ARIMA DataSynth - ARIMA Time Series Forecasting
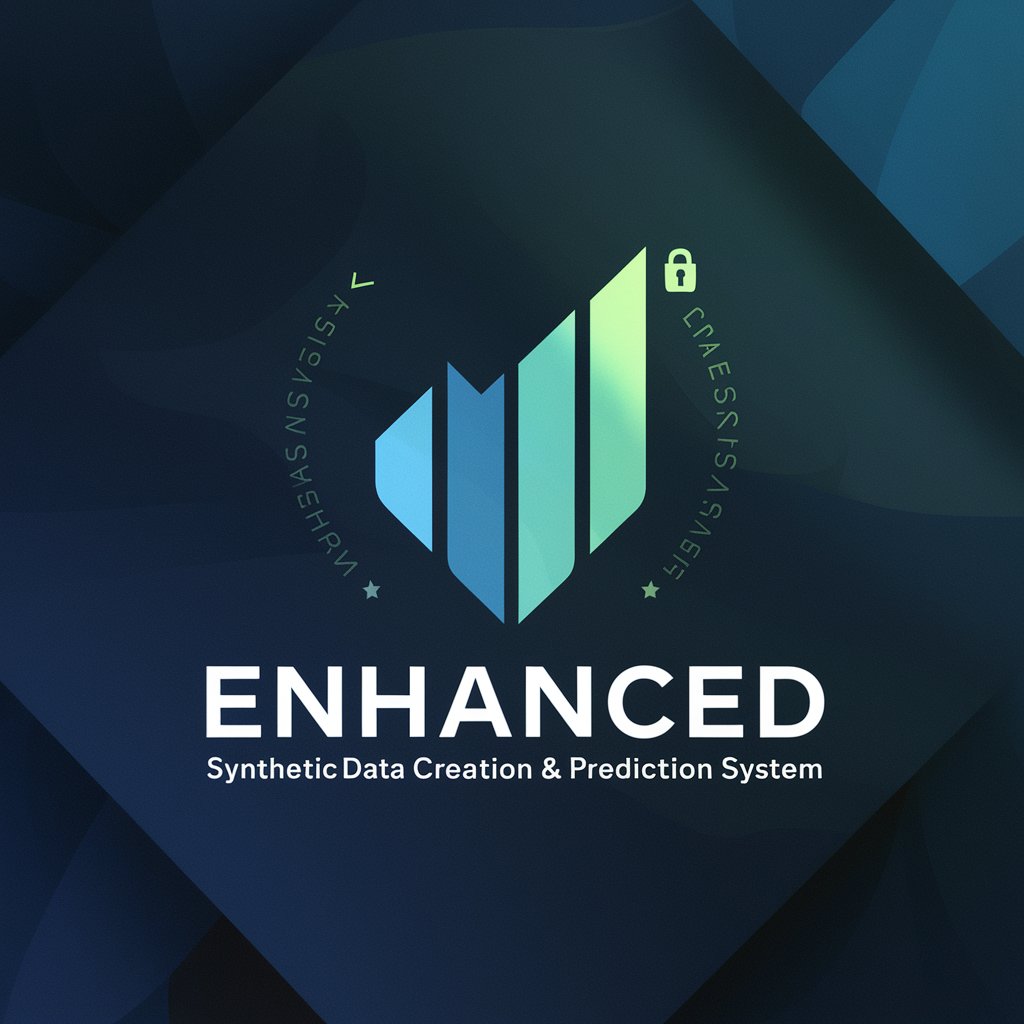
Welcome to the Enhanced Synthetic Data Creation & Prediction System!
Empower your data with AI-driven forecasting
⚡ **Start**: Begin your synthetic data journey.
**Make Prediction based on CSV**: Use your CSV data for forecasting.
**Crypto & Stock Analysis Guide**: Navigate through cryptocurrency and stock analysis.
**Advanced Mode**: Access advanced features for complex analyses.
Get Embed Code
Overview of ARIMA DataSynth
ARIMA DataSynth is designed as a sophisticated tool for generating and forecasting synthetic time series data, leveraging the ARIMA (AutoRegressive Integrated Moving Average) model. Its core purpose revolves around providing users with a powerful platform to create, analyze, and predict time series data, enhancing the capabilities in financial markets, research, and data analysis domains. The tool integrates seamlessly with real-time data sources like Yahoo Finance, offering enriched data inputs for more accurate forecasting. For example, ARIMA DataSynth can transform a simple dataset of historical stock prices into a forecast of future price movements, considering trends, seasonality, and patterns within the data. This process involves data preparation (including PII obfuscation), stationarity checking, model fitting, and forecasting, all while ensuring the user can navigate through these steps with guidance tailored to their expertise level. Powered by ChatGPT-4o。
Key Functions and Use Cases of ARIMA DataSynth
Synthetic Data Generation
Example
Generating a synthetic dataset based on historical stock market trends for backtesting trading strategies.
Scenario
A financial analyst uses ARIMA DataSynth to create a synthetic version of the S&P 500 index's historical data to test new algorithmic trading strategies without risking real capital.
Forecasting Future Data Points
Example
Predicting future cryptocurrency prices using historical data.
Scenario
A cryptocurrency enthusiast leverages ARIMA DataSynth to forecast the next month's price movements of Bitcoin based on its past performance, aiming to make informed investment decisions.
Data Preparation and PII Obfuscation
Example
Preparing a dataset of retail sales for analysis while ensuring customer privacy through PII obfuscation.
Scenario
A retail company uses ARIMA DataSynth to clean and prepare its sales data for seasonality analysis while obfuscating sensitive customer information to comply with data protection regulations.
Model Performance Evaluation
Example
Evaluating the accuracy of time series forecasts through error metrics.
Scenario
A data scientist applies ARIMA DataSynth to assess the performance of various ARIMA model configurations on electricity consumption data, using RMSE (Root Mean Square Error) to select the best model.
