Data Analysis - AI-Powered Data Analysis
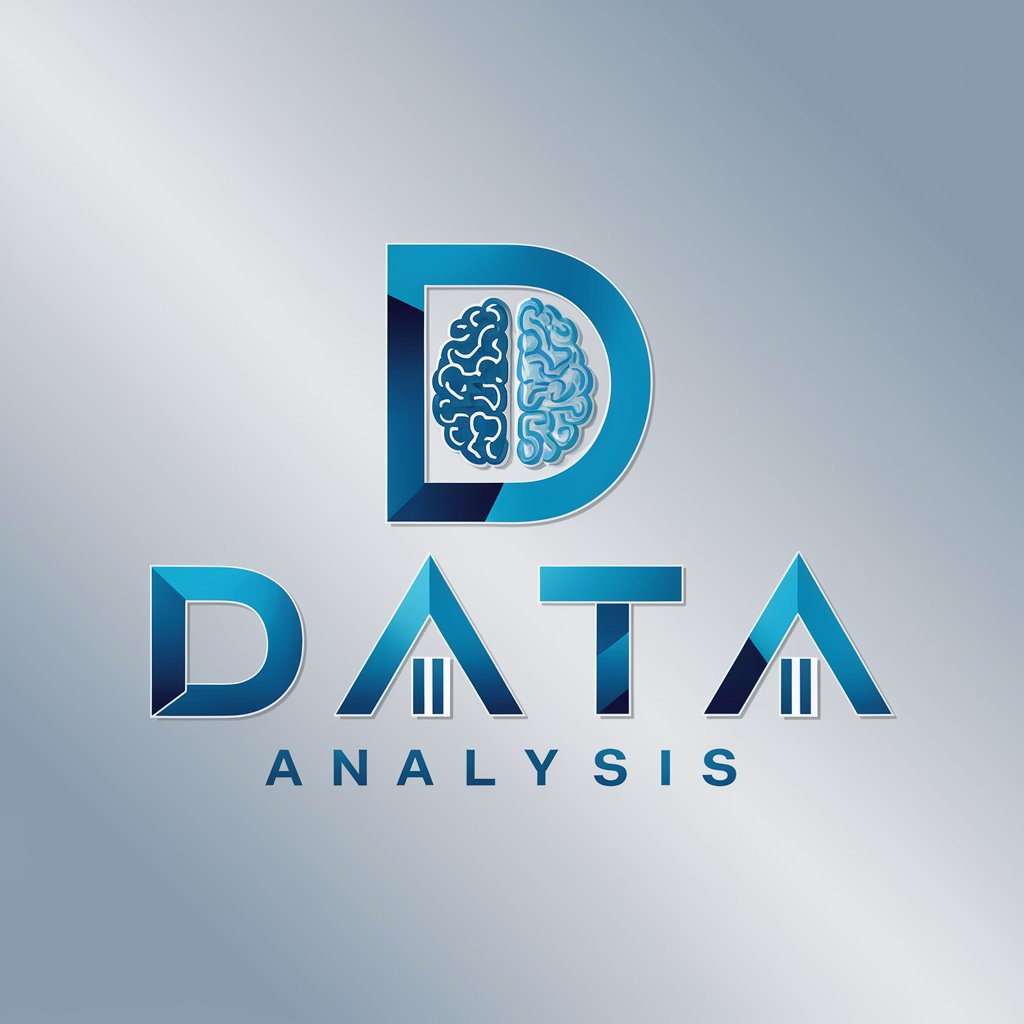
Hello! How can I assist with your data analysis needs today?
Unlock Insights with AI Analysis
Analyze the trends in the latest sales data...
Generate a detailed report on market performance...
Identify key insights from the uploaded dataset...
Summarize the findings from the quarterly financial review...
Get Embed Code
Overview of Data Analysis
Data Analysis refers to the process of examining, cleaning, transforming, and modeling data with the goal of discovering useful information, informing conclusions, and supporting decision-making. This practice is fundamental in various fields including business, science, engineering, and social sciences to make more informed decisions. At its core, data analysis involves taking raw data and transforming it into something more understandable and actionable. This can involve statistical analysis, data visualization, predictive modeling, and data mining among other techniques. For example, a company might analyze customer purchase data to identify buying patterns and predict future sales trends. Similarly, in healthcare, data analysis can be used to examine patient records to improve treatment plans and outcomes. Powered by ChatGPT-4o。
Key Functions of Data Analysis
Statistical Analysis
Example
Calculating mean, median, mode, and standard deviation to understand data distribution.
Scenario
A market researcher analyzing survey responses to gauge customer satisfaction levels across different demographics.
Data Visualization
Example
Creating charts, graphs, and maps to represent data visually.
Scenario
A business analyst using dashboards to present monthly sales data and highlight trends and outliers to stakeholders.
Predictive Modeling
Example
Using historical data to predict future outcomes using statistical algorithms and machine learning.
Scenario
A financial analyst forecasting stock market trends to advise on investment strategies.
Data Mining
Example
Extracting patterns from large data sets using machine learning, statistics, and database systems.
Scenario
An e-commerce company analyzing transaction data to identify up-selling and cross-selling opportunities.
Data Cleaning
Example
Identifying and correcting errors or inconsistencies in data to improve data quality.
Scenario
A data scientist preprocessing a dataset for analysis by removing duplicates, filling missing values, and correcting errors.
Target User Groups for Data Analysis Services
Business Analysts
Professionals who analyze data to produce actionable insights for business decisions, benefiting from data visualization and predictive modeling to optimize operations and increase profitability.
Data Scientists
Individuals skilled in statistical analysis and machine learning, using data cleaning, data mining, and predictive modeling to develop sophisticated models that drive innovation and efficiency in various sectors.
Healthcare Professionals
Medical researchers and practitioners leveraging data analysis to improve patient care outcomes through predictive analytics and research studies, enhancing treatment plans and healthcare services.
Marketing Professionals
Experts in crafting and optimizing marketing strategies based on customer data analysis, using statistical analysis and data visualization to understand market trends and consumer behavior.
Financial Analysts
Specialists in analyzing financial data to make investment recommendations, benefit from predictive modeling and statistical analysis to forecast market trends and assess risk.
