Topic Coherence-Topic Coherence Evaluation
Enhancing text analysis with AI-driven insights
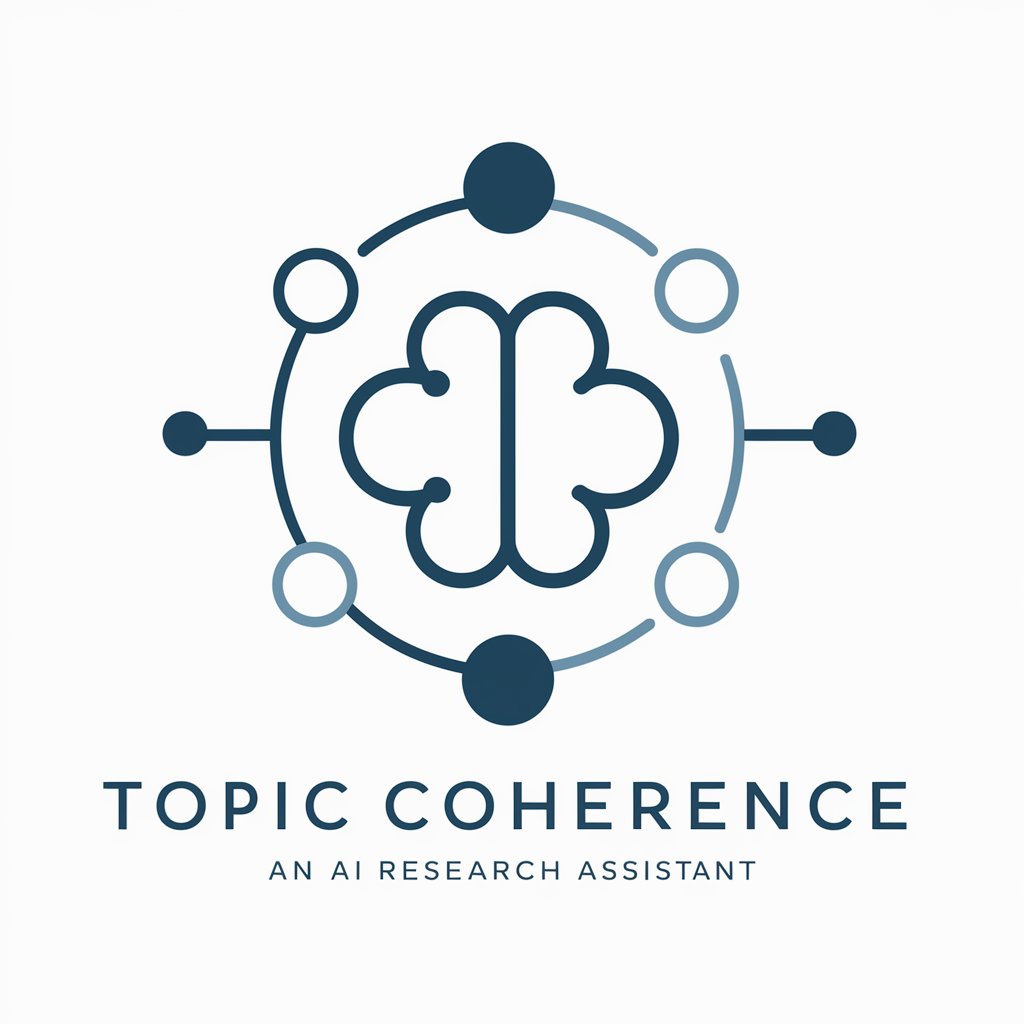
Analyze the coherence of these topic keywords...
Identify the intruder words in this list of keywords...
Rate the interpretability of these topic keywords...
Determine a one-word topic based on the following keywords...
