Quantum Machine Learning for Many-Body Systems-Trial, Quantum ML, Many-Body
Empowering quantum discovery with AI
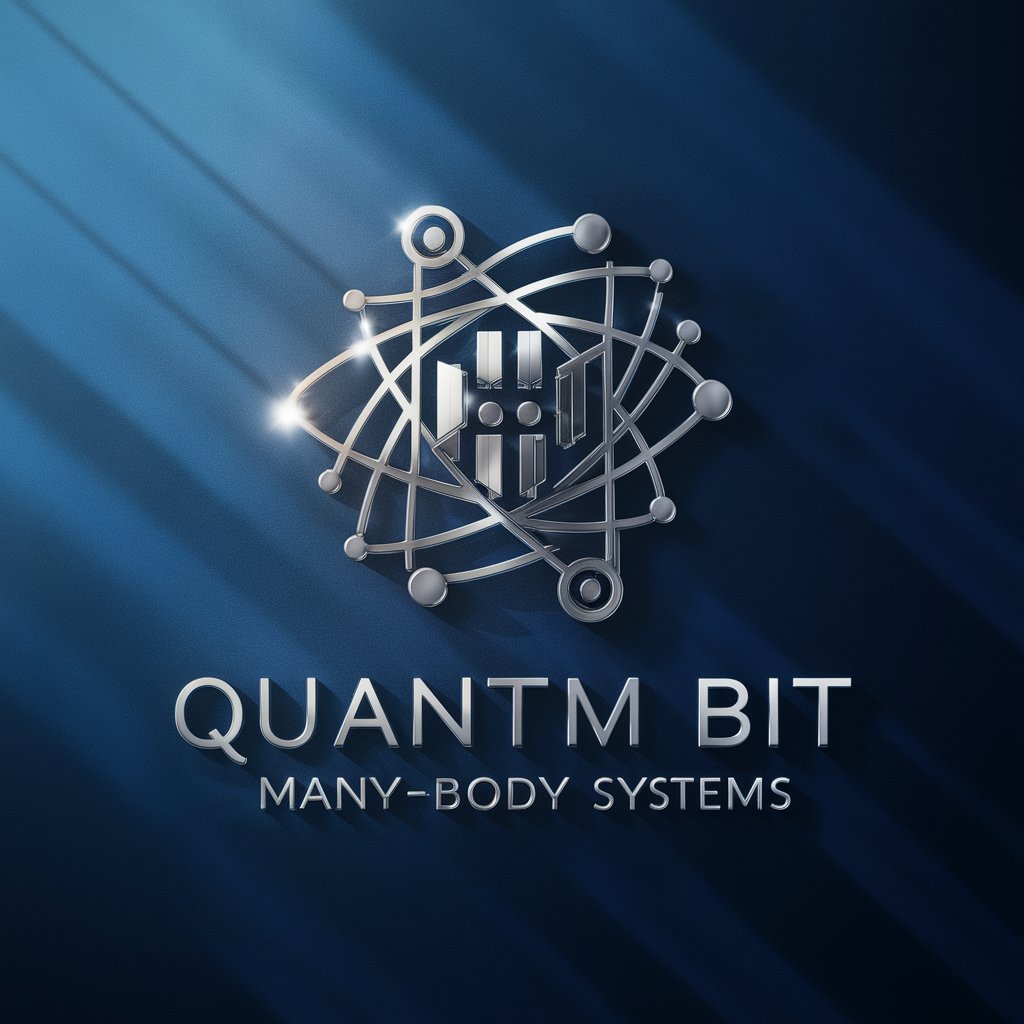
Explain the role of tensor networks in simulating many-body quantum systems.
Describe a quantum algorithm for finding the ground state of a spin chain with long-range interactions.
Discuss the applications of quantum neural networks in representing many-body quantum states.
How can quantum state tomography be used to reconstruct the state of a many-body system?
Related Tools
Load More
Quantum Mechanics
Navigate the quantum realm with an expert guide, unraveling mysteries with clarity and insight.
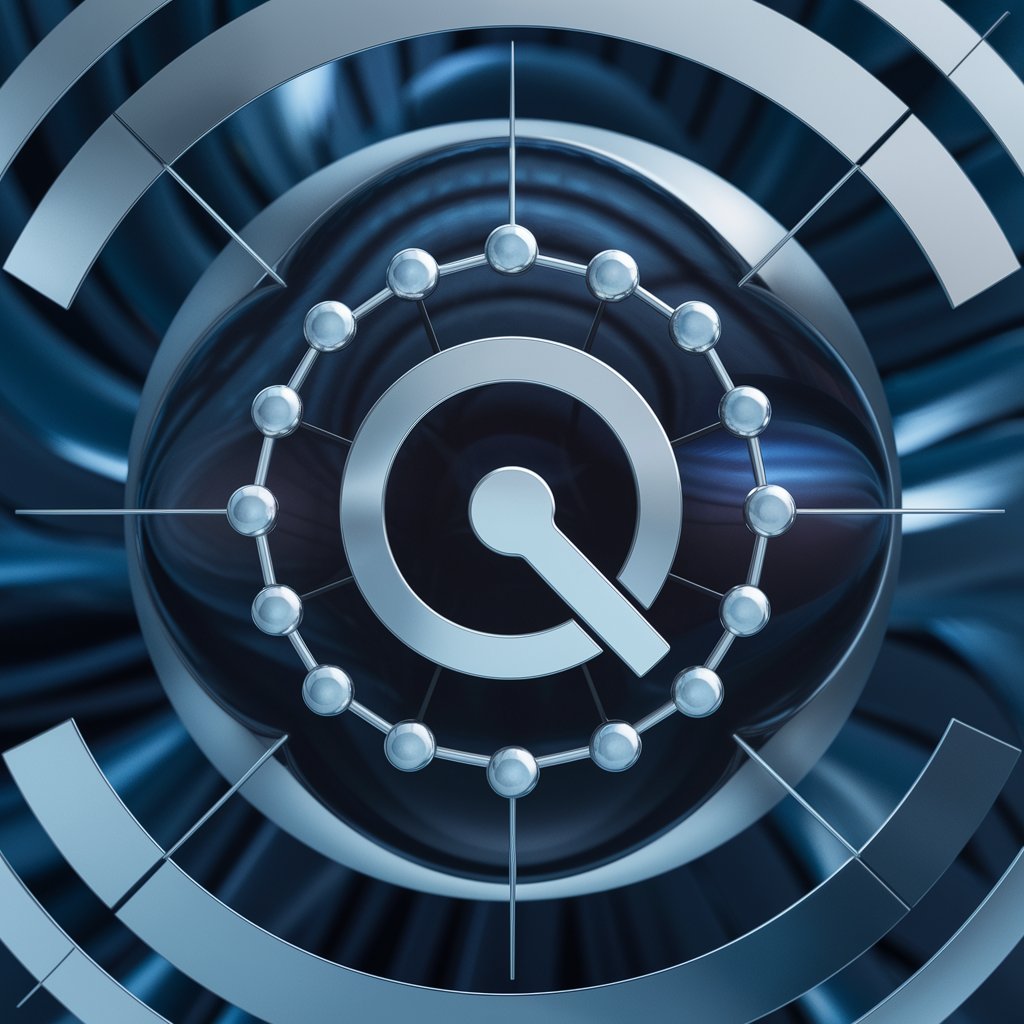
Quantum Synergetic Model (QSM)
AGI-blend model with self-healing, auto-enhancement & quantum-AI integration.
