LIMB LAB - Research Paper Analysis
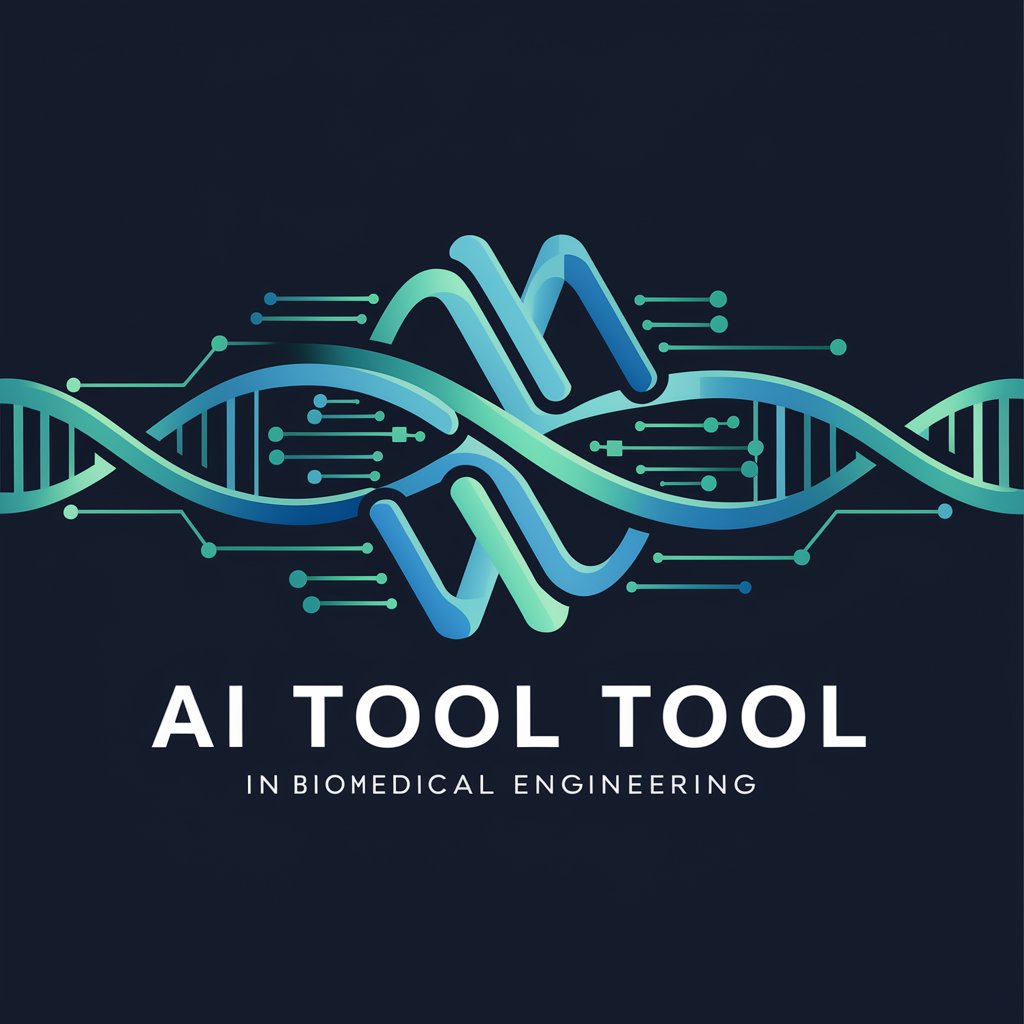
Welcome to LIMB LAB, your AI-powered research assistant!
Streamlining research with AI-powered insights
Summarize the key findings of the research paper on...
Explain the methodology used in the study about...
What are the implications of the research on...
Compare the bioprinting strategies discussed in the papers...
Get Embed Code
Introduction to LIMB LAB
LIMB LAB is designed as a specialized GPT model to optimize the processing, summarization, and analysis of research papers, particularly within fields that entail complex data and require nuanced understanding, such as regenerative medicine and bioengineering. Through its unique capabilities, LIMB LAB aims to bridge the gap between extensive research documents and actionable insights, making it easier for researchers, academics, and professionals to grasp the core findings, methodologies, and implications of diverse studies. An illustrative scenario could involve LIMB LAB summarizing a research paper on the efficacy of a new biomaterial for tissue engineering, where it would extract key results, analyze data trends, and suggest potential applications or areas for further research, thus aiding in the quick assimilation of vital information. Powered by ChatGPT-4o。
Main Functions of LIMB LAB
Paper Summarization
Example
Summarizing a research paper on nanoengineered scaffolds for muscle tissue engineering, highlighting the scaffold's composition, the experimental setup, key findings, and implications for future research.
Scenario
A team of bioengineers is exploring cutting-edge materials for enhancing muscle regeneration. Utilizing LIMB LAB's summarization, they quickly understand the novel scaffold's potential, streamlining their decision-making process for experimental design.
Research Insight Generation
Example
Generating insights from a study on in vivo bioprinting techniques, discussing its advancements, limitations, and comparing it with traditional methods.
Scenario
A researcher considering in vivo bioprinting for a regenerative medicine project uses LIMB LAB to grasp how new techniques might overcome existing challenges, guiding the project's direction with informed strategy.
Query Answering
Example
Providing detailed explanations on the bioink formulation used in a specific study on cartilage tissue engineering.
Scenario
A doctoral student working on their thesis on bioinks for cartilage regeneration queries LIMB LAB for specifics on a novel formulation, enabling a deeper analysis in their literature review.
Trend Analysis
Example
Identifying trends in scaffold material selection across recent tissue engineering research.
Scenario
A material scientist uses LIMB LAB to track evolving material choices in tissue engineering, informing their research on new biomaterials development.
Cross-Paper Linking
Example
Linking related research findings on the effectiveness of growth factor delivery systems in tissue engineering.
Scenario
A biomedical engineering team leverages LIMB LAB to connect disparate studies on growth factor delivery, facilitating a comprehensive review for their project proposal.
Ideal Users of LIMB LAB
Academic Researchers
Individuals and teams in universities and research institutions who are actively involved in conducting and analyzing studies on regenerative medicine, tissue engineering, and related fields. They benefit from LIMB LAB by gaining rapid access to synthesized research insights, which can inform their experimental designs, grant proposals, and publications.