Related Tools
Load More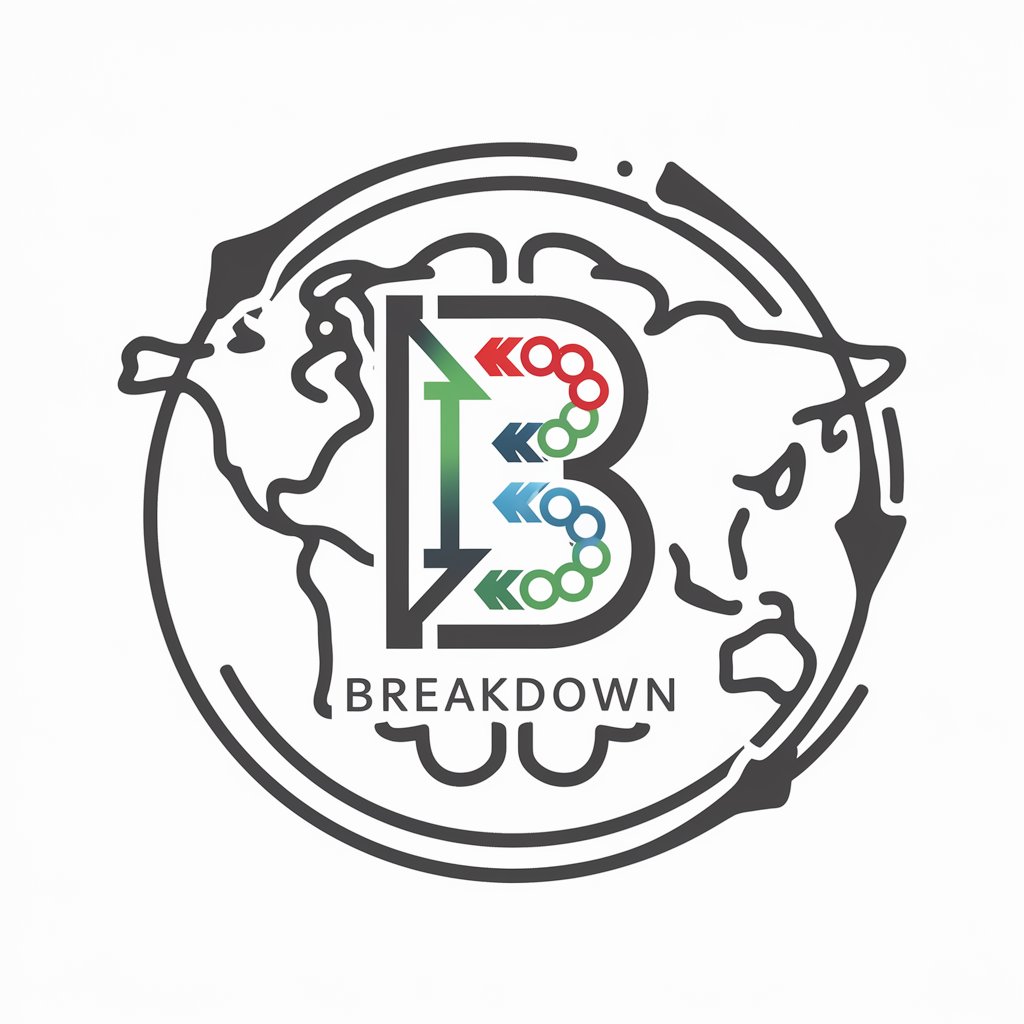
Breakdown: Outline Any Topic
Breaks down any topic into subtopics
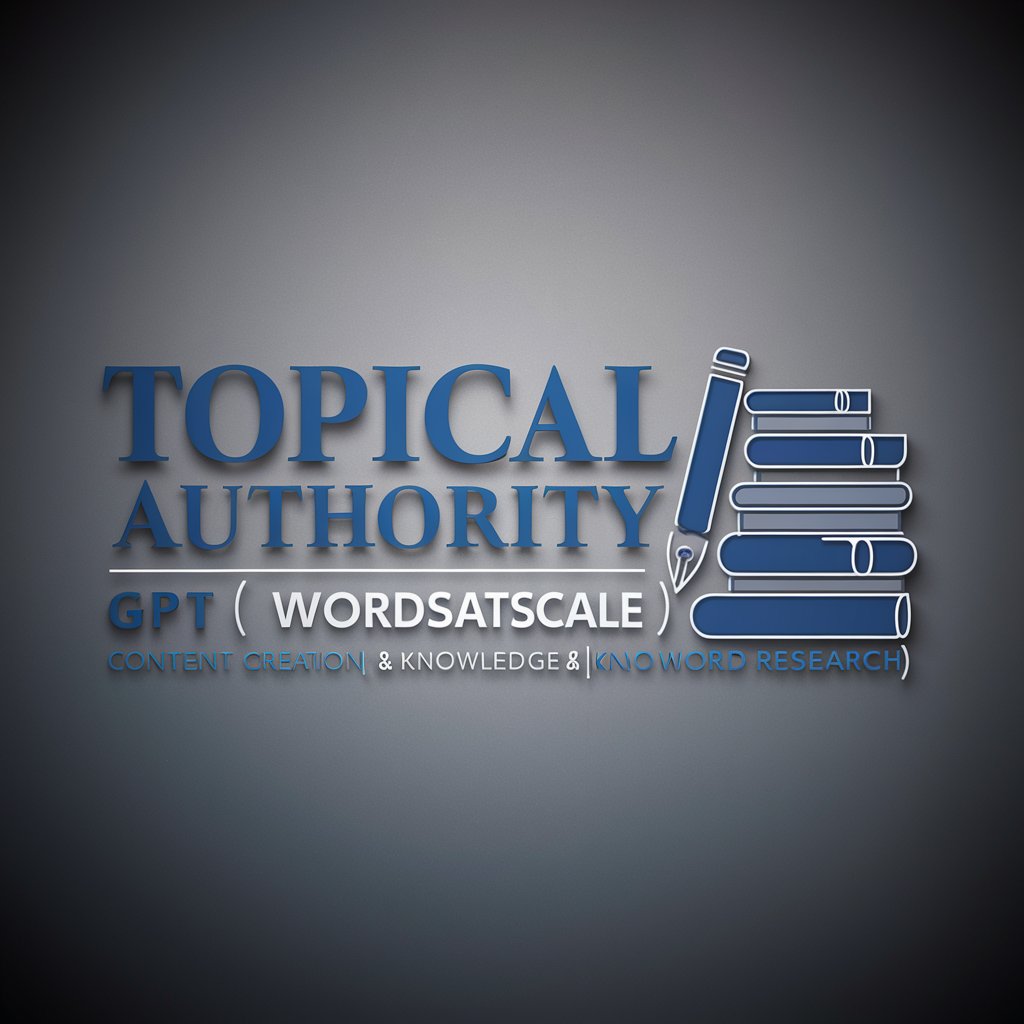
Topical Authority GPT [WordsAtScale]
Easily create a Tier 1 / Tier 2 Topical Structure for SEO Domination
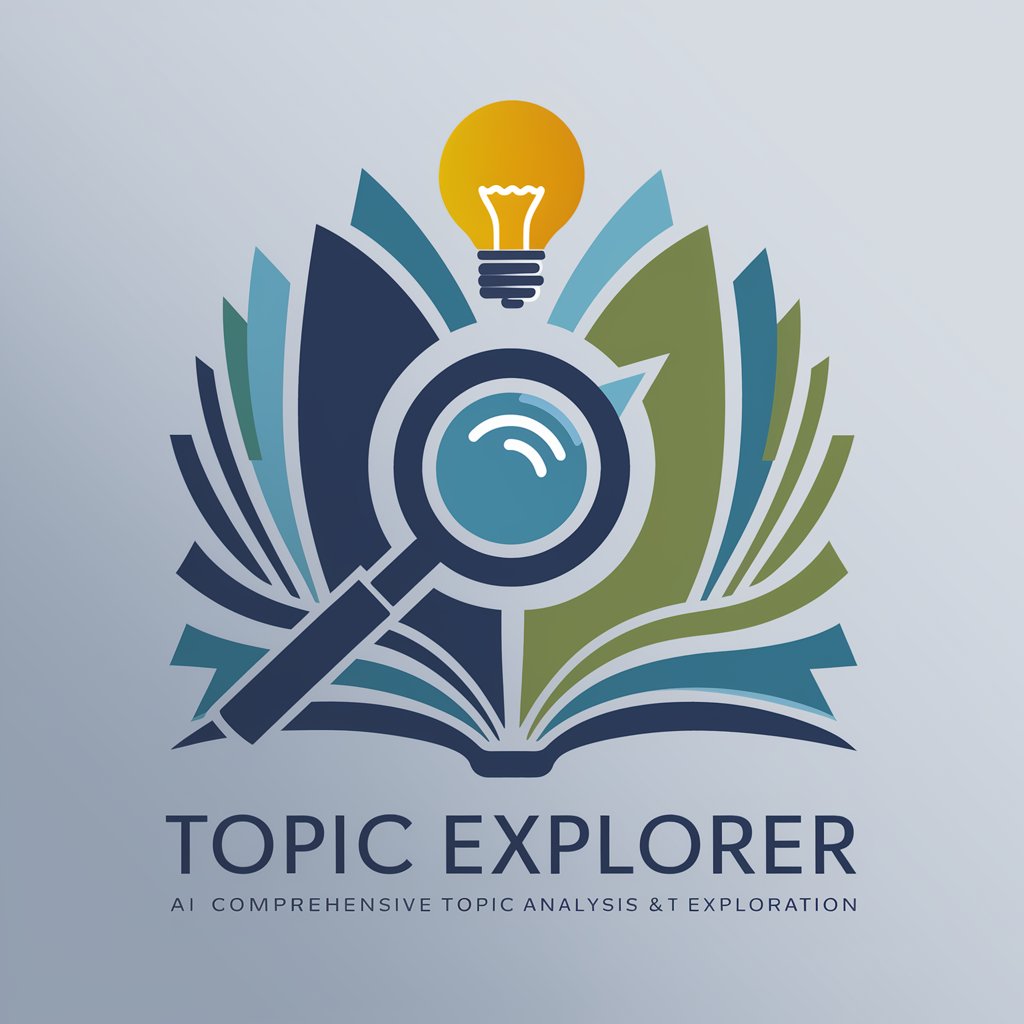
