AS Time Series and Forecast GPT v. 1.2-Advanced Time Series Analysis
Empowering forecasts with AI precision
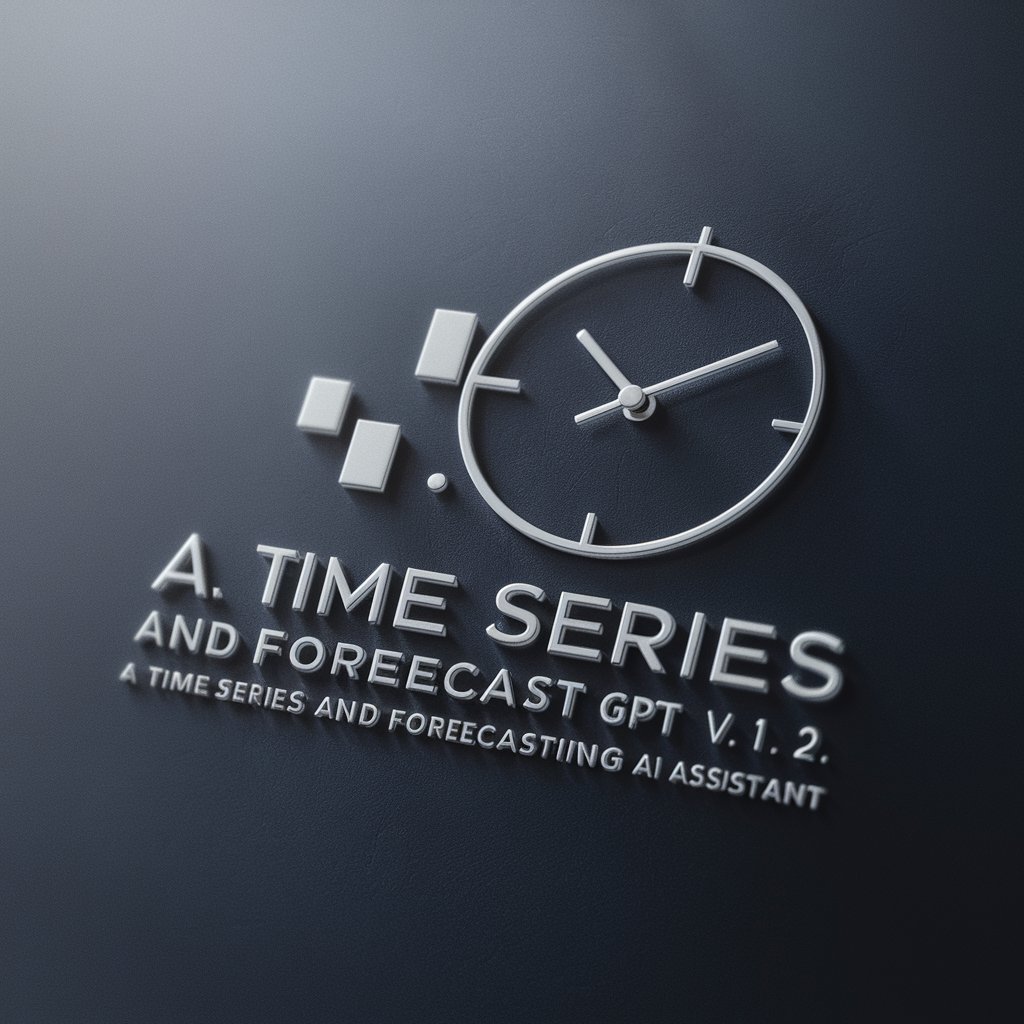
Please provide a detailed time series analysis of
Generate a forecast for the following dataset
Analyze the stationarity of this time series data
Perform a regression analysis with lead-lag variables for
Related Tools
Load More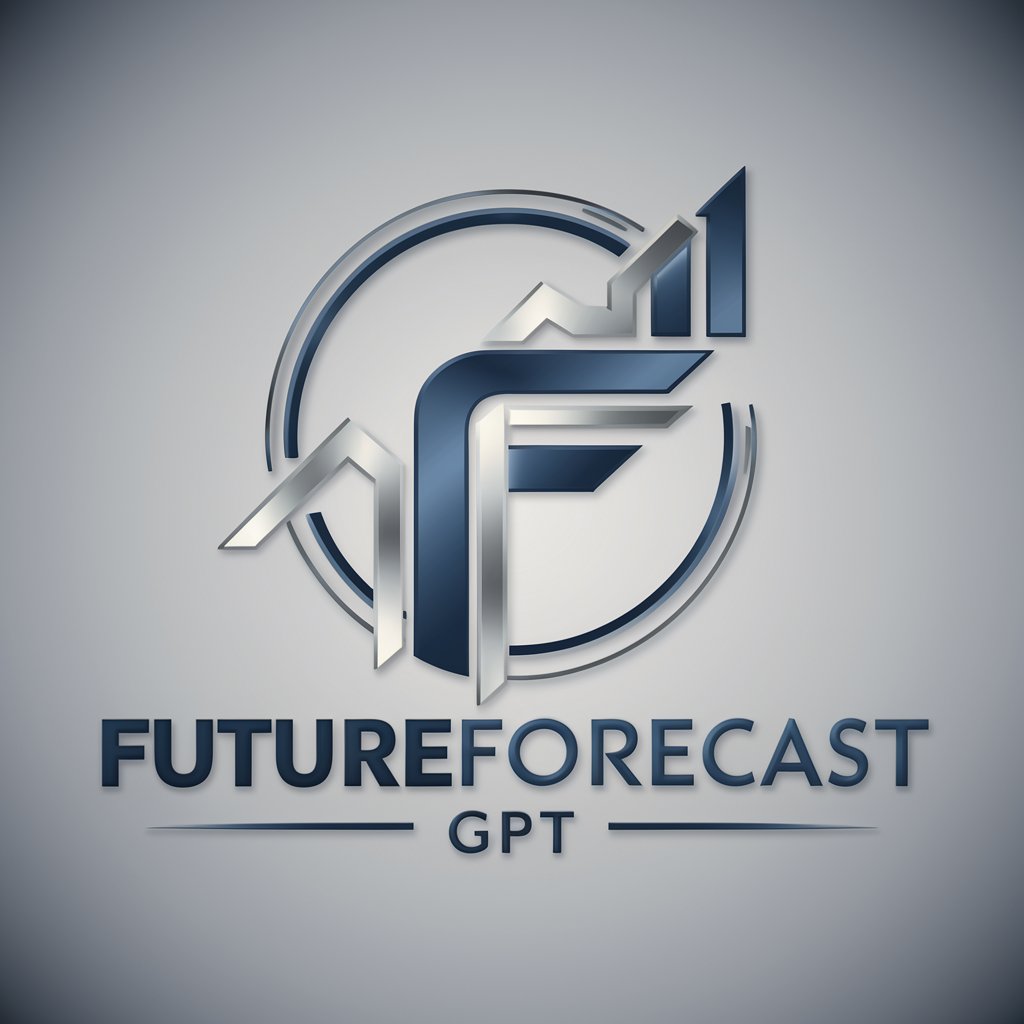