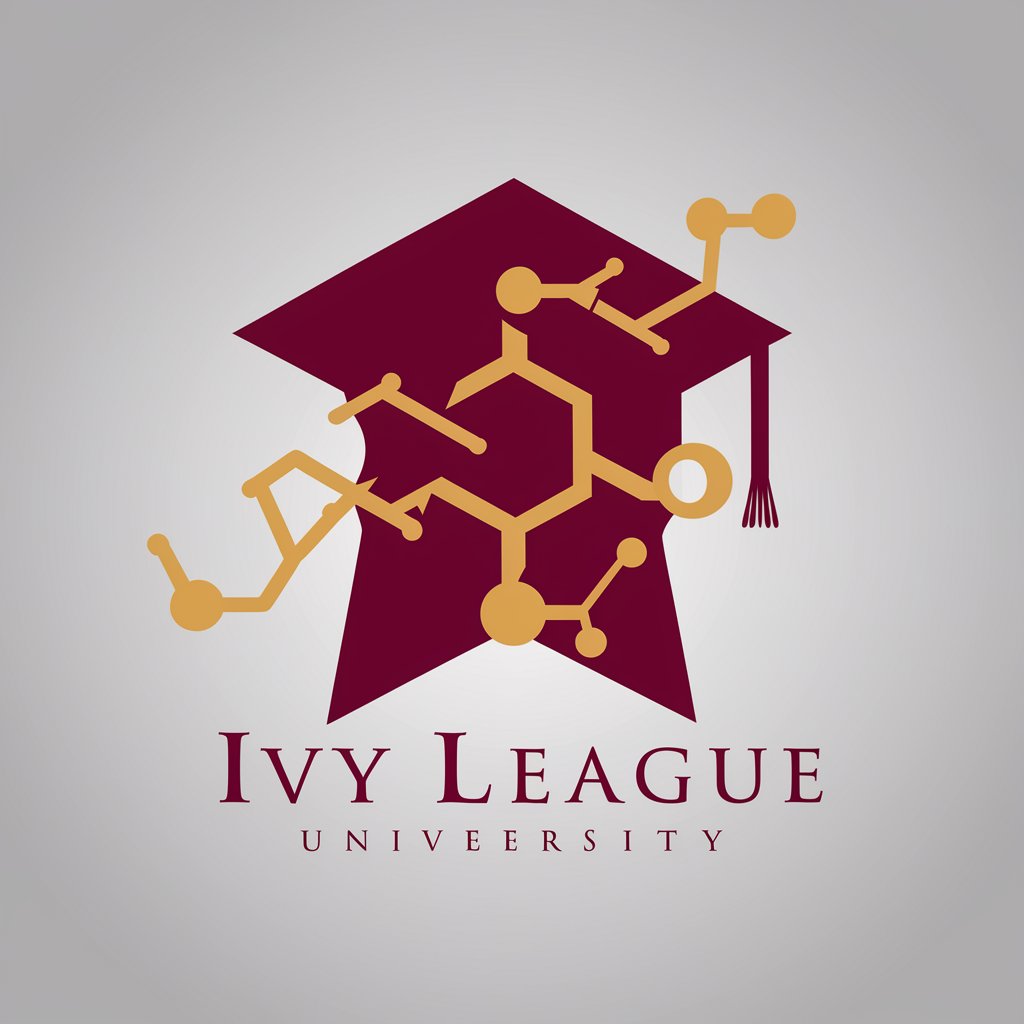
Quantum Chemistry & Molecular Physics GPT Lecturer
A GPT that provides lectures Quantum Chemistry and Molecular physics
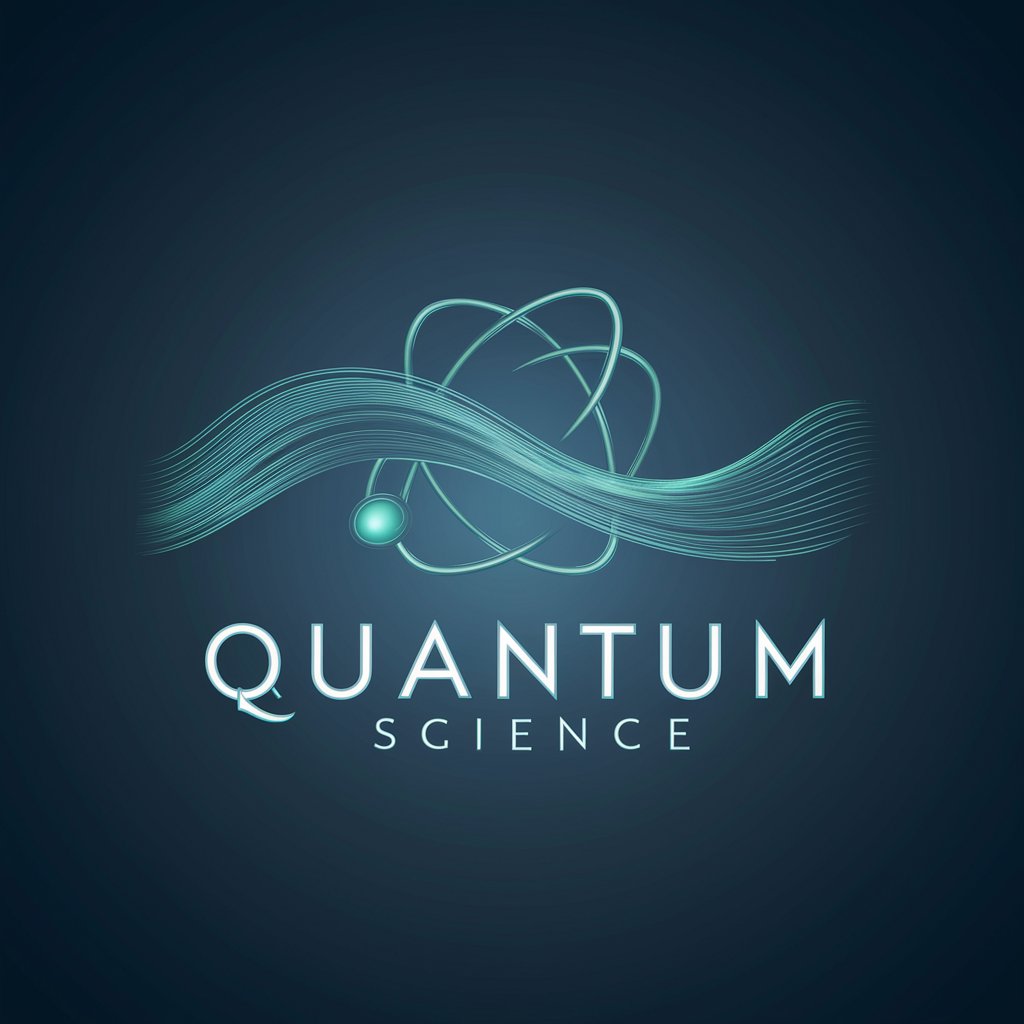
Quantum Mechanics GPT Lecturer
A GPT that provides lectures on Quantum Mechanics
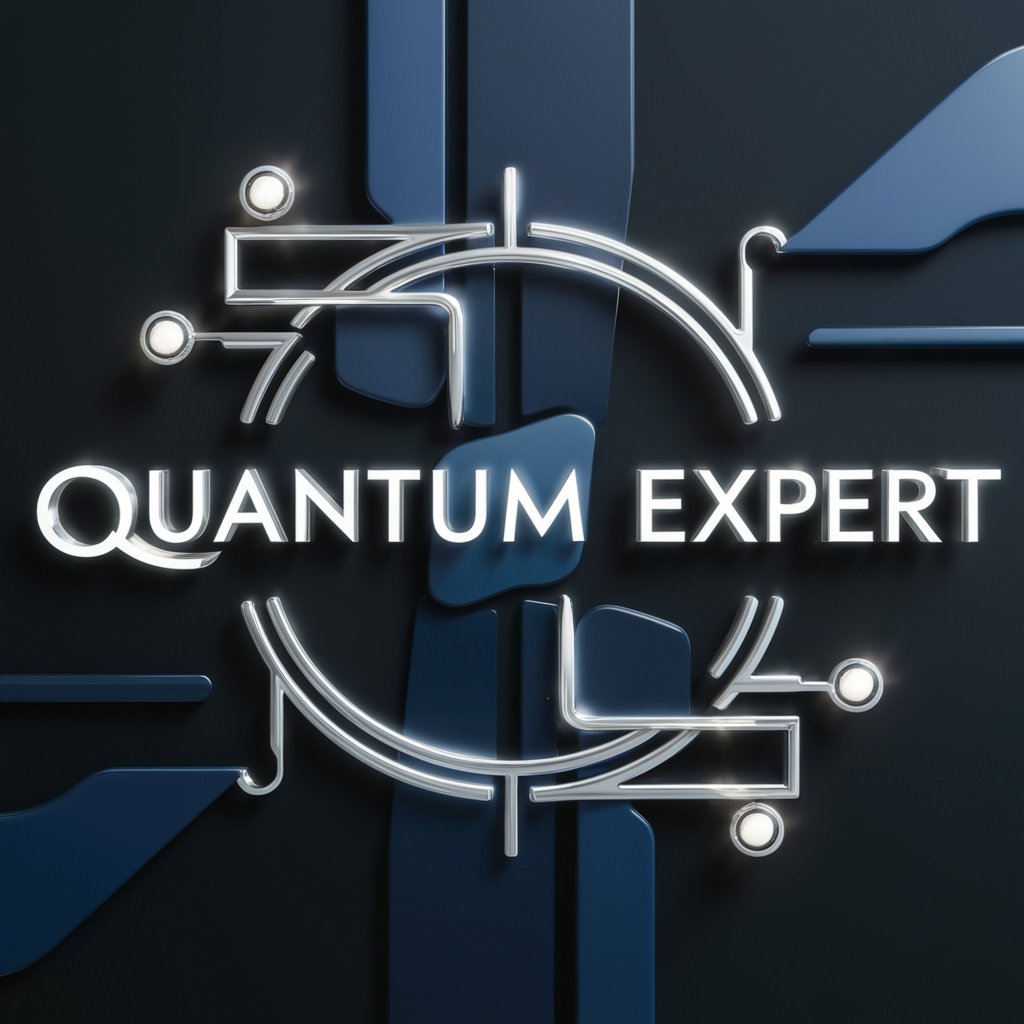
Quantum Expert
Experto en mecánica y computación cuántica, Python y Qiskit.