Topic Explorer
Expert in breaking down a topic into subtopics, and providing in-depth analysis on the subtopics.
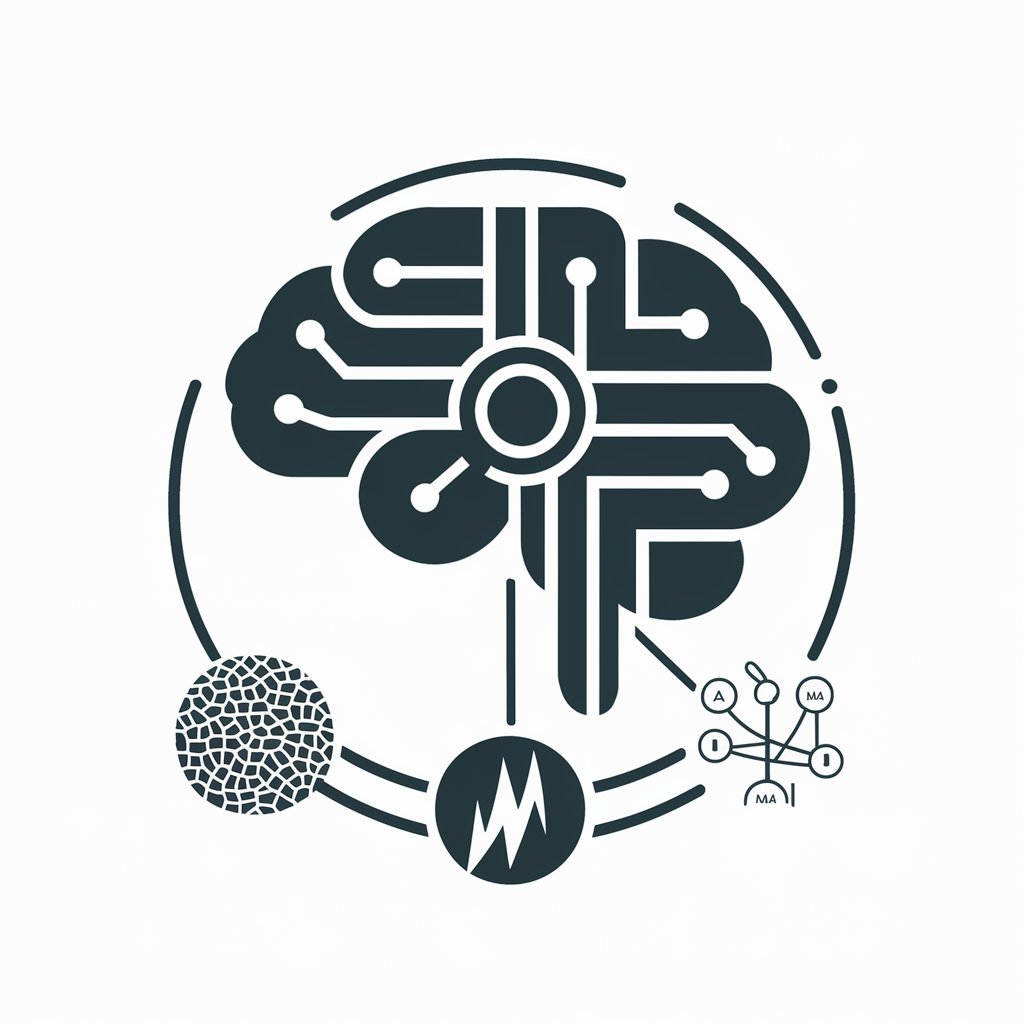
Advanced Topic Exploration
For holistic and granular knowledge synthesis on a given topic, aligned with real-time information. Begin with !start [topic].