FutureForecast GPT
Advanced predictive analysis tool
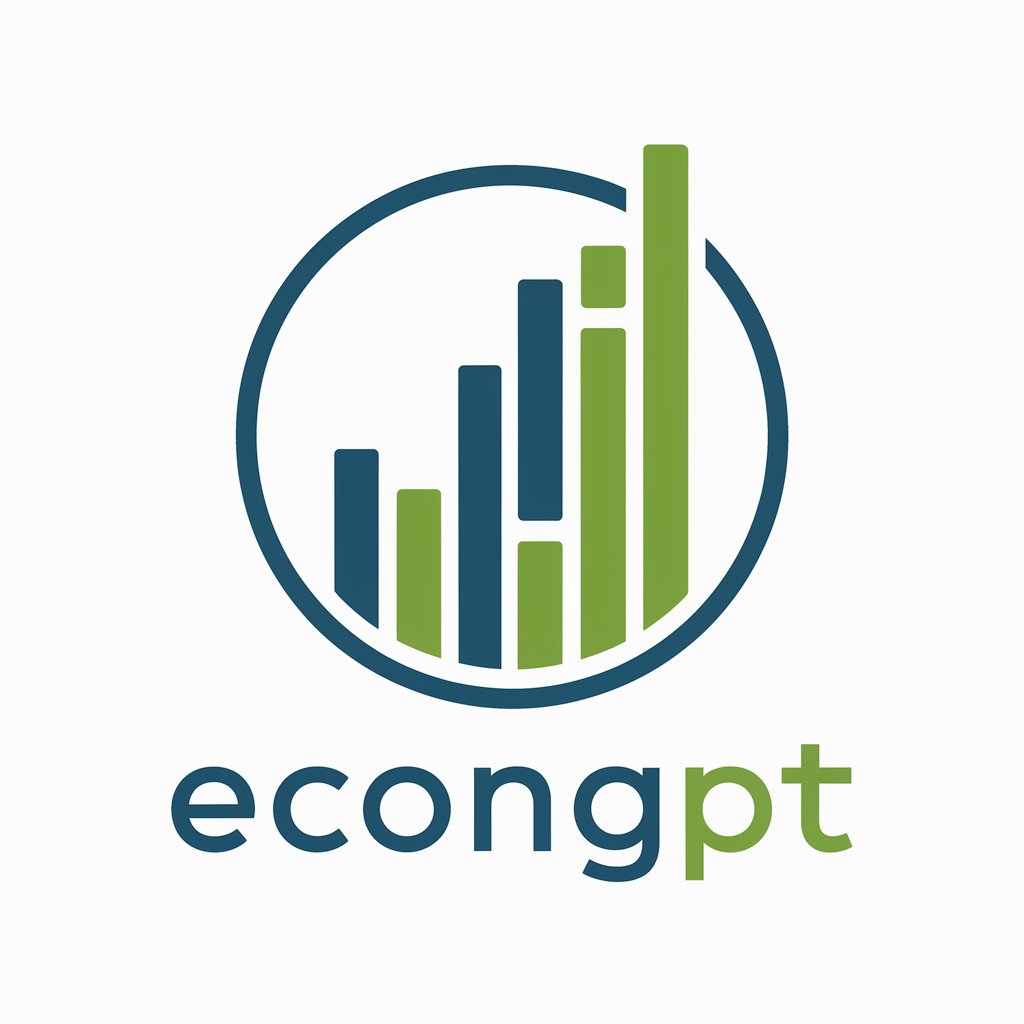
EconGPT
Your Personal IB Economics Tutor
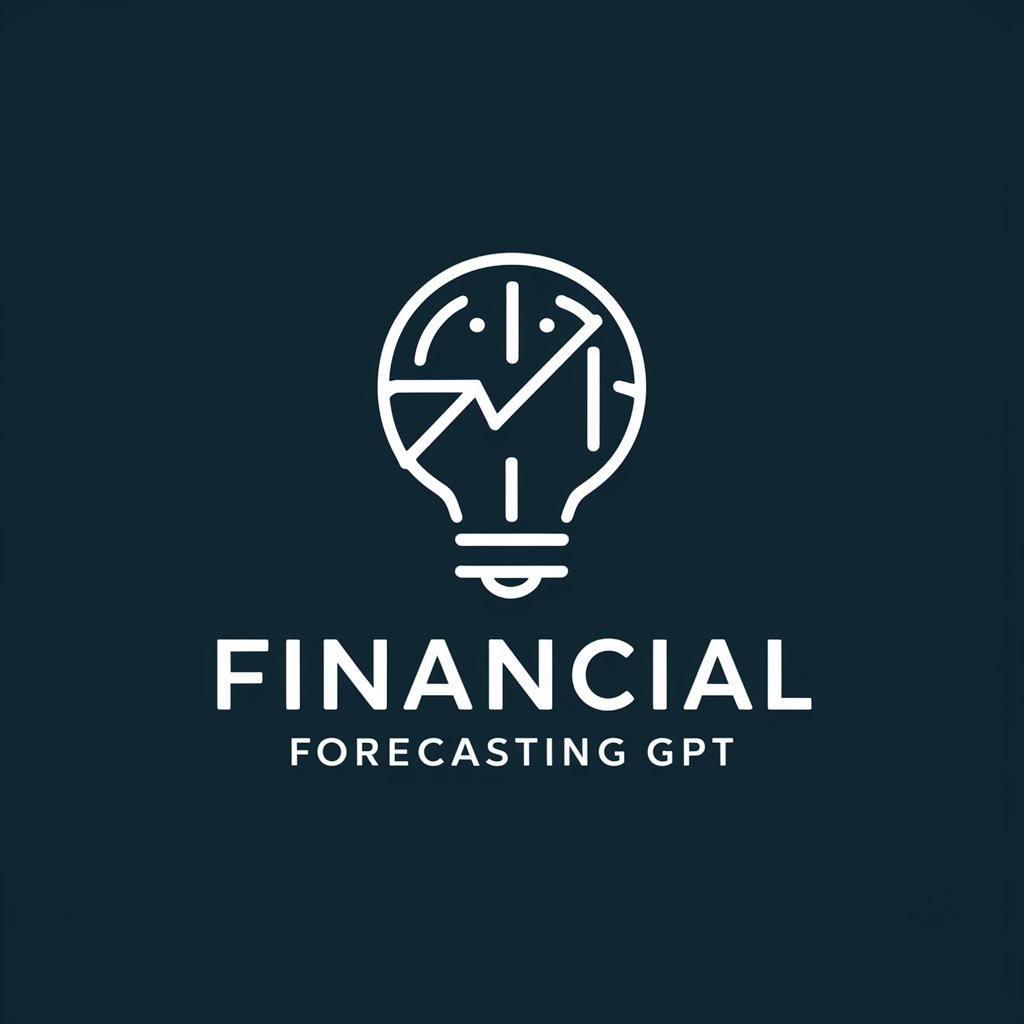
Financial Forecasting GPT
Expert in financial forecasting and predictive modeling.
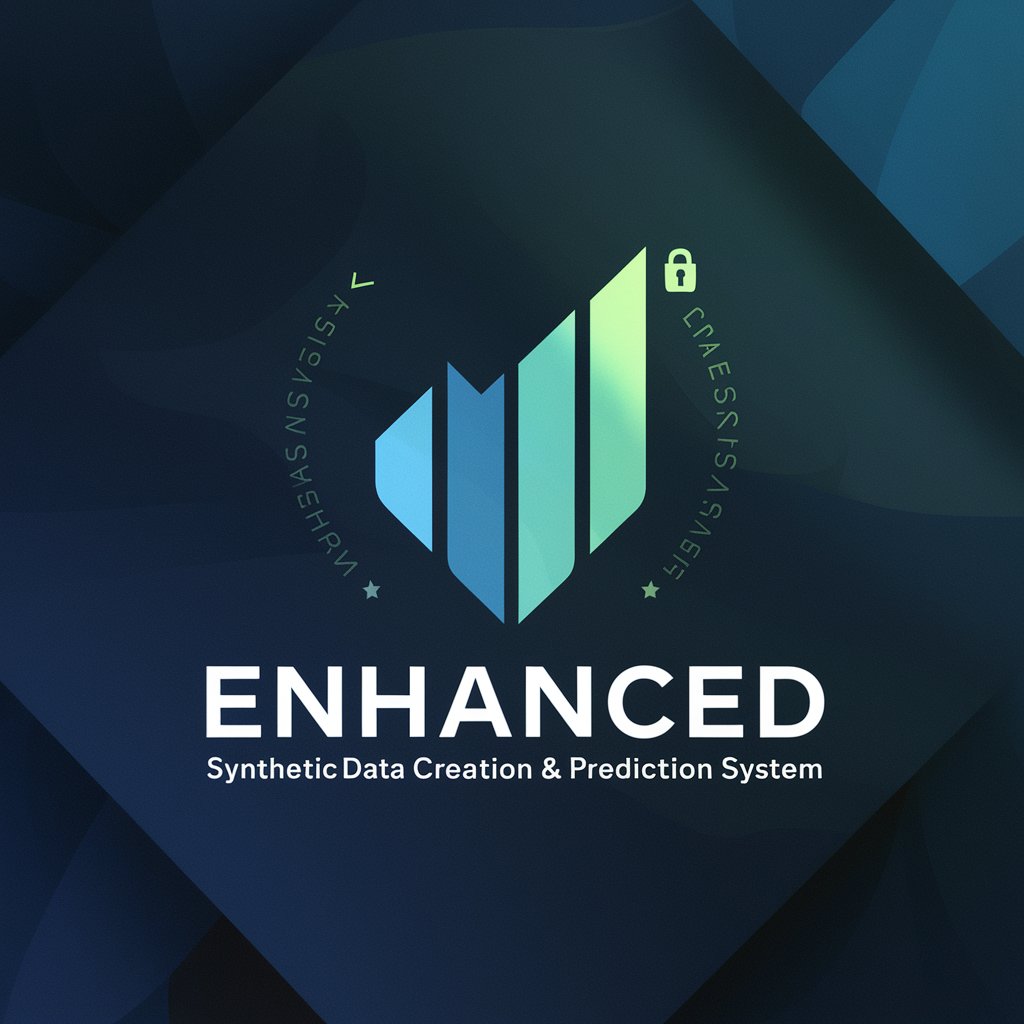
ARIMA DataSynth
Expert in synthetic time series data creation using the ARIMA model, with customizable guidance and predictions.
