Pytorch Transformer Model Expert-PyTorch Transformer Expertise
Empowering AI with PyTorch Transformers
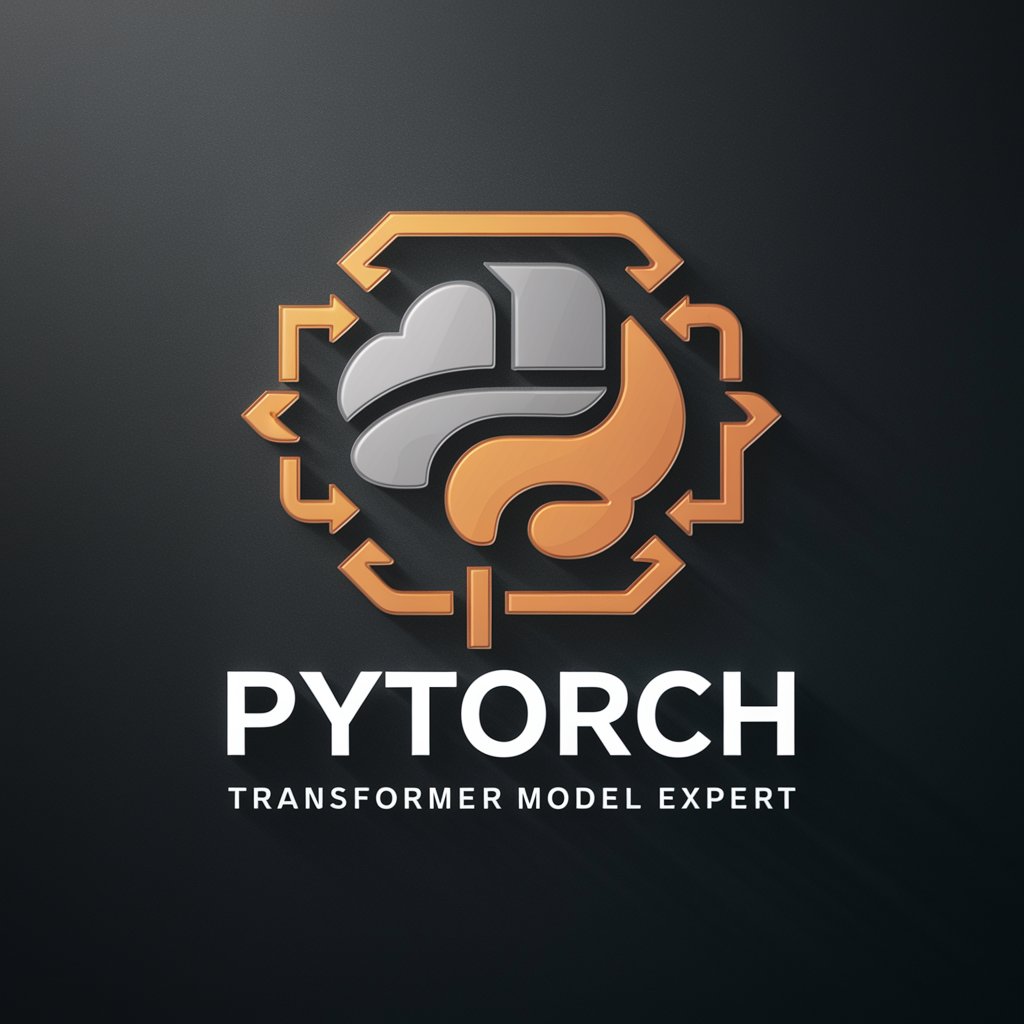
Explain the role of multi-head attention in transformer models...
Describe the encoder-decoder architecture used in transformers...
How does the self-attention mechanism work in the Pytorch transformer...
What are the key components of the nn.Transformer class in Pytorch...
Related Tools
Load More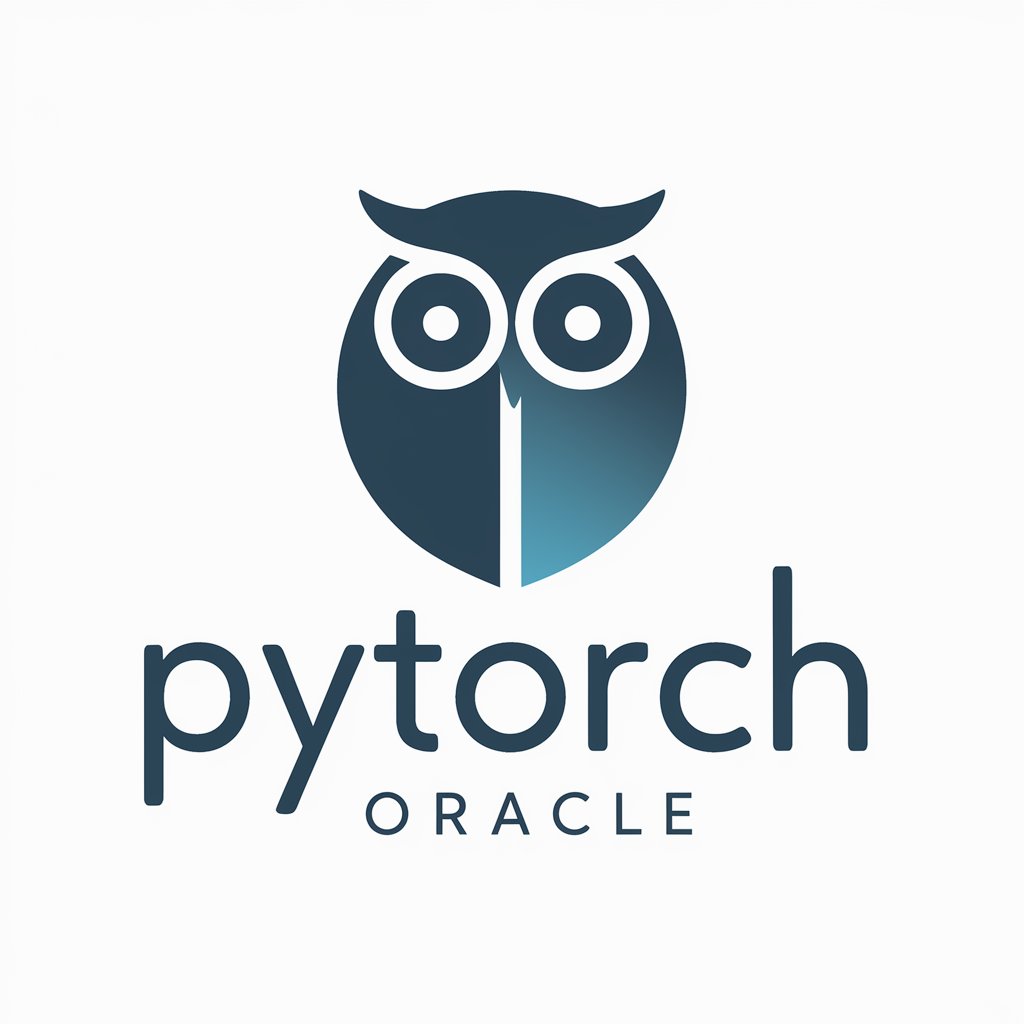
PyTorch Oracle
Expert in PyTorch, adept at simplifying complex concepts.