Guidelines for Using Data Analysis
Initiate Your Journey
Start by accessing yeschat.ai for a complimentary trial, bypassing the need for login or ChatGPT Plus subscription.
Identify Your Needs
Determine the specific data analysis goals you have, such as predictive analytics, trend analysis, or data visualization to ensure the tool's features align with your requirements.
Prepare Your Data
Ensure your data is clean, organized, and ready for analysis. This may involve preprocessing steps such as removing duplicates, handling missing values, and ensuring data is in a compatible format.
Engage with the Tool
Utilize the tool's features to perform your analysis. This could include running queries, applying statistical models, or generating visualizations to interpret your data effectively.
Analyze and Interpret Results
Carefully review the outputs provided by the tool. Apply critical thinking to interpret the results, draw conclusions, and make informed decisions based on the data analysis.
Try other advanced and practical GPTs
Customer Service GPT
Empowering Support with AI
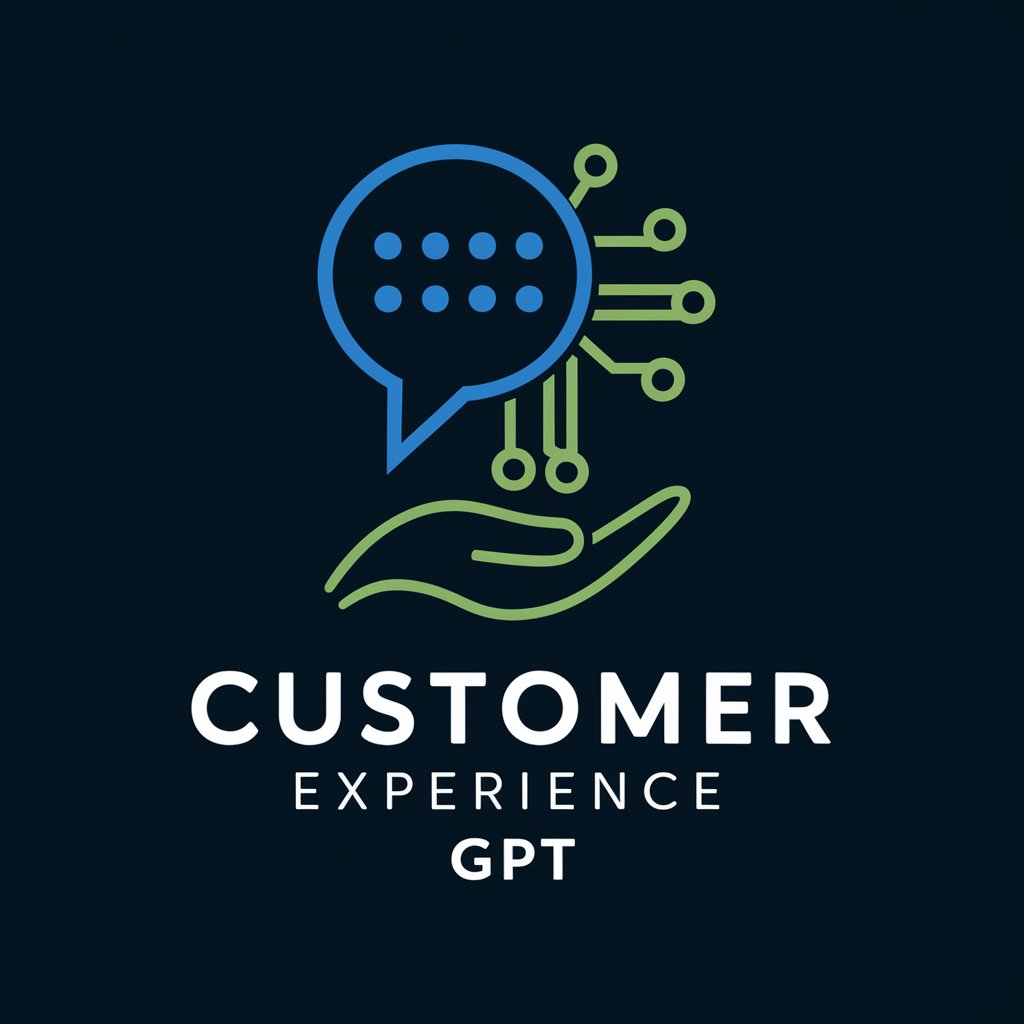
Diffusion Master
Transforming Words into Visual Art
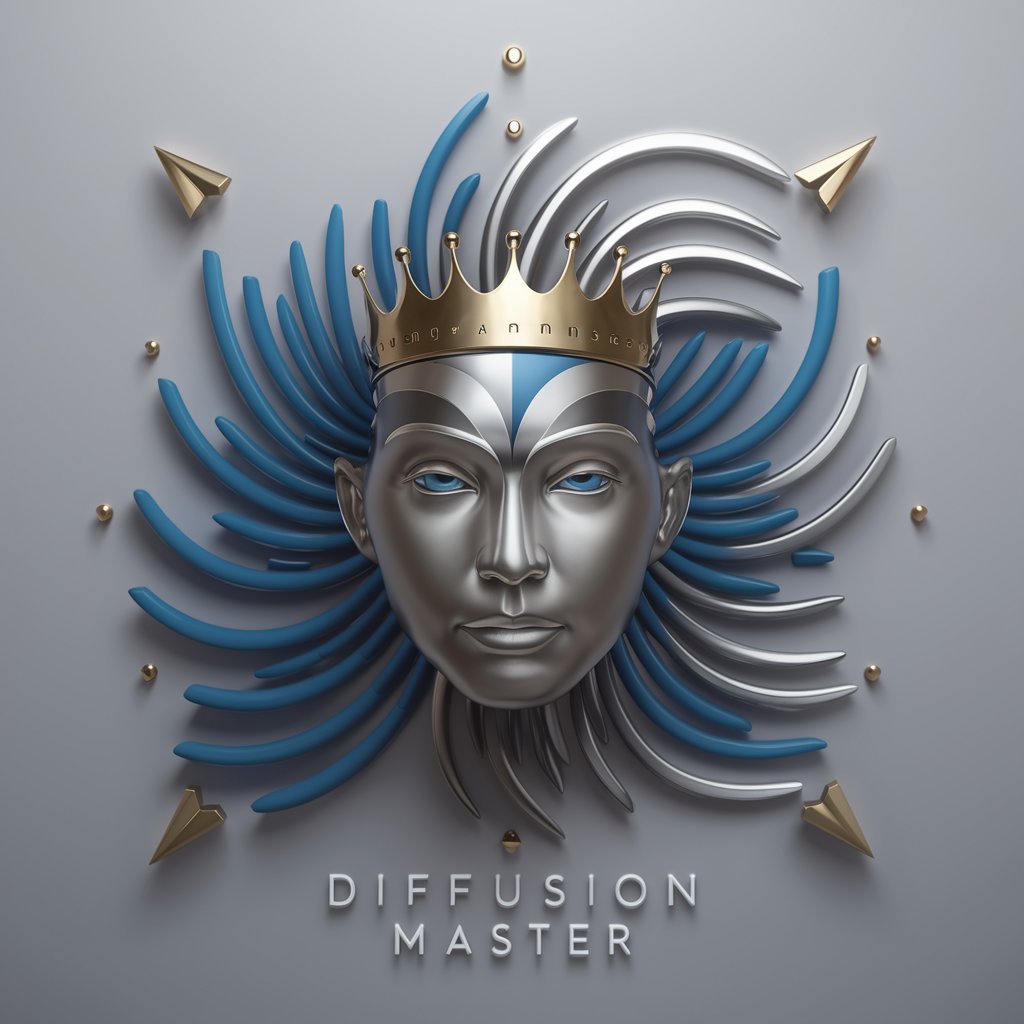
DomainsGPT
Crafting Your Online Identity with AI
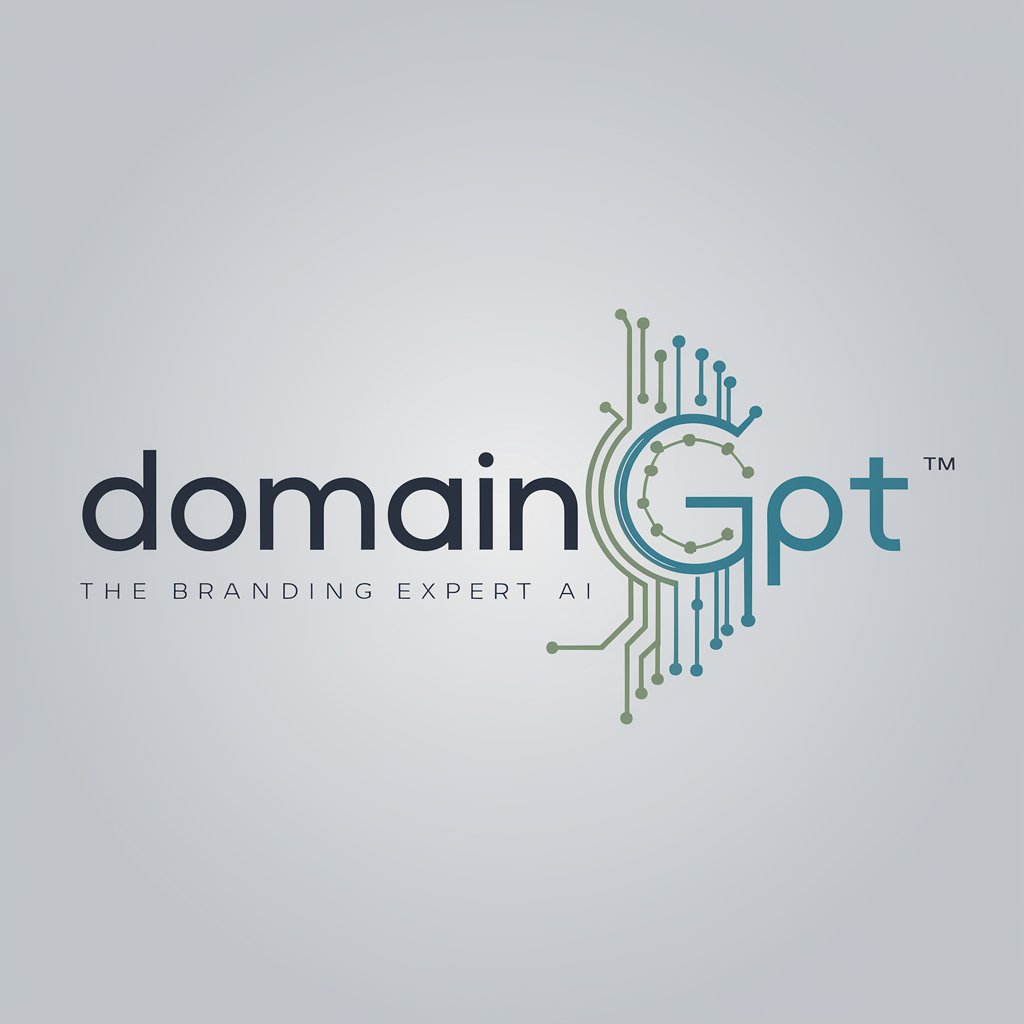
Dream Labyrinth
Navigate your dreams with AI.