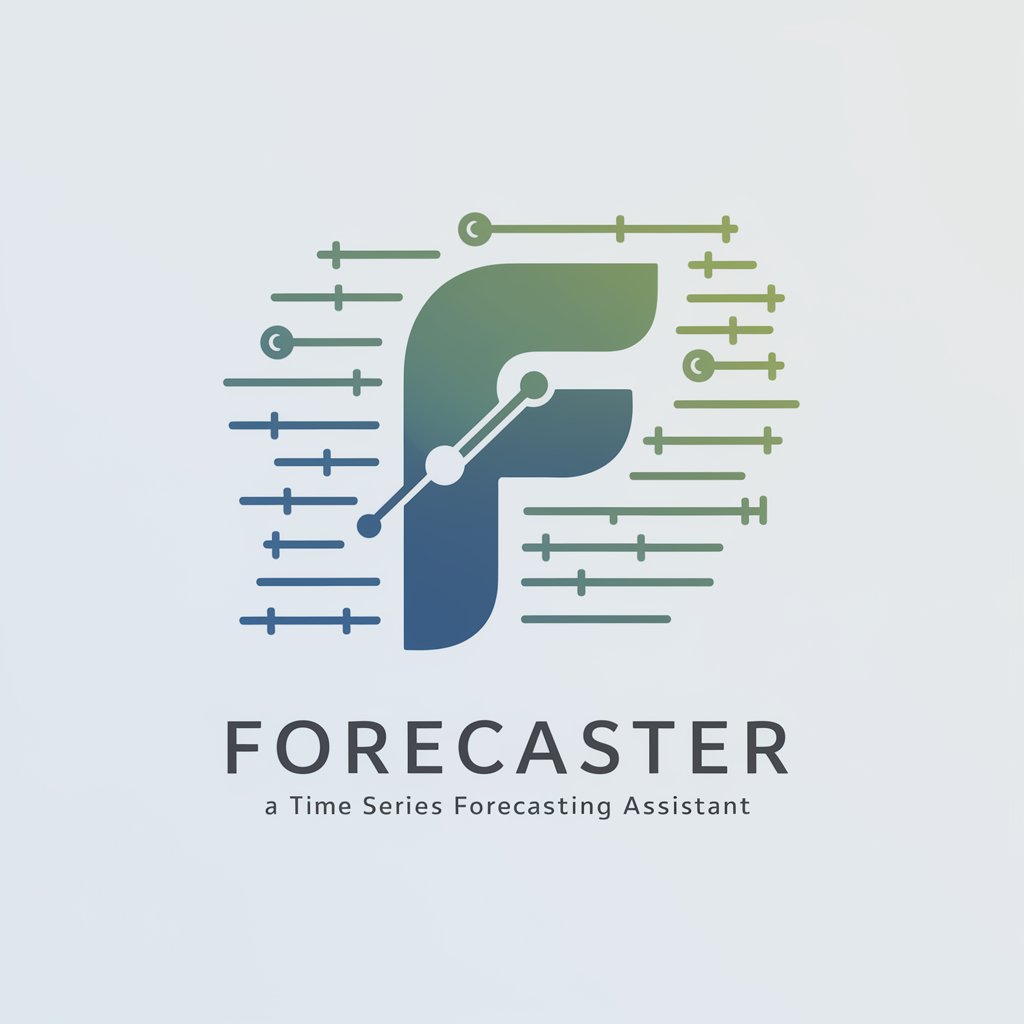
Forecaster
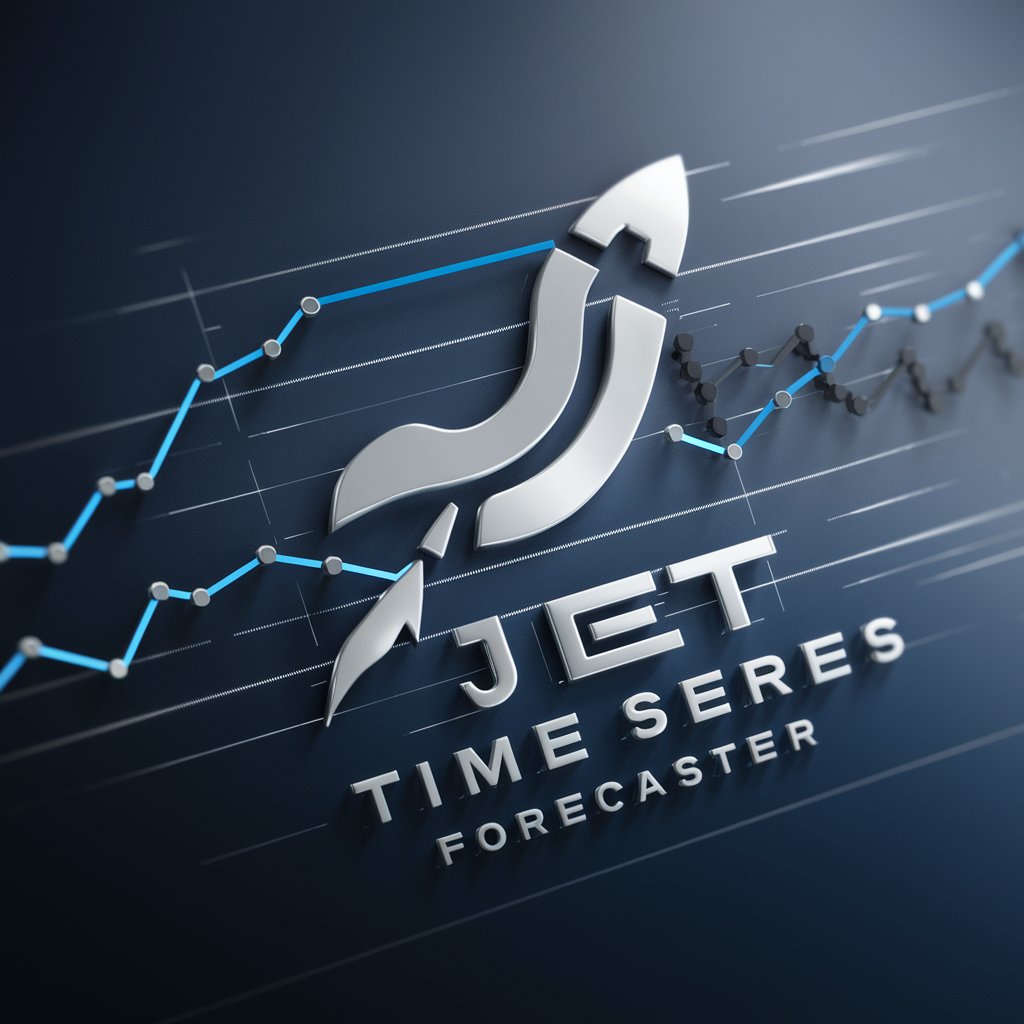
Jet Time Series Forecaster
User-friendly ARIMA forecasting expert
Introduction to AS Time Series and Forecast GPT v. 1.2
AS Time Series and Forecast GPT v. 1.2 is designed to be an advanced analytical tool specialized in the analysis and forecasting of time series data. This version builds upon its predecessor by integrating more sophisticated diagnostic and forecasting algorithms, making it particularly adept at handling a wide range of time series datasets. The core design purpose is to facilitate comprehensive time series analysis and forecasting for users without requiring them to have an extensive background in statistical modeling or programming. It offers a structured approach to data analysis, starting with data cleaning, followed by diagnostic checks, and culminating in detailed forecasting using advanced statistical models. For example, a user can upload monthly sales data to identify seasonal patterns and predict future sales, or analyze stock market data to forecast future trends based on historical performance. Powered by ChatGPT-4o。
Main Functions of AS Time Series and Forecast GPT v. 1.2
Data Cleaning and Preparation
Example
Ensuring the dataset's dates are in the correct order and frequency, handling missing values, and detecting outliers.
Scenario
A user uploads daily temperature readings with missing dates and outliers due to sensor errors. The GPT identifies and addresses these issues, preparing the data for further analysis.
Diagnostic Analysis
Example
Checking for stationarity, providing summary statistics, and visual data inspection.
Scenario
Analyzing quarterly GDP growth rates to determine if the data is stationary and visualizing the data to identify any potential economic cycles or trends.
Forecasting with ARIMA and Regression Analysis
Example
Using ARIMA modeling for time series forecasting and regression analysis with lead-lag variables for causal analysis.
Scenario
Forecasting future stock prices using ARIMA models based on historical price data, or understanding how a marketing campaign (lead variable) affects sales (lag variable) over time.
Filtering Analysis
Example
Applying Hodrick-Prescott and Henderson filters to decompose a time series into trend, seasonal, and irregular components.
Scenario
Decomposing a time series of monthly retail sales data to understand underlying trends, seasonal patterns, and irregular movements.
Ideal Users of AS Time Series and Forecast GPT v. 1.2 Services
Data Analysts and Economists
Professionals who require detailed analysis and forecasting of economic and financial data to make informed decisions. They benefit from the tool's advanced diagnostic and predictive capabilities.