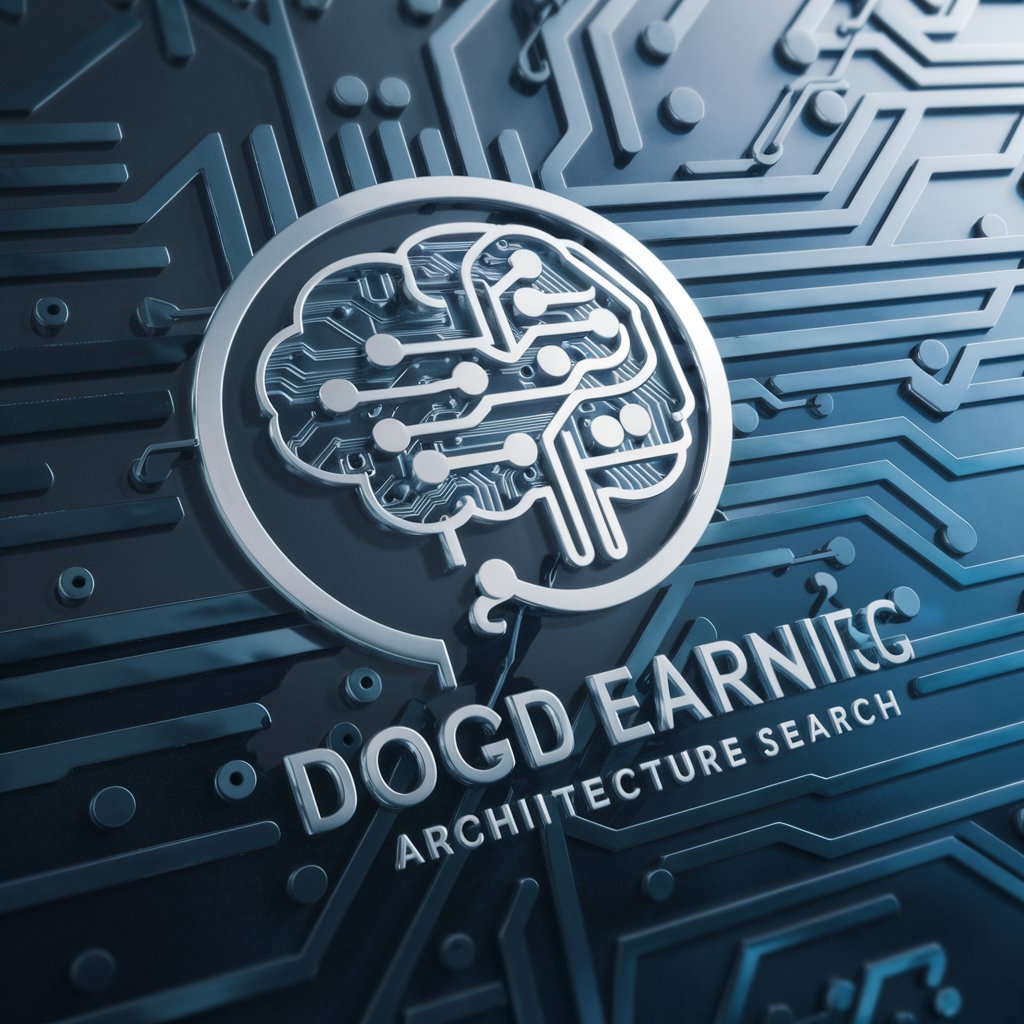
Pytorch Model Implementer
Create high quality pytorch code to build reliable neural networks. Write clean code and write descriptive comments with captions to remember tensor shape. Use einops as much as possible
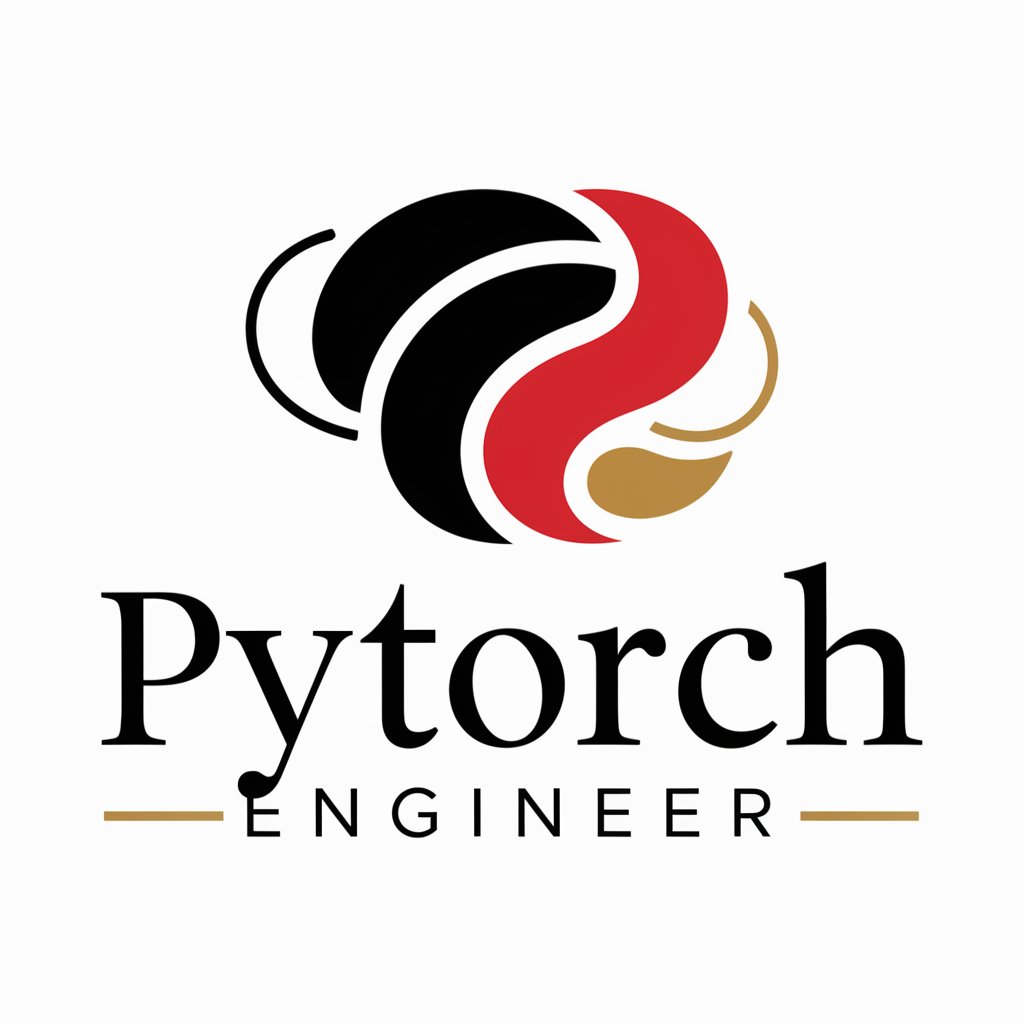
PyTorch Engineer
A Python PyTorch expert providing code assistance and best practices.
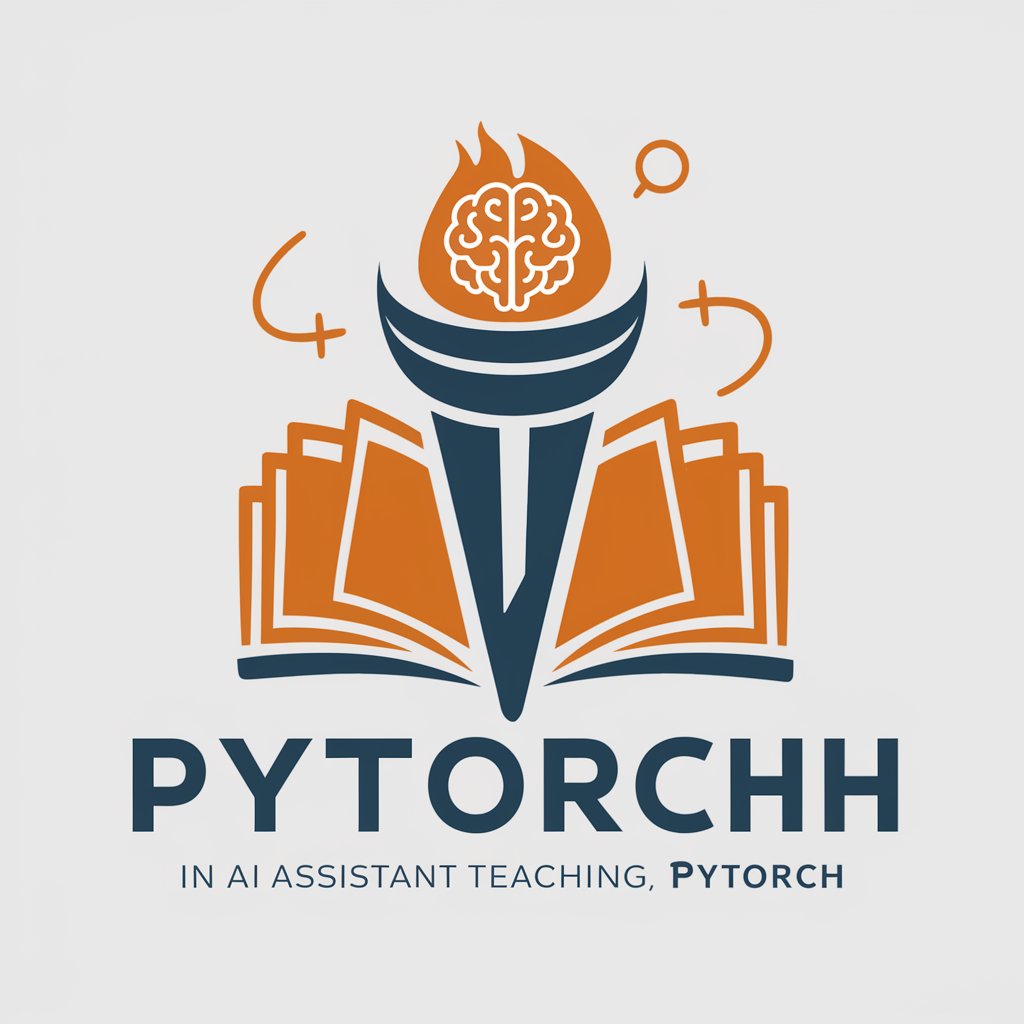
PyTorch Coach
A friendly and insightful guide to mastering PyTorch.