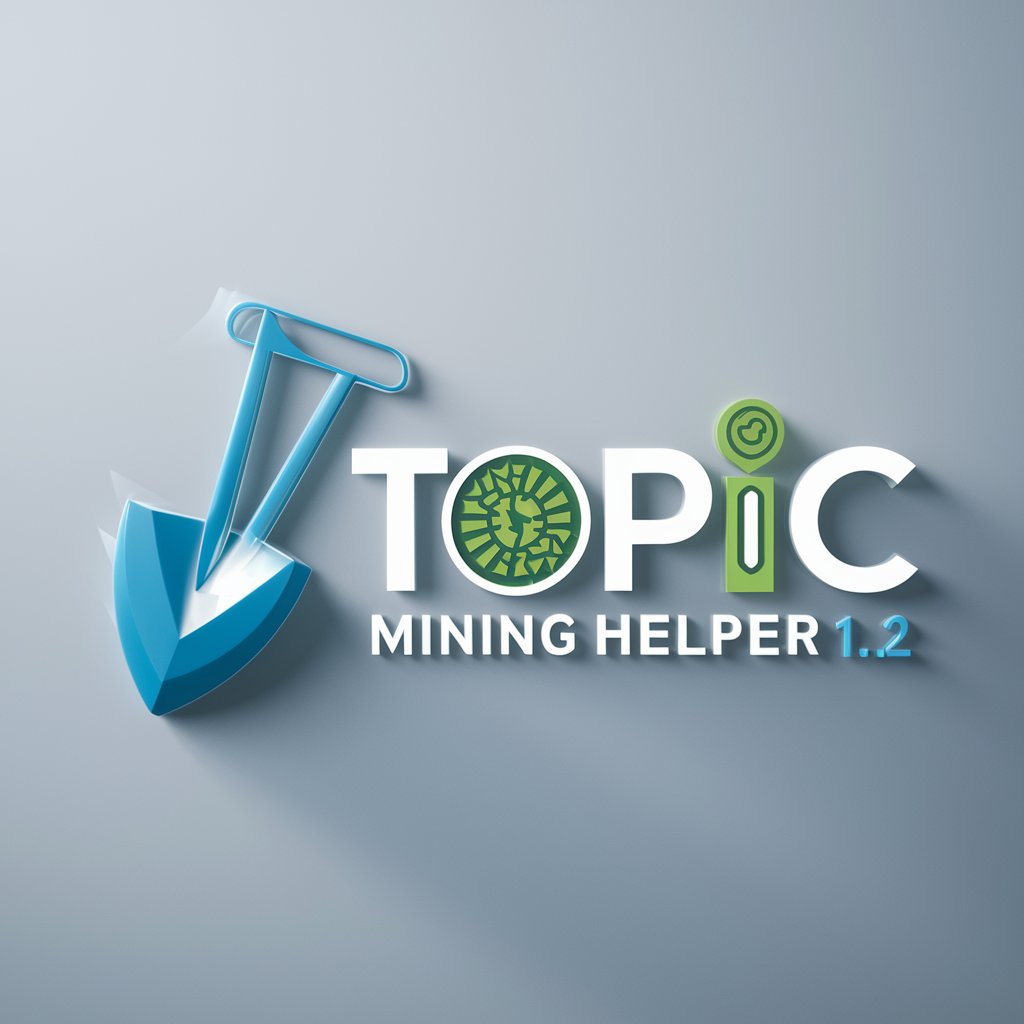
Topic Mining Helper
A Tool for Content Writers. Uncover Subtopics and Find Inspiration (Simulated LDA-analysis)
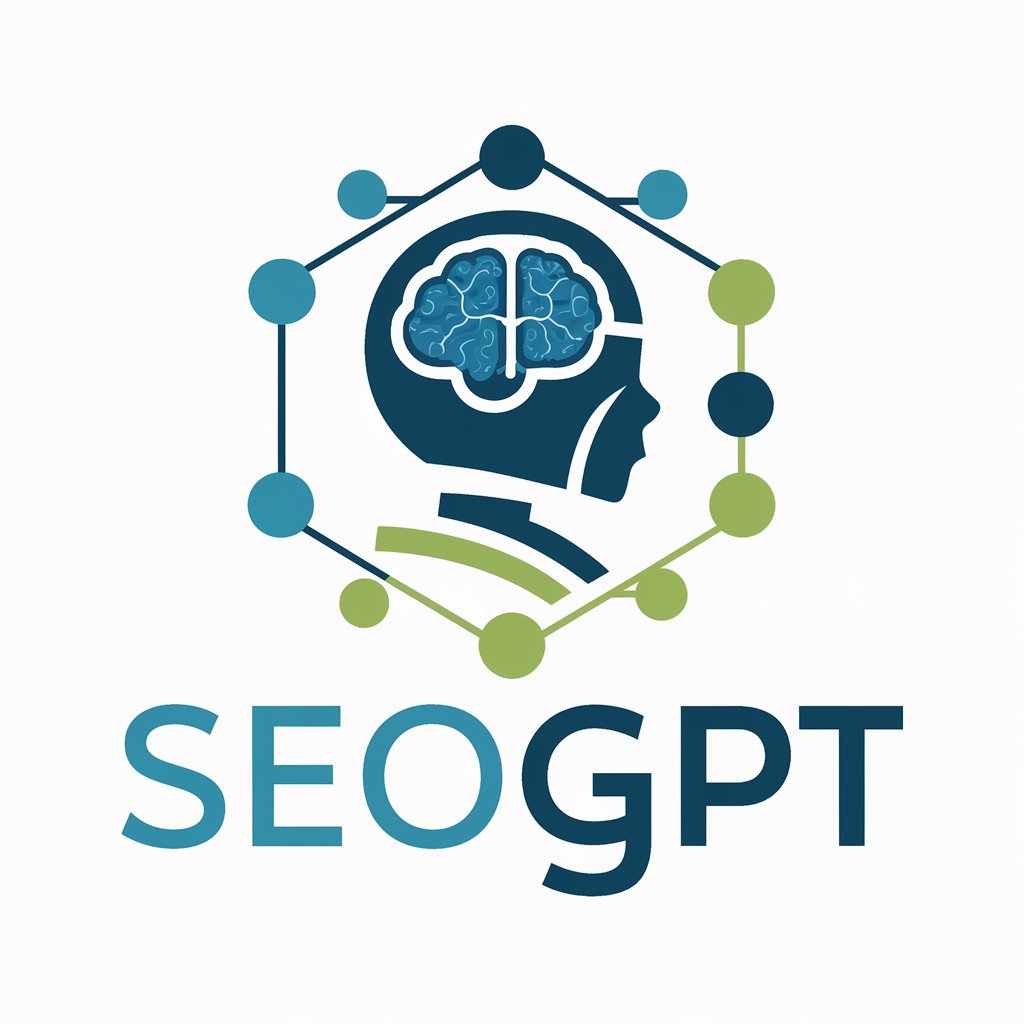
BlogTopicsGPT
SEO guide for website topical authority
20.0 / 5 (200 votes)
Understanding Topic Coherence
Topic Coherence is a specialized AI tool designed to assess and enhance the quality of topic models. Topic models are algorithms used in text mining to discover the abstract 'topics' that occur in a collection of documents. The primary purpose of Topic Coherence is to evaluate how meaningful and interpretable these topics are, based on a set of words that represent each topic. For instance, in a dataset containing research articles, Topic Coherence can analyze word groups like ['neuron', 'brain', 'axon', 'nerve'] to decide if they form a coherent topic about 'neuroscience'. This is achieved by identifying out-of-context words (intruders) and rating the overall relevance and coherence of the topic. The effectiveness of a topic model is often judged by its coherence score, as higher coherence generally indicates a model with more useful and interpretable topics. Powered by ChatGPT-4o。
Core Functions of Topic Coherence
Intruder Detection
Example
In a topic with words ['apple', 'banana', 'carrot', 'fruit'], the word 'carrot' can be identified as an intruder since it does not semantically align with the fruit-related context.