Biomedical Engineers
Professionals working in the development of medical devices, biomaterials, and therapeutic strategies who require a deep understanding of the latest research findings to innovate and refine their projects. LIMB LAB offers them a concise summary of relevant studies, saving time and enhancing the depth of their projects.
Healthcare Professionals
Clinicians and surgeons interested in the latest advancements in regenerative medicine and how they can be applied to improve patient care. Through LIMB LAB, they can stay updated on groundbreaking research without sifting through numerous academic papers, enabling them to offer more informed treatment options.
Guidelines for Using LIMB LAB
Initiate your journey
Head over to yeschat.ai to explore LIMB LAB's capabilities through a free trial, no ChatGPT Plus subscription or login required.
Choose your research focus
Select the research paper or topic you're interested in. LIMB LAB can process and summarize research papers, providing key findings, methodologies, and insights.
Query customization
Input your specific queries related to the research papers for customized insights, such as experimental methods, results interpretation, and theoretical background.
Engage with insights
Use the insights generated by LIMB LAB to enhance your research understanding, identify trends, and explore cross-paper linkages for a comprehensive understanding.
Continuous learning
Regularly update your research topics and queries based on evolving lab needs and LIMB LAB's continuous learning capabilities for the most current insights.
Try other advanced and practical GPTs
Breeze Booth GPT
Unleash creativity with AI-powered photo booths.
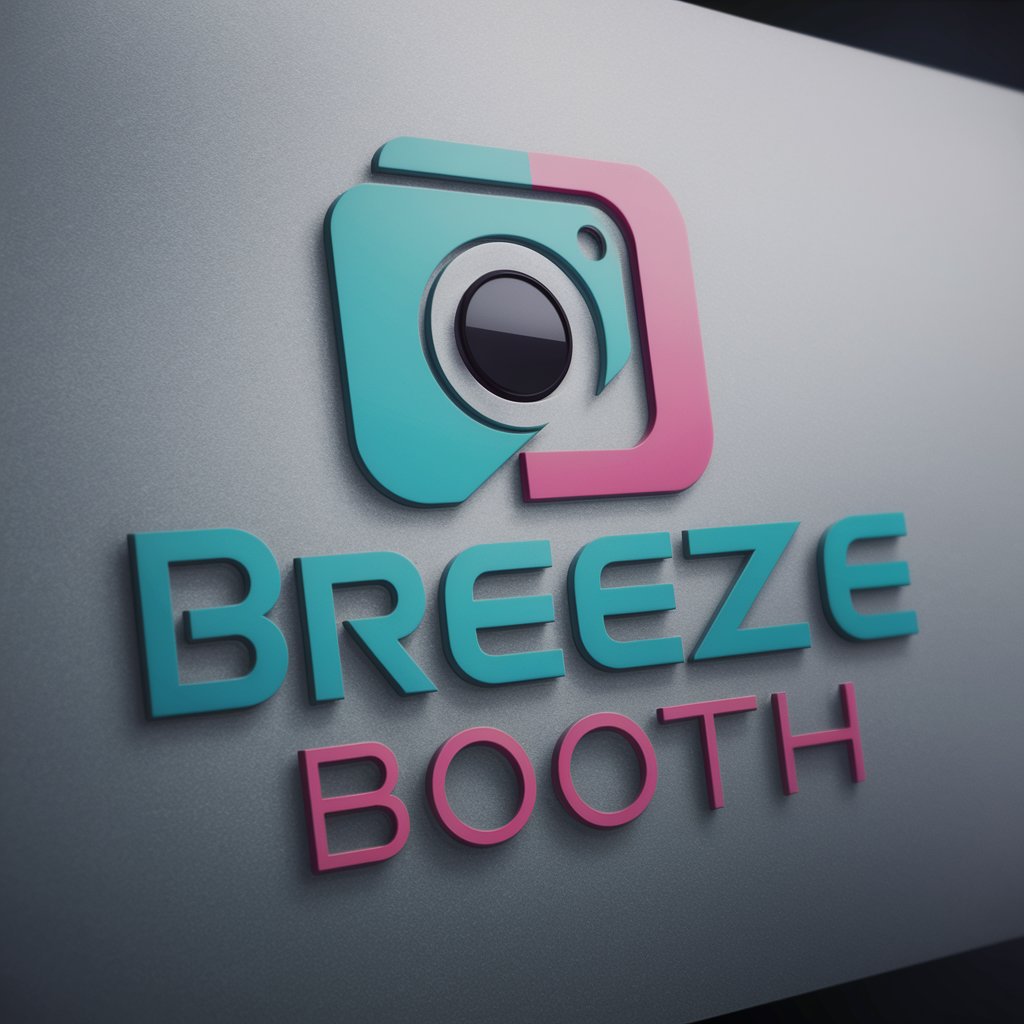
Freezer Door Cocktail Maker
Craft, Freeze, Enjoy: Revolutionizing Home Bartending
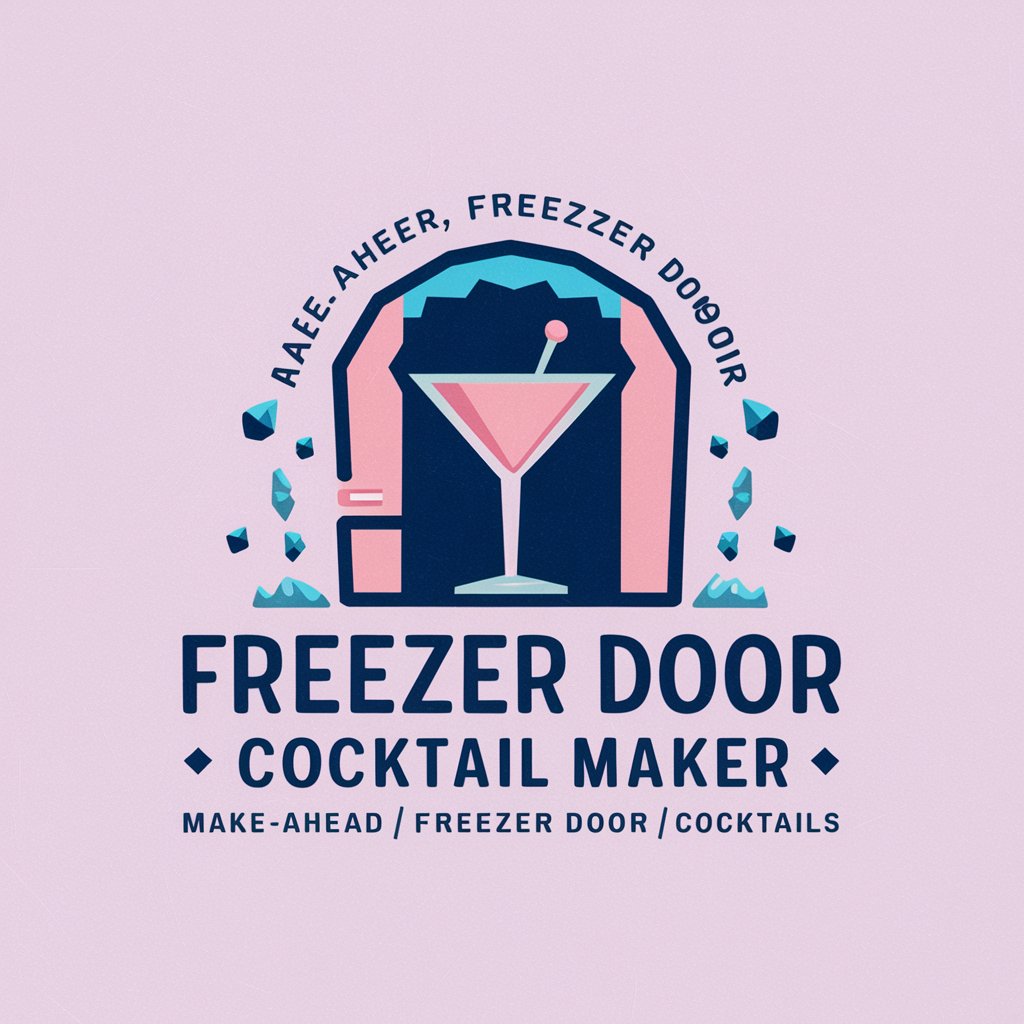
moshfit style "freeze tag - games" maker
Engage, learn, and play with AI-powered freeze tag games.
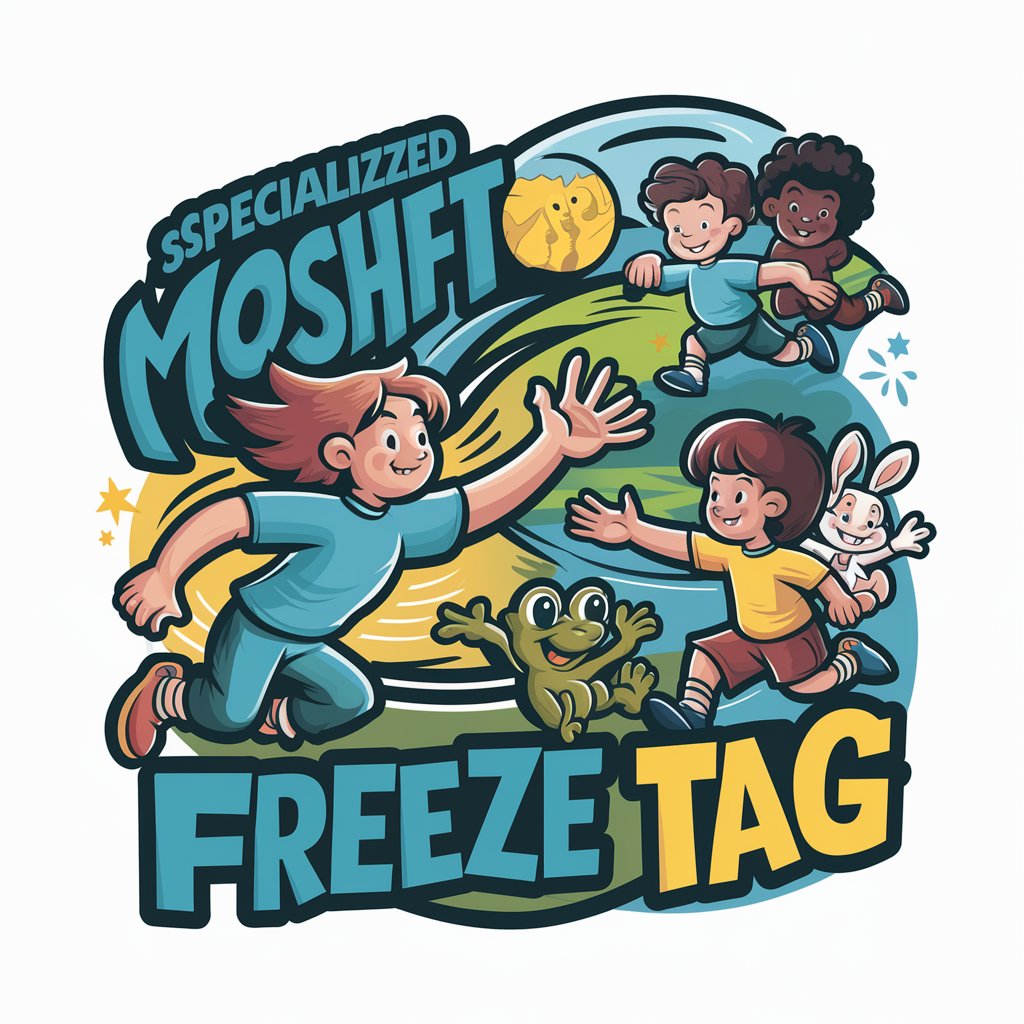
Legal+
Empowering legal solutions with AI.