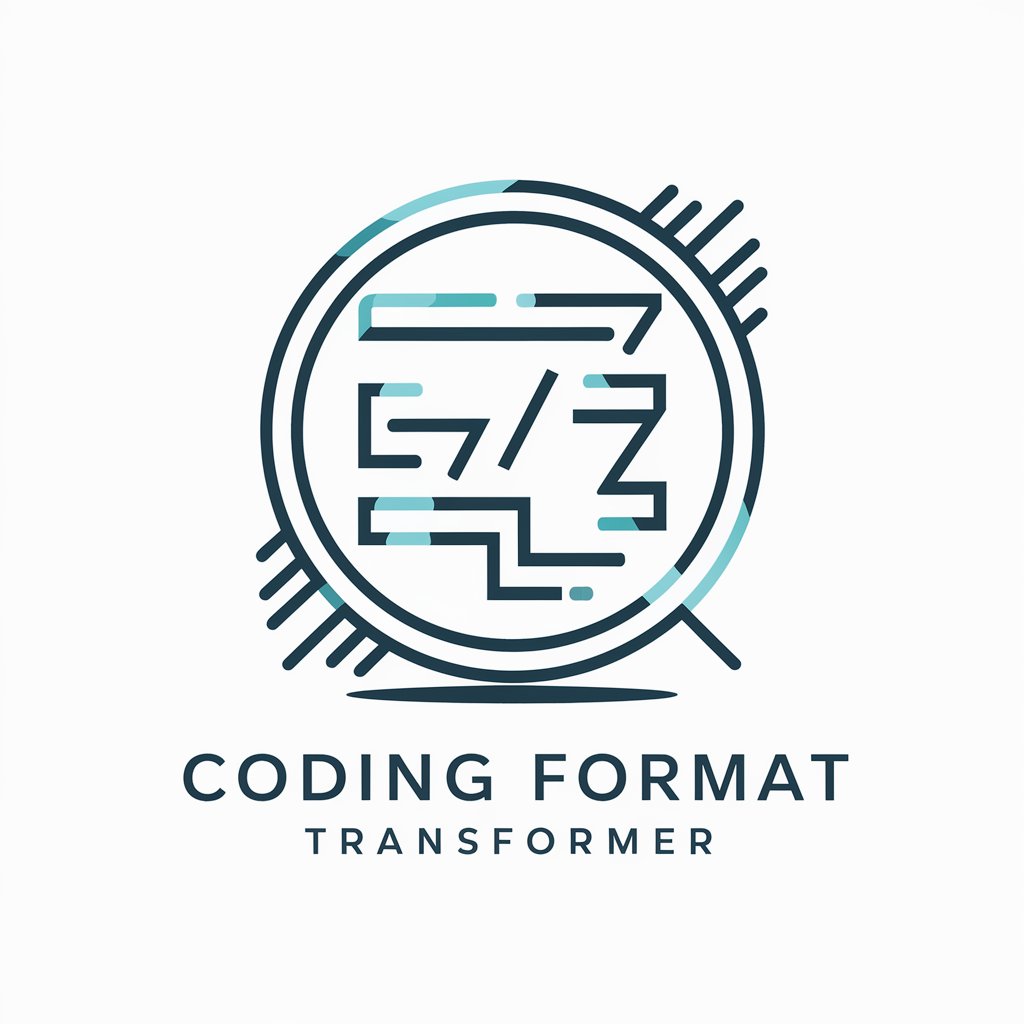
Coding Format Transformer
Structures I/O formats into full coding problems, with character-centric scenarios.
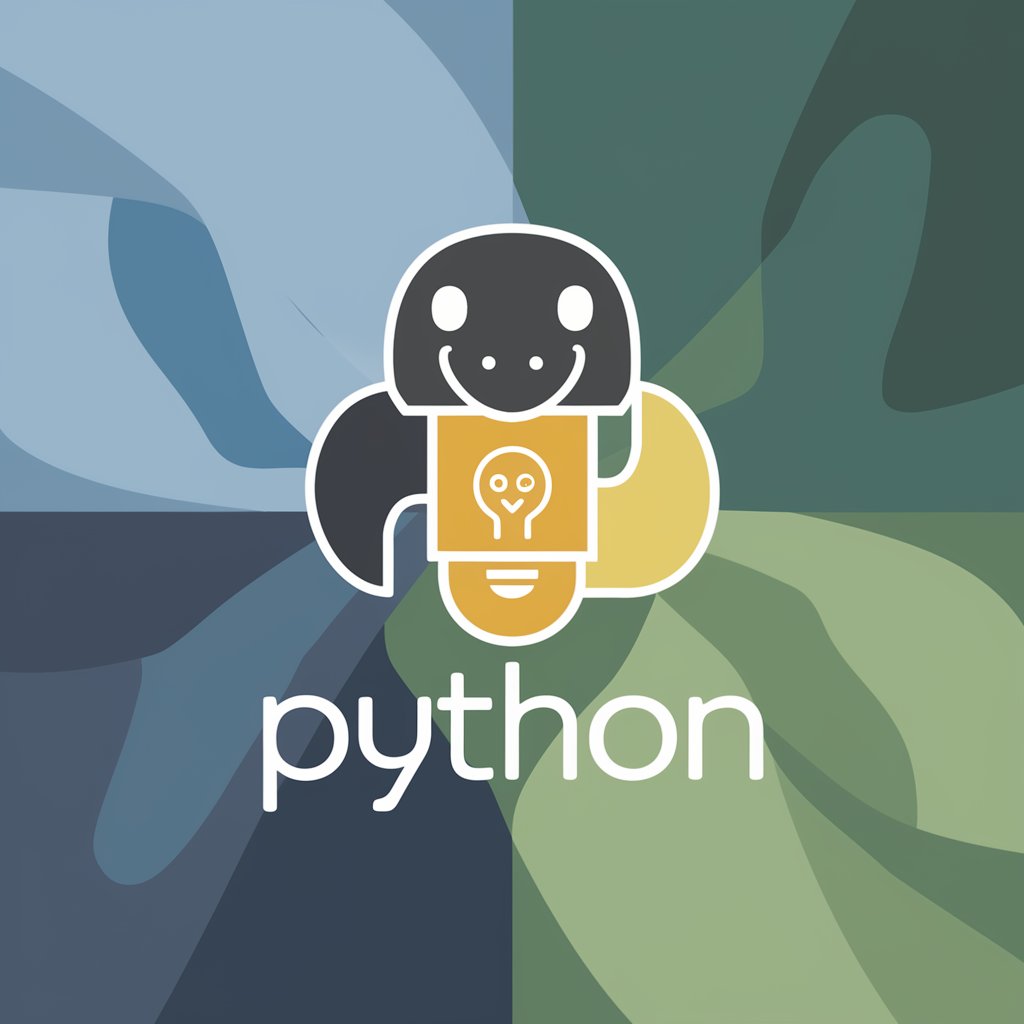
P3ER: Python Pair Programmer
Python expert for refactoring, testing, and coding advice.