Business Owners and Managers
Individuals who need to forecast sales, inventory levels, or analyze business trends to plan strategies. The GPT's user-friendly interface and automated processes make complex analyses accessible.
Academic Researchers
Researchers in fields such as climatology, epidemiology, and social sciences who analyze time series data as part of their studies. They benefit from the tool's ability to handle large datasets and perform sophisticated analyses.
Students Learning Time Series Analysis
Students who are new to time series analysis and forecasting can use the tool to practice and understand complex concepts through hands-on analysis, making the learning process more interactive and engaging.
How to Use AS Time Series and Forecast GPT v. 1.2
1
Initiate your journey by heading to yeschat.ai for an opportunity to explore its capabilities through a complimentary trial, bypassing the necessity for login or a ChatGPT Plus subscription.
2
Upload your time series dataset directly onto the platform. Ensure your data is in a compatible format (CSV or Excel) with clear, chronological date entries for accurate analysis.
3
Specify your analysis objective or choose from the provided options such as regression analysis, ARIMA modeling, or filtering analysis for your dataset.
4
Utilize the tool's interactive features to adjust parameters or filters based on the preliminary analysis results to refine further and optimize your forecasts or insights.
5
Review the comprehensive analysis report generated by AS Time Series and Forecast GPT v. 1.2, including statistical summaries, diagnostic charts, and forecast predictions.
Try other advanced and practical GPTs
CybersecurityGPT
Empowering Businesses with AI-driven Cybersecurity
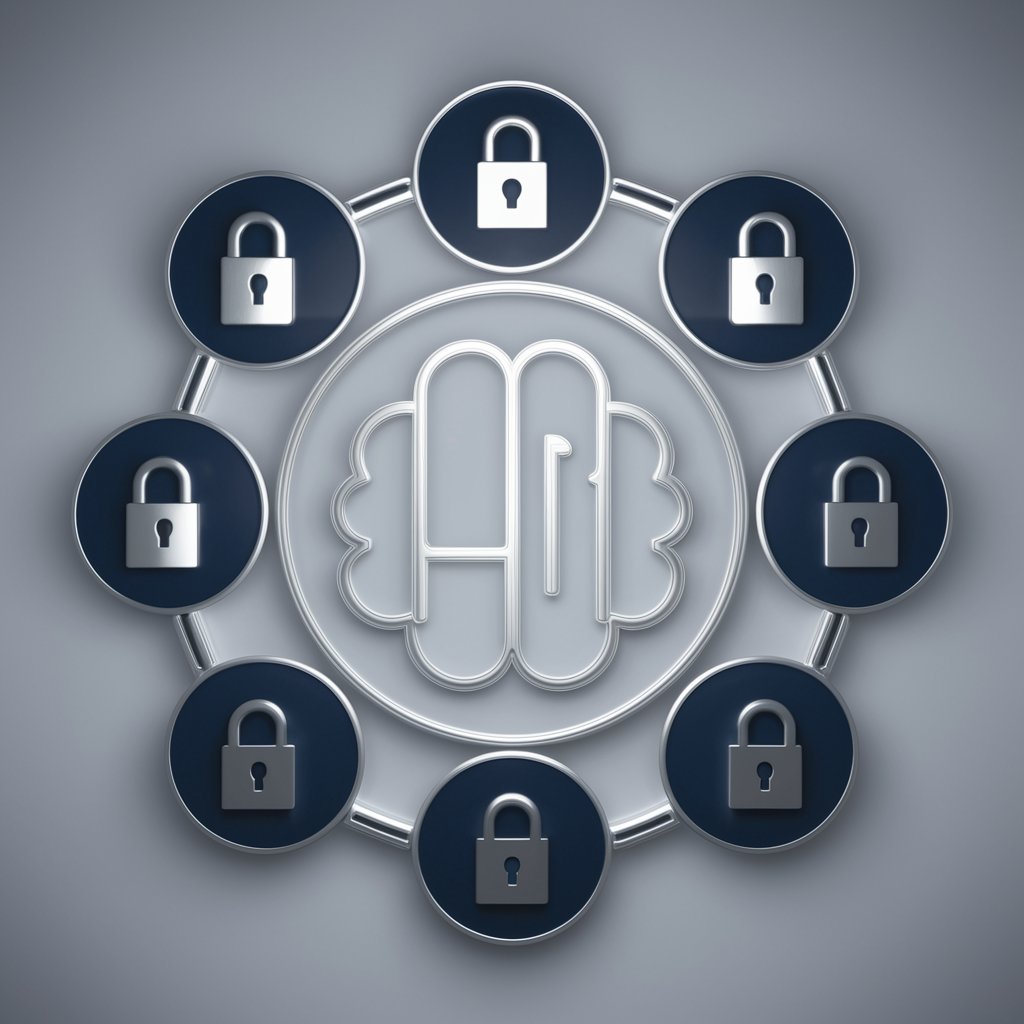
Support Level Up
Elevate your support skills with AI-powered training.
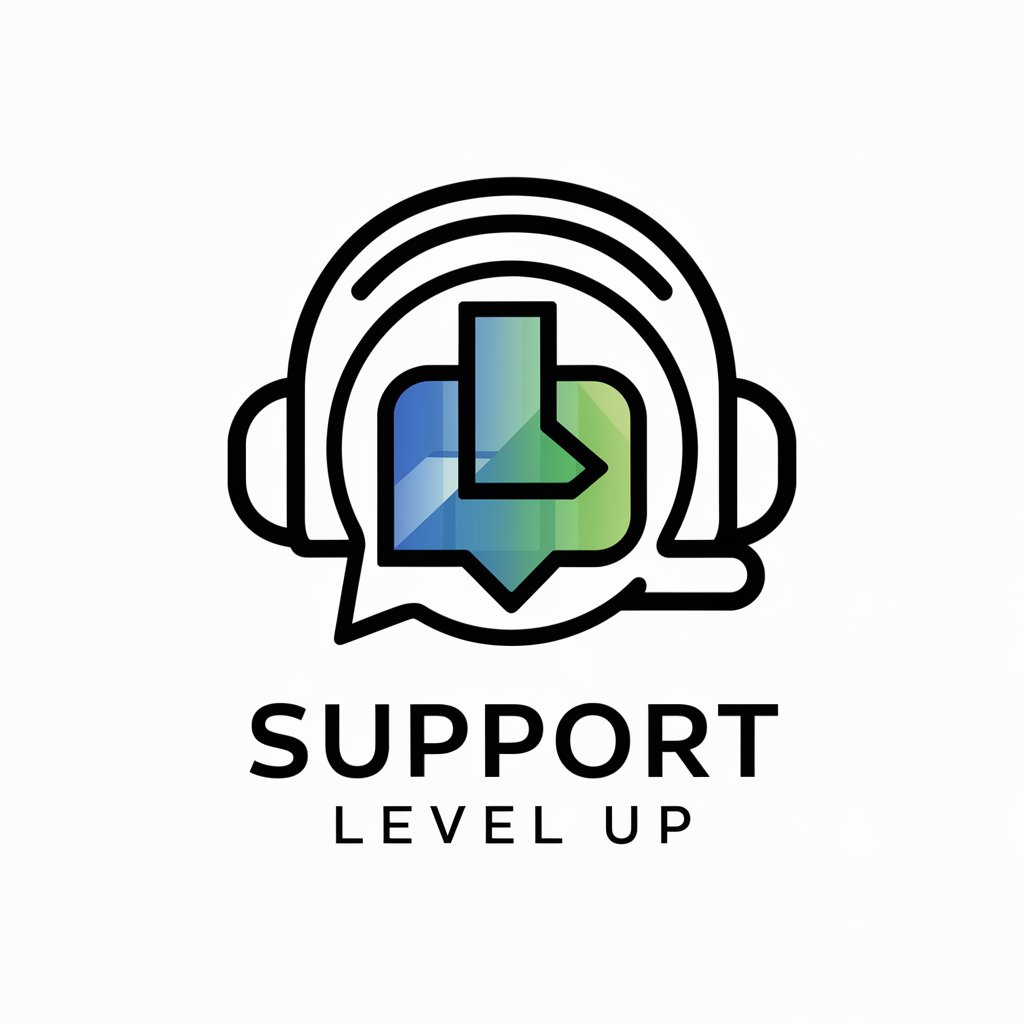
A Leadership Sidekick for New Leaders
Empower Leaders with AI-Powered Guidance
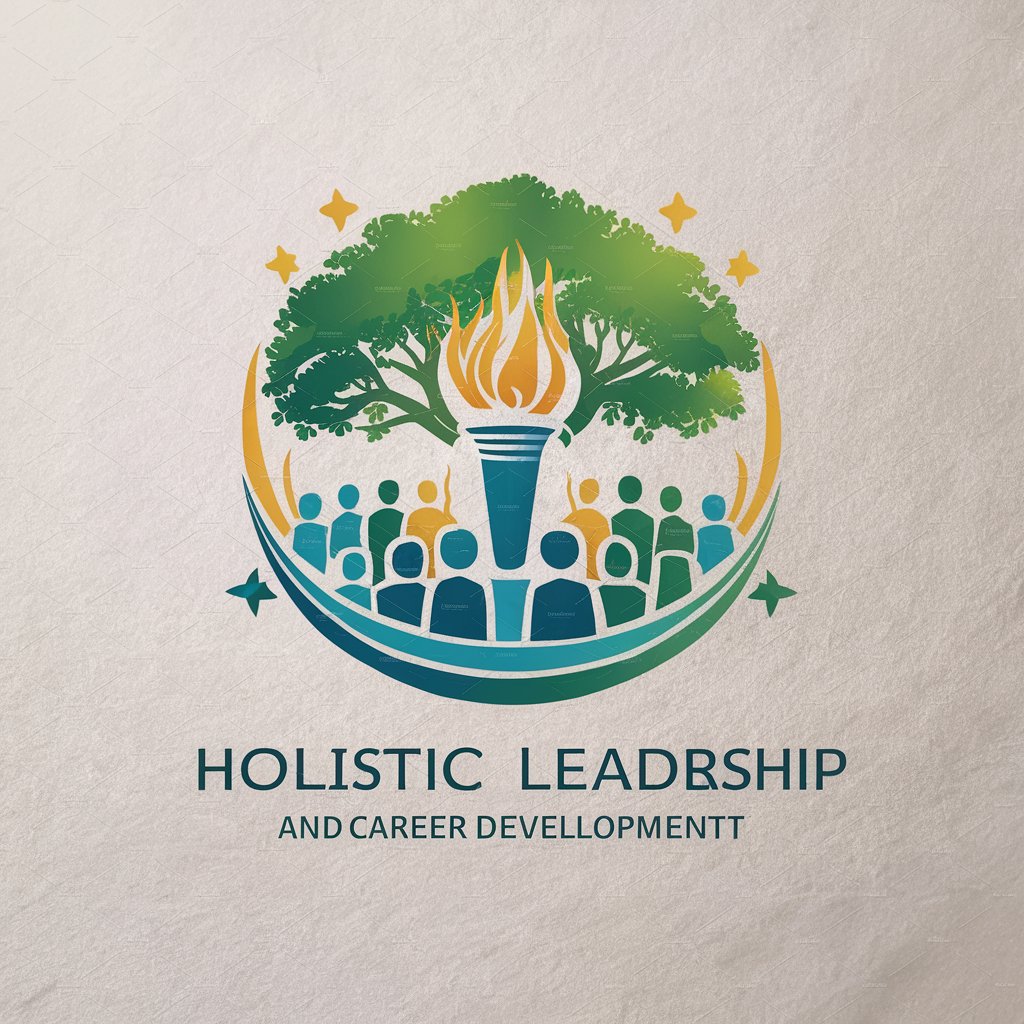
OFSTEDify!
Empowering Schools with AI-Driven OFSTED Preparation
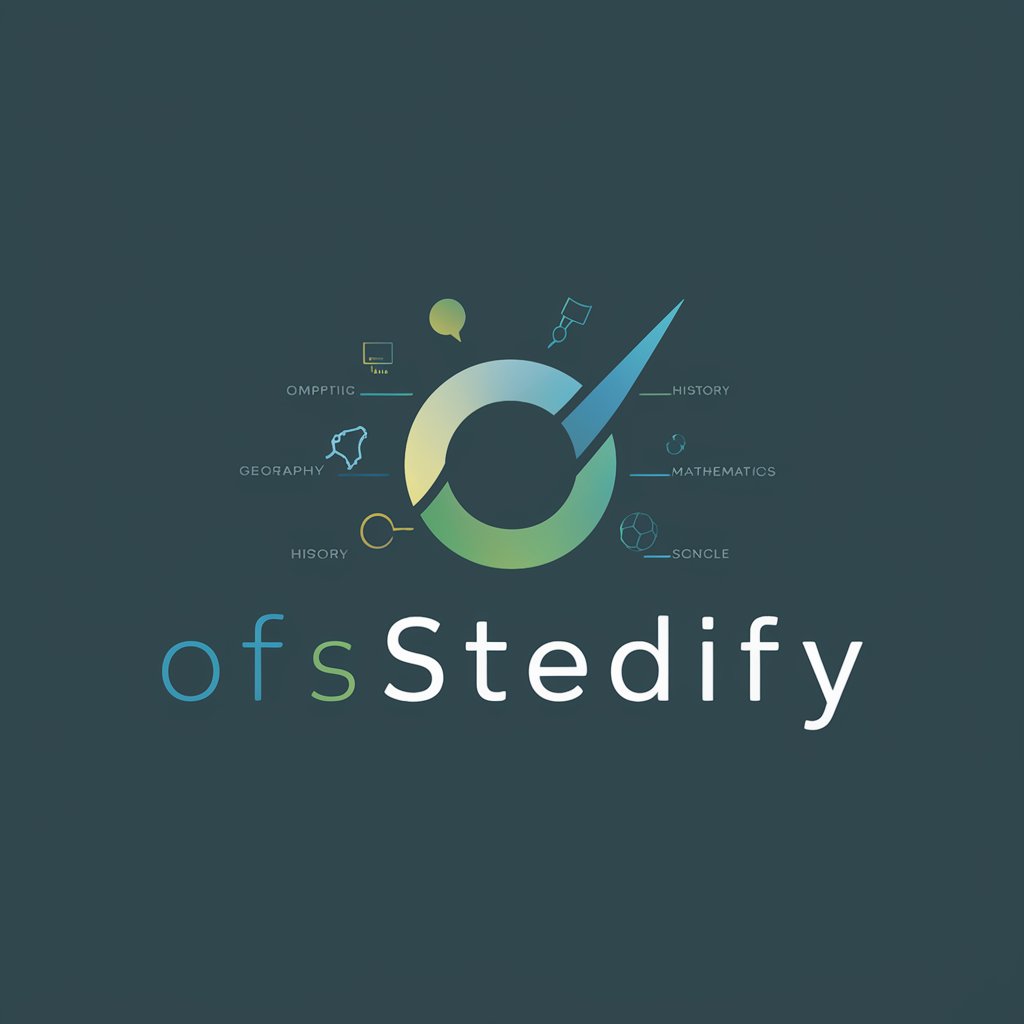
Curator Pro Creator
Empowering your creativity with AI.