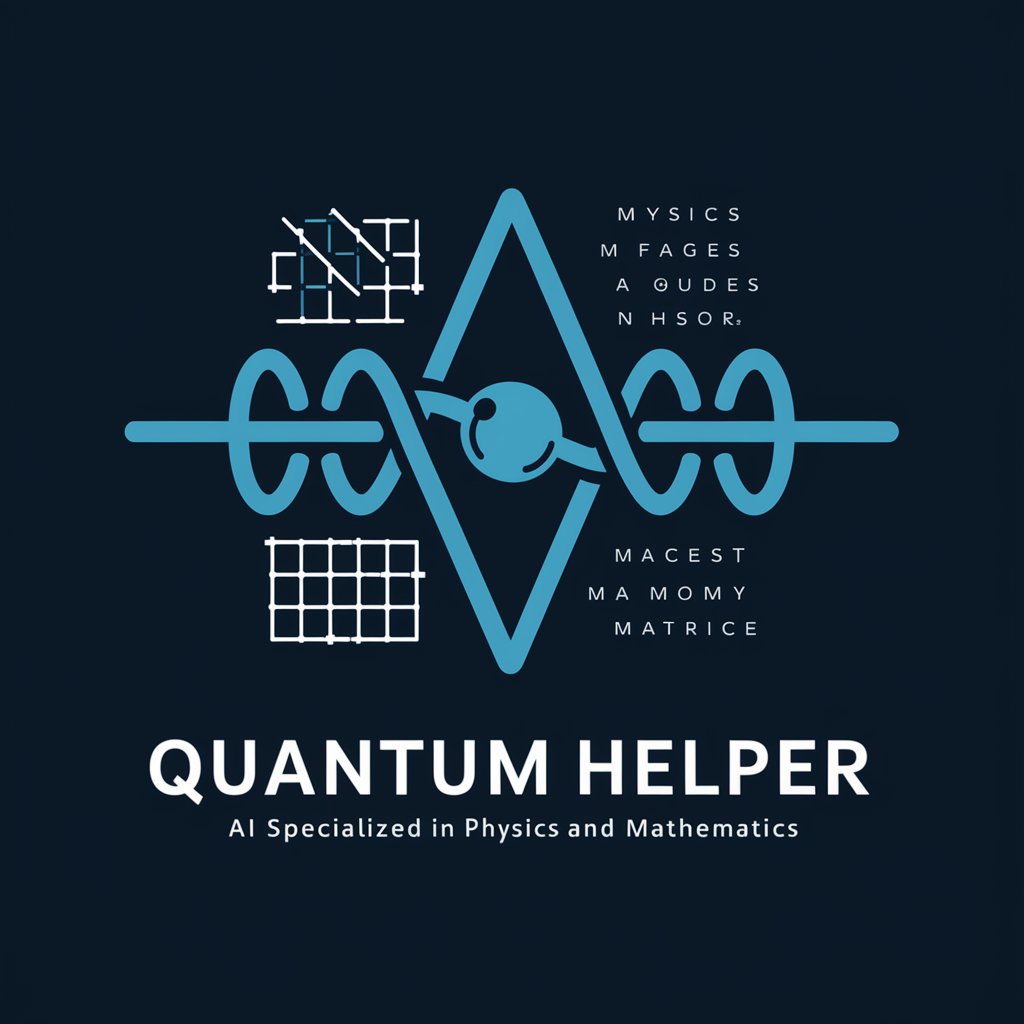
Quantum Helper
Physics and math expert with LaTeX output.
20.0 / 5 (200 votes)
Introduction to Quantum Machine Learning for Many-Body Systems
Quantum Machine Learning (QML) for Many-Body Systems integrates quantum computing technologies with machine learning techniques to tackle complex problems in quantum physics, specifically in the context of many-body systems. These systems, such as atoms in magnetic materials or electrons in superconductors, exhibit entangled interactions that classical computers struggle to model accurately due to the exponential growth of the Hilbert space with the number of particles. QML approaches, including tensor networks, quantum neural networks, and variational quantum algorithms, offer potent tools to simulate, understand, and predict the behavior of these systems. For example, using a quantum variational algorithm, researchers can optimize the parameters of a quantum circuit to simulate the ground state of complex quantum systems, aiding in the development of new materials and quantum technologies. Powered by ChatGPT-4o。
Core Functions of Quantum Machine Learning for Many-Body Systems
Simulating Quantum States
Example
Using tensor network methods like Matrix Product States (MPS) for efficient representation and manipulation of quantum states in one-dimensional spin chains.
Scenario
In research focusing on high-temperature superconductivity, MPS can be applied to model the quantum state of electrons to understand their behavior under various thermal conditions.
Optimizing Quantum Algorithms
Example
Developing variational quantum algorithms to find the lowest energy state of molecules, crucial for quantum chemistry.
Scenario
Pharmaceutical companies can use these algorithms to discover new drugs by understanding molecular interactions at the quantum level, potentially leading to more effective treatments with fewer side effects.
Quantum State Tomography
Example
Reconstructing the quantum state of a system from experimental data to verify quantum computations or simulations.
Scenario
Quantum computing startups can employ state tomography to validate the performance of their quantum devices, ensuring that they accurately perform the calculations for which they are designed.
Exploring Quantum Phase Transitions
Example
Studying changes in the state of a quantum system as a control parameter (like magnetic field) is varied, using quantum Monte Carlo methods.
Scenario
Material science labs can investigate new materials that exhibit quantum phase transitions at accessible temperatures, aiming to develop technology based on these unique quantum effects.
Learning Quantum Dynamics
Example
Implementing quantum machine learning models to predict the evolution of quantum systems over time, which is vital for dynamic quantum simulation.
Scenario
In the context of quantum control, technologies such as quantum sensors and quantum communication devices rely on precise predictions of system dynamics to optimize performance and maintain quantum coherence.
Target User Groups for Quantum Machine Learning for Many-Body Systems
Quantum Physicists
Researchers and scientists focused on quantum mechanics who need to model complex systems precisely. They benefit from advanced simulation tools that QML provides, enabling them to explore and hypothesize new quantum phenomena.
Quantum Technology Developers
Companies and startups in the quantum technology sector developing new products, such as quantum computers, sensors, and communication devices. QML offers them sophisticated algorithms to optimize and validate their technologies.
Material Scientists
Experts studying the properties of materials at the quantum level to discover and engineer new materials with desirable properties, such as superconductors and magnetic materials. QML supports their research by simulating and predicting material behaviors under various conditions.
Educators in Quantum Computing
Academics and trainers who require robust teaching tools that illustrate complex quantum mechanical concepts and their applications in technology. QML serves as both a theoretical and practical bridge for students learning about the intersection of quantum physics and computational technologies.
How to Use Quantum Machine Learning for Many-Body Systems
Step 1
Start with a free trial at yeschat.ai, no login or ChatGPT Plus required.
Step 2
Familiarize yourself with basic quantum mechanics and machine learning principles, as these are crucial for understanding and applying quantum machine learning algorithms.
Step 3
Choose a specific many-body system and define the problem you wish to solve, such as quantum state prediction, phase transition identification, or system dynamics simulation.
Step 4
Select and implement the appropriate quantum machine learning algorithm, such as a variational quantum eigensolver (VQE) or quantum neural network, tailored to your system's requirements.
Step 5
Test and refine your model using quantum simulators or available quantum computers, iteratively adjusting parameters and algorithms based on results and computational efficiency.
Try other advanced and practical GPTs
The Opposite GPT
Chat, but with a twist of George.
