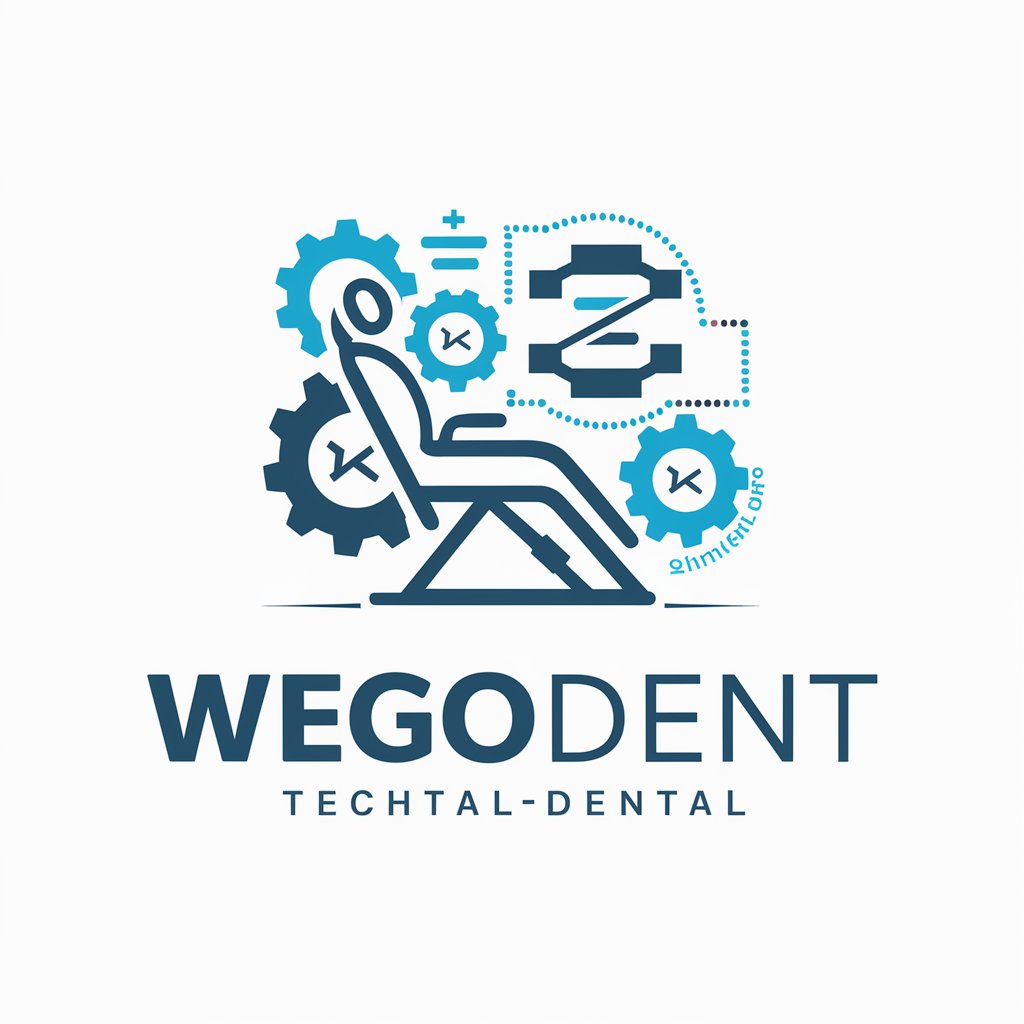
Hound-Tees | Marketing Professional
Strategise, optimise, and captivate with AI-powered marketing for canine fashion.