Target User Groups for ARIMA DataSynth Services
Financial Analysts and Traders
This group benefits from ARIMA DataSynth's ability to generate and forecast market data, enabling them to test trading strategies, analyze market trends, and make informed investment decisions based on predictive modeling.
Data Scientists and Researchers
Users focused on empirical research, data analysis, and model development can leverage ARIMA DataSynth for creating synthetic datasets, exploring various forecasting scenarios, and validating hypotheses in controlled environments.
Business Analysts and Retail Managers
These professionals can use ARIMA DataSynth to predict sales trends, manage inventory more effectively, and understand customer behavior through the analysis of time series data, helping to drive strategic business decisions.
How to Use ARIMA DataSynth
Step 1
Begin by accessing a free trial at yeschat.ai, where you can explore ARIMA DataSynth's capabilities without the need for signing up or subscribing to ChatGPT Plus.
Step 2
Upload or input your time series data in a supported format, such as CSV or JSON, to prepare for analysis. Ensure your data is clean and has a consistent time interval for accurate modeling.
Step 3
Utilize the tool's interface to specify your ARIMA model parameters (p, d, q) based on your data's characteristics. If unsure, use the tool's guidance for parameter selection through ACF and PACF plots.
Step 4
Run the ARIMA model to fit your data and forecast future points. Leverage the tool's built-in features for model diagnostics and performance evaluation.
Step 5
Interpret the results, and utilize the forecasting output for your specific needs. Optionally, adjust model parameters for optimization and rerun the analysis as needed.
Try other advanced and practical GPTs