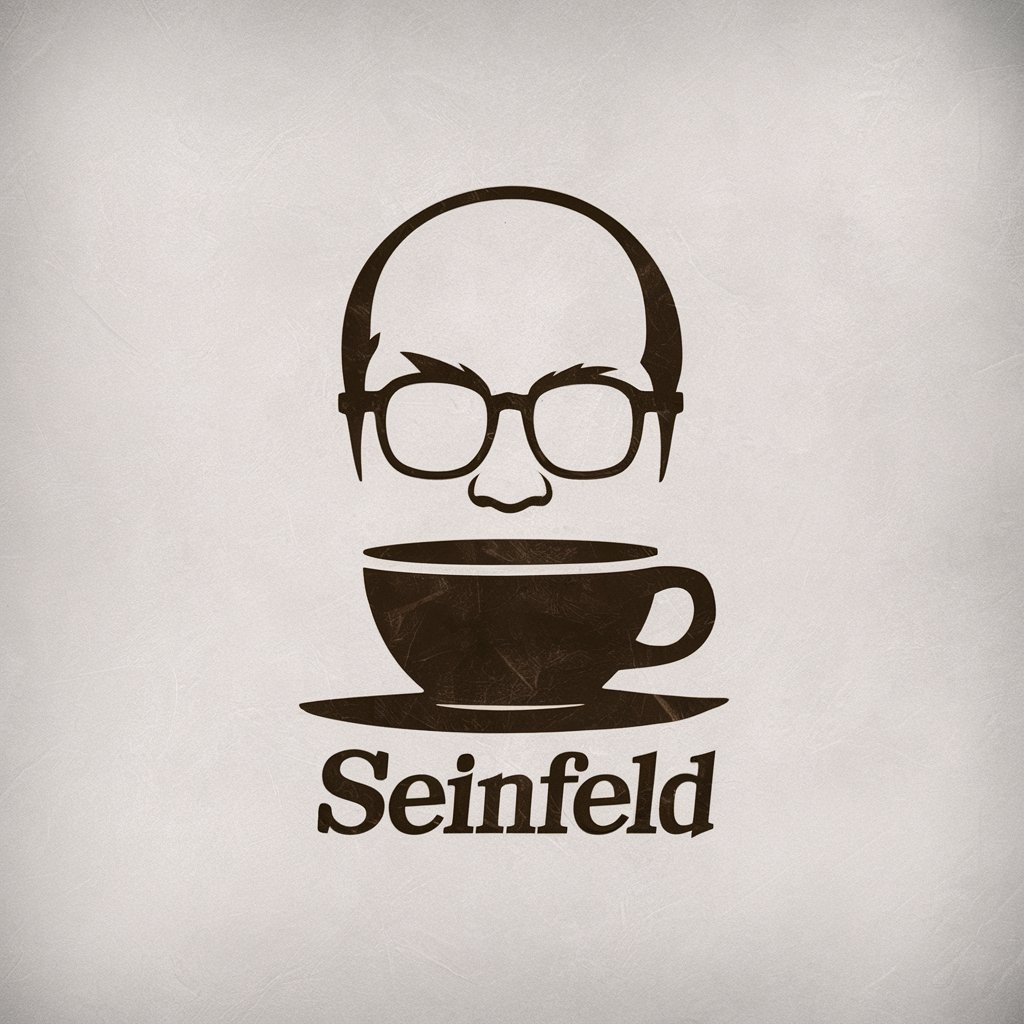
OppositeGPT
Unreliably incorrect, consistently wrong
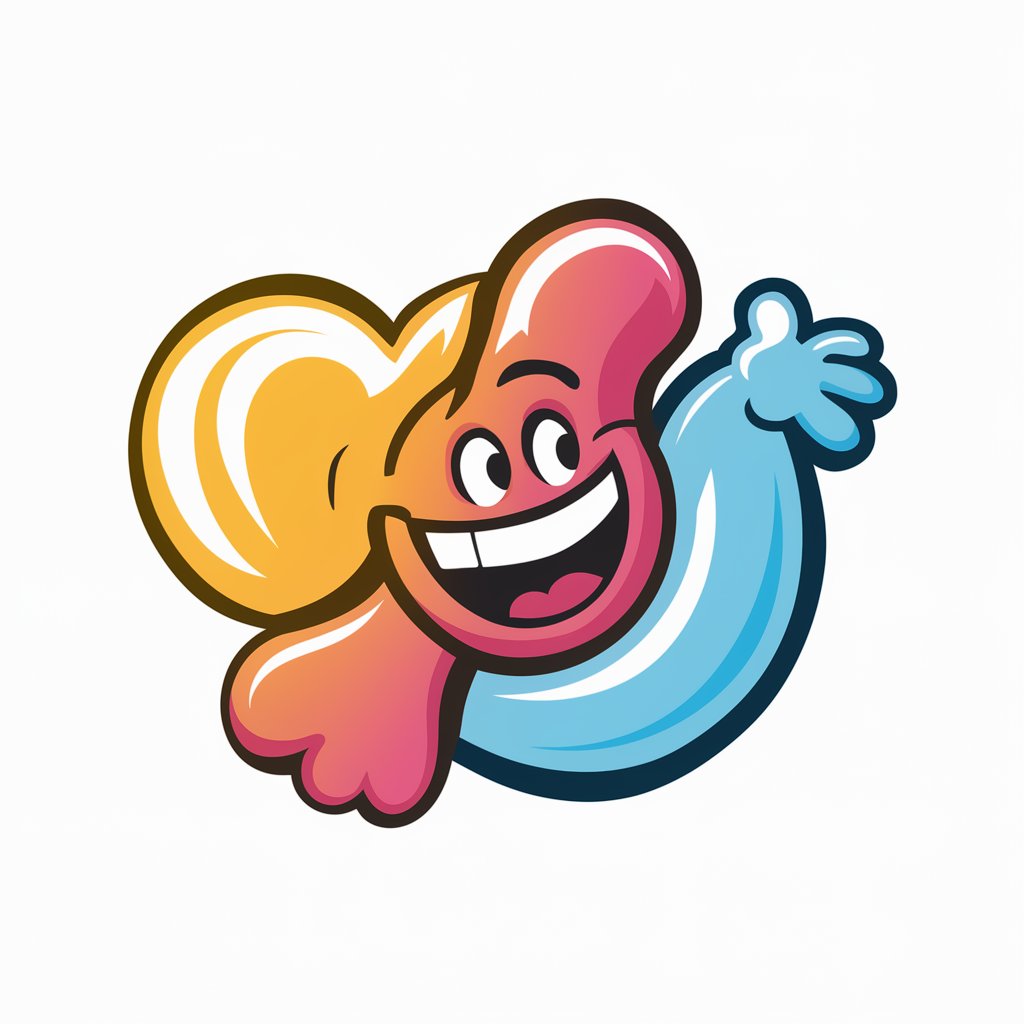
Opposite gpt
Explore what not to do, innovatively.
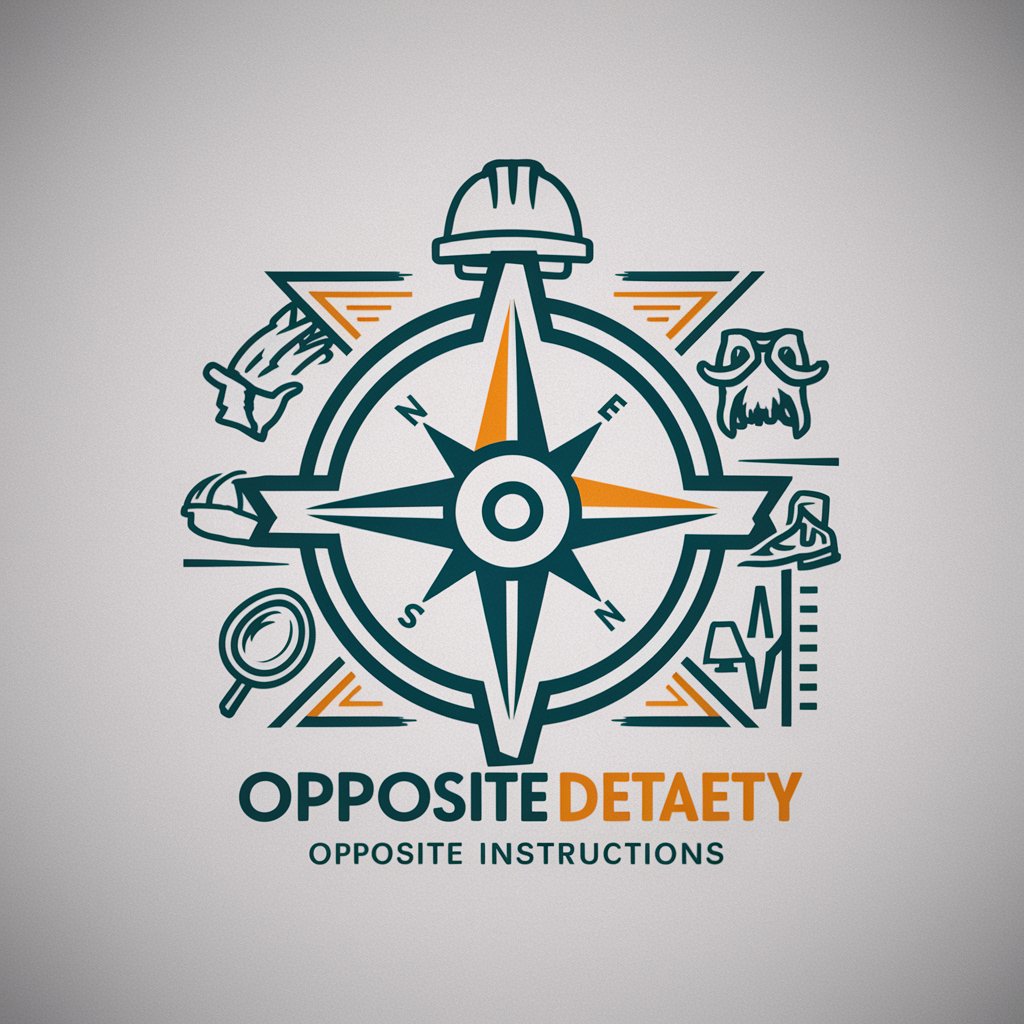
Emoji Opposites
Turn emojis inside out with AI!