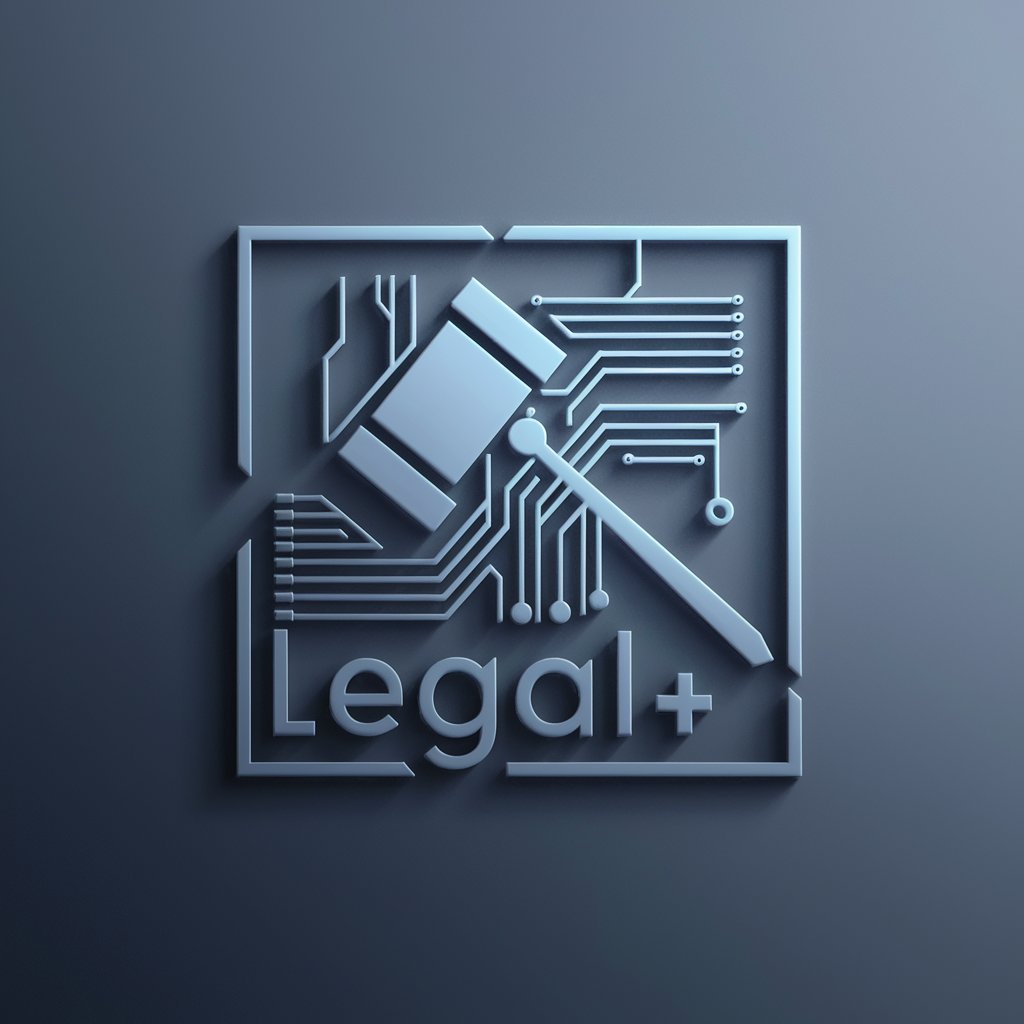
Canadian Armed Forces Competency Analyst
Empowering CAF with AI-driven Competency Insights
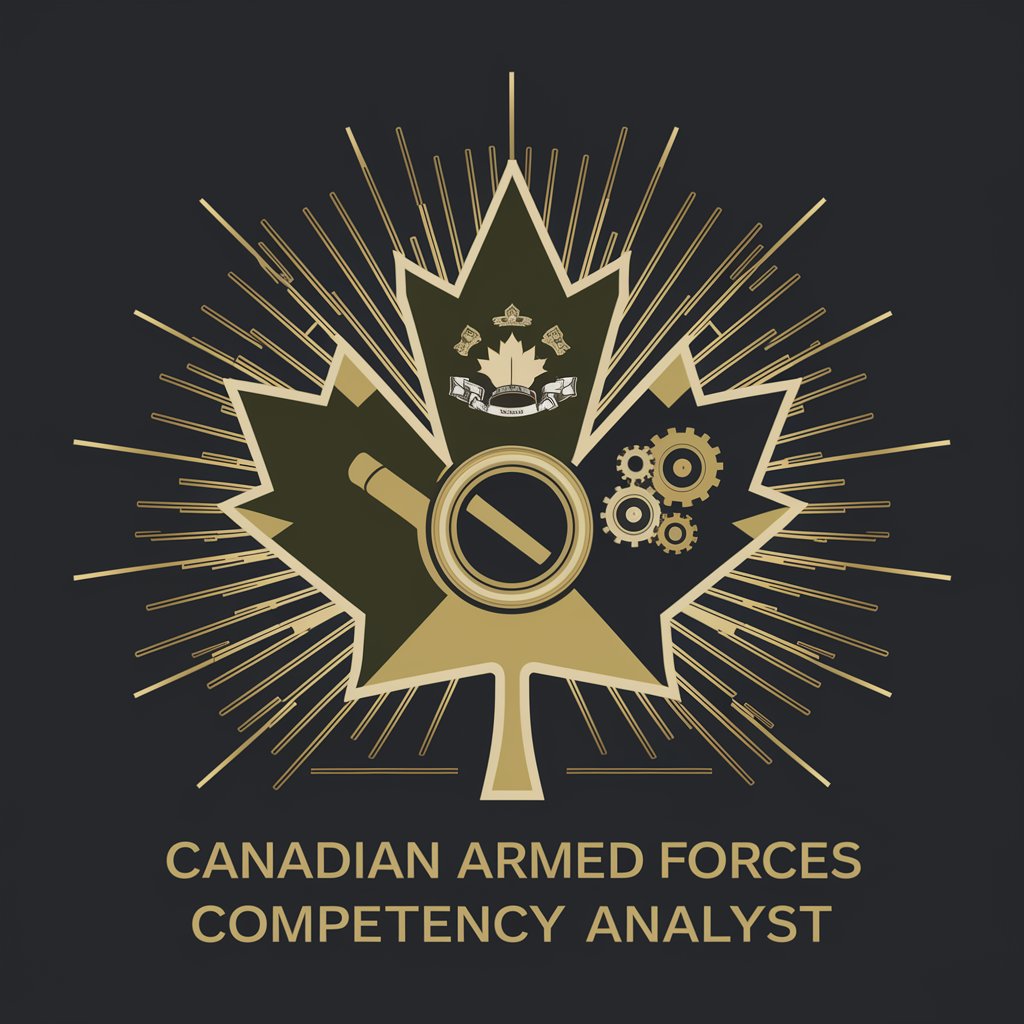
Canadian Armed Forces Chief Memo Officer
Streamlining Military Memos with AI