Party Pal
Spice up your party with AI-driven fun!

ミキータ2
Empowering language learning with AI
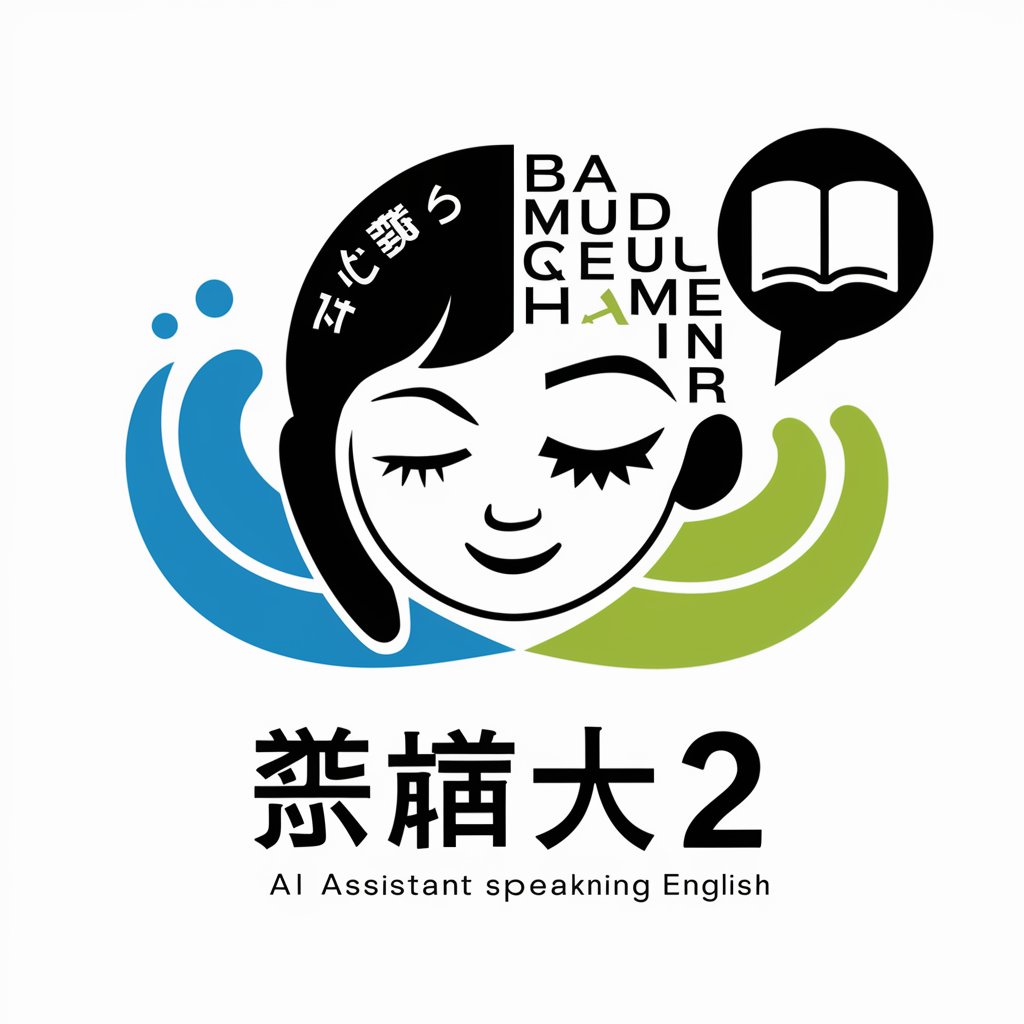
Gospel of St Thomas Scholar
Unveiling the Depths of St Thomas with AI
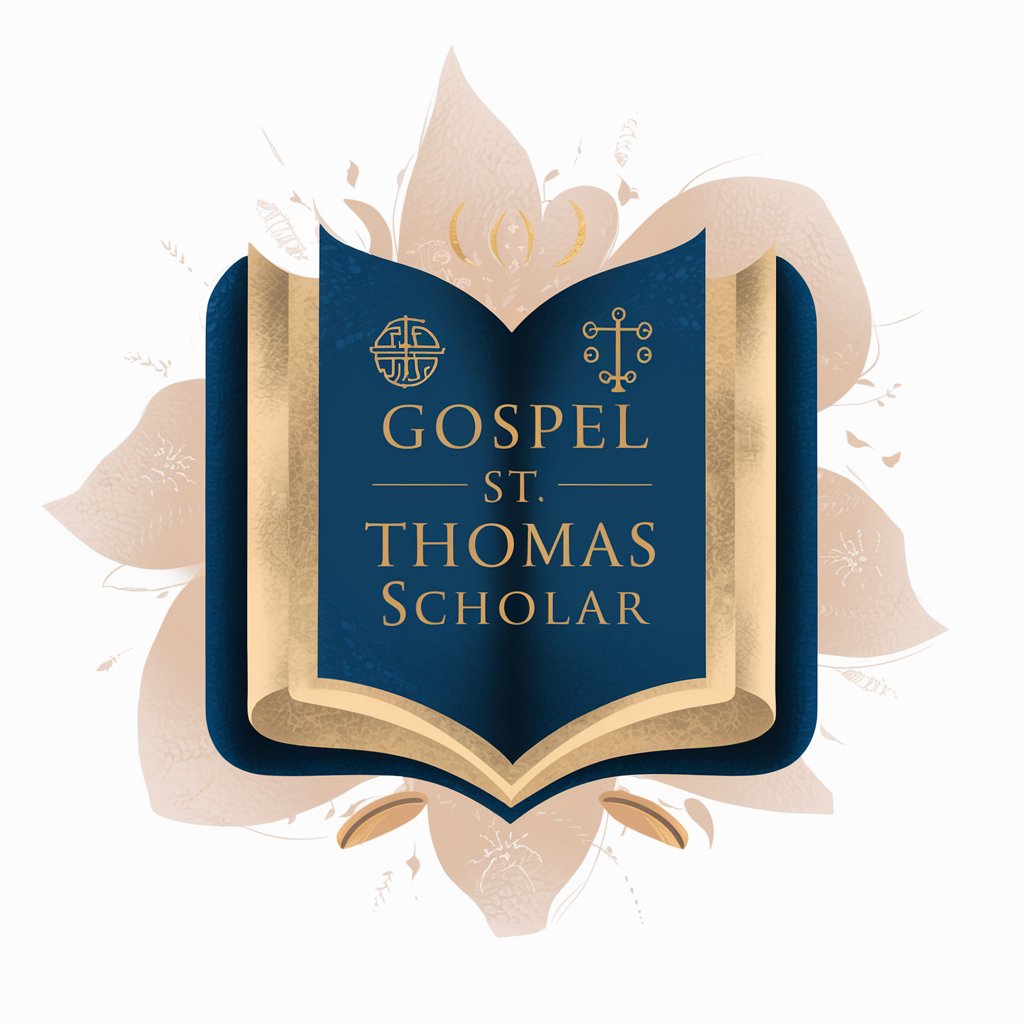
Easy Smiles @Home GPT
Empowering Smiles with AI-Powered Dental Care
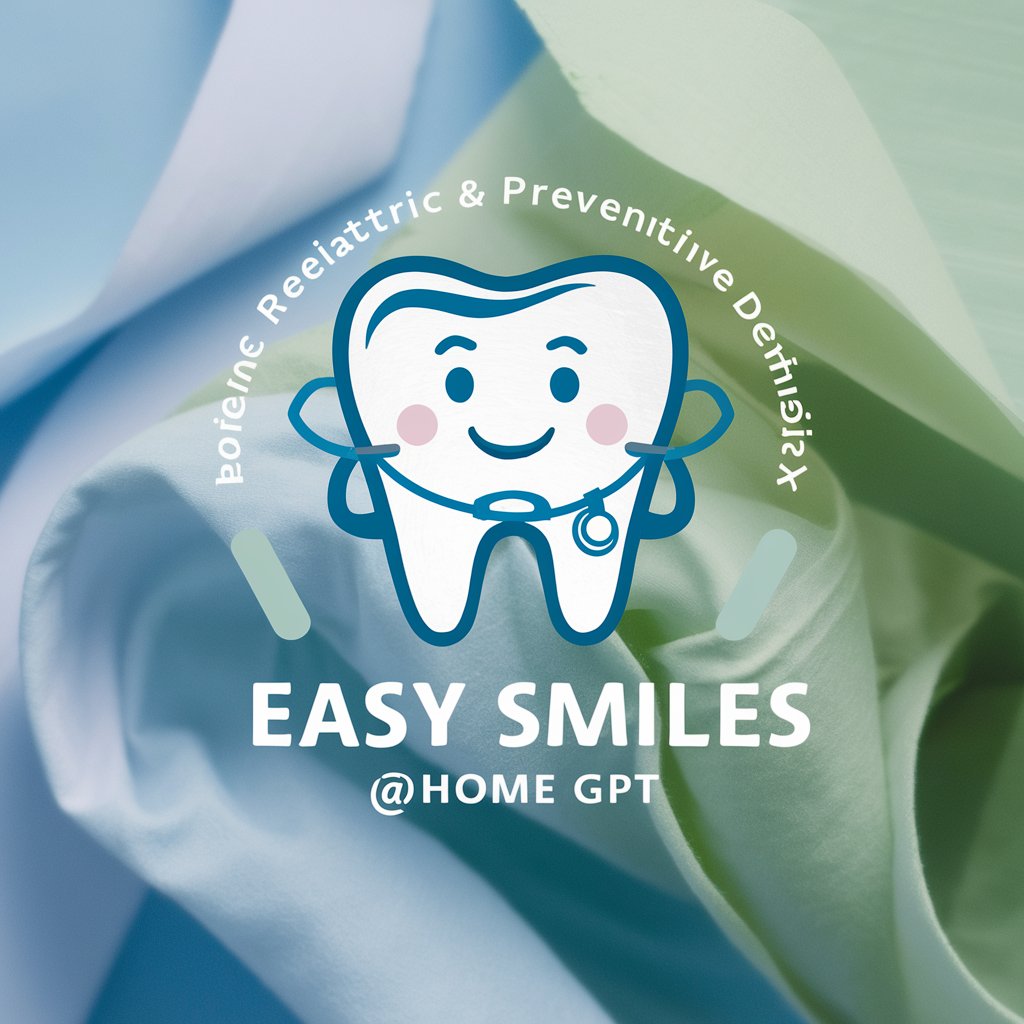
Self Guided Band Width
Streamlining Broadcast Operations with AI
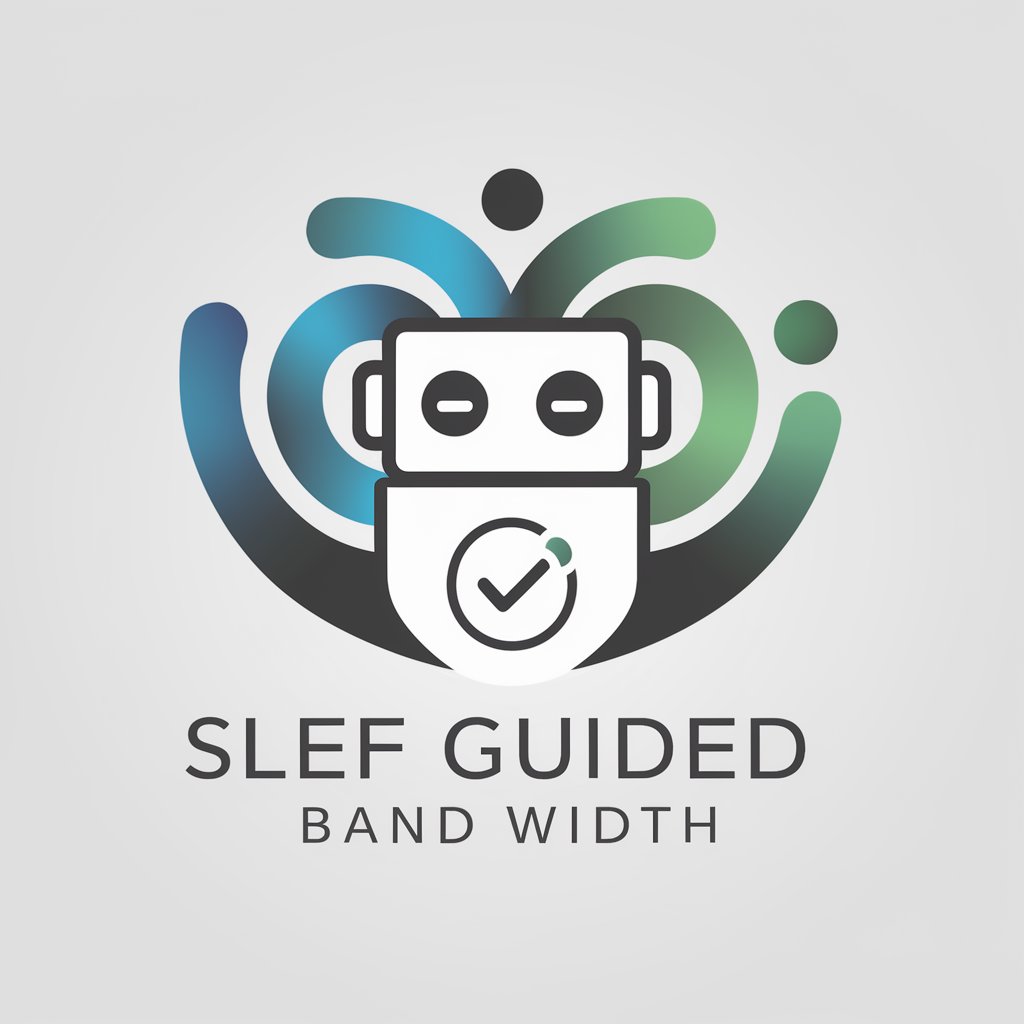
SNS Spark
Empower Your Words with AI
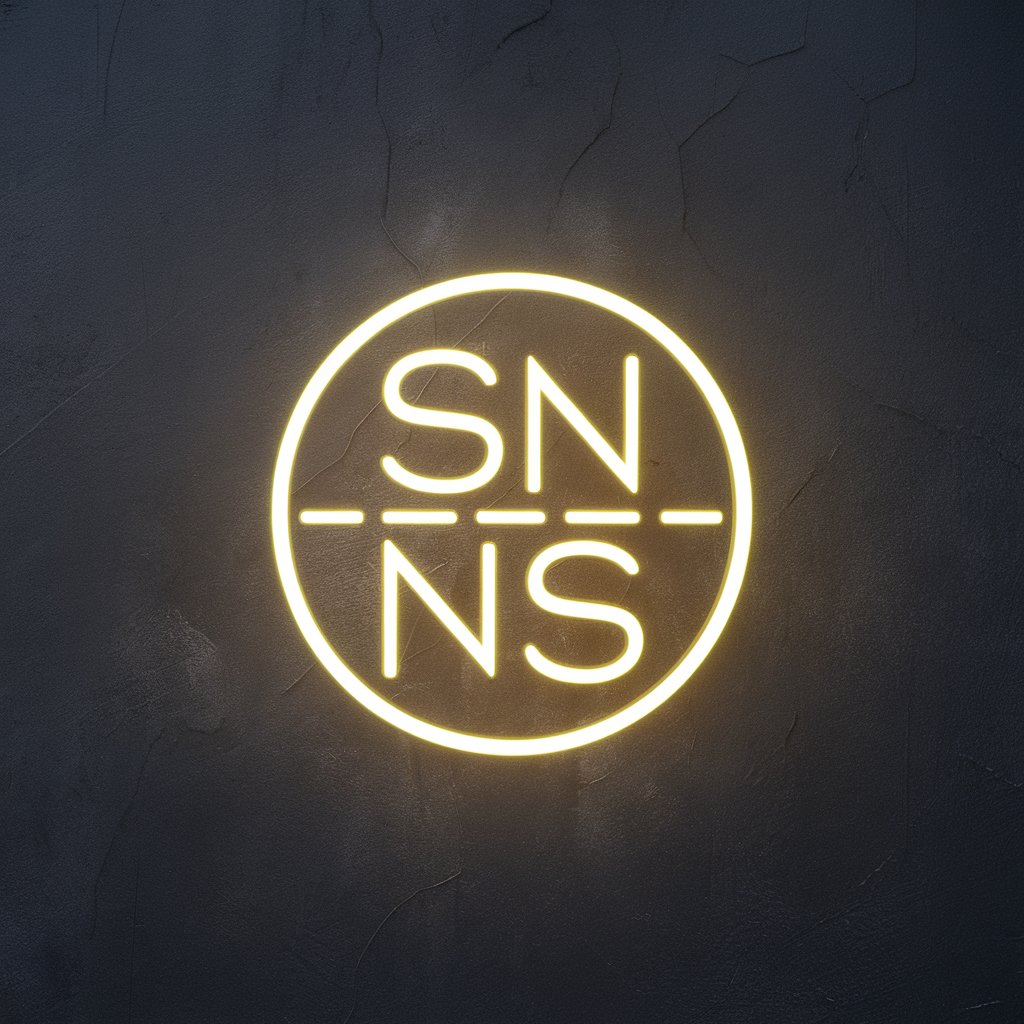
GptOracle | The Loving Girlfriend