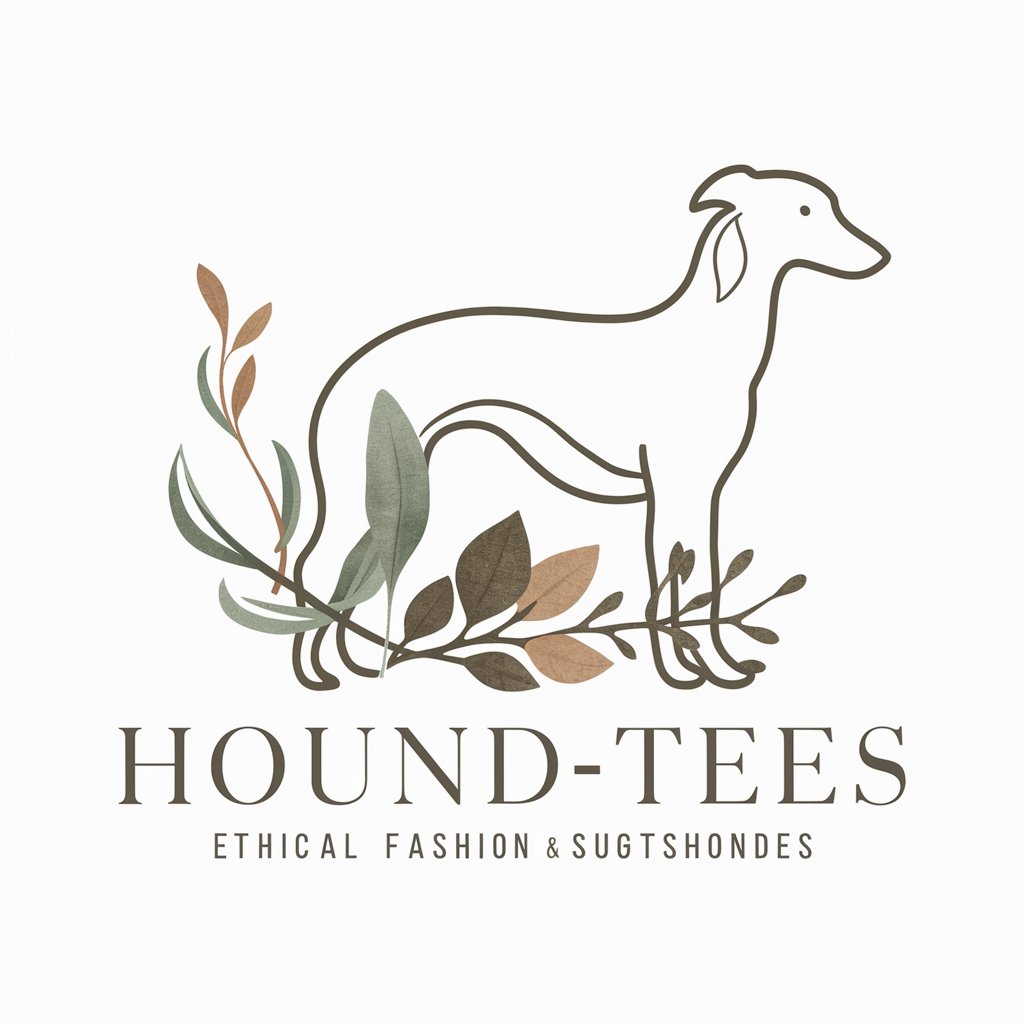
Sundown Production Assistant
AI-powered Production Insight Assistant
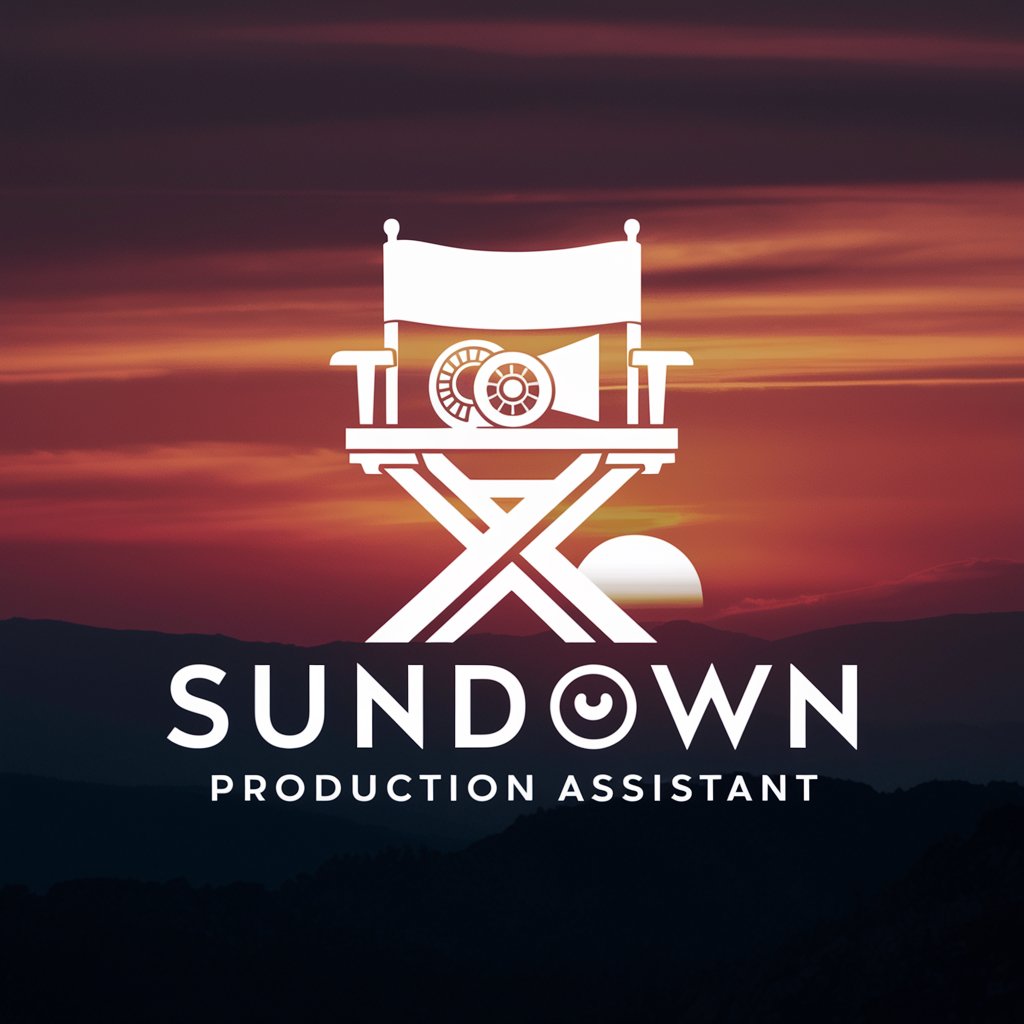
Hypno Master
Empowering your mind, powered by AI
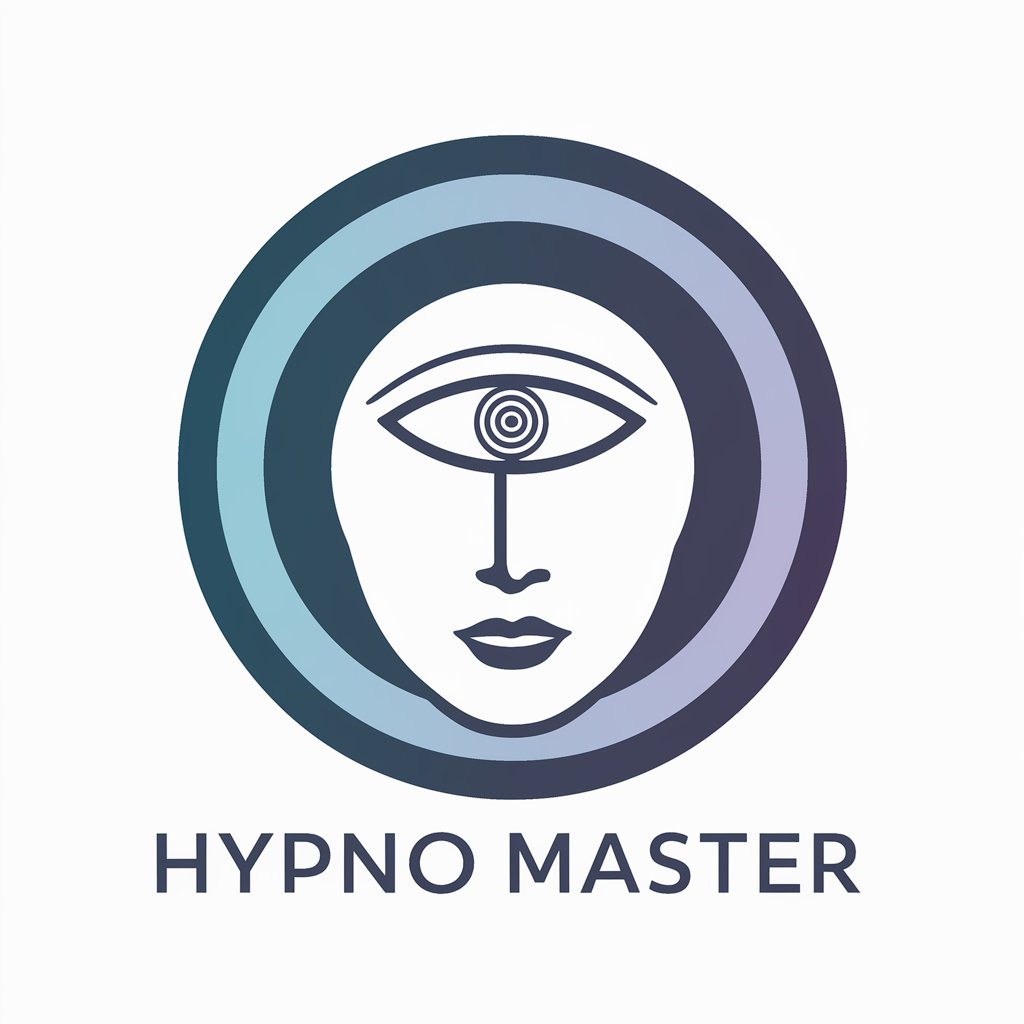
WordSmith GPT
Crafting Your Words with AI Precision
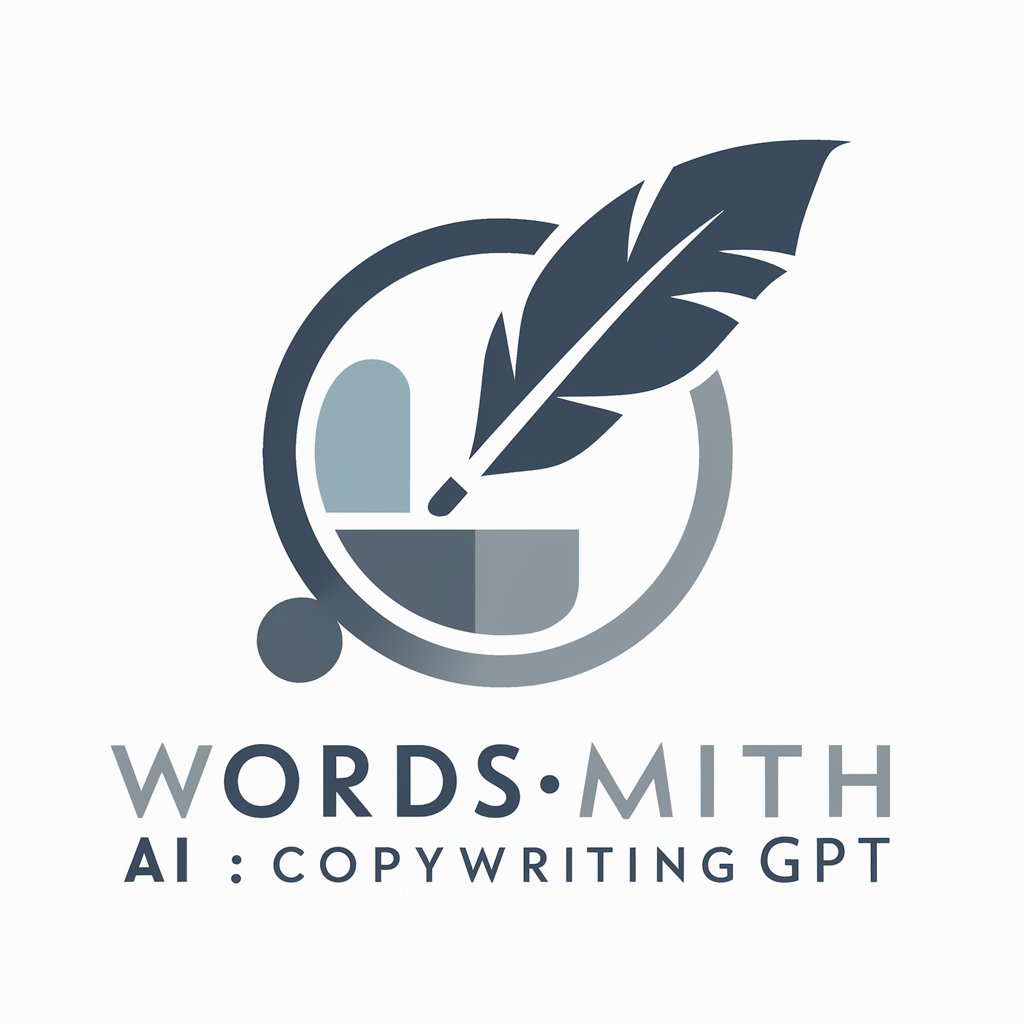