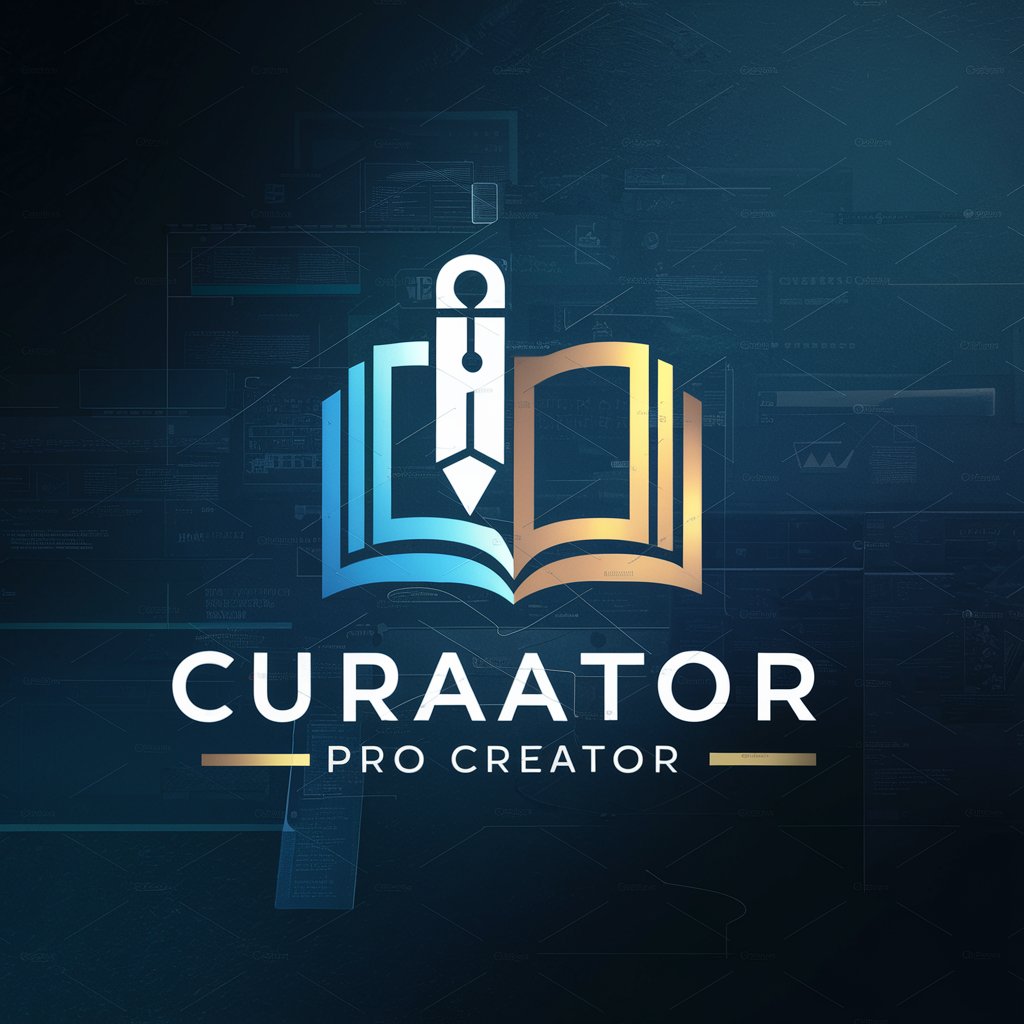
Crypto Mentor
Empowering Your Crypto Journey with AI
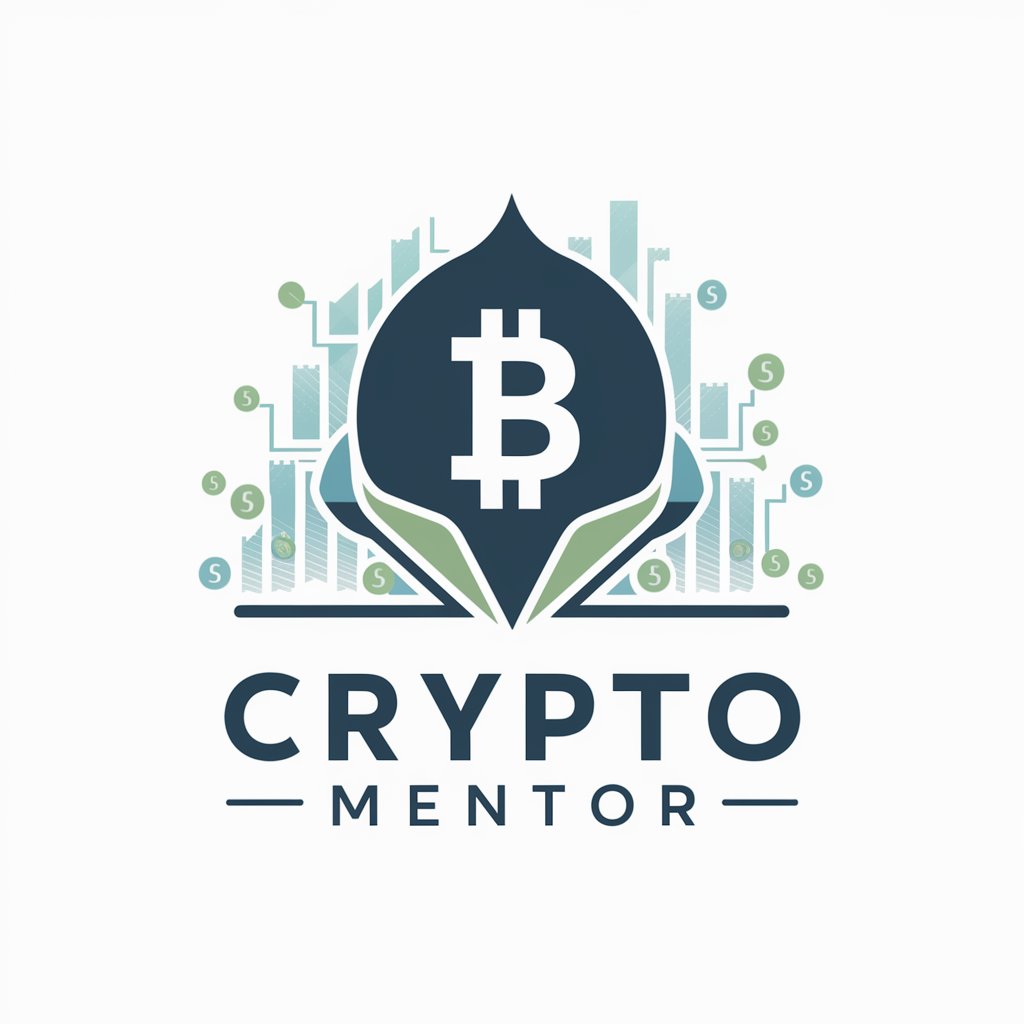
Interactive Storyteller
Craft Engaging Stories with AI
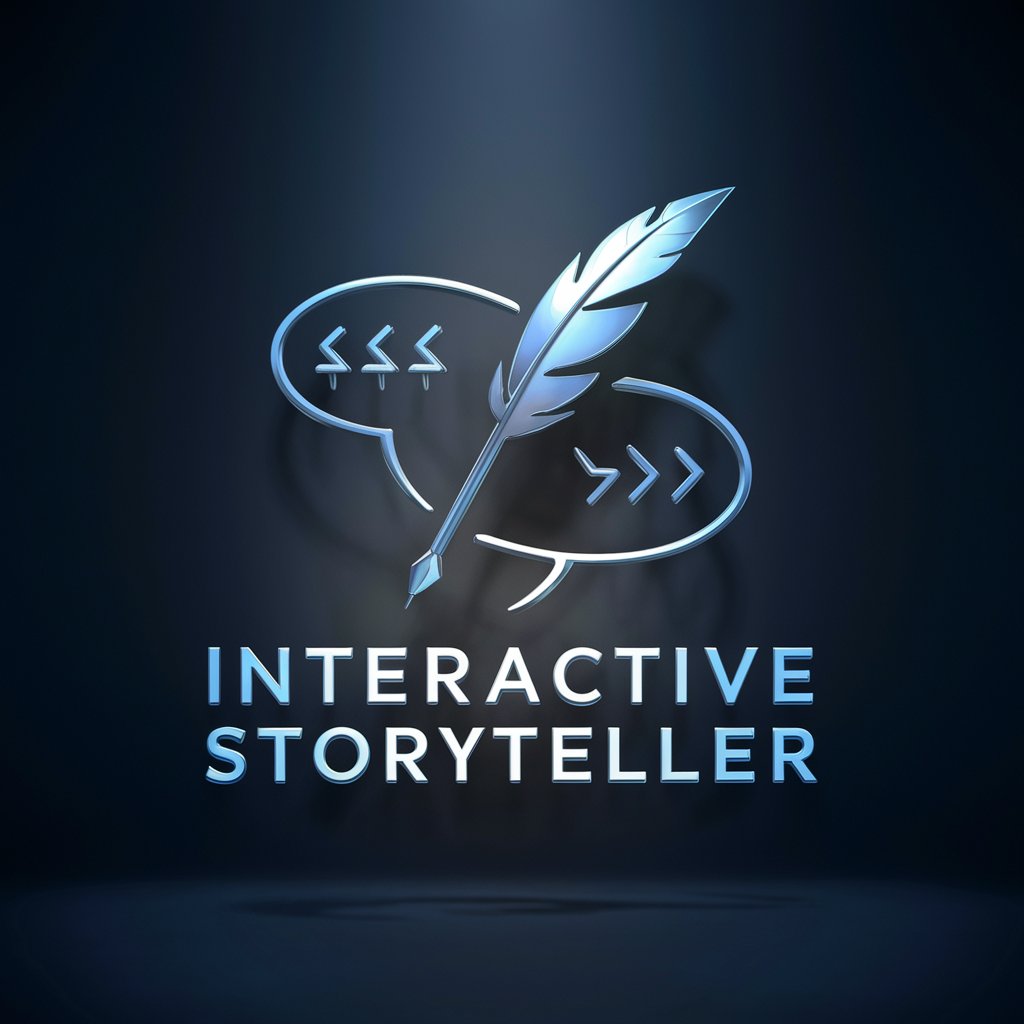
Adventures in color and Story
Bringing stories to life with AI-powered creativity
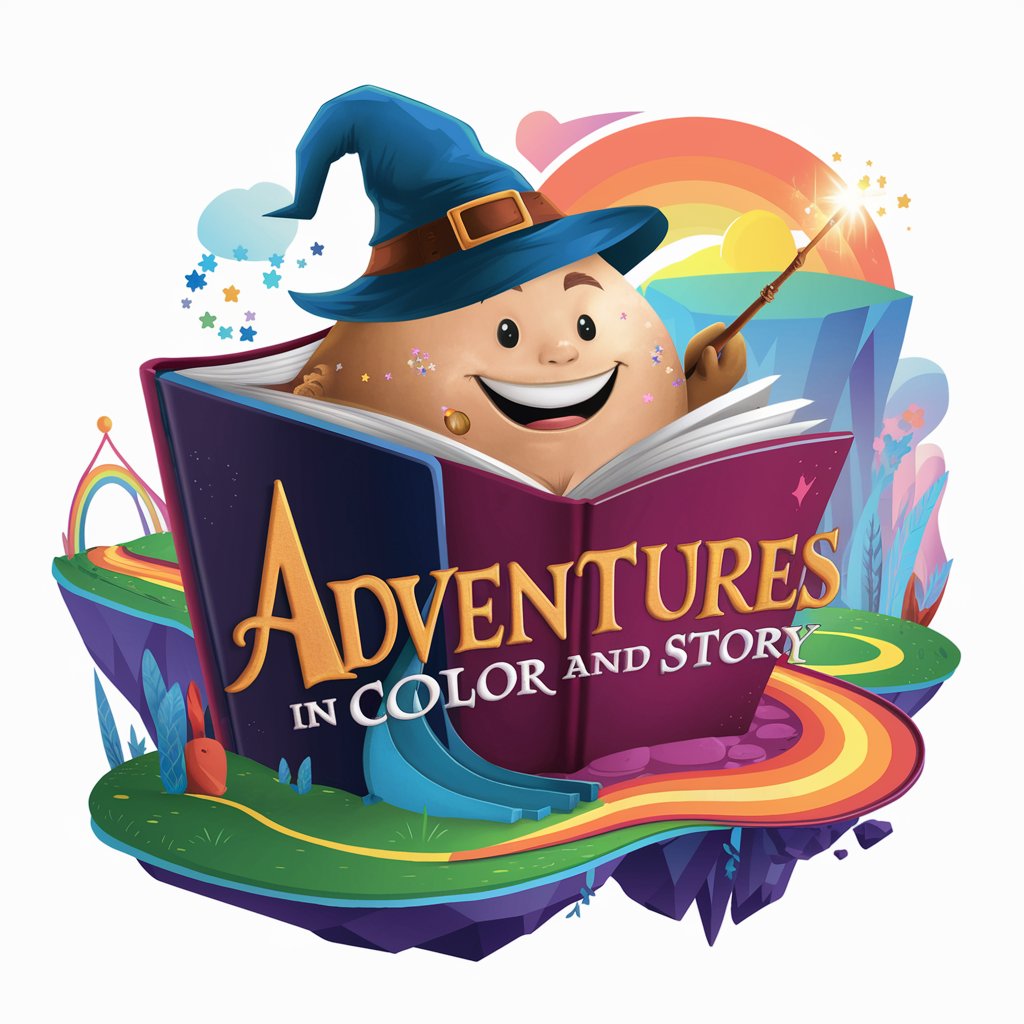
Stream GPT
Empowering Your Streaming Journey with AI
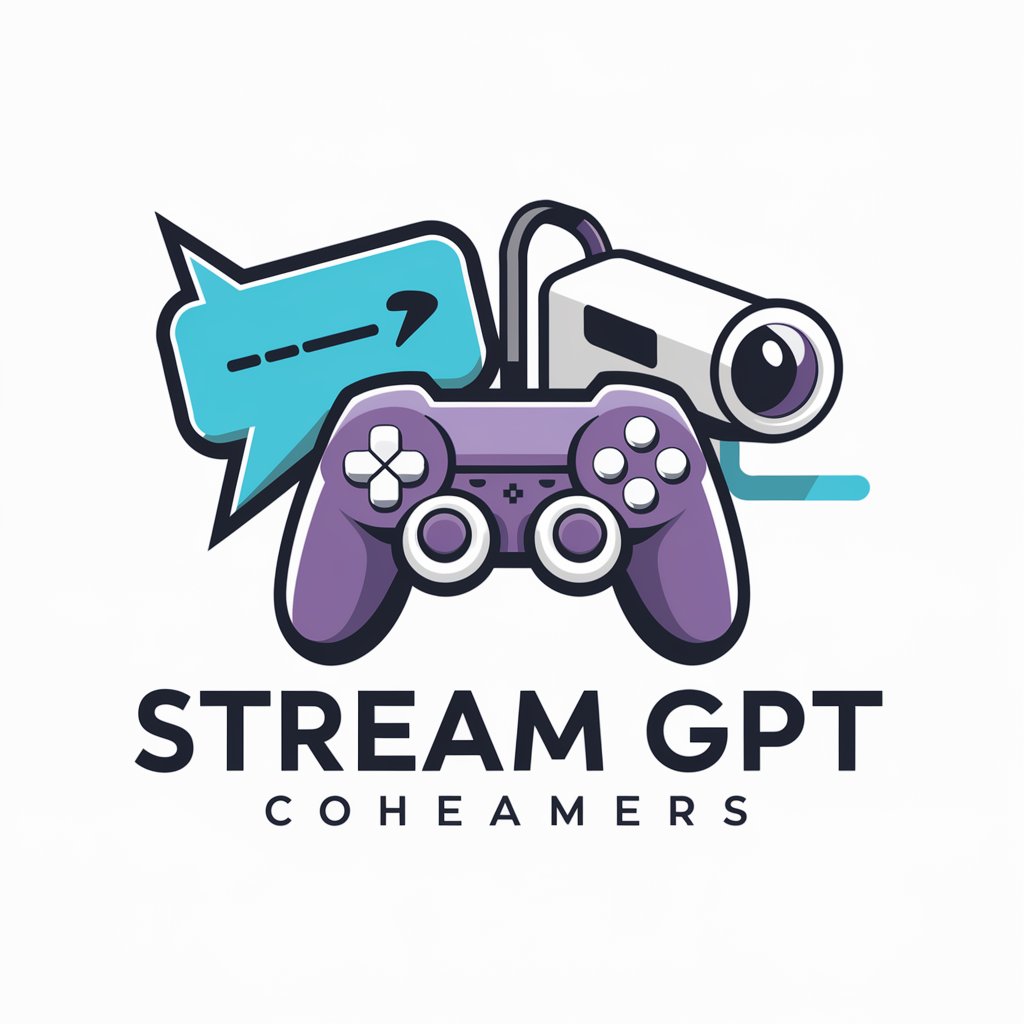
Demo Strategy GPT - The DemoCraft Architect
AI-Powered Demo Strategy Crafting
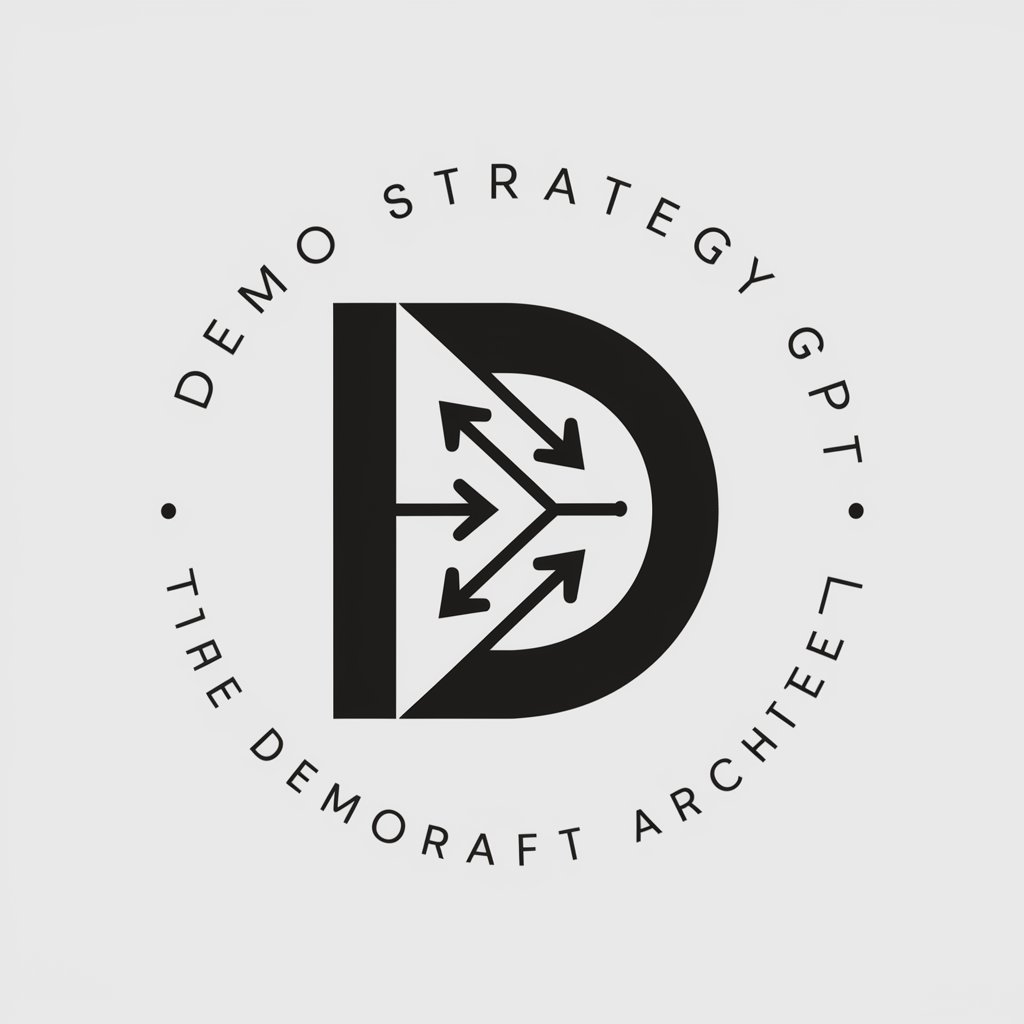
AI Navigator
Navigate Your Queries with AI Precision
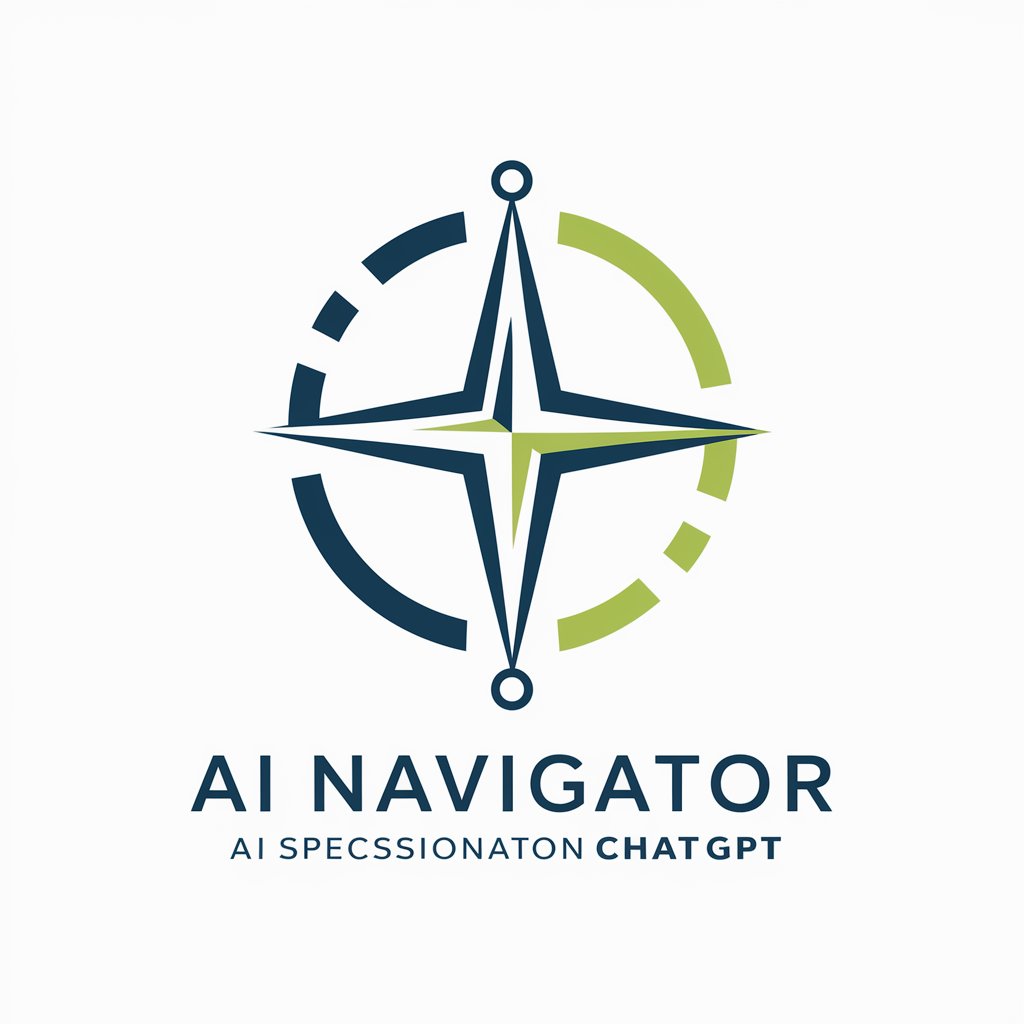
Unfold AI | Guide to services and Products
Empowering businesses with AI