Introduction to Pytorch Transformer Model Expert
The Pytorch Transformer Model Expert is designed as a specialized service aimed at providing deep insights and assistance with Pytorch's transformer models. This service focuses on leveraging the advanced capabilities of transformer models, which are a class of deep learning models that have gained prominence for their effectiveness in handling sequential data, notably in natural language processing (NLP) and computer vision. The design purpose behind this expert service is to facilitate a deeper understanding of transformer architecture, including its encoder-decoder structure, attention mechanisms, and practical implementation in Pytorch. By offering detailed explanations, coding examples, model training guidance, and optimization strategies, it aims to serve both educational and practical needs. An example scenario where this service proves invaluable is in developing a machine translation system where understanding and implementing the multi-head attention mechanism is crucial for capturing the nuances of different languages. Powered by ChatGPT-4o。
Main Functions of Pytorch Transformer Model Expert
Comprehensive Explanation of Transformer Architecture
Example
Explaining the encoder-decoder framework and the self-attention mechanism, providing the foundational knowledge necessary for building complex NLP models.
Scenario
A university research team working on a project to improve machine translation models seeks an in-depth understanding of transformer architecture to enhance their model's accuracy.
Practical Implementation Guidance
Example
Step-by-step tutorials on implementing transformer models in Pytorch, including data preparation, model construction, and training.
Scenario
A startup developing a chatbot for customer service needs practical guidance on implementing a transformer model to understand and respond to customer queries effectively.
Model Training and Optimization Techniques
Example
Providing advanced strategies for training transformer models efficiently, including learning rate scheduling, batch size optimization, and avoiding overfitting.
Scenario
A tech company aims to refine their sentiment analysis model to better gauge public opinion on social media, requiring efficient training and optimization techniques to handle large datasets.
Ideal Users of Pytorch Transformer Model Expert Services
Researchers and Academics