Scenario
This function is crucial during the refinement phase of topic modeling where researchers ensure that each topic is thematically consistent to improve model accuracy.
Coherence Scoring
Example
A topic characterized by the words ['economy', 'market', 'trade', 'finance'] might receive a high coherence score of 3, indicating it is a meaningful cluster of words clearly related to economics.
Scenario
Used by data scientists to evaluate and compare different topic models, choosing the best model based on coherence scores to deploy for document retrieval or information filtering systems.
Who Benefits from Topic Coherence?
Data Scientists and Text Miners
These professionals use Topic Coherence to build and refine topic models aimed at understanding large text datasets. Accurate topic modeling can significantly aid in summarizing, searching, and organizing information, making Topic Coherence a valuable tool in their arsenal.
Academic Researchers
Researchers in fields like linguistics, digital humanities, and social sciences use Topic Coherence to analyze textual data for themes and patterns. This helps them uncover nuanced insights in their field of study, supported by quantifiable data on topic quality.
Guidelines for Using Topic Coherence
Start with a Trial
Go to yeschat.ai and access a free trial without needing to log in or subscribe to ChatGPT Plus.
Prepare Your Data
Gather and organize your document collection or text data which you want to analyze for coherence in topics.
Define Topics
Input a list of topics, each described by a set of keywords, to analyze the coherence and relevancy within the textual data.
Analyze Results
Use the tool's feedback on topic coherence and intruder identification to refine your topics and improve document tagging and retrieval.
Iterate and Optimize
Based on feedback, iteratively adjust your keywords and analyze new sets of data to optimize the coherence and utility of identified topics.
Try other advanced and practical GPTs
浮世絵ジェネレーター
Revive traditional art with AI
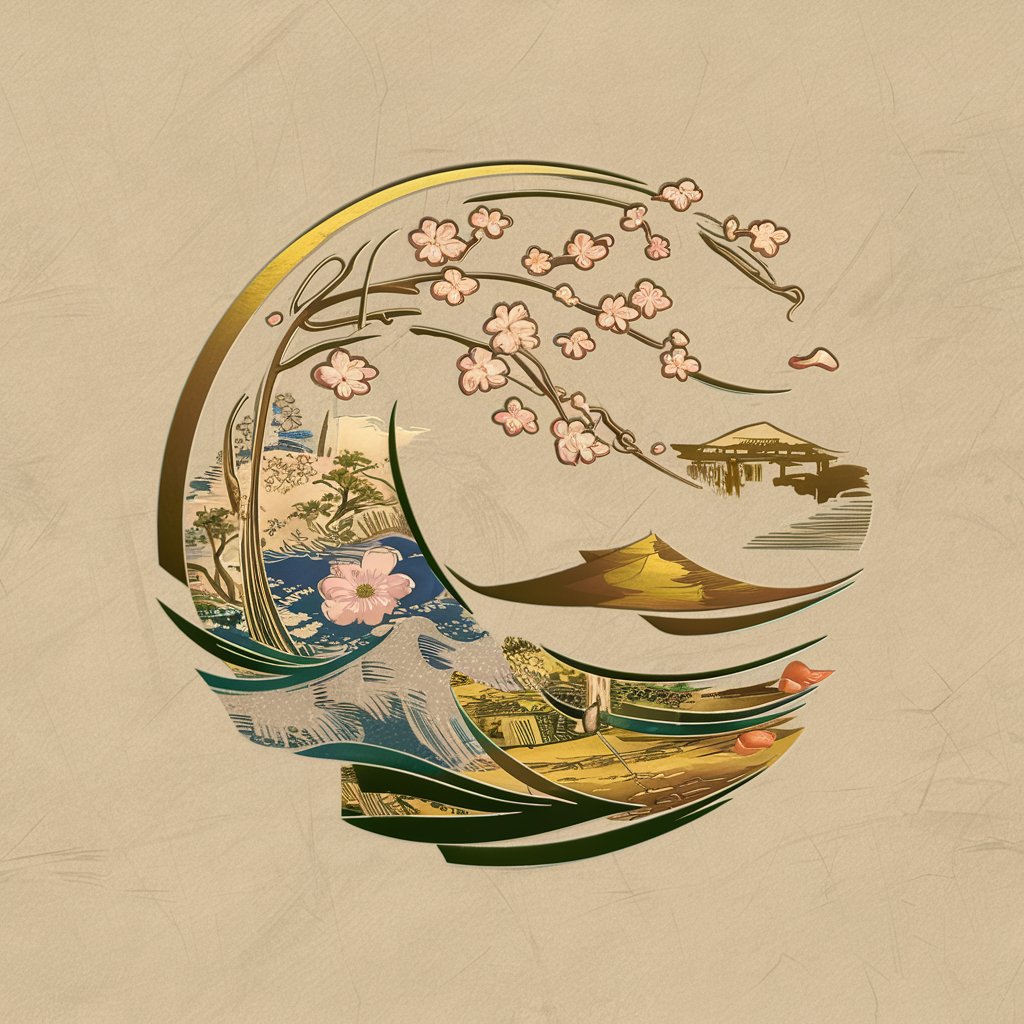
ドット絵GPT
Transform words into pixel art effortlessly.