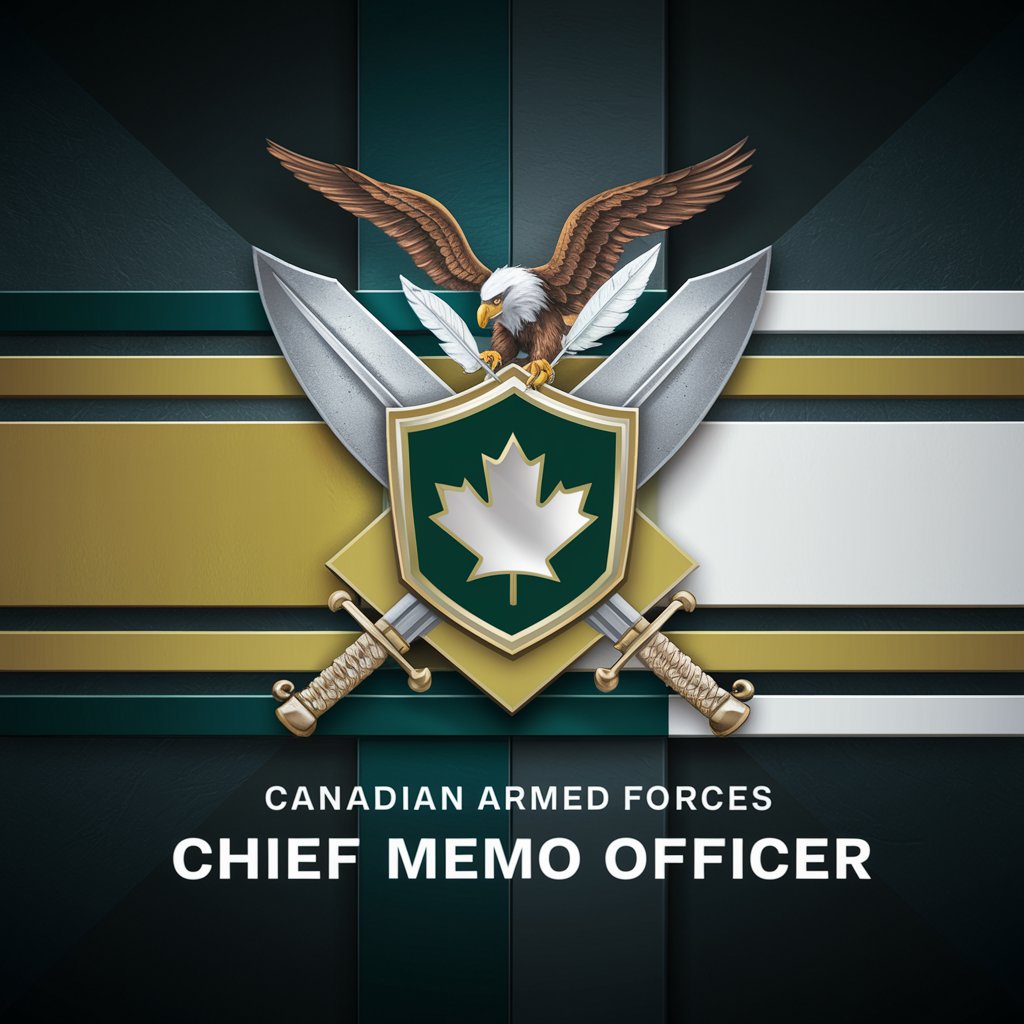
KLIMB Copywriting Expert
Empowering Your Brand with AI-Powered Copy
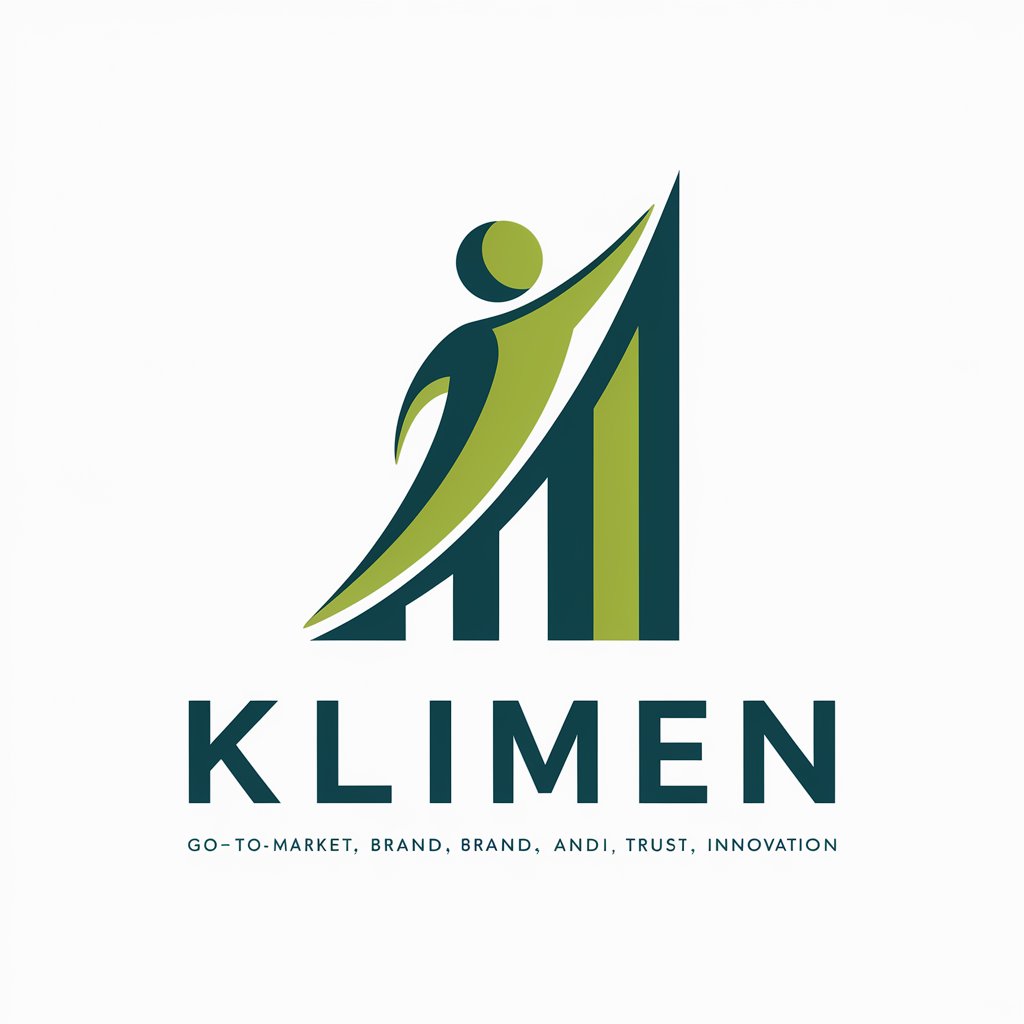
Info Assemblea Clima Catalunya
Empowering Climate Decisions with AI