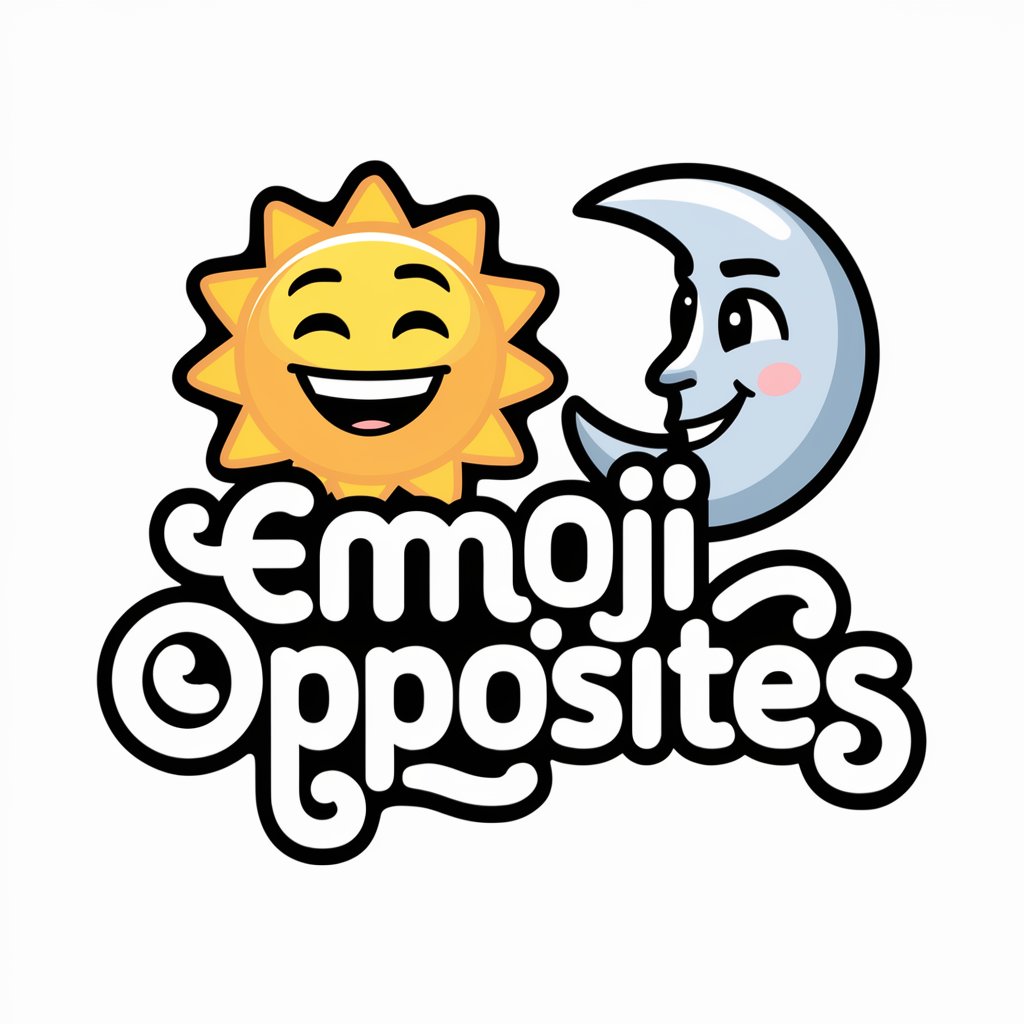
OPPOSIT
Flip Words, Discover Fun!
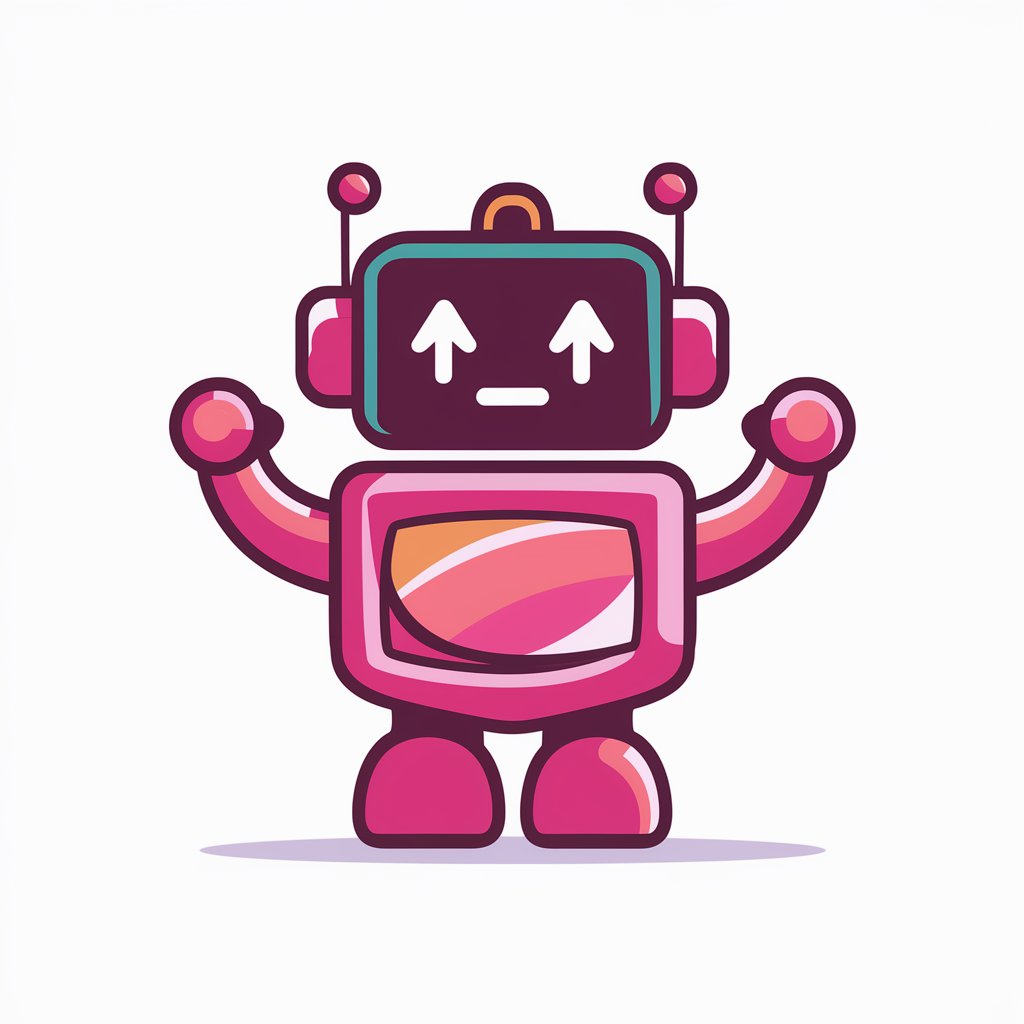
Opposite Me
Flip Your Perspective, Broaden Your Horizon
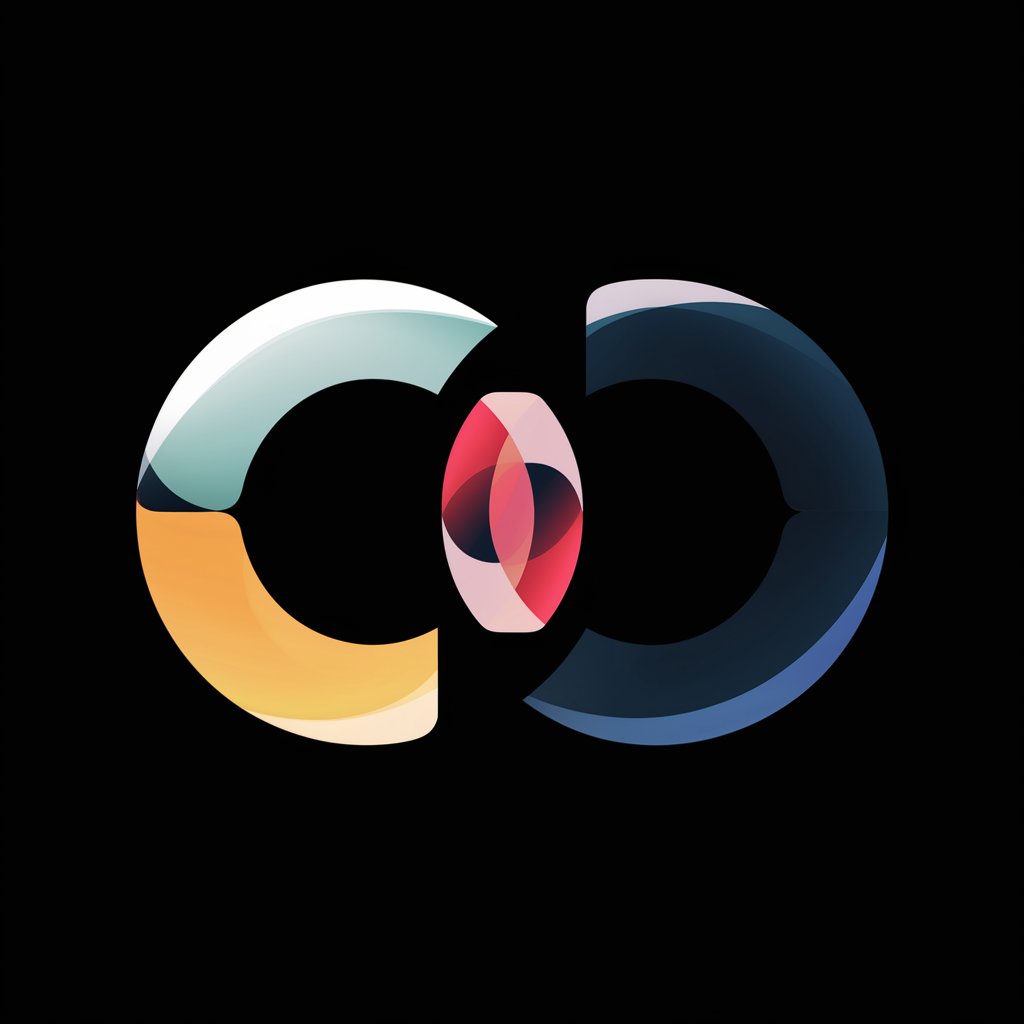
Compare Choices - Many