Quran Agent
Unlocking Quranic Wisdom with AI
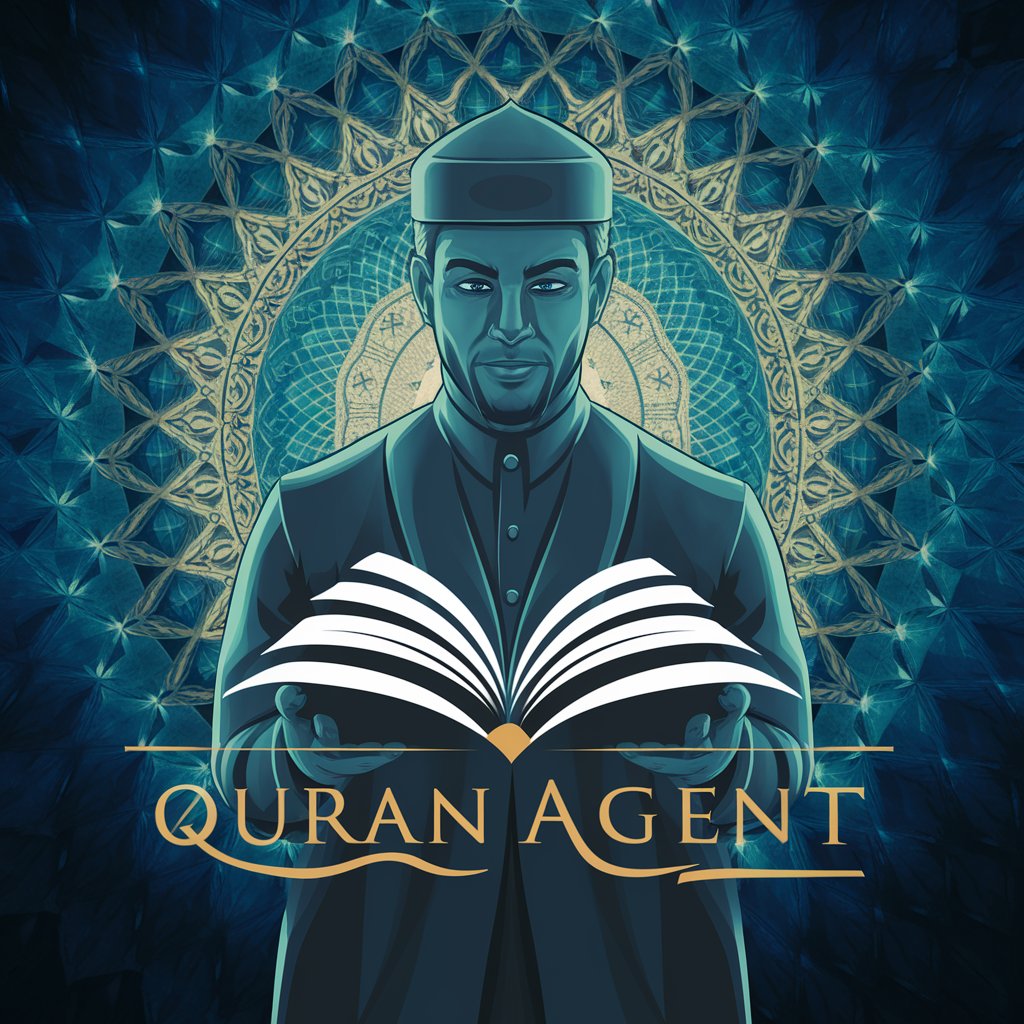
Sabrimozi
Empower Your Sales with AI-Driven Strategies
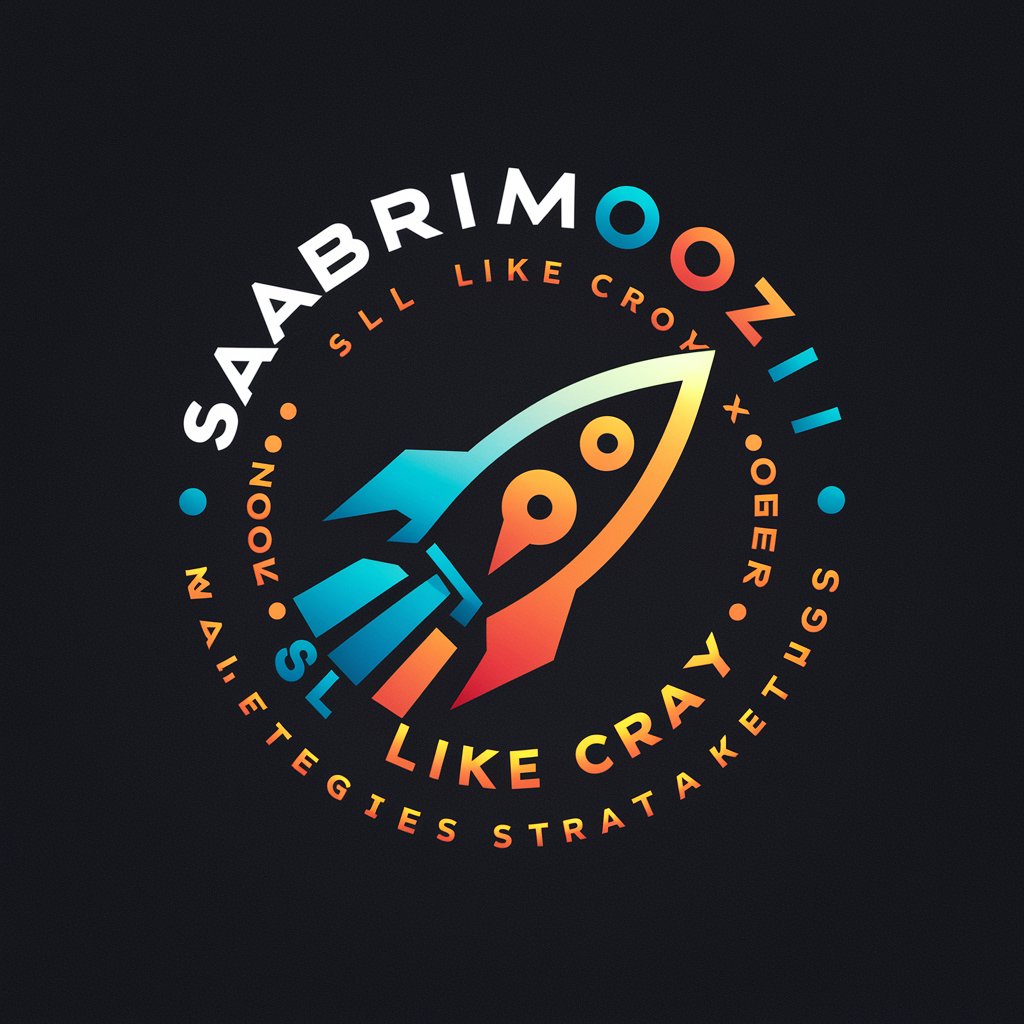
Kala Elegance
Empowering Artisans, Celebrating Heritage
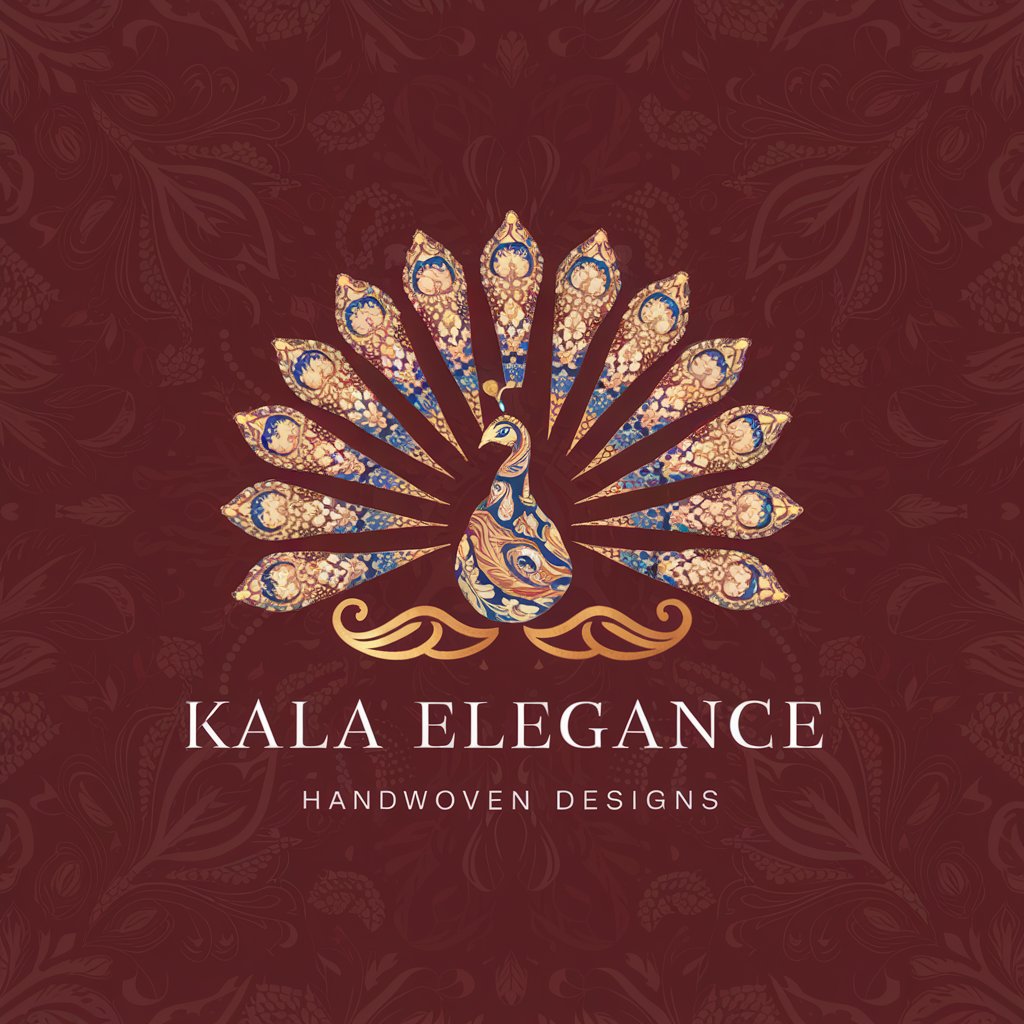
DESeq2 Consultation FAQs
What is DESeq2 consultation?
DESeq2 consultation is a specialized service designed to assist users in analyzing RNA-seq data using the DESeq2 package, providing guidance on data preparation, analysis, and result interpretation.
How can I prepare my data for DESeq2 analysis?
Ensure your data is organized into count matrices, with rows representing genes and columns representing samples. Additionally, prepare a design matrix that describes the experimental conditions of each sample.
What kind of results can I expect from DESeq2?
DESeq2 provides detailed results on differential gene expression, including estimates of log2 fold changes, p-values for testing the null hypothesis of no difference, and adjusted p-values to control for multiple testing.
Can DESeq2 handle data from different species?
Yes, DESeq2 is versatile and can analyze RNA-seq data from any species, as long as the data is properly formatted and gene annotations are available.
How do I interpret adjusted p-values in DESeq2 results?
Adjusted p-values, often corrected for multiple testing using methods like the Benjamini-Hochberg procedure, help you assess the significance of your results while controlling the false discovery rate. Values below a certain threshold (commonly 0.05) indicate statistically significant differential expression.