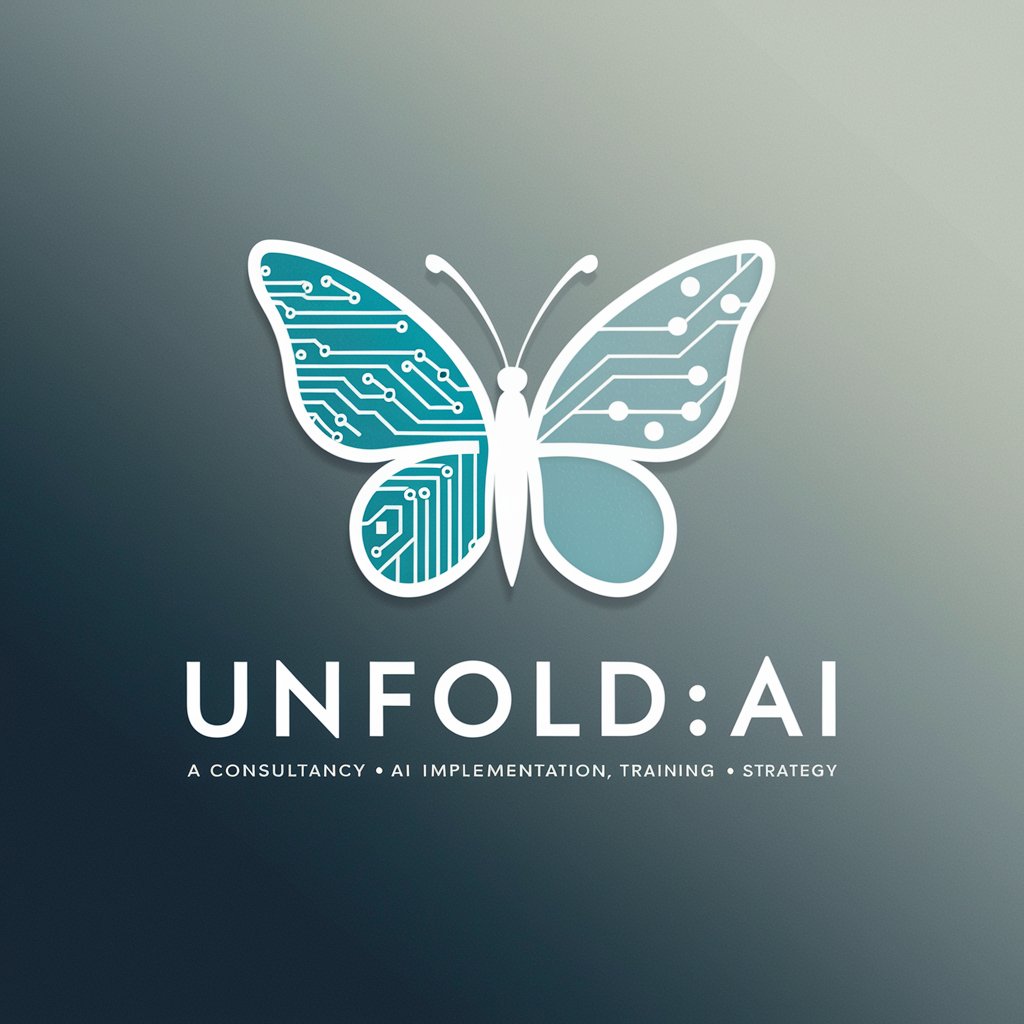
Frequently Asked Questions About AS Time Series and Forecast GPT v. 1.2
What types of time series analysis can AS Time Series and Forecast GPT v. 1.2 perform?
This tool is equipped to perform a variety of analyses, including but not limited to regression analysis with lead-lag variables, ARIMA (AutoRegressive Integrated Moving Average) modeling, and filtering analysis using Hodrick-Prescott and Henderson filters.
Can I use this tool for non-financial data?
Absolutely, AS Time Series and Forecast GPT v. 1.2 is versatile and can analyze any time series data, whether it's financial, environmental, health-related, or any other domain that involves time-dependent data.
How does the tool ensure the accuracy of forecasts?
The tool employs advanced statistical techniques to diagnose data for stationarity, seasonality, and autocorrelation issues, adjusting models accordingly to improve forecast accuracy.
Is there any prerequisite knowledge required to use this tool effectively?
While having a basic understanding of time series analysis concepts can be beneficial, the tool is designed to be user-friendly for both novices and experts. It provides guidance and explanations throughout the analysis process.
How does this tool handle large datasets?
AS Time Series and Forecast GPT v. 1.2 is built to efficiently manage and process large datasets, ensuring rapid analysis and minimizing wait times for users.