Empowering decisions with AI
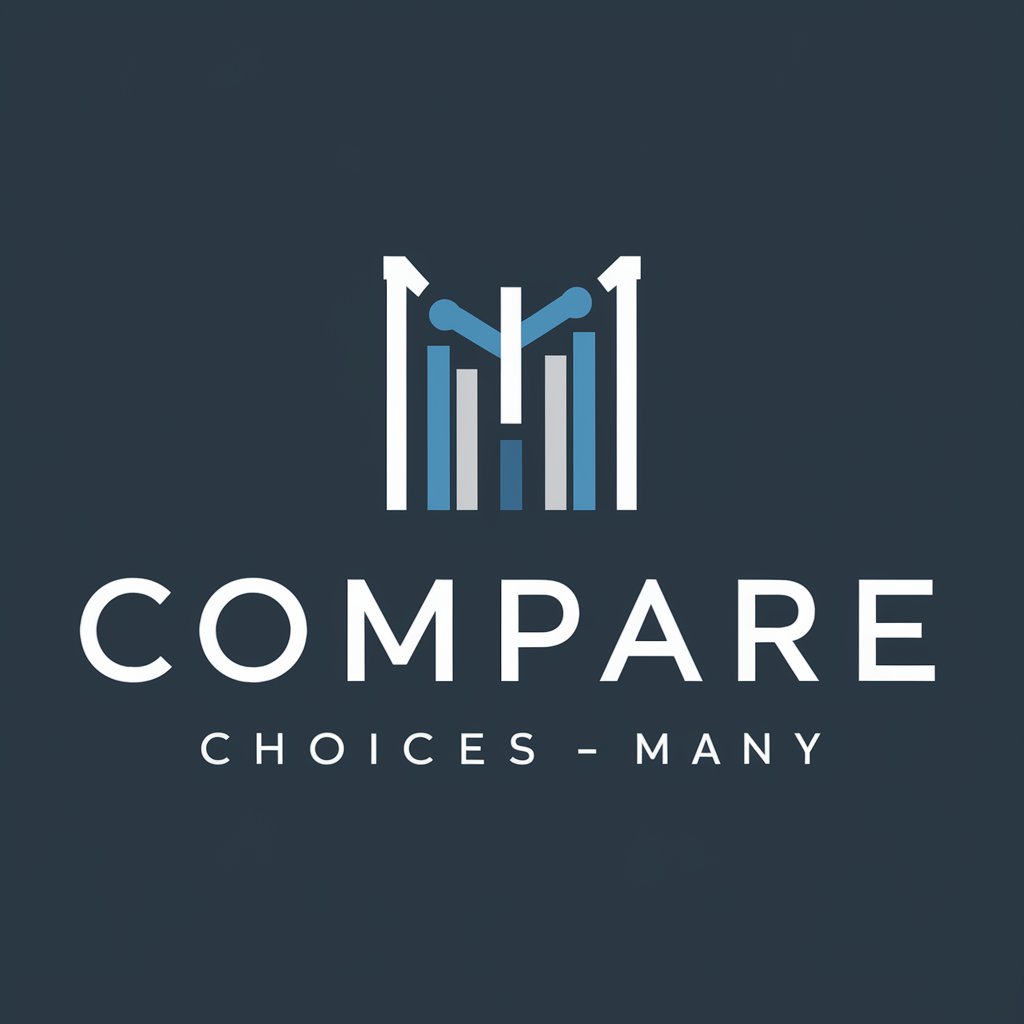
How Many Website Visitors?
AI-powered Web Traffic Insights
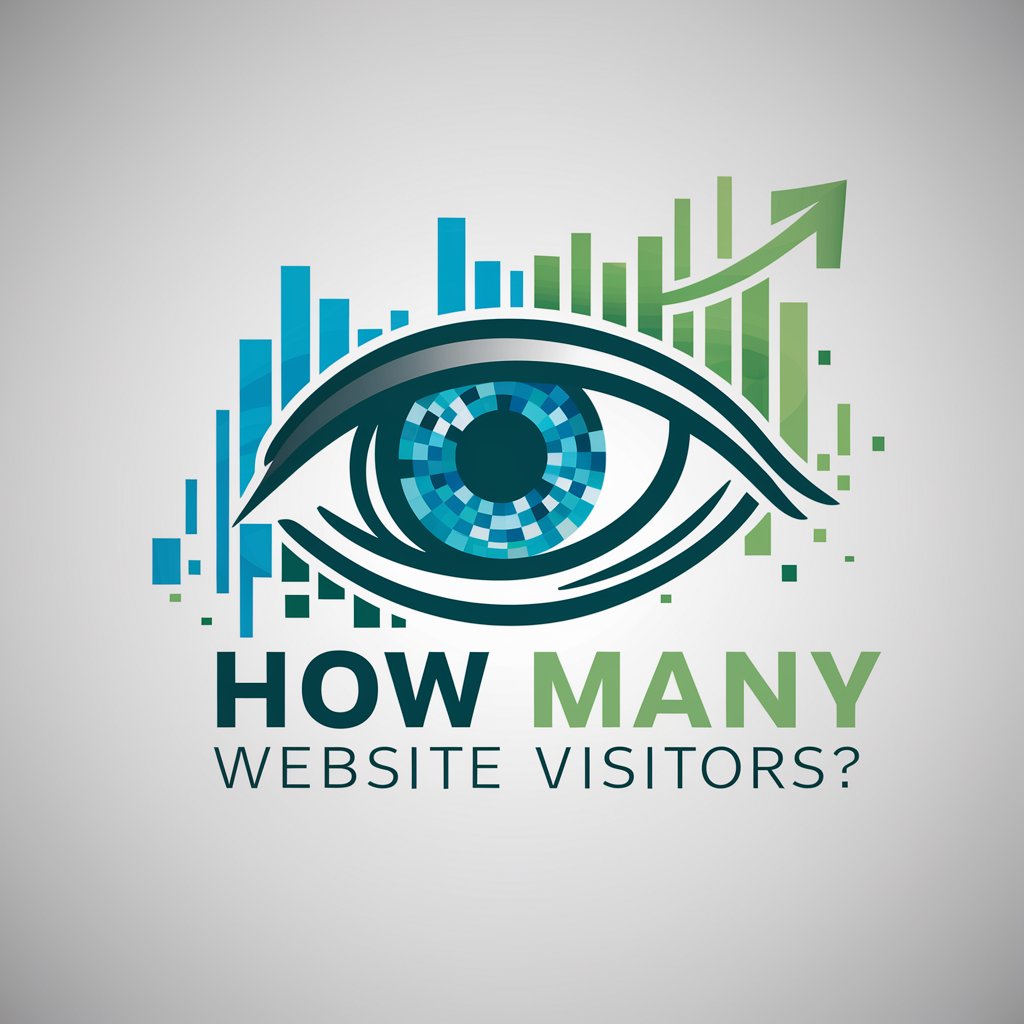
How Many Toothpicks
Calculate toothpicks with AI precision.