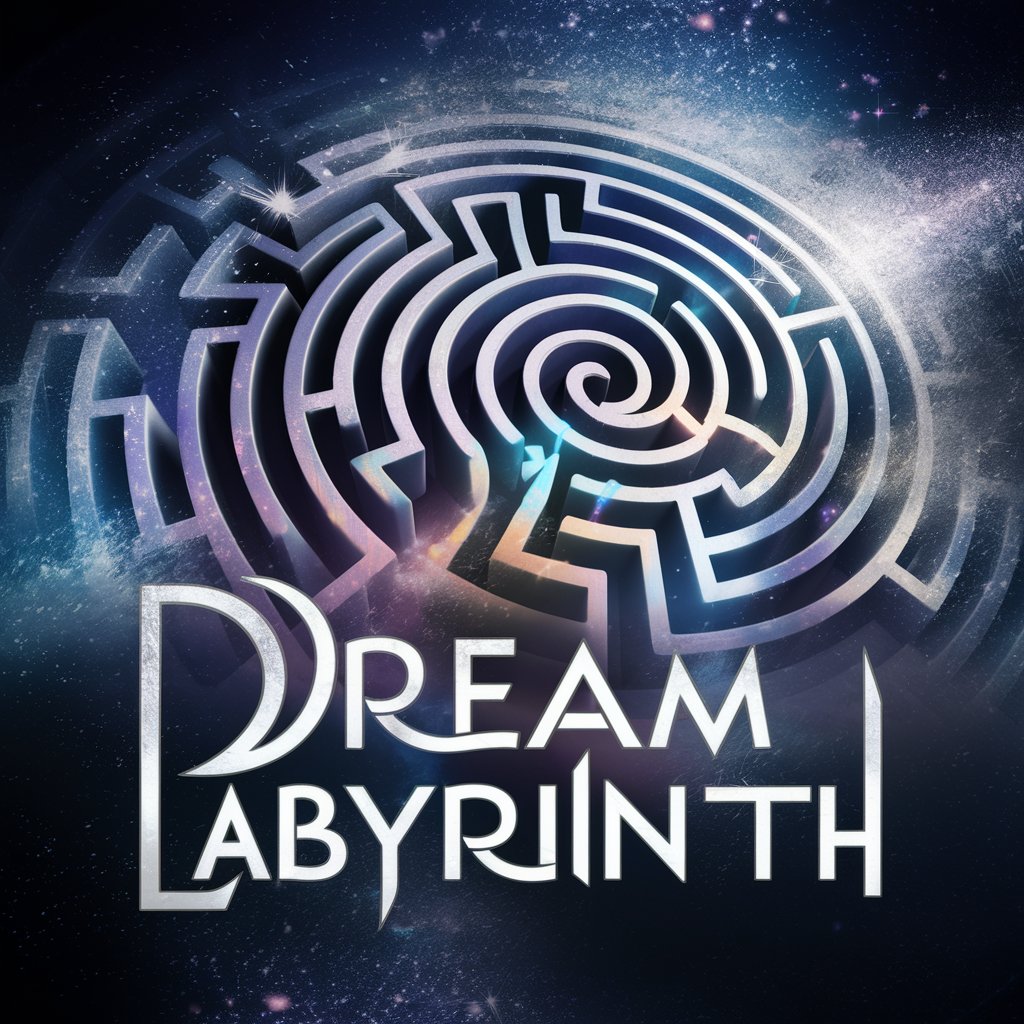
Email Responder Pro
Crafting Professional Replies Instantly
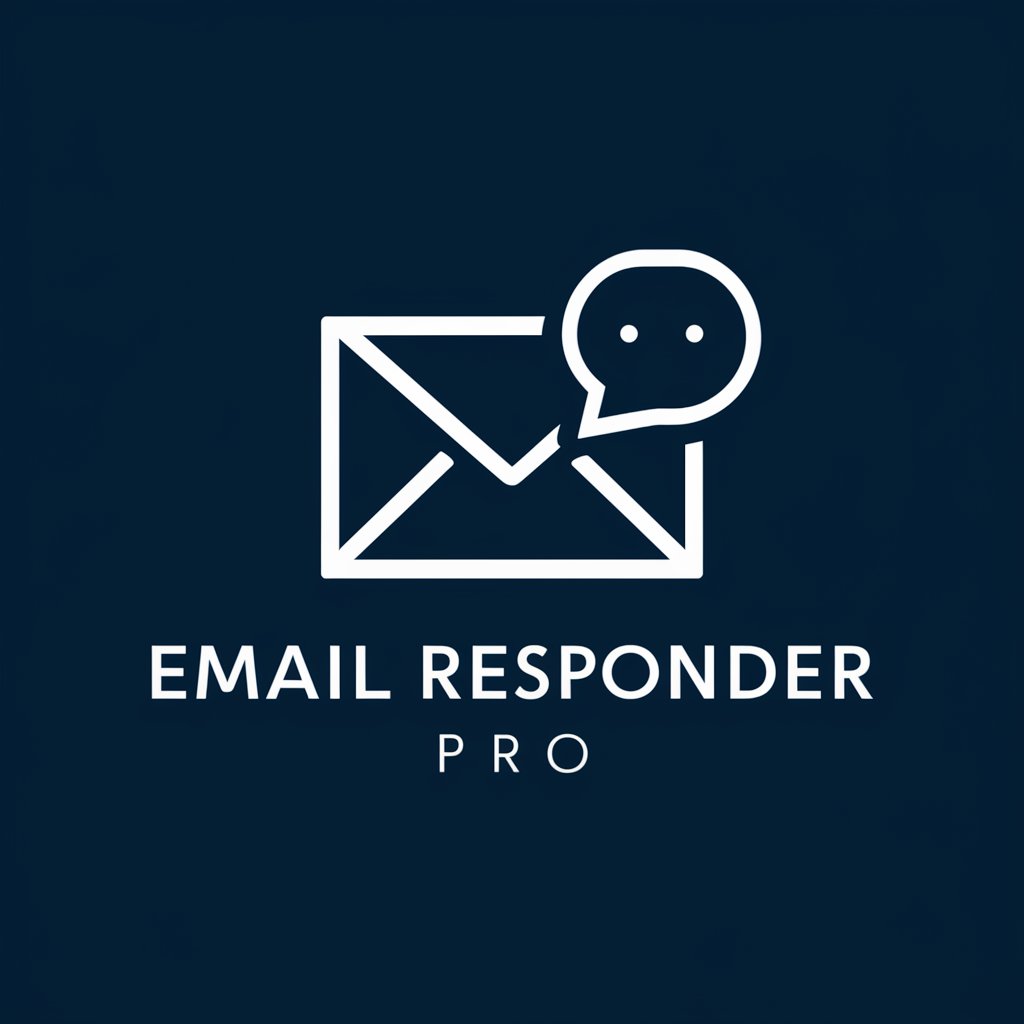
EmojAI
Transform Text into Emojis with AI
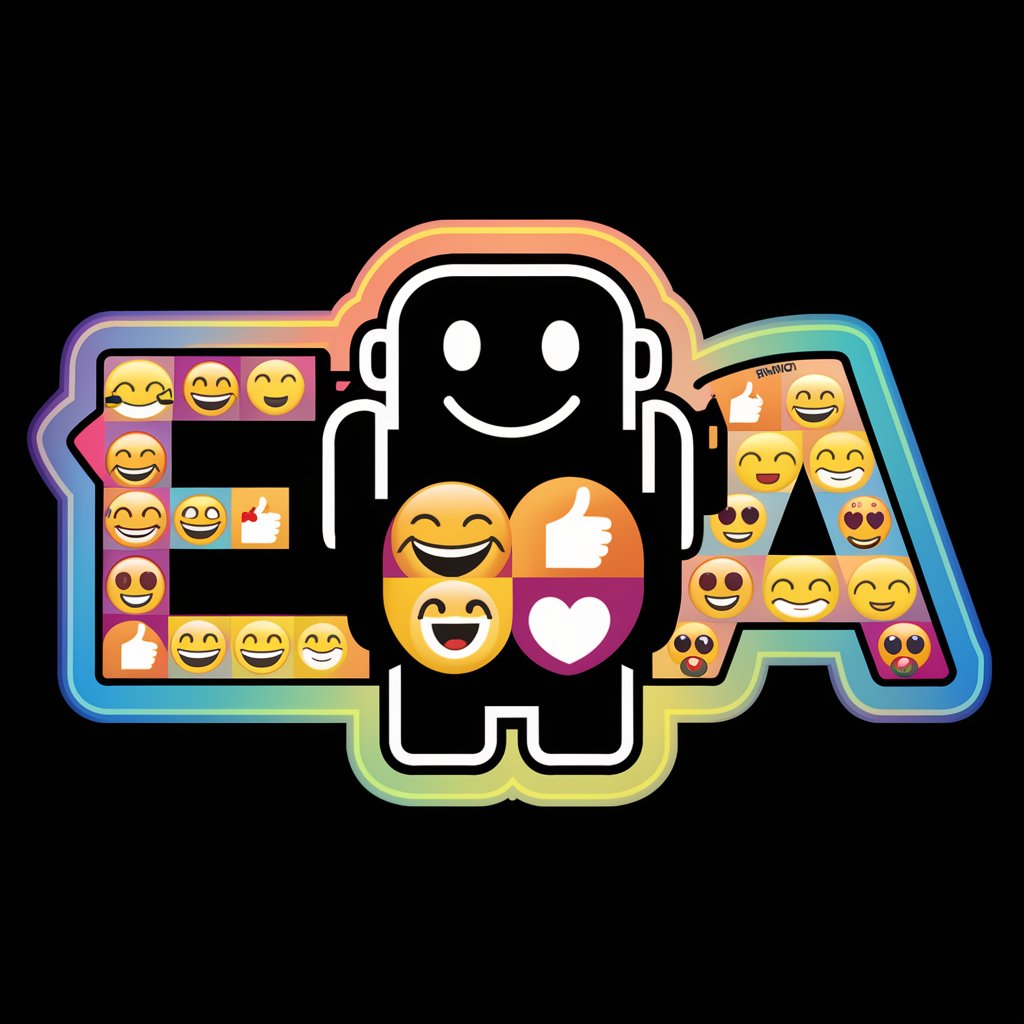
TextNinja
Empower your text with AI-driven insights
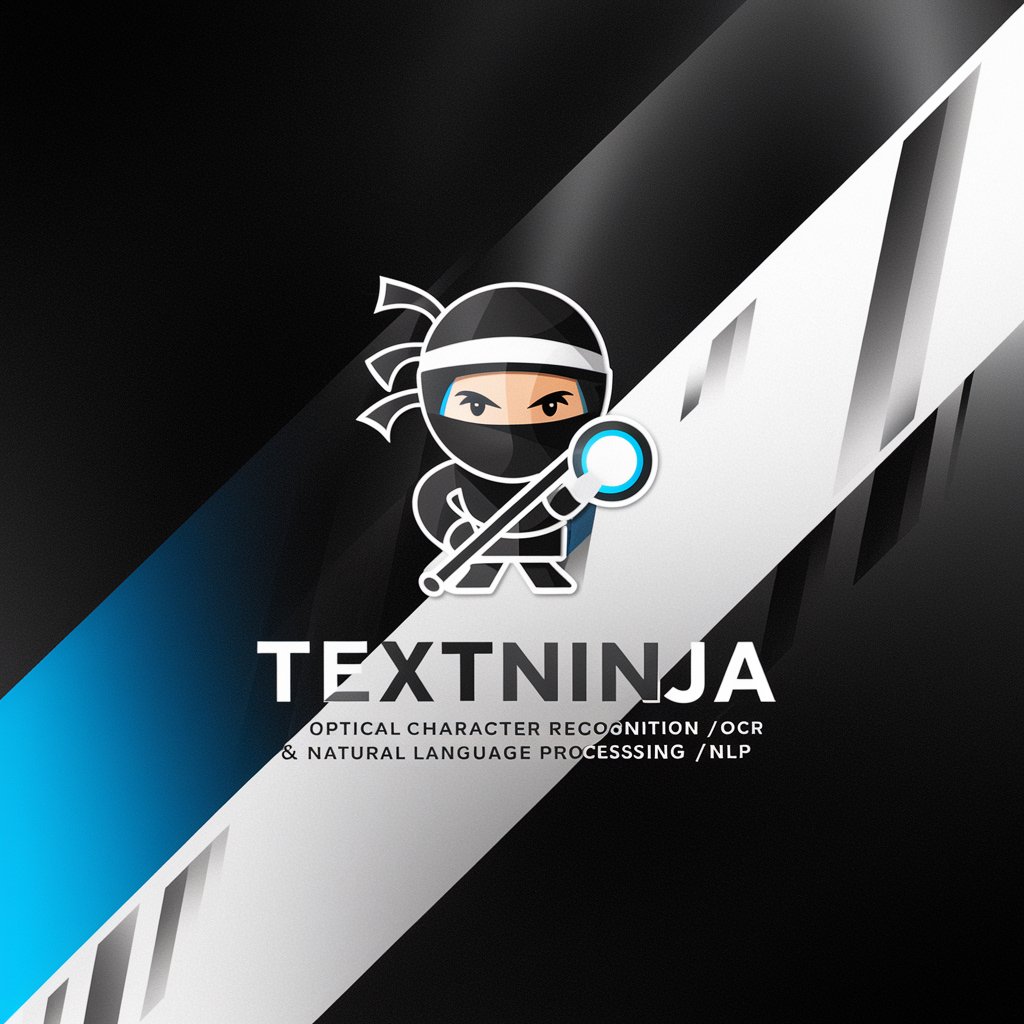
logoNinja
Empower Your Brand with AI-Driven Design
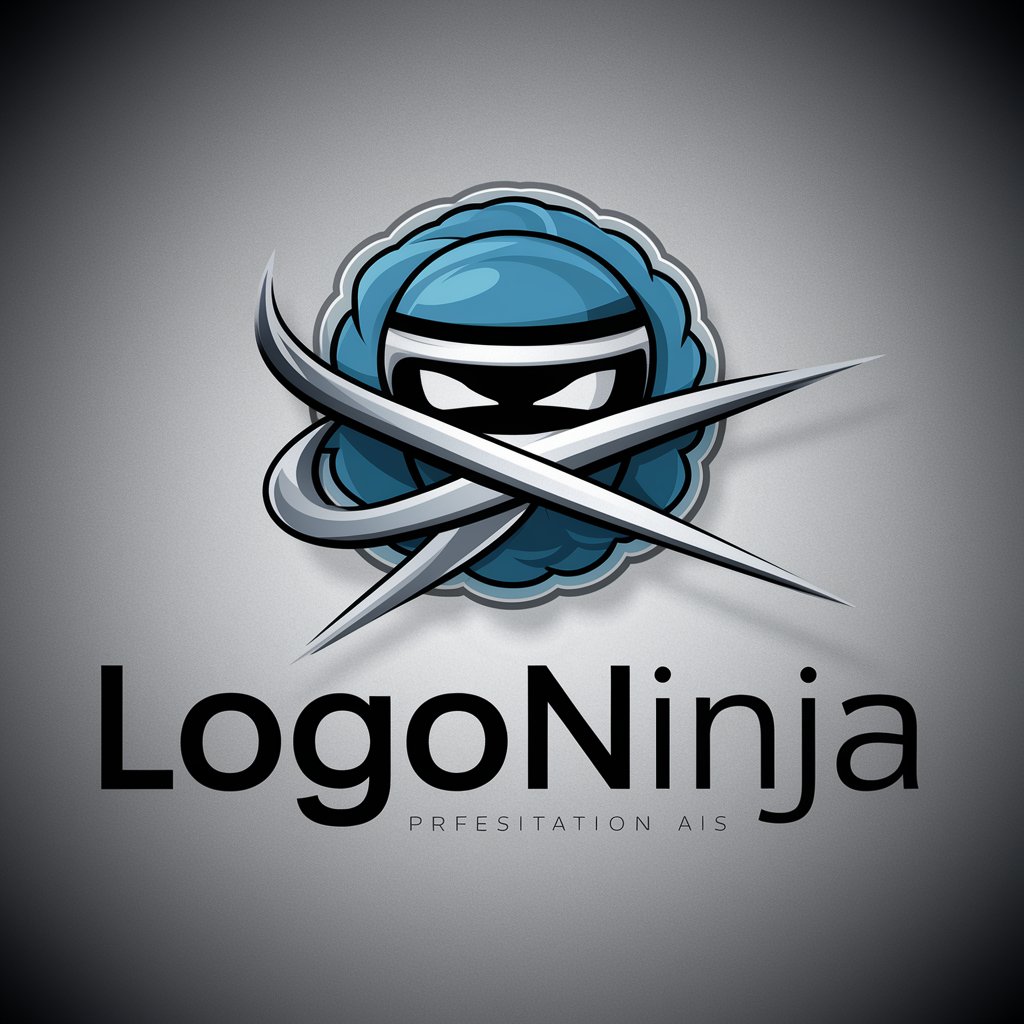
Anetha (Investment Analyst)