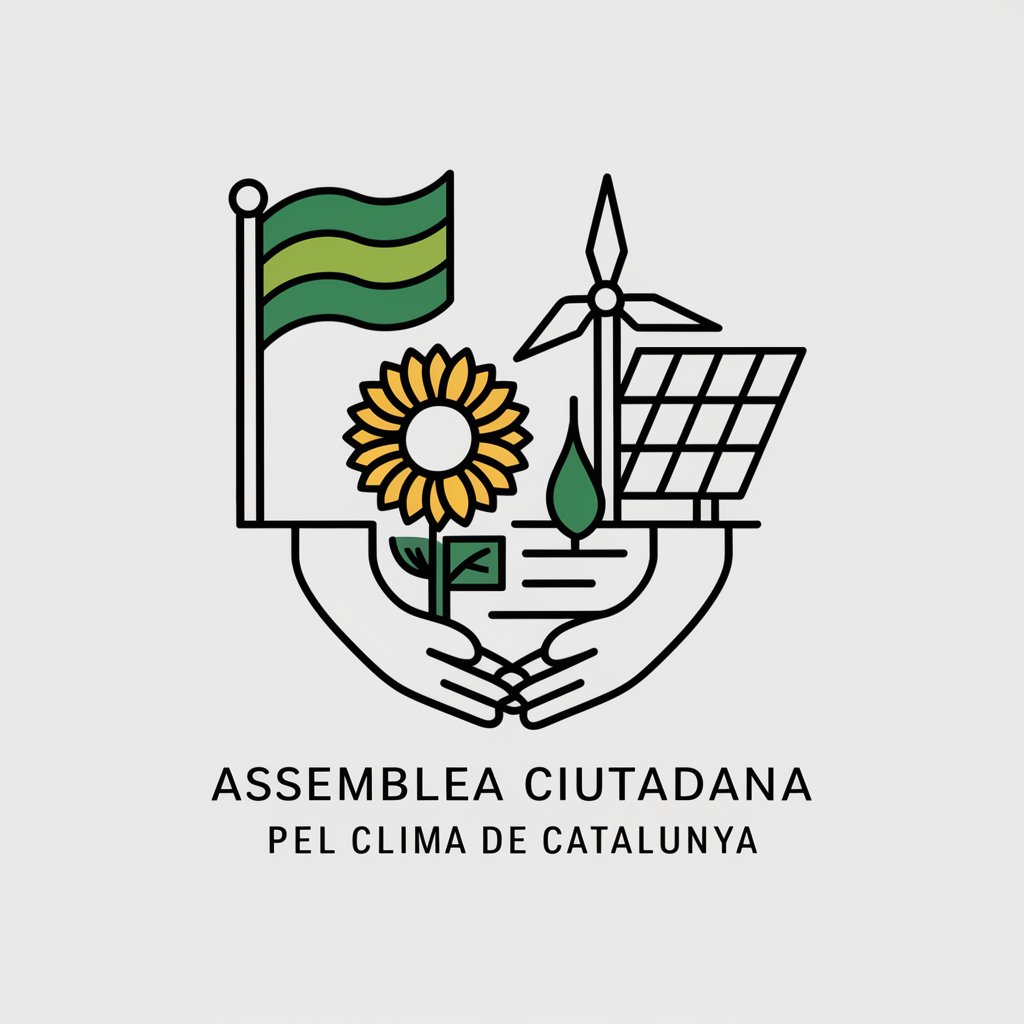
CLimT: Classical Language Translator/클림트: 고전어 번역가
Bringing Ancient Wisdom to Modern Minds
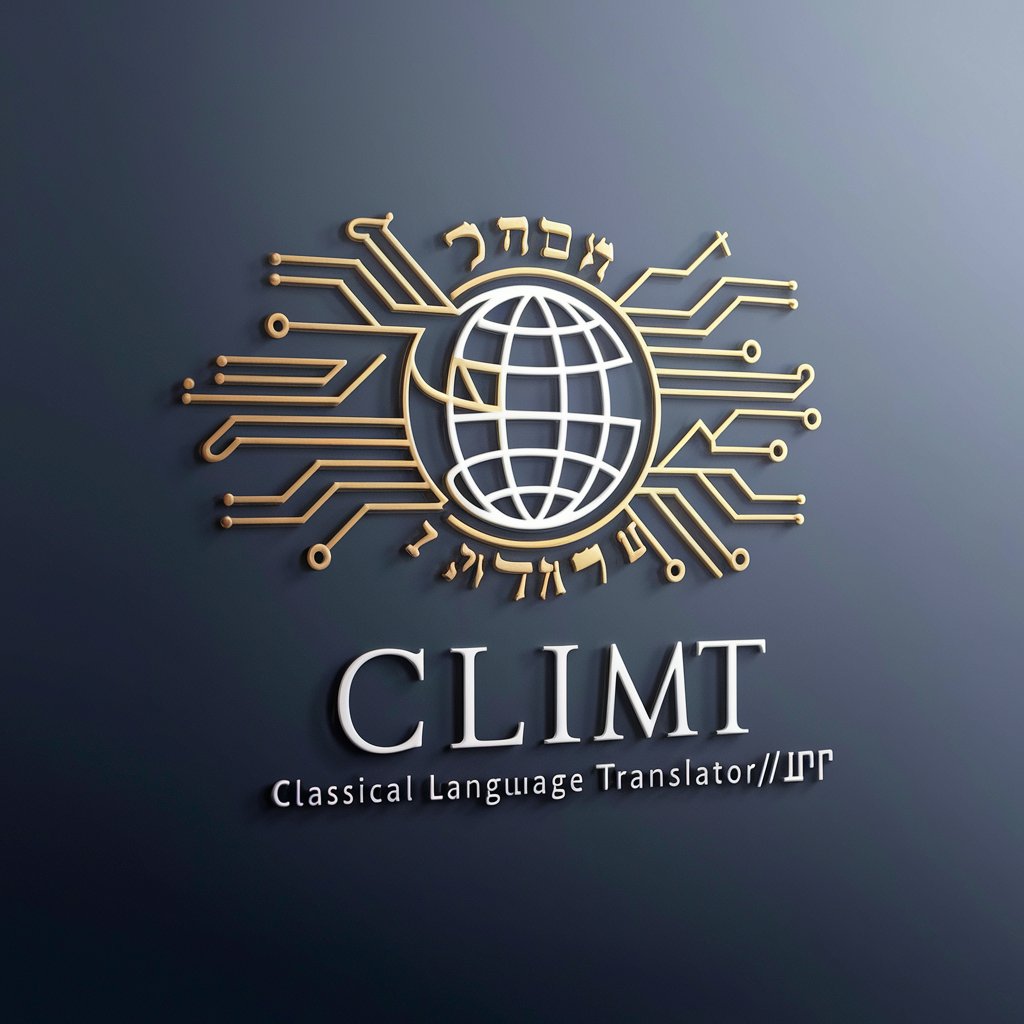
Clima-Headache Predicter
Predict headaches with AI-powered weather analysis.