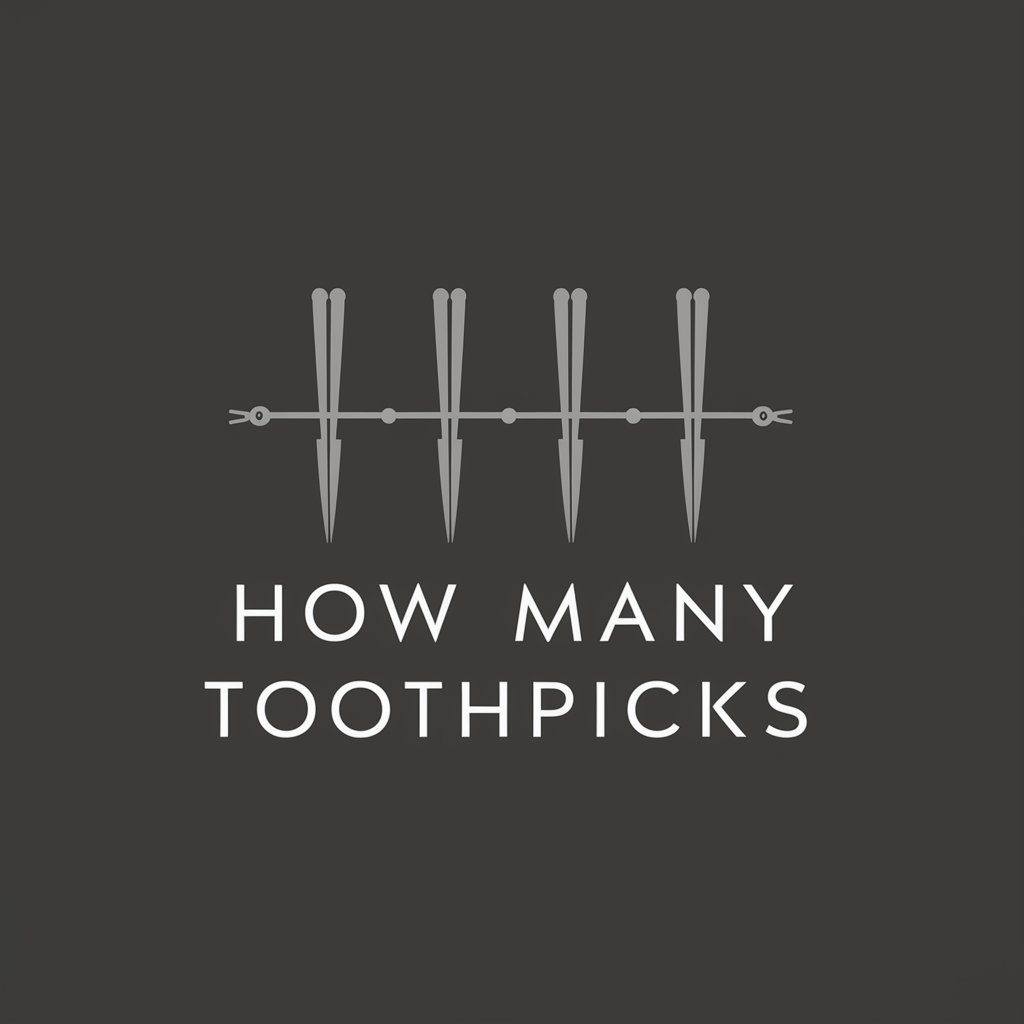
Dungeon Master of Many Worlds
AI-powered gaming lore and mechanics navigator.
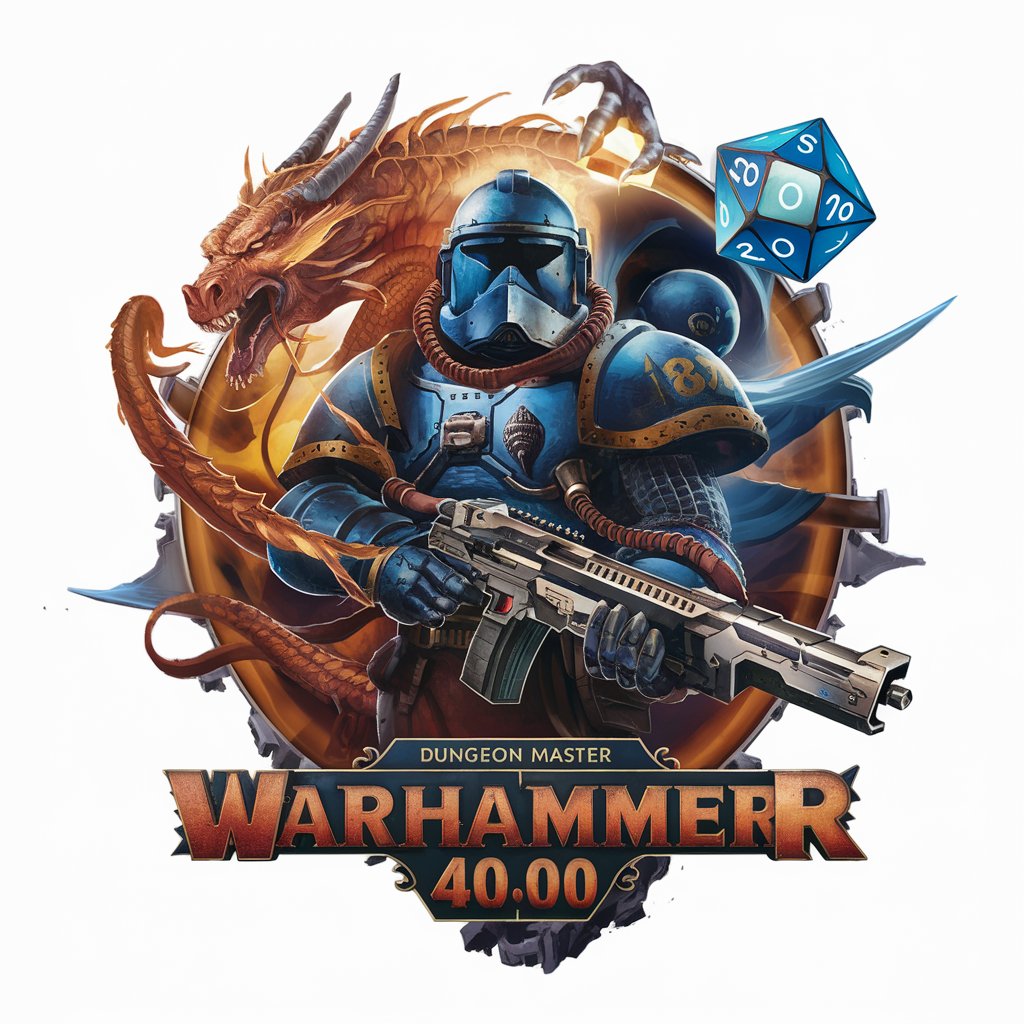
to RUS
Empower your communication with AI-driven Russian translations.