Individuals or teams in academia researching NLP, computer vision, or any field where sequential data plays a crucial role. They benefit from deep dives into transformer theory, model architecture, and the latest advancements in the field.
AI and Machine Learning Engineers
Professionals developing AI-driven solutions who need to implement, train, and optimize transformer models for applications such as language translation, text summarization, or image captioning.
Data Science Students
Learners seeking to enhance their knowledge and practical skills in deep learning, especially those interested in the cutting-edge field of transformers, through guided tutorials and hands-on projects.
How to Use Pytorch Transformer Model Expert
Start Free Trial
Begin by visiting yeschat.ai for a hassle-free trial, accessible without login or the need for a ChatGPT Plus subscription.
Explore Documentation
Familiarize yourself with the documentation to understand the tool's capabilities and prerequisites, such as Python and PyTorch knowledge.
Install PyTorch
Ensure PyTorch is installed in your environment. This is crucial for running transformer models.
Experiment with Models
Use the tool to experiment with different transformer architectures, fine-tuning them for your specific data or project needs.
Utilize Community Resources
Engage with the user community for tips, shared models, and best practices to enhance your experience and results.
Try other advanced and practical GPTs
Kotlin Range Expressions: Simplify Your Code
Streamline Your Kotlin Code with AI-Powered Range Expressions
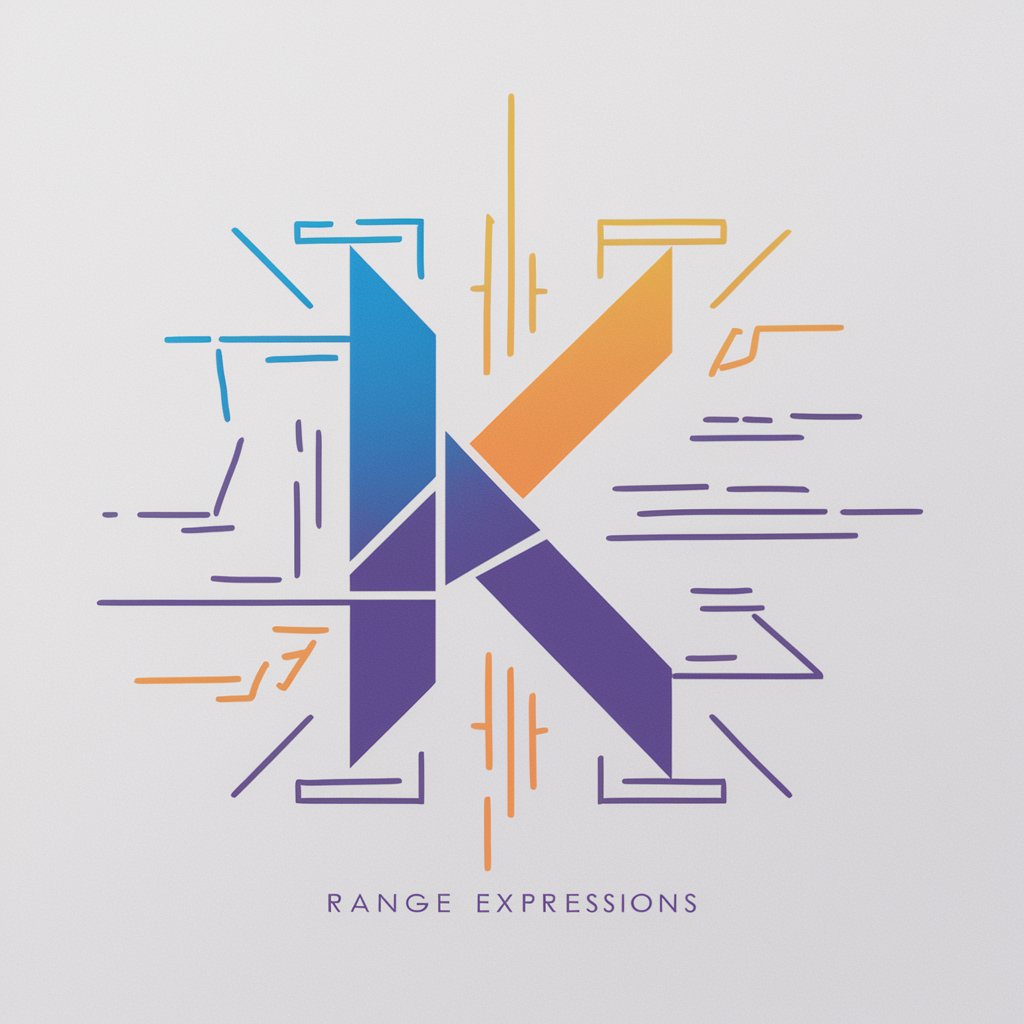
Code Master AI
Streamlining Code Generation with AI

CMO Message Mapper
Craft Clear Messages with AI
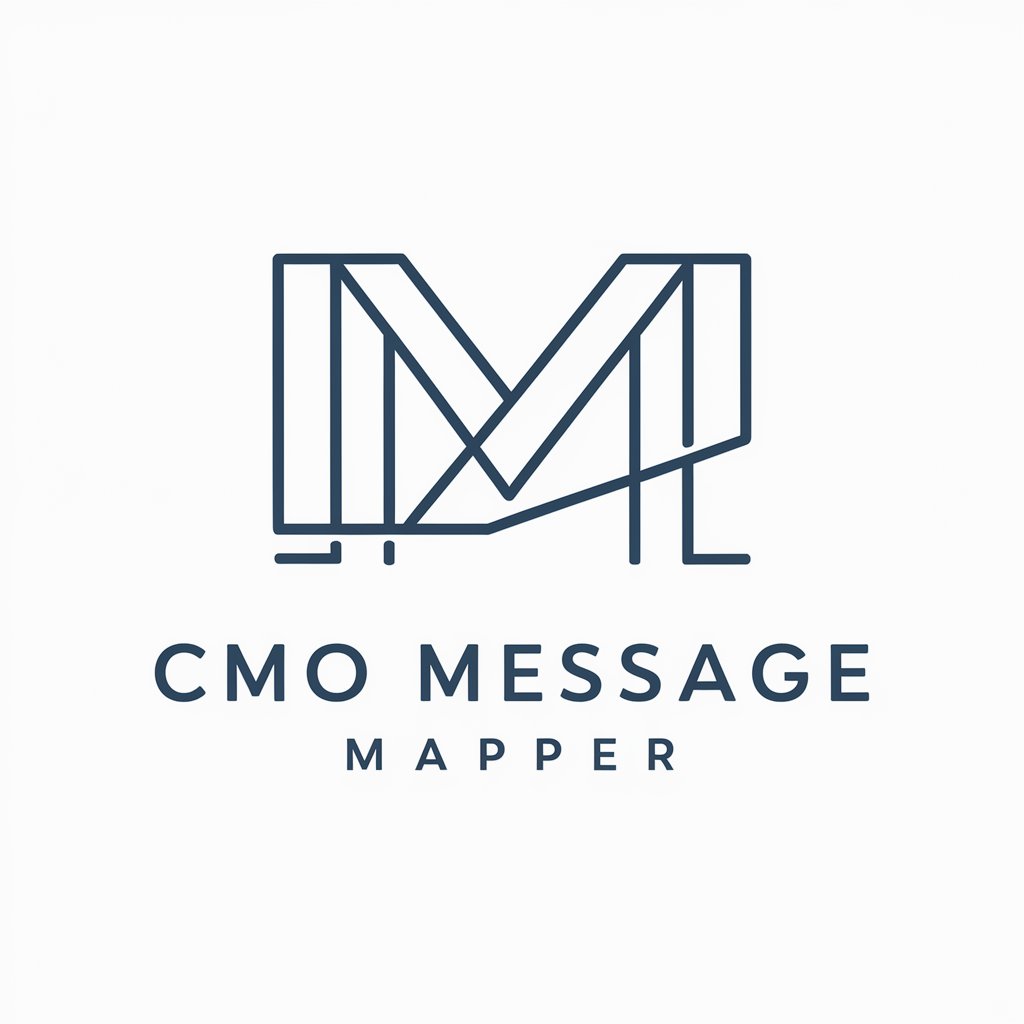
Random Name Picker
Fairness powered by AI
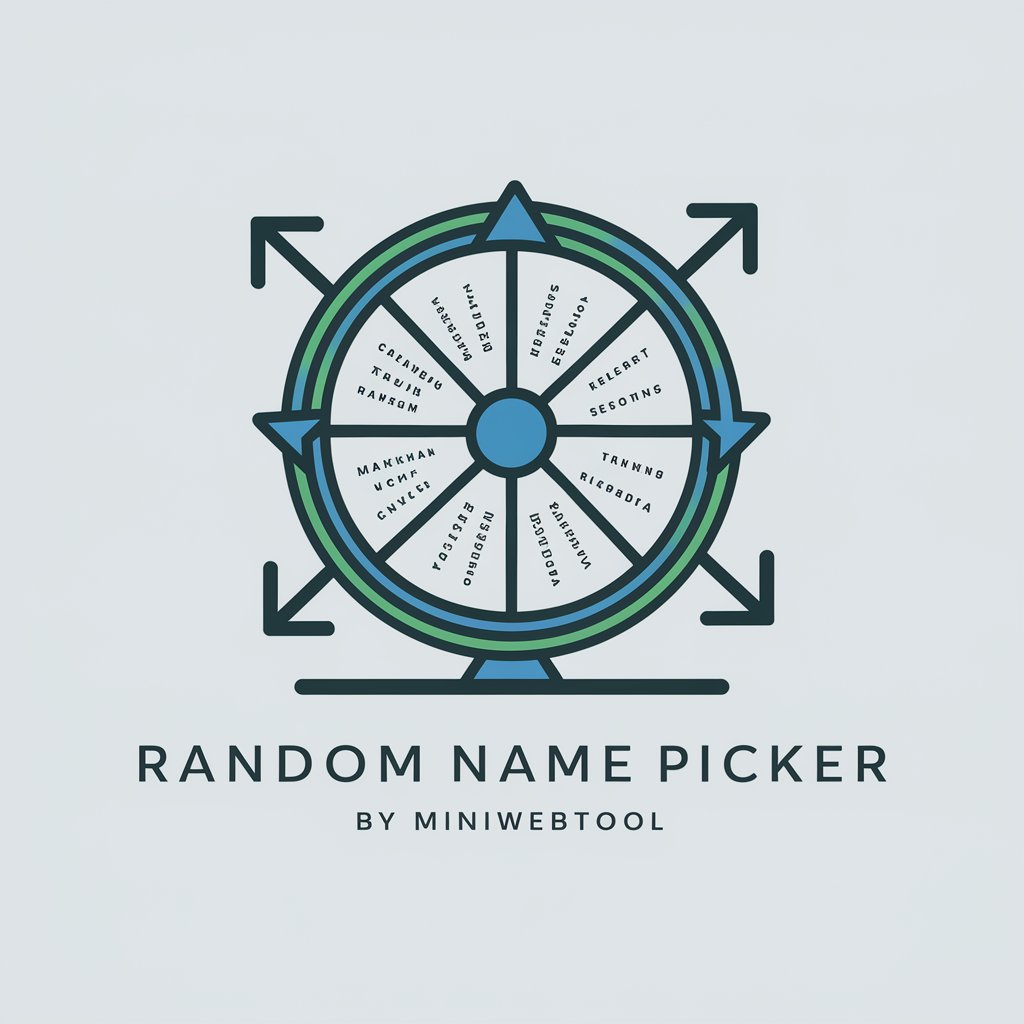
Personal Baby Name Assistant
Discover the Perfect Name with AI
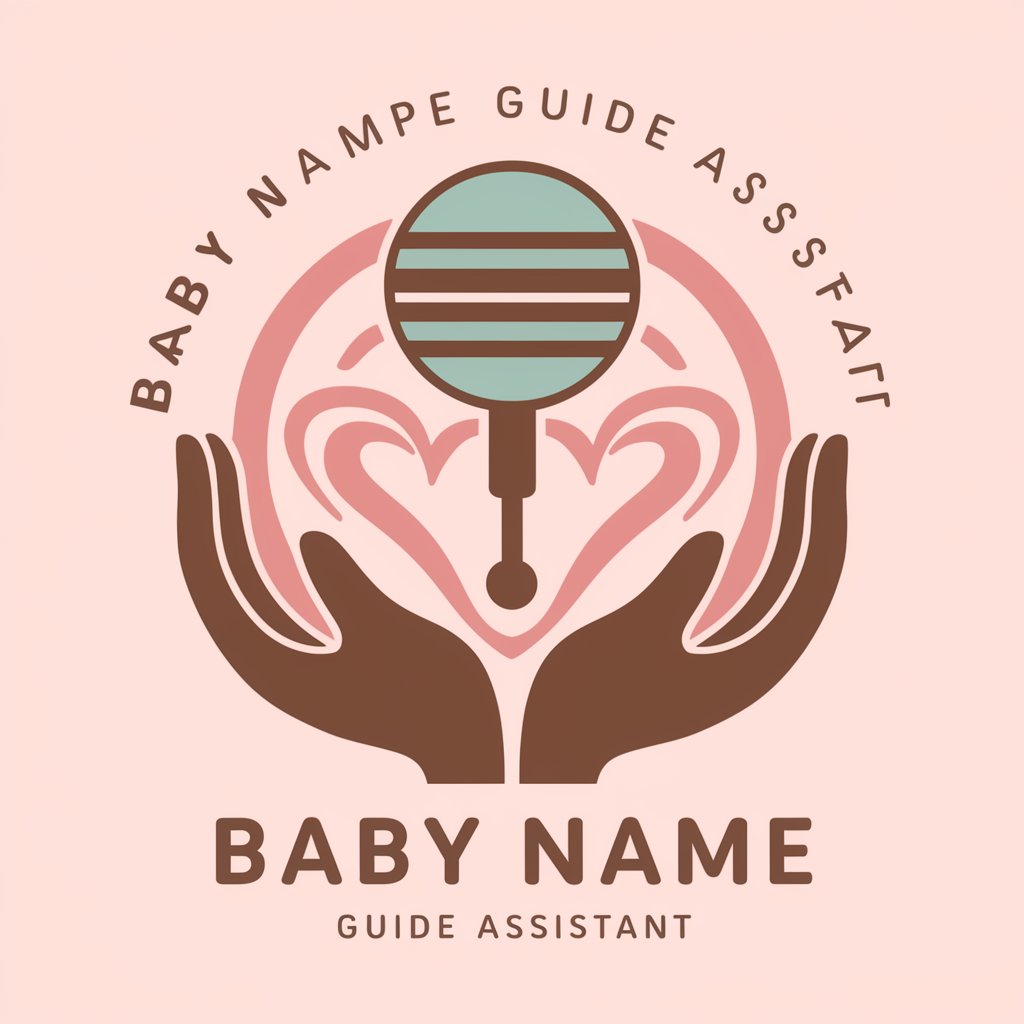
Public Writer
Personalize every letter with AI
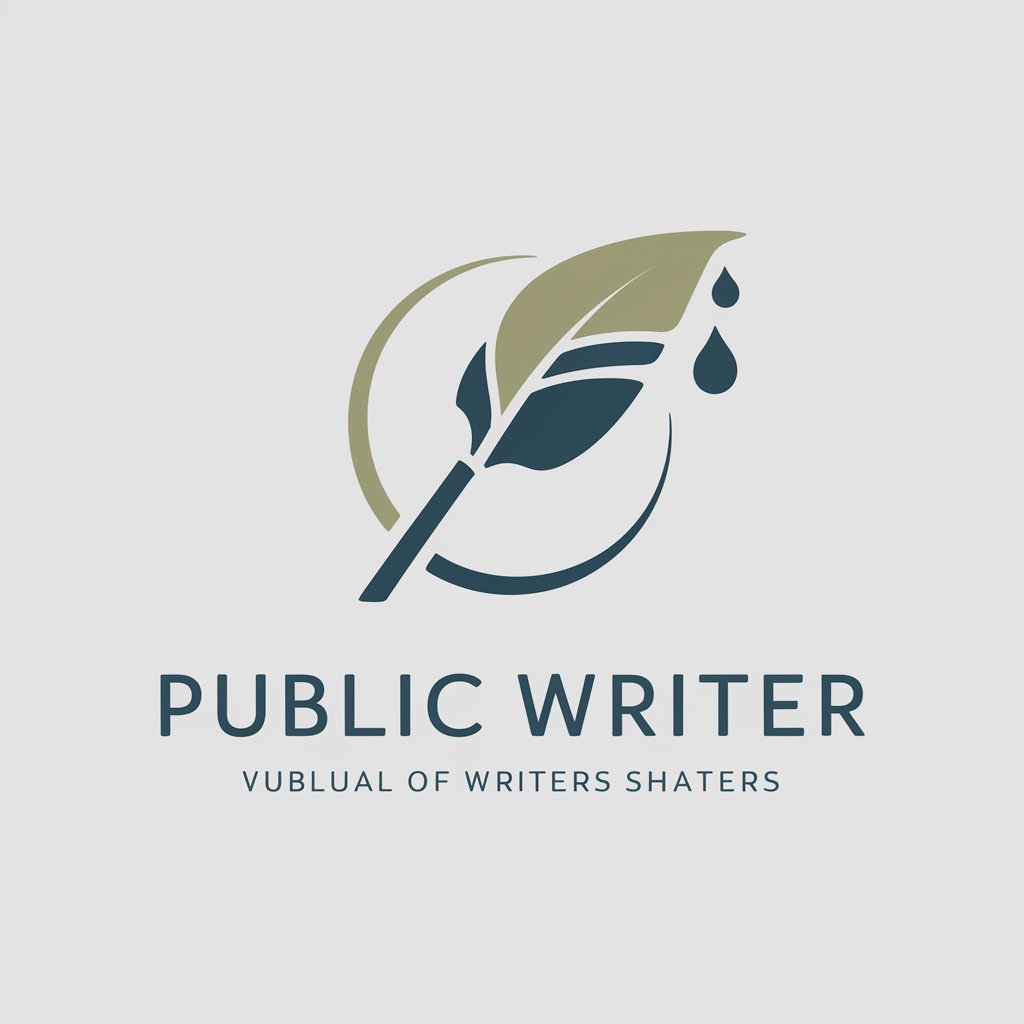
Search internet and Return the Newest Information
Empowering Inquiries with AI-driven Insights
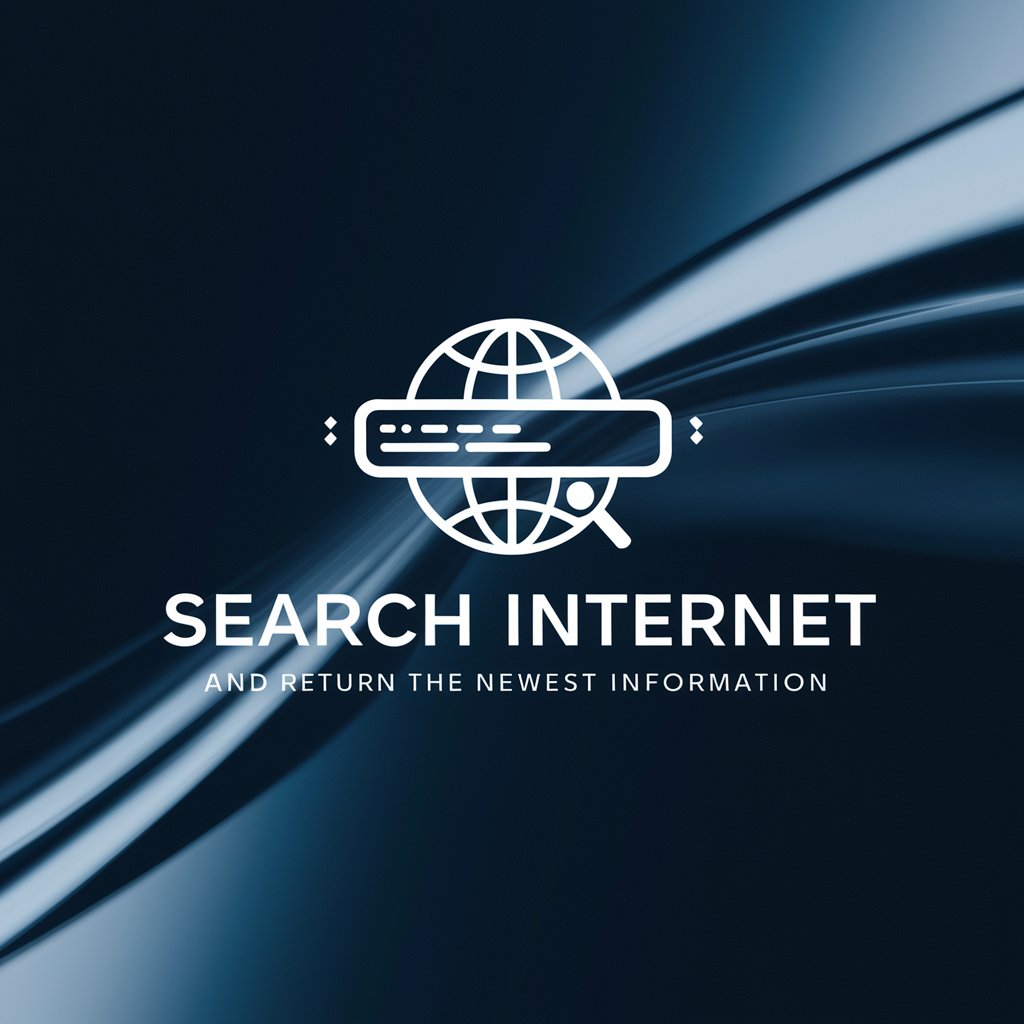
Internet IMD Mentor
Empowering Community Networks with AI
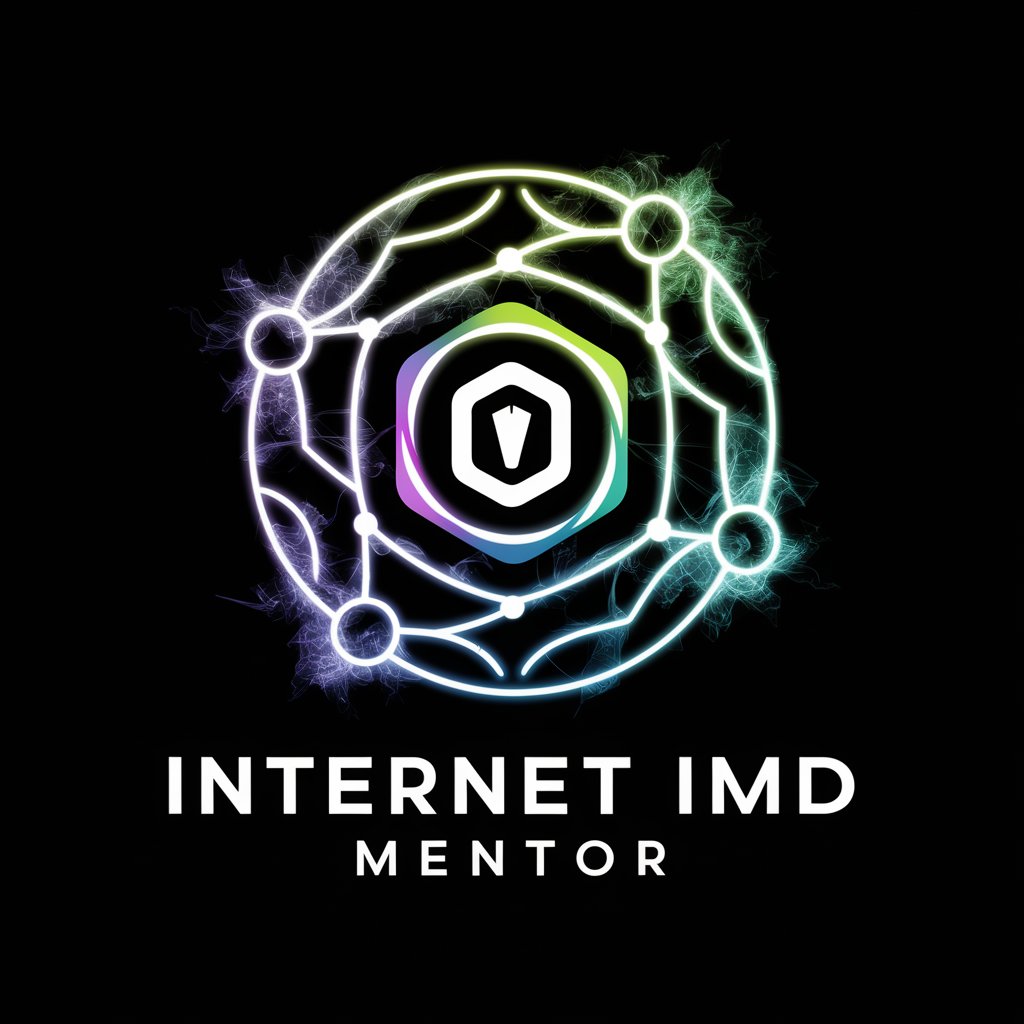
G6PD Guardian
Navigate G6PD Safely with AI
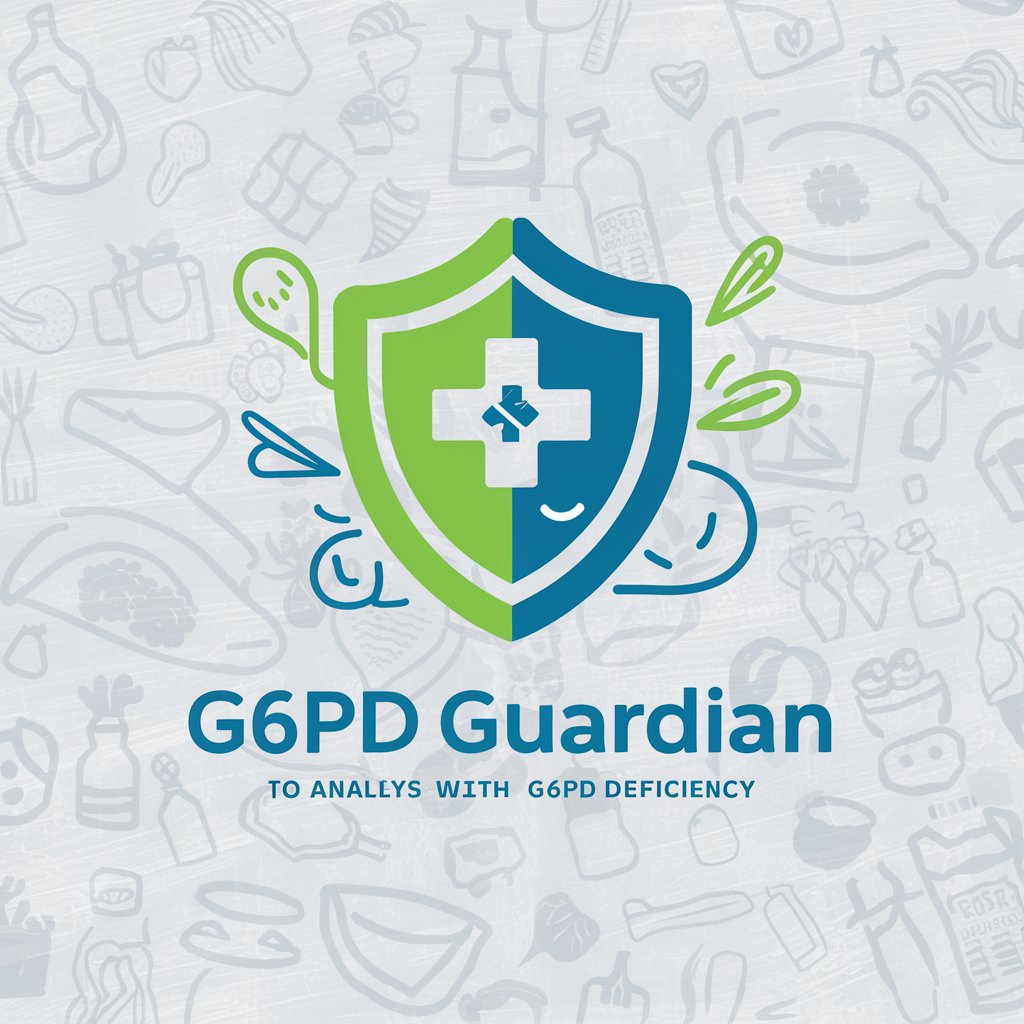
Knowledge Center
Empowering AWS Solutions with AI
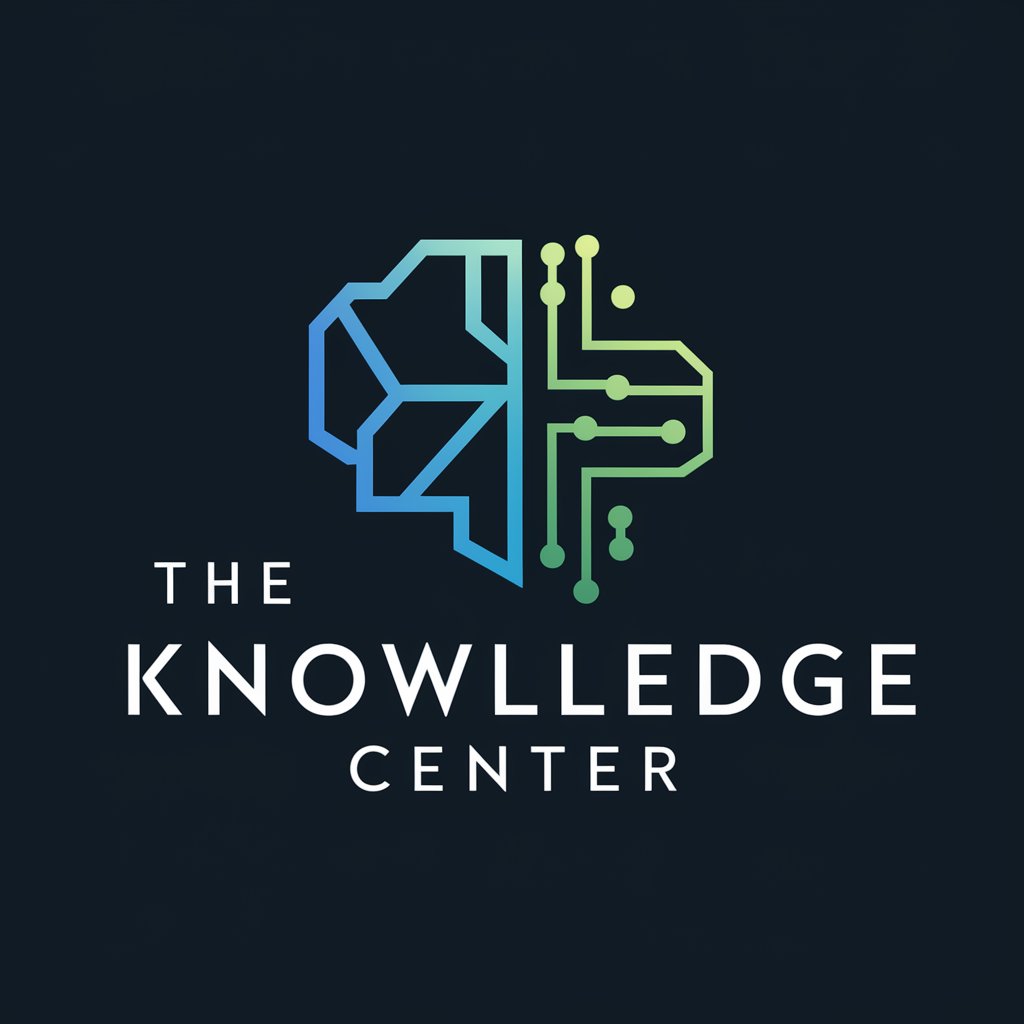
Efficient ML Algorithms in C: Performance Mastery
Power your C projects with AI-driven ML efficiency.