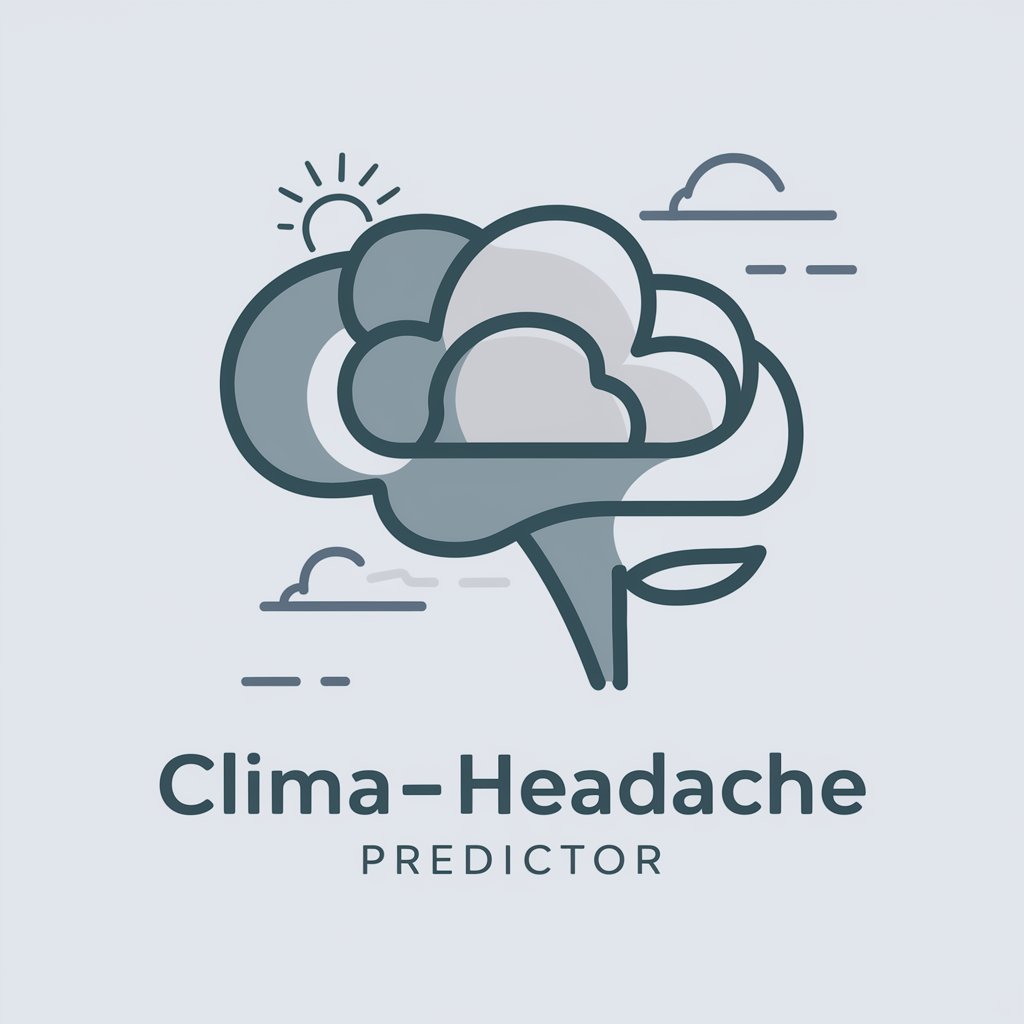
Yandex DIRECT Helper
Optimize Yandex ads with AI-powered insights
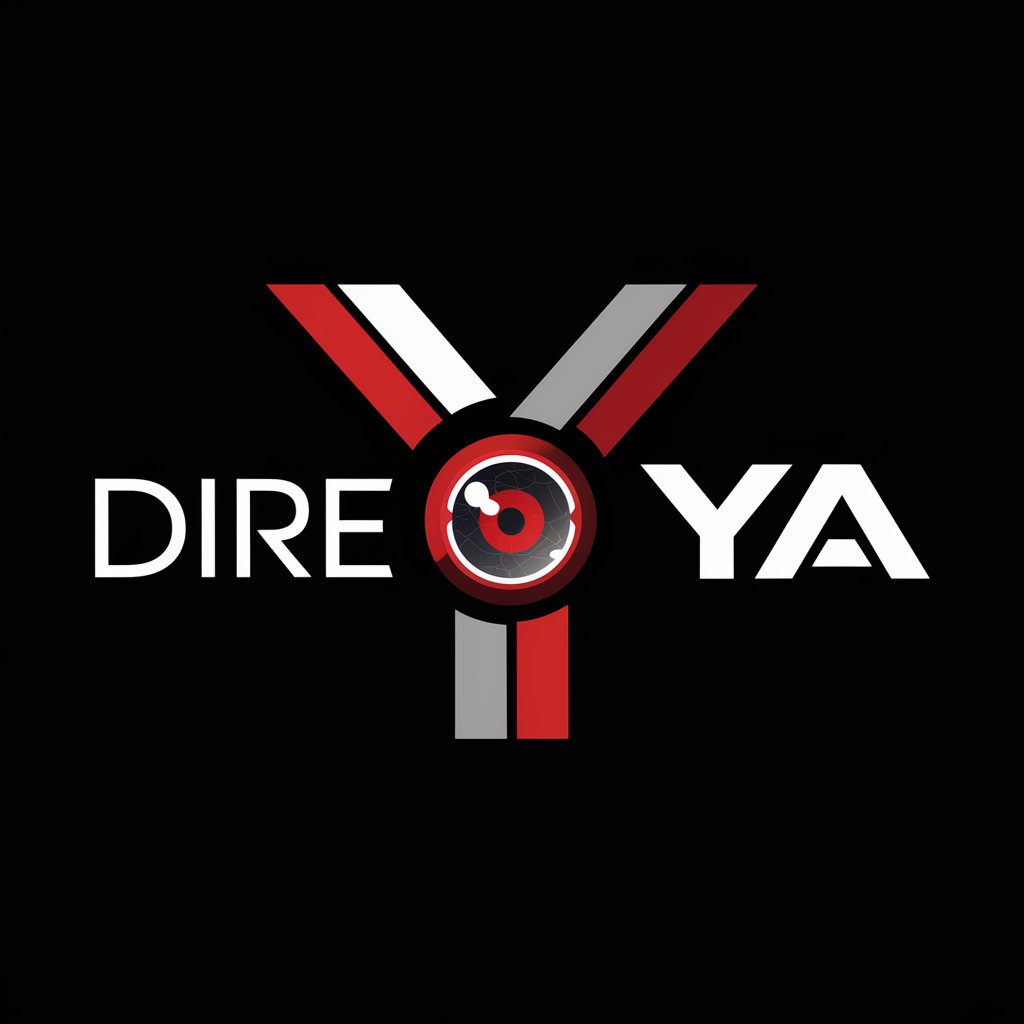
Newsletter
Streamlining Financial News with AI Precision
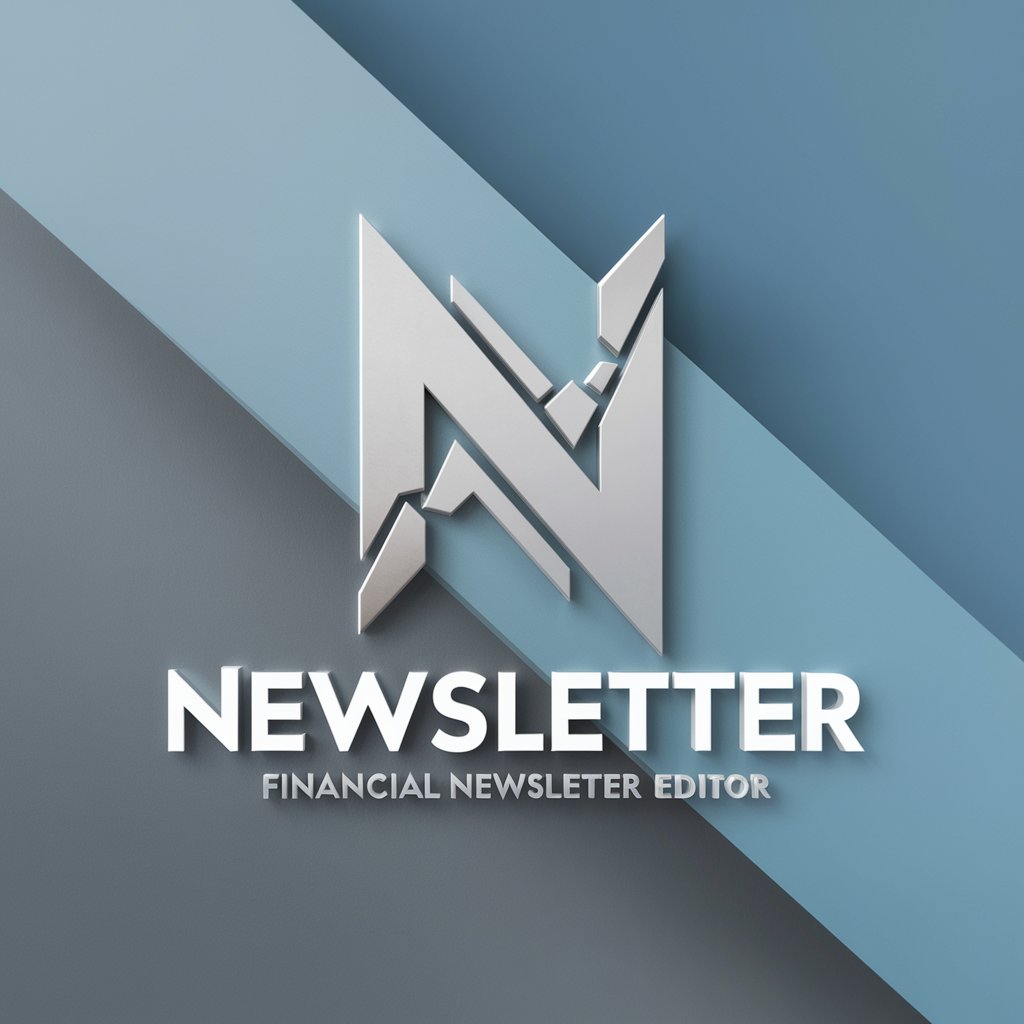
In-depth Q&A About LIMB LAB
What is LIMB LAB?
LIMB LAB is a specialized tool designed for processing and summarizing research papers, offering key findings, methodologies, and generating insights into research topics.
How does LIMB LAB ensure accuracy in data representation?
LIMB LAB prioritizes accurate representation by maintaining the integrity and context of the original research, focusing on clear and precise summaries and insights.
Can LIMB LAB identify trends in research topics?
Yes, LIMB LAB is capable of identifying trends and patterns in research topics over time, providing users with insights into the evolution of specific research areas.
Is LIMB LAB suitable for users without a deep technical background?
Absolutely, LIMB LAB is designed to offer user-friendly interaction, providing clear, concise, and easily understandable responses tailored to the user's level of expertise.
How can LIMB LAB contribute to cross-paper linking?
LIMB LAB suggests connections and references among different research papers, enhancing the user's understanding by linking related findings and methodologies across studies.