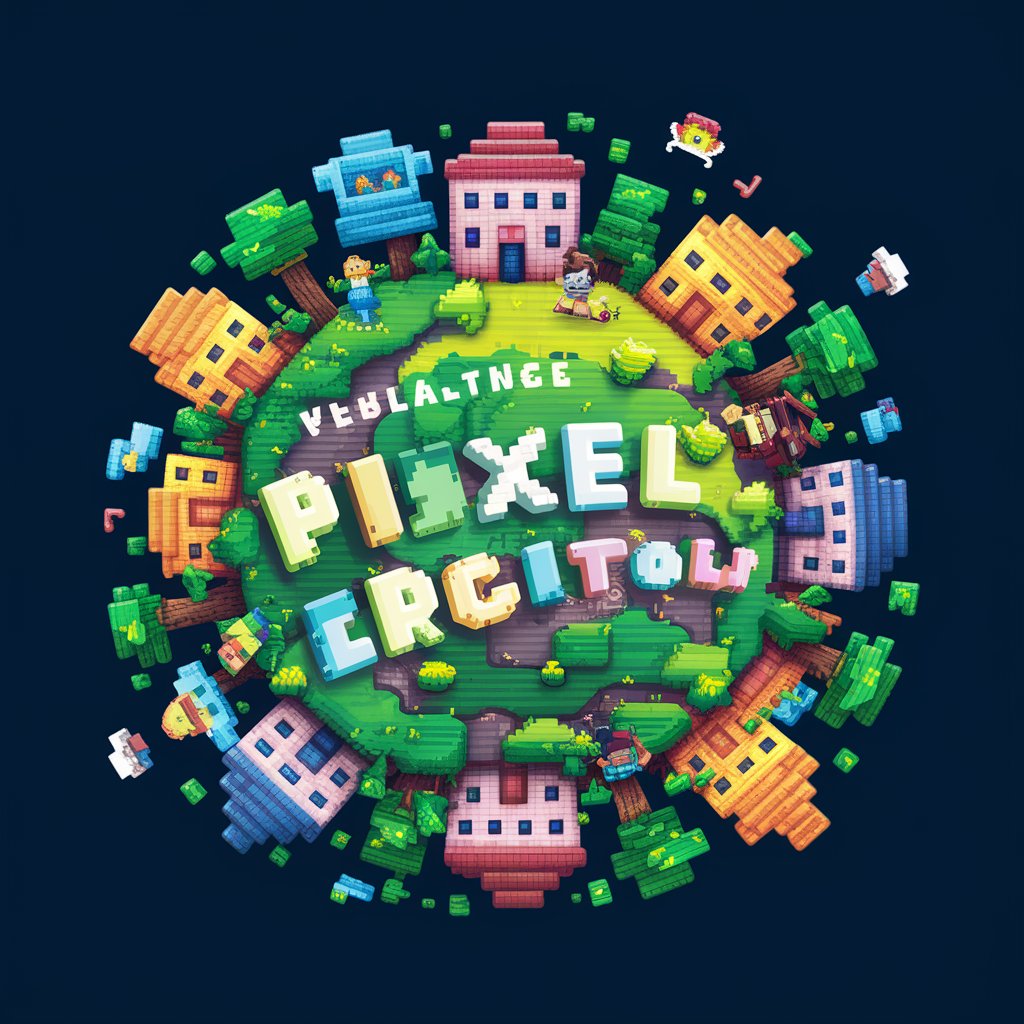
ポンチ絵クリエイター
Transform sketches into precise mechanical designs.
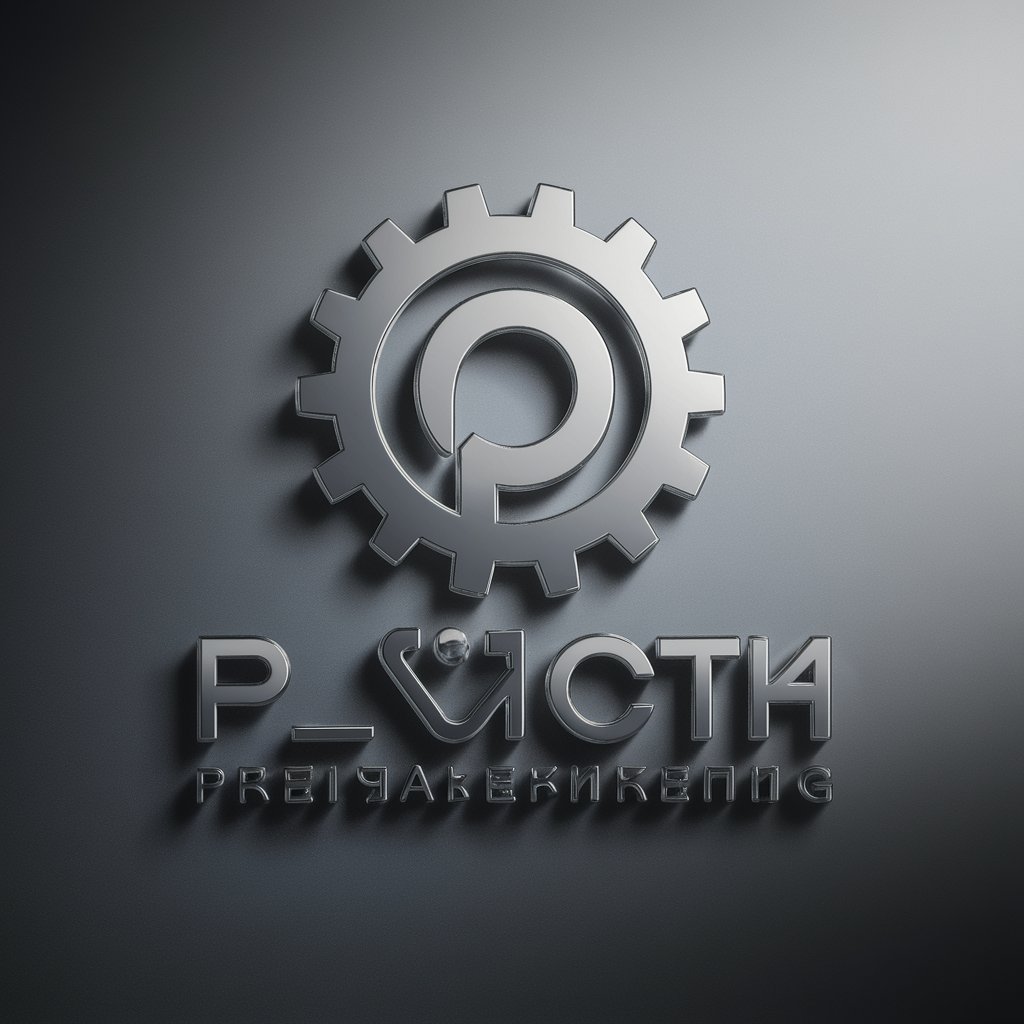
ファミコン風ドット絵クリエイター
Turn images into nostalgic 8-bit art.