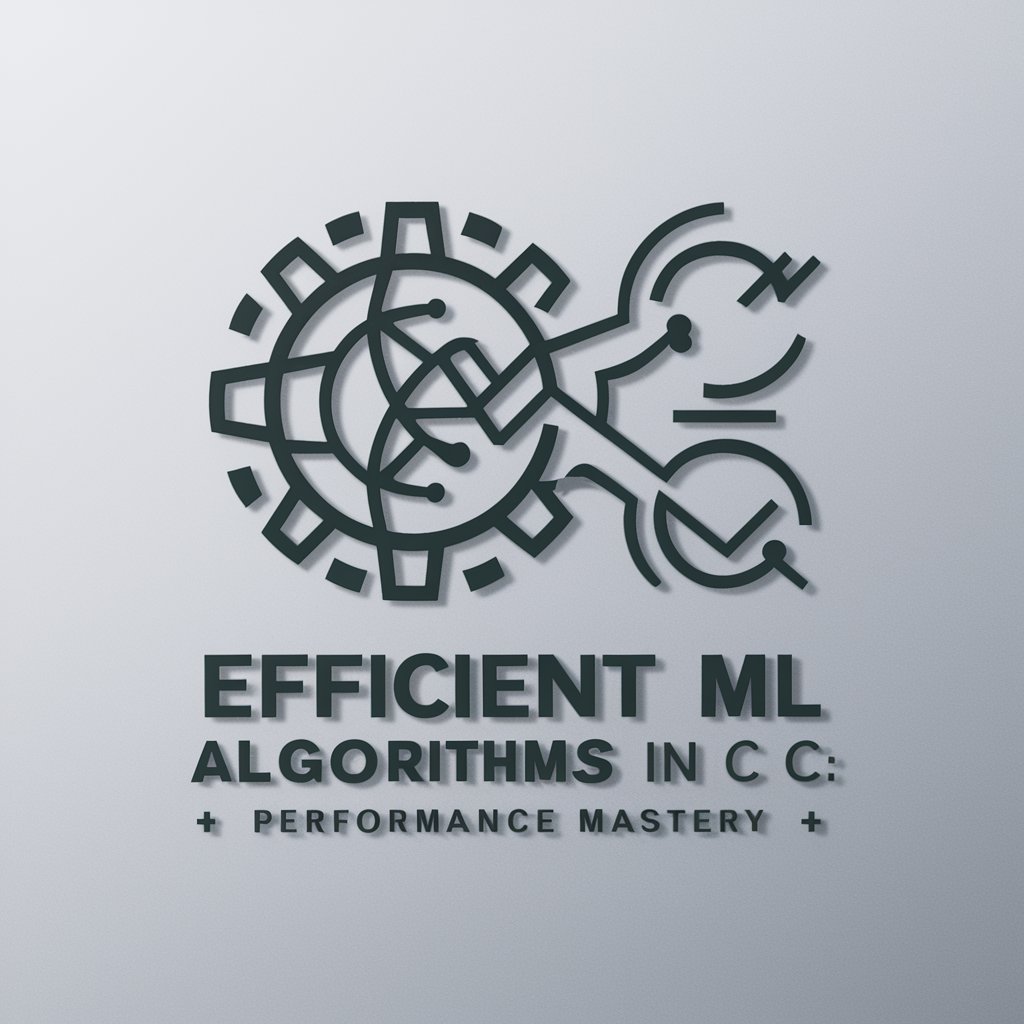
Spark Data Revolution
Empower your data with AI-driven Spark optimization.
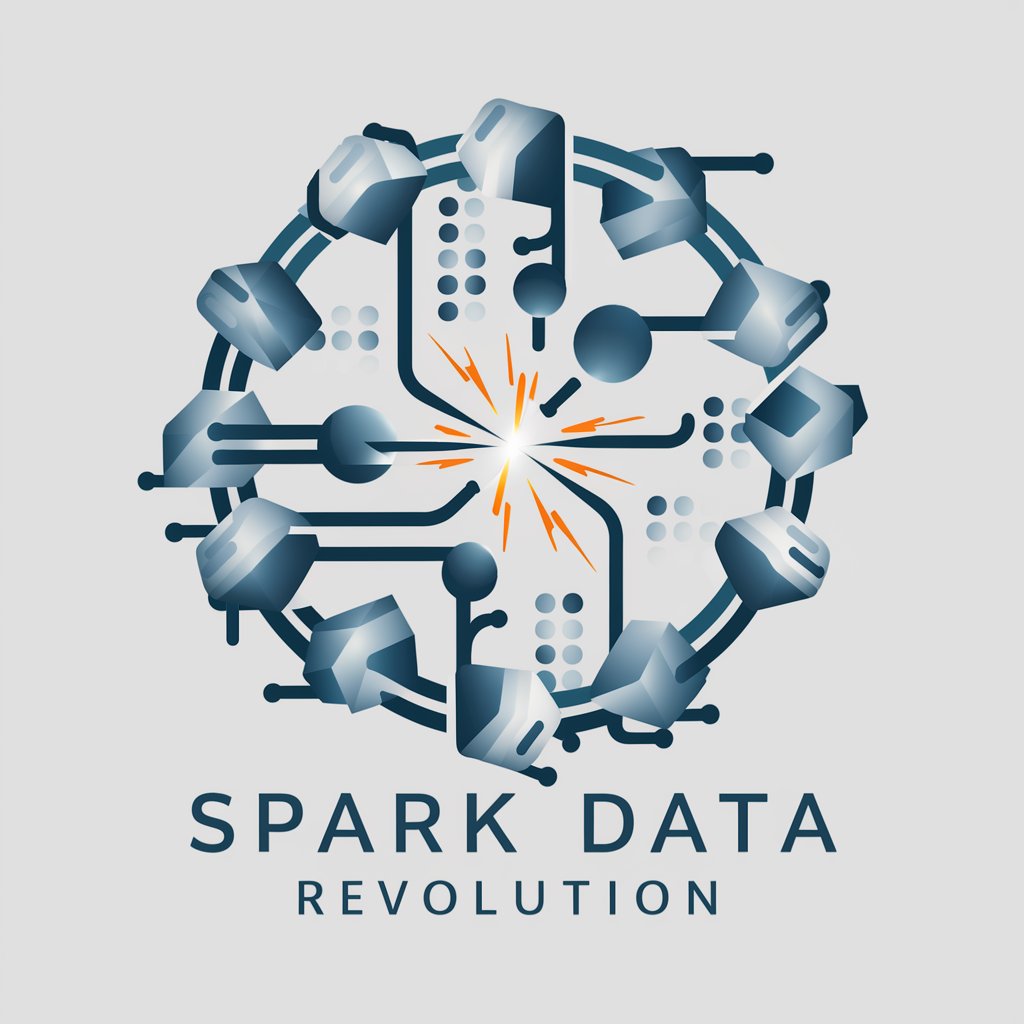
Q&A about Pytorch Transformer Model Expert
What is Pytorch Transformer Model Expert?
It's a specialized tool designed to simplify working with transformer models in PyTorch, providing functionalities for model training, evaluation, and implementation.
Can I use it without prior machine learning knowledge?
While it's accessible to beginners, a foundational understanding of machine learning concepts and PyTorch is recommended to fully leverage the tool's capabilities.
What are the common use cases?
It's widely used in natural language processing tasks, such as text classification, language modeling, and machine translation, as well as for research and educational purposes.
Is there support for custom transformer models?
Yes, the tool supports customization and fine-tuning of pre-existing models or the creation of new transformer architectures to suit specific project needs.
How can I optimize my model's performance?
Optimizing performance involves experimenting with different hyperparameters, using advanced training techniques, and leveraging the tool's resources for best practices.