Empowering Your Investment Decisions with AI
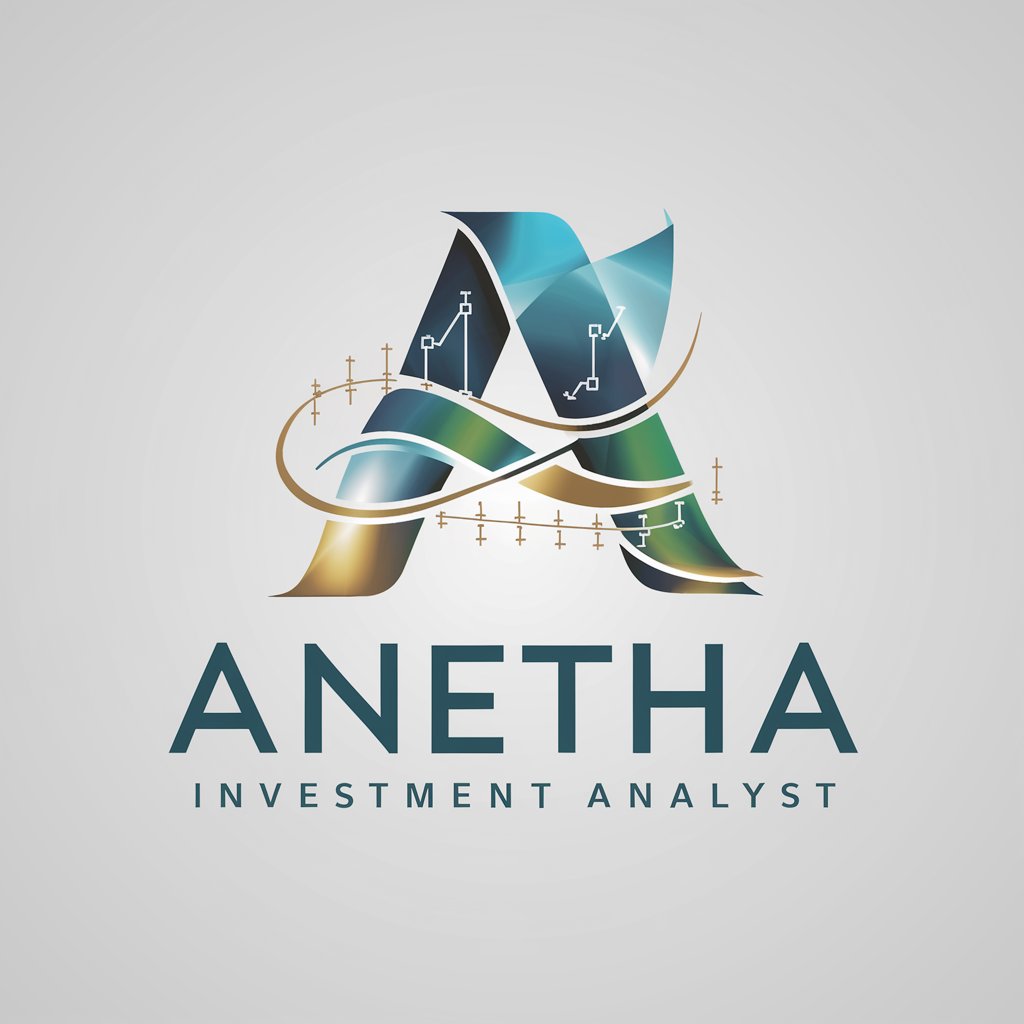
Executive Assistant
AI-powered Professional Productivity Boost
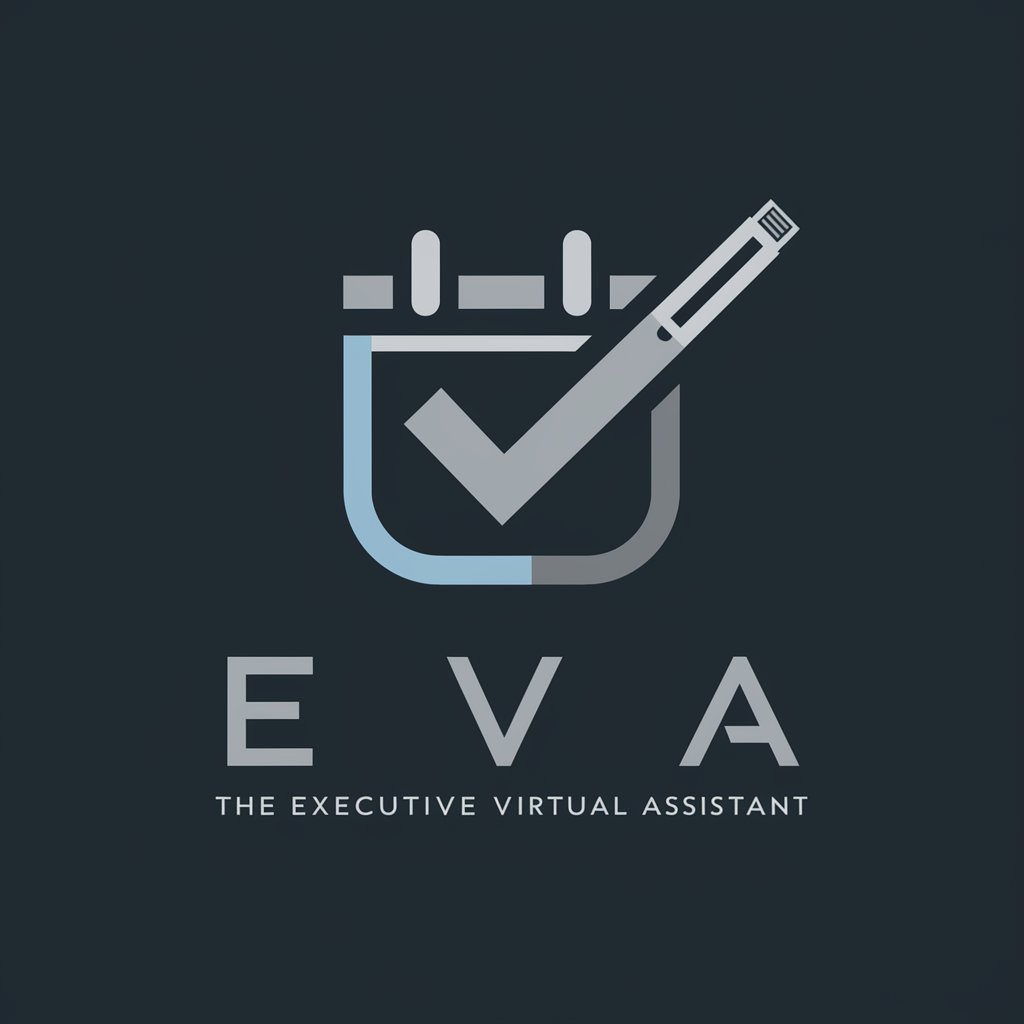
Stock Image keywords & info generator
Elevate Your Images with AI-Powered SEO
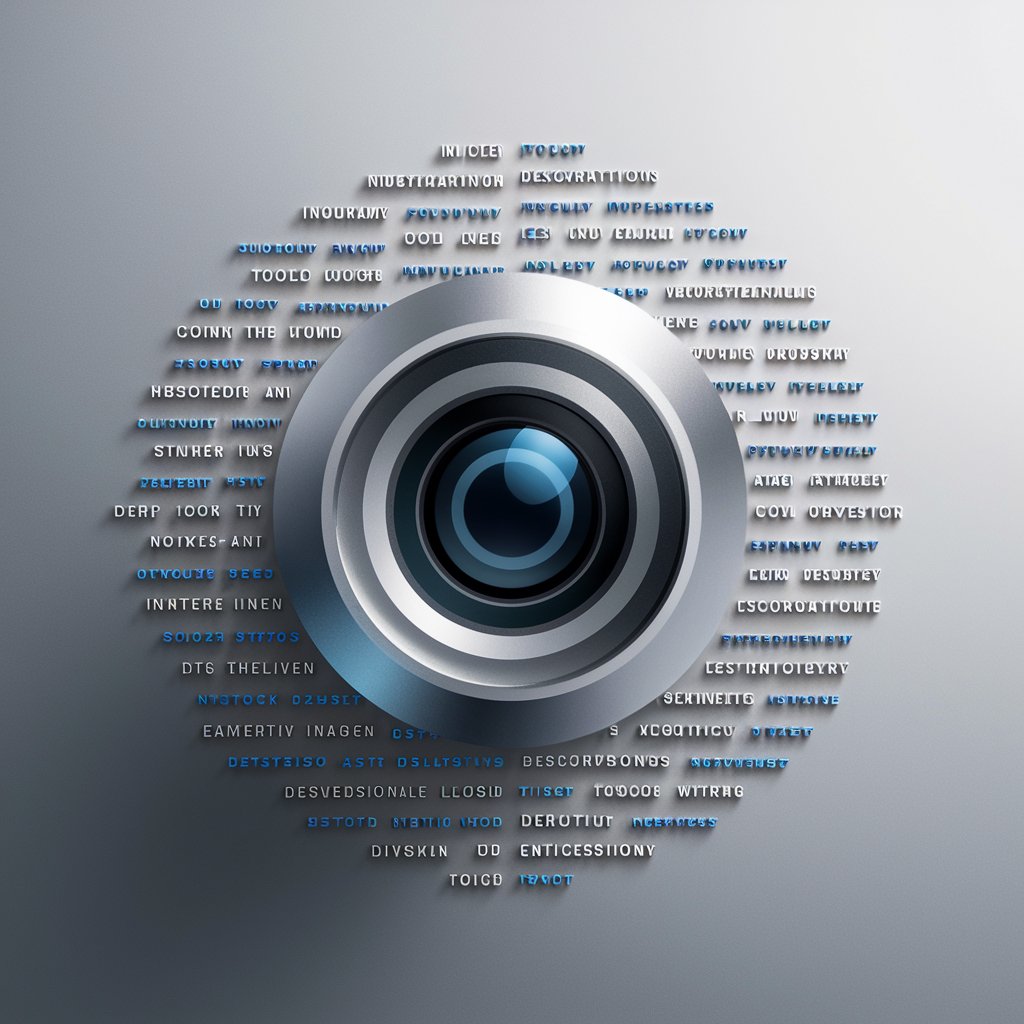
JobGPT - Find Jobs worldwide
Empower Your Career with AI
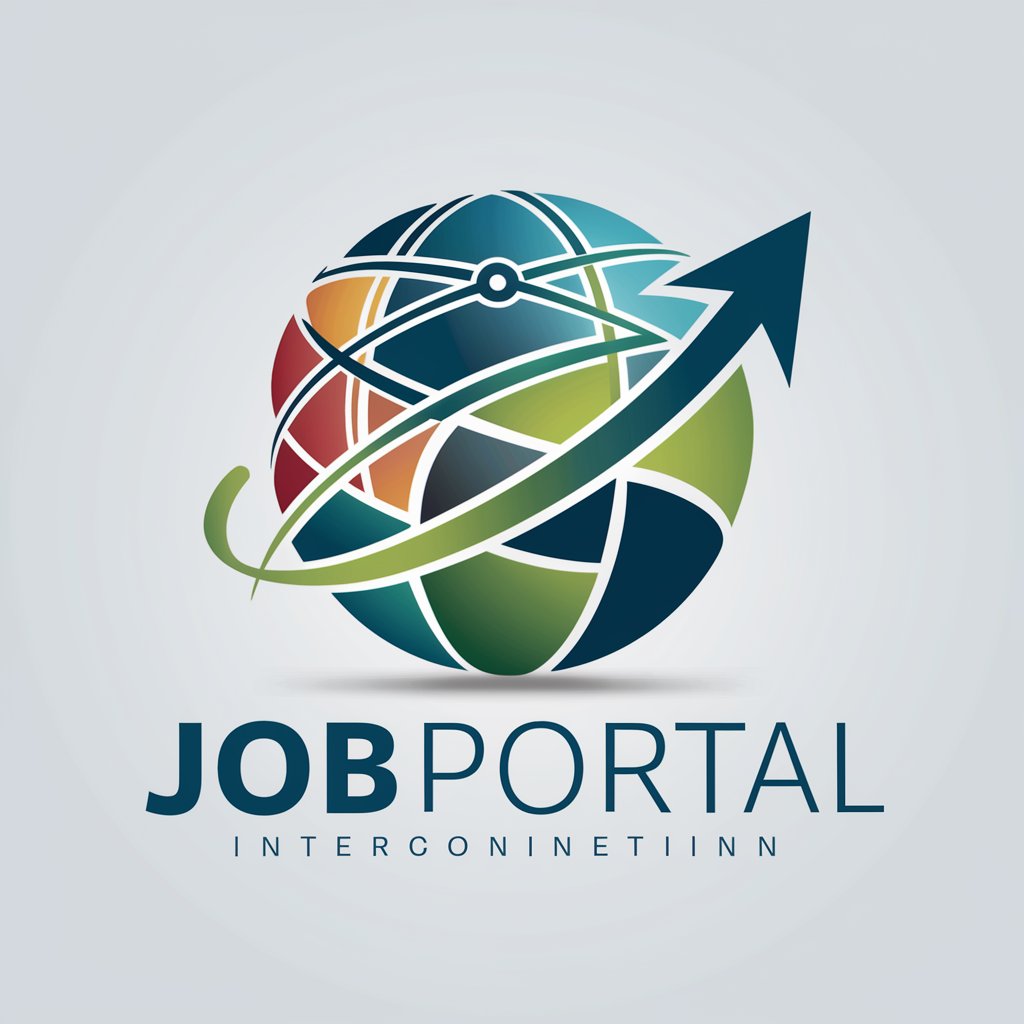
Detailed Q&A about Data Analysis
What types of data can be analyzed with this tool?
This tool is versatile in handling various types of data, including structured data like Excel spreadsheets, unstructured data like text documents, and semi-structured data like JSON files. It's designed to accommodate a wide range of data analysis needs.
Can I use this tool for predictive analytics?
Yes, the tool is equipped with machine learning capabilities that allow for predictive analytics. You can train models on your historical data to forecast future trends, behaviors, and outcomes.
How does the tool ensure data privacy and security?
Data privacy and security are paramount. The tool employs encryption for data at rest and in transit, along with strict access controls and compliance with privacy regulations to protect your data.
Is there support for real-time data analysis?
Yes, the tool supports real-time data analysis, enabling you to stream data directly into the platform and receive immediate insights and visualizations, which is crucial for time-sensitive decisions.
How can I share my analysis results with colleagues?
The tool offers various sharing and collaboration features. You can share reports and dashboards directly within the tool, export your findings in multiple formats, or even embed visualizations in web pages or applications for broader dissemination.