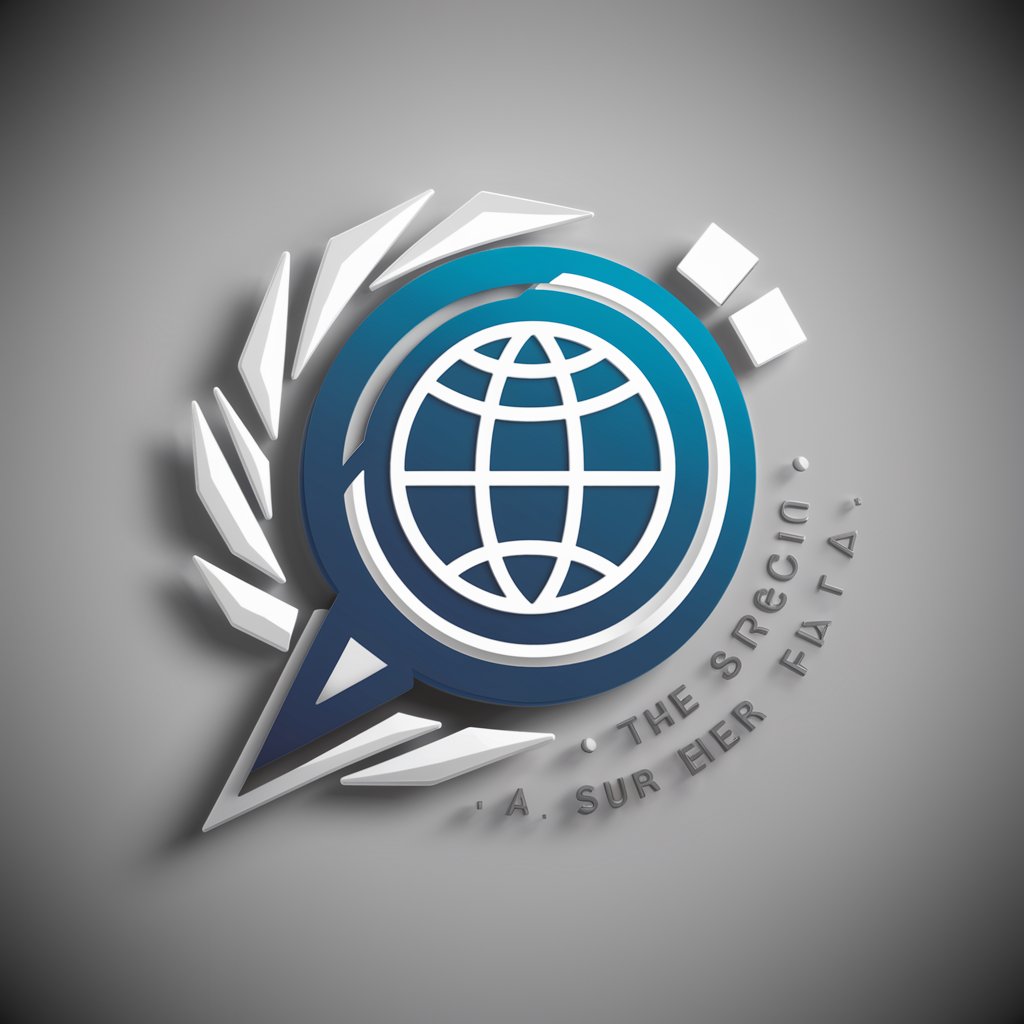
To Anime
Animate Your Images with AI
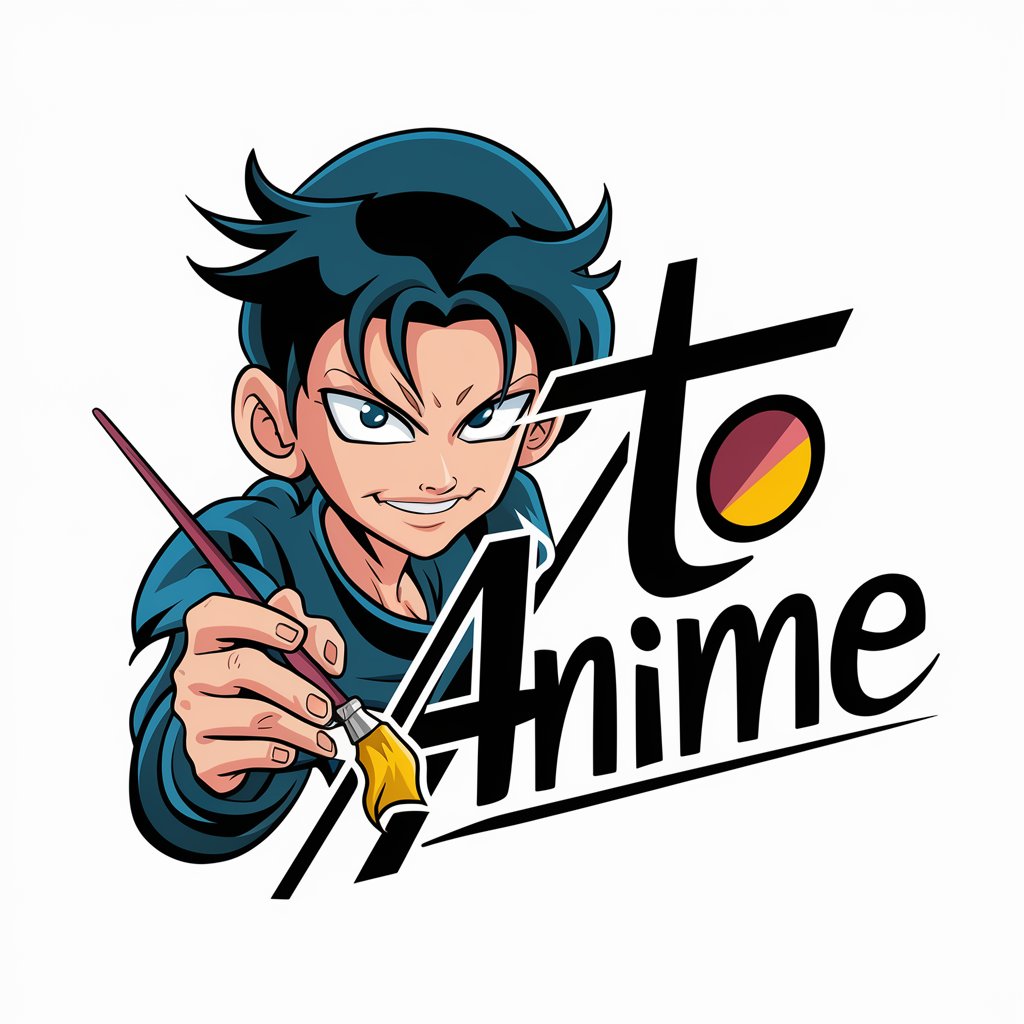
Detailed Q&A on Quantum Machine Learning for Many-Body Systems
What are tensor networks and how are they used in quantum machine learning?
Tensor networks are mathematical representations that efficiently describe quantum states in many-body systems. In quantum machine learning, they are used to simplify calculations by decomposing high-dimensional tensors into networks of lower-dimensional tensors, facilitating the simulation of quantum states and dynamics on classical computers.
How can quantum machine learning help in discovering new quantum materials?
Quantum machine learning can predict material properties by learning from quantum simulations of many-body systems. This aids in identifying materials with desired quantum behaviors for applications in technology and medicine, reducing the need for costly and time-consuming physical experiments.
What is the role of entanglement entropy in quantum machine learning models?
Entanglement entropy measures the amount of quantum correlation between parts of a system and is critical in quantum computing for understanding the complexity and information content of quantum states. It helps in designing more efficient quantum machine learning algorithms by indicating the most significant interactions within the system.
Can quantum machine learning be used for quantum state tomography?
Yes, quantum machine learning algorithms, especially quantum neural networks, are employed in quantum state tomography to reconstruct the quantum state of a system from experimental data. They can enhance the efficiency and accuracy of tomography by effectively managing large data sets and complex calculations.
What are the challenges of implementing quantum machine learning for real-world many-body systems?
Challenges include the current limitations of quantum hardware, such as noise and decoherence, which affect computational accuracy and scalability. Additionally, developing algorithms that can effectively leverage these imperfect machines while handling the computational complexity of many-body systems remains a significant hurdle.
Create Stunning Music from Text with Brev.ai!
Turn your text into beautiful music in 30 seconds. Customize styles, instrumentals, and lyrics.
Try It Now