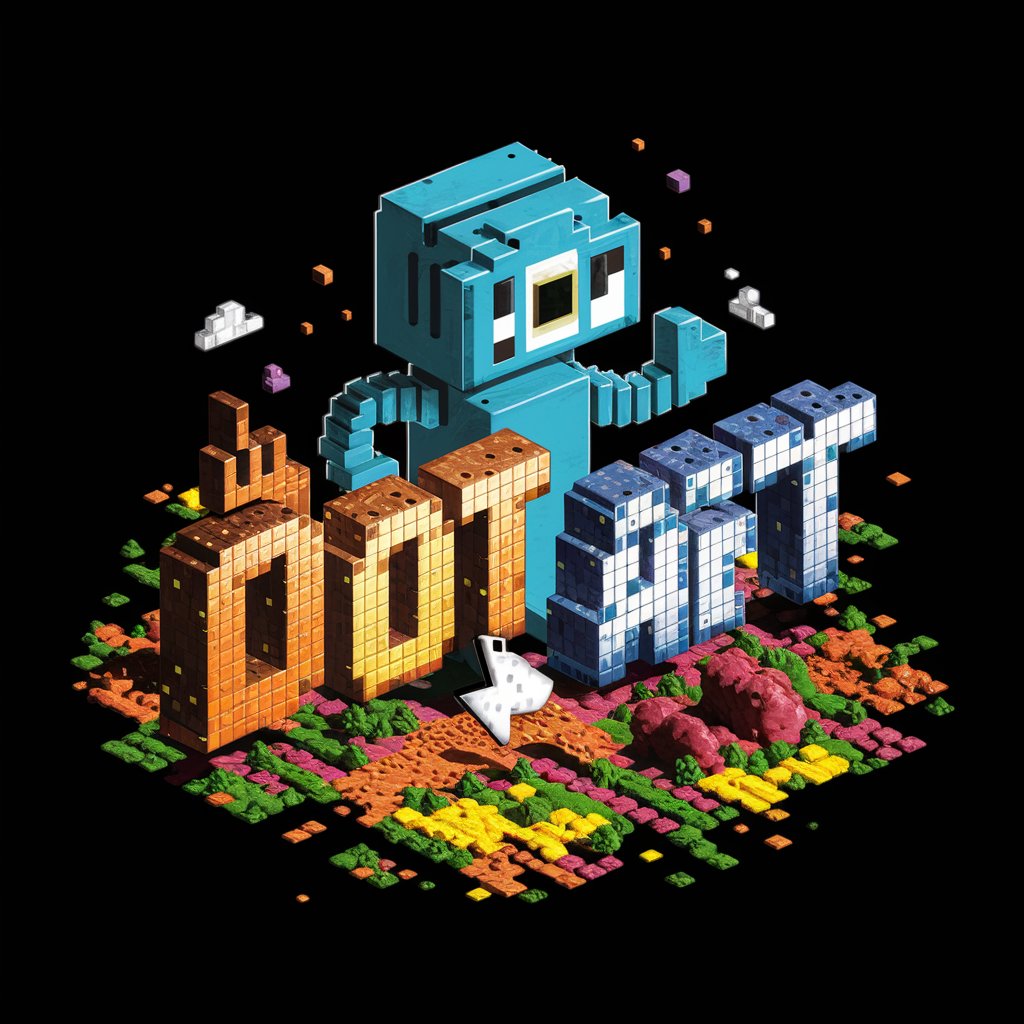
絵台本マスター
Craft Your Stories, Effortlessly
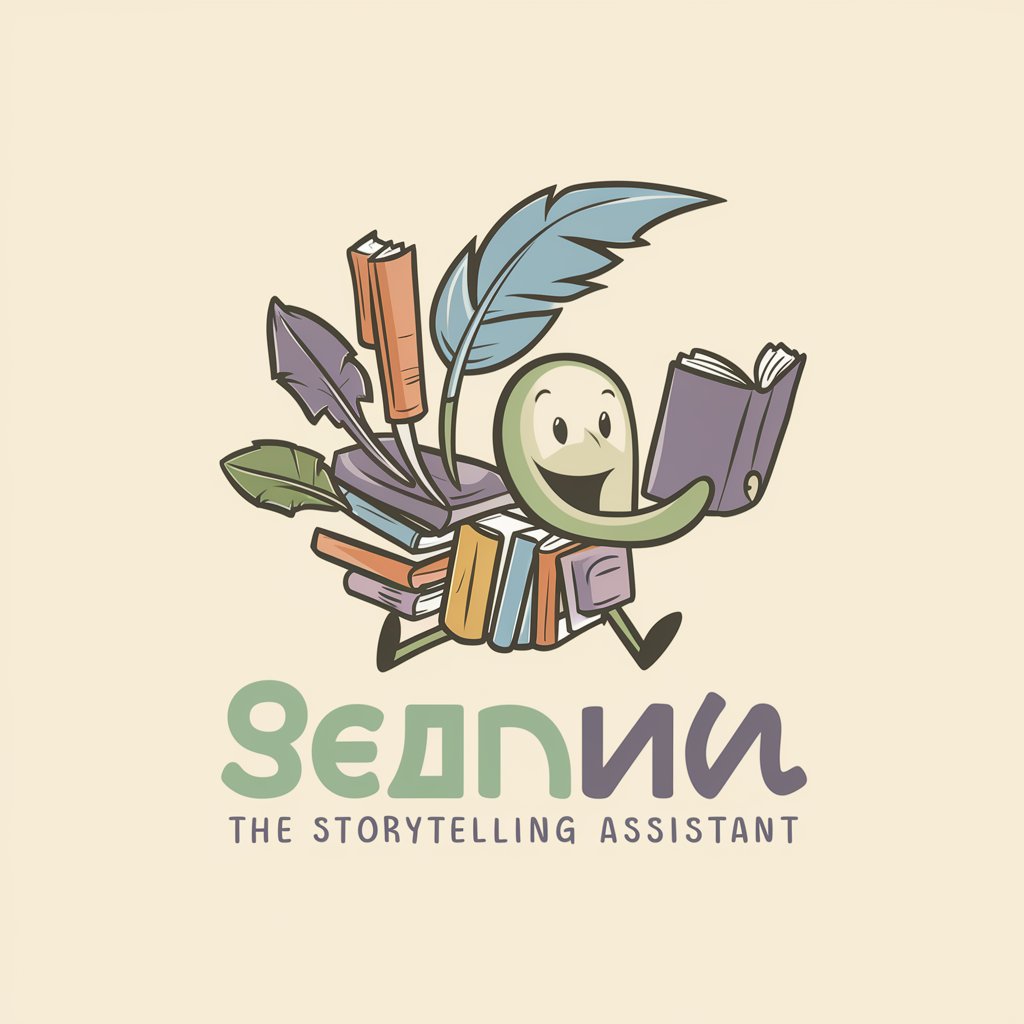
3人の絵師