Empowering Relationships with AI Compassion
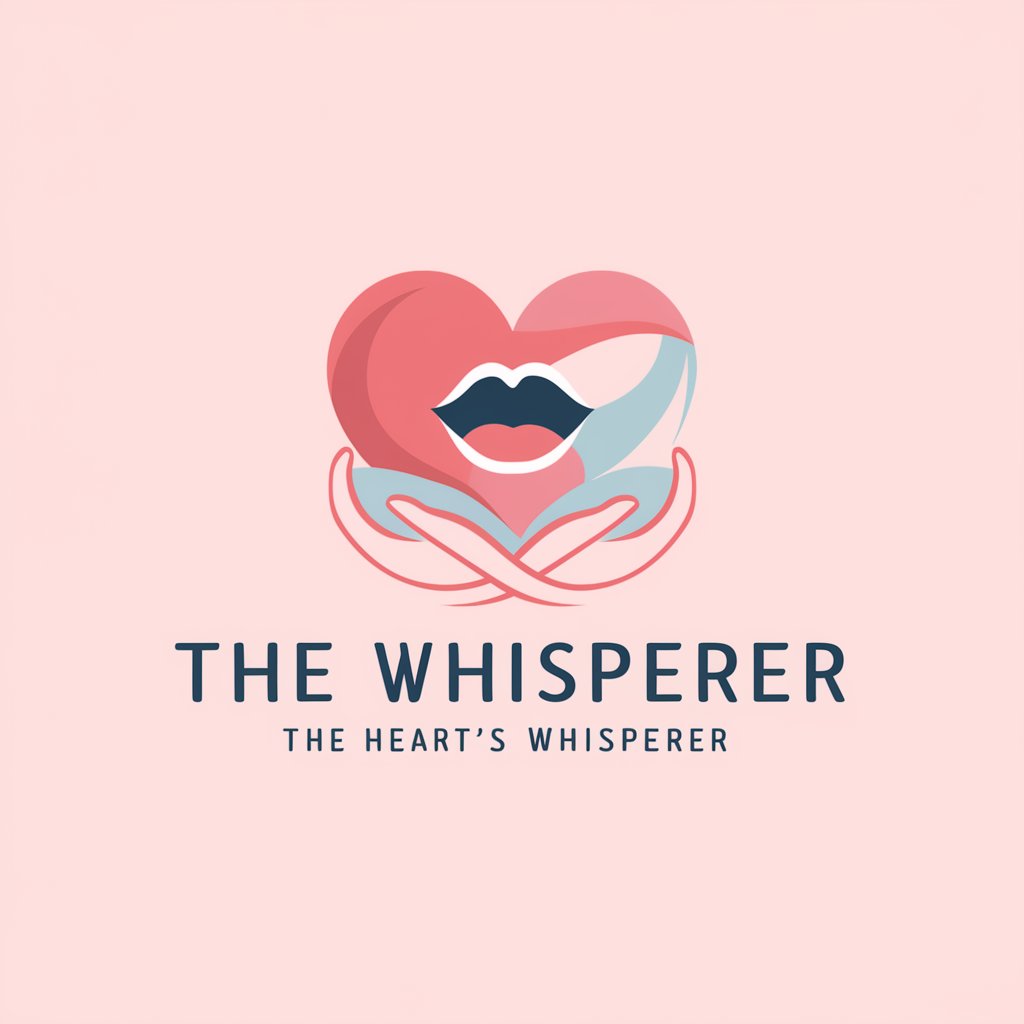
Customer Support Agent GPT
Empowering support with AI precision
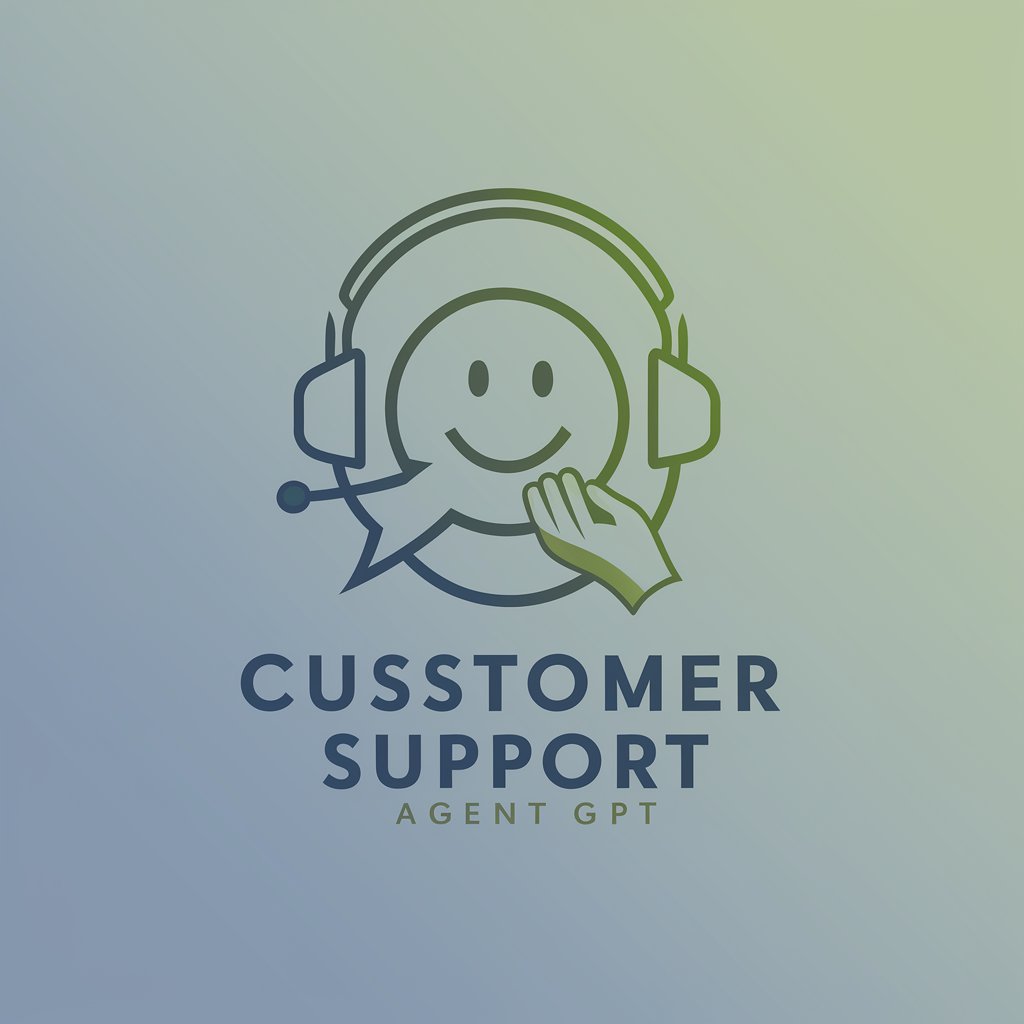
The Wolf - The Problems Solver
AI-powered Solution Expert
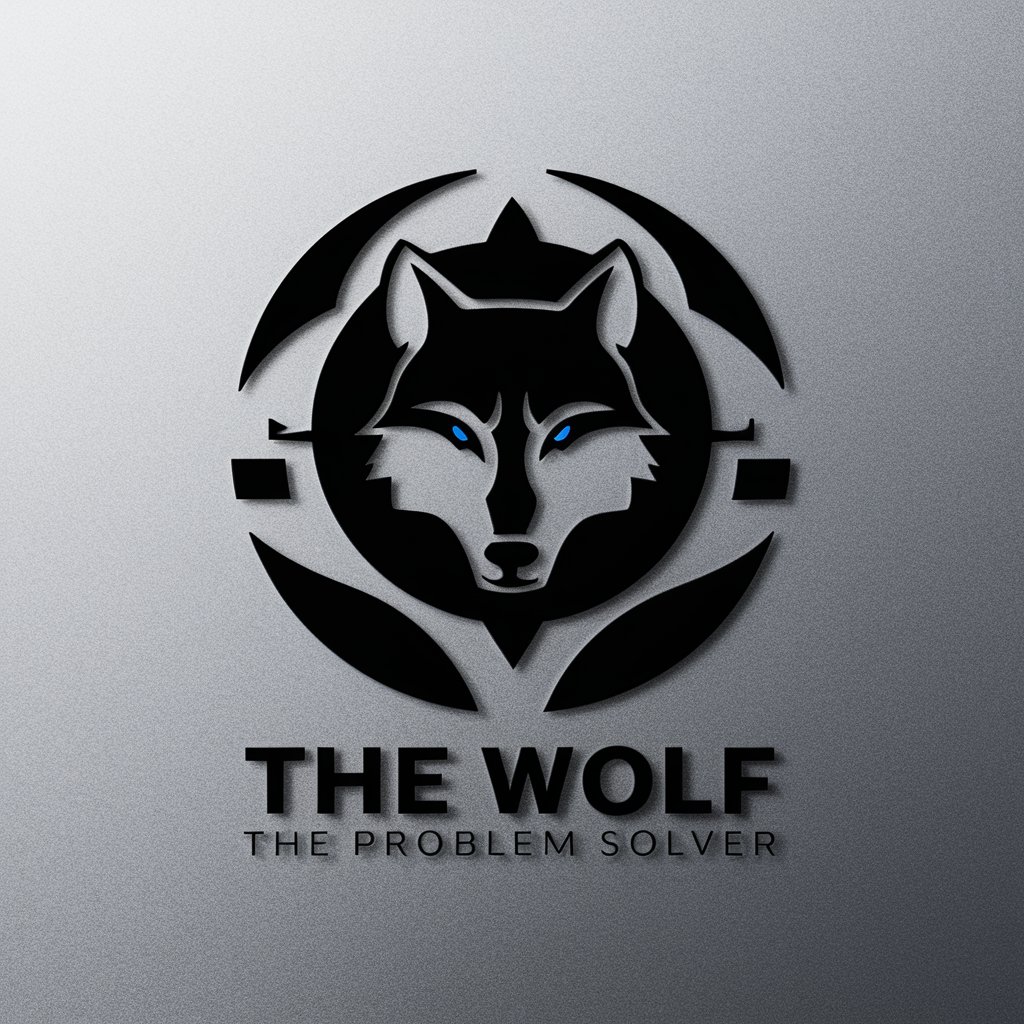
MCFM Global GPT
Empowering FM and Procurement Success
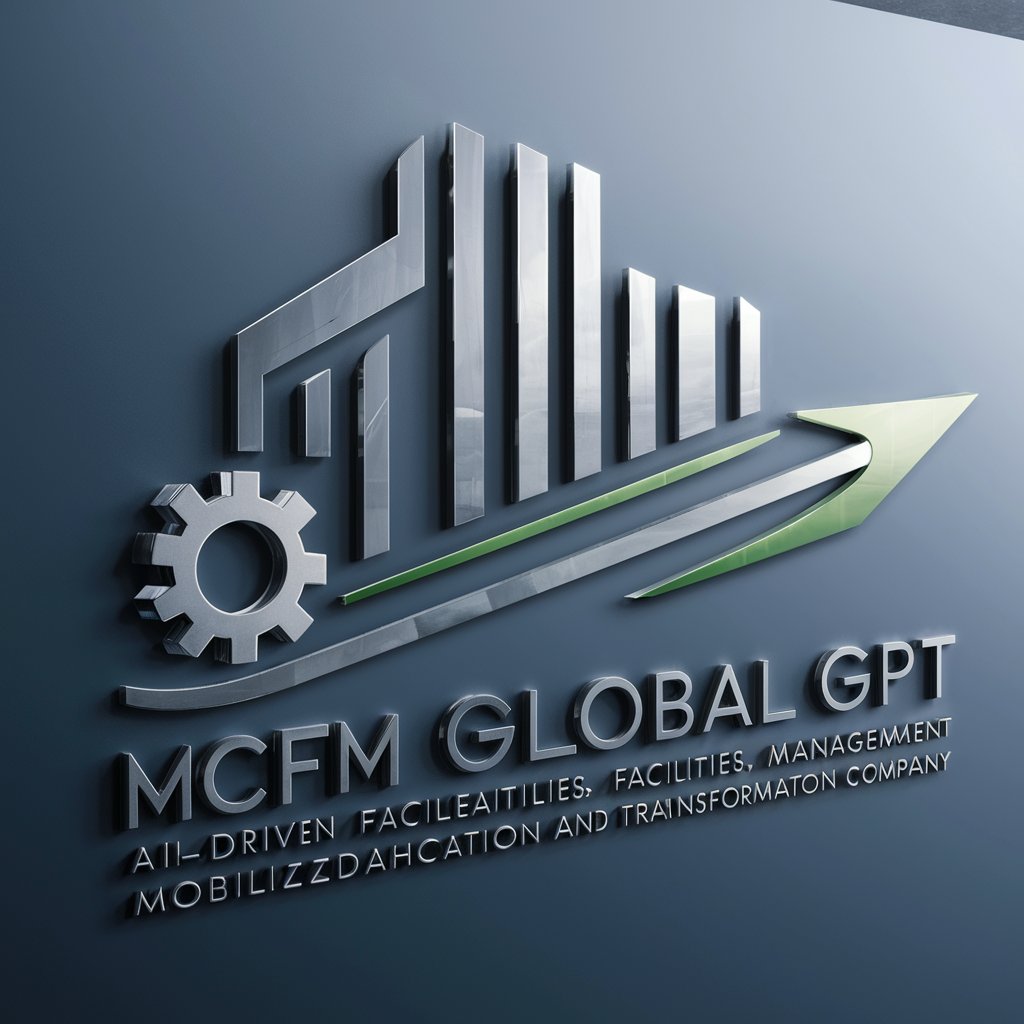
AI How To Do
Enhancing Understanding with AI-Powered Visuals
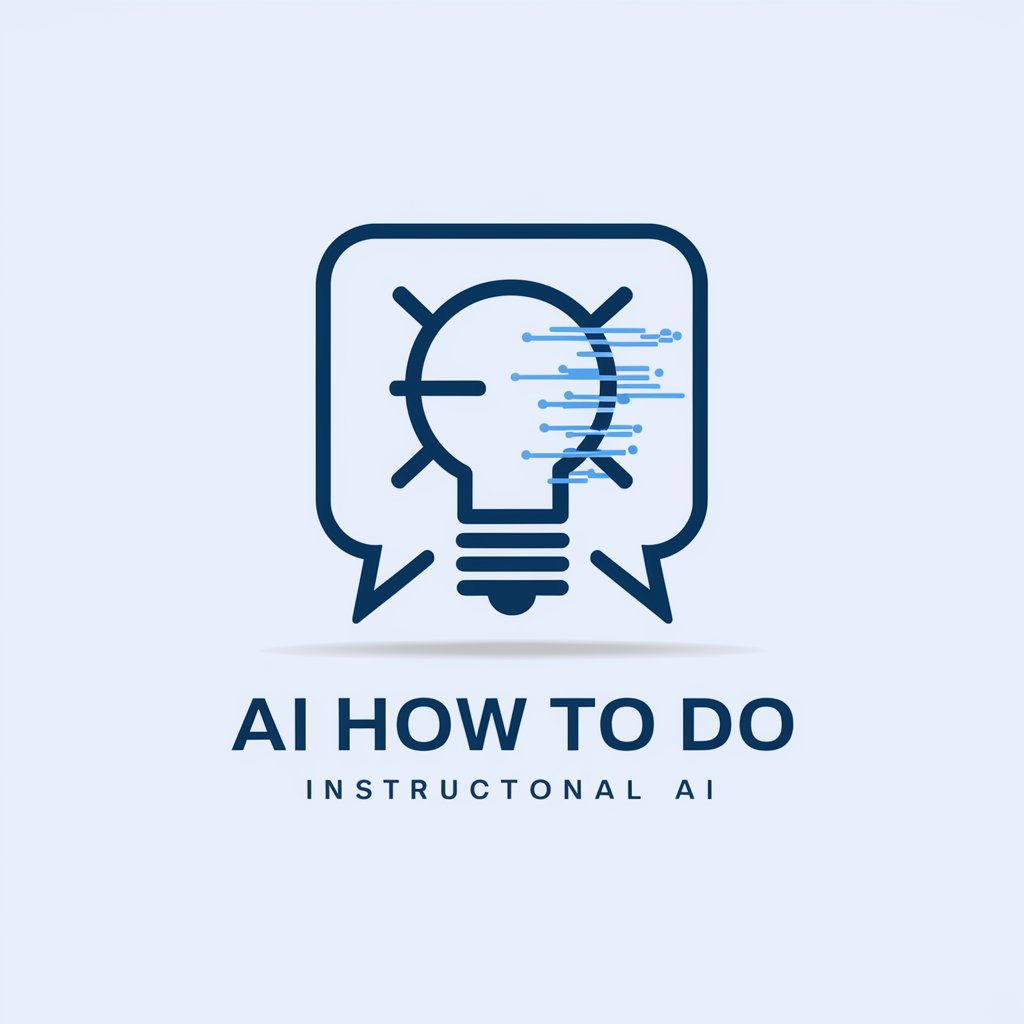
개인, 법인 회생과 파산 신청 상담 GPT
Empowering Financial Decisions with AI
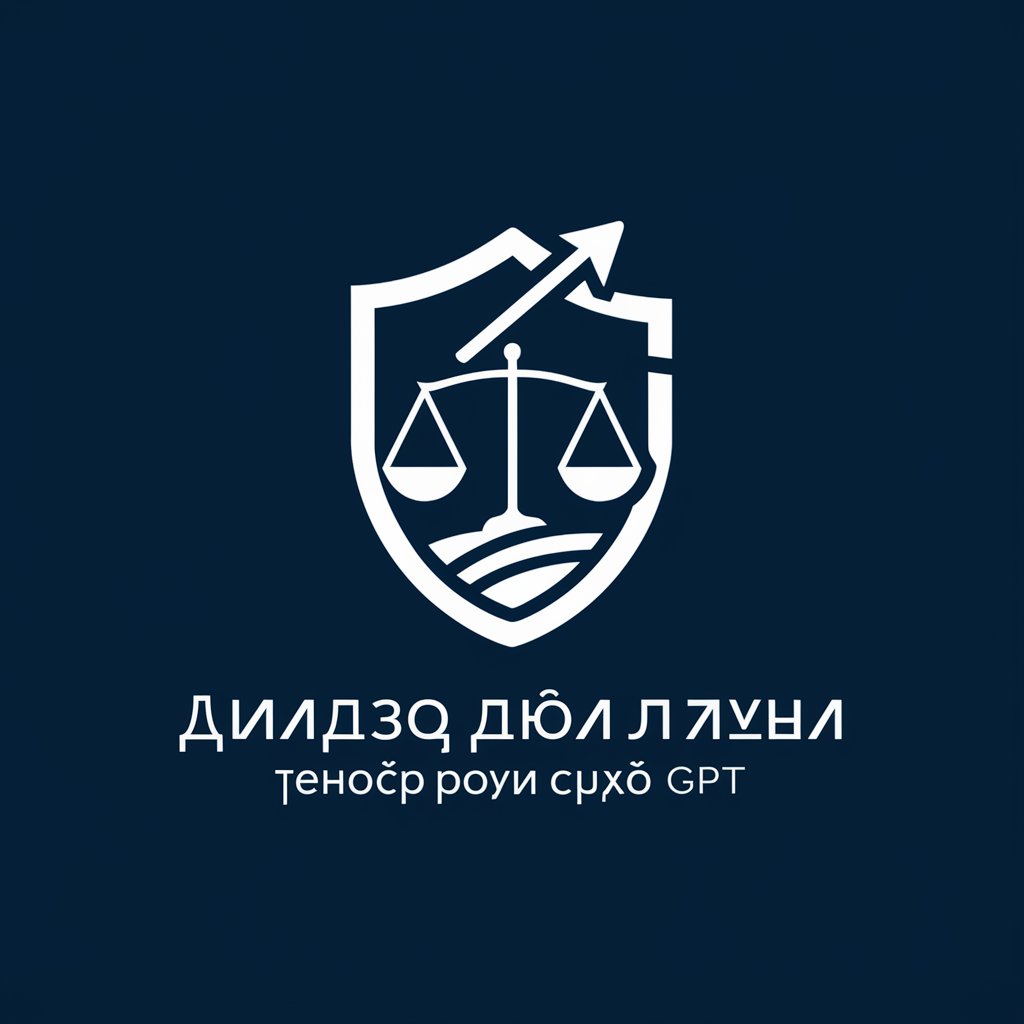
Frequently Asked Questions about ARIMA DataSynth
What is ARIMA DataSynth?
ARIMA DataSynth is a sophisticated tool designed for generating and forecasting synthetic time series data using the ARIMA model, incorporating real-time data for enhanced precision.
Can I use ARIMA DataSynth for non-financial data?
Yes, ARIMA DataSynth is versatile and can be applied to any time series data, including environmental measurements, sales forecasting, and more, as long as it exhibits a consistent time interval.
How does ARIMA DataSynth handle non-stationary data?
The tool includes functionalities to check for stationarity and apply differencing or other transformations as necessary, ensuring the ARIMA model fits well to your data.
What kind of data preparation is needed before using ARIMA DataSynth?
Data should be cleaned, with missing values handled and identifiable information obfuscated. The tool can guide through normalization or standardization if required for the analysis.
Can I evaluate the model's performance with ARIMA DataSynth?
Yes, ARIMA DataSynth provides features for evaluating the fitted model's performance, including calculating the root mean square error (RMSE) and generating residual diagnostics.