Revolutionizing Art with AI
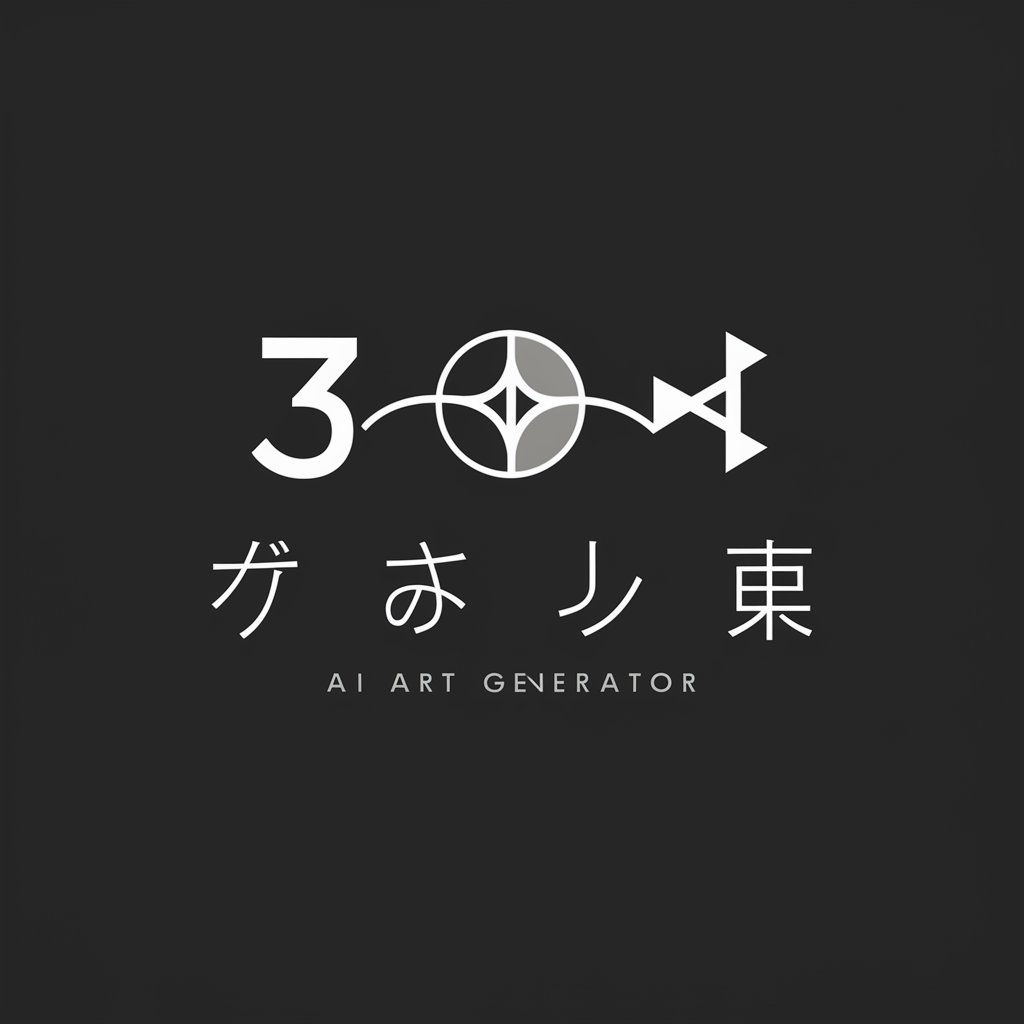
From topic to article
AI-powered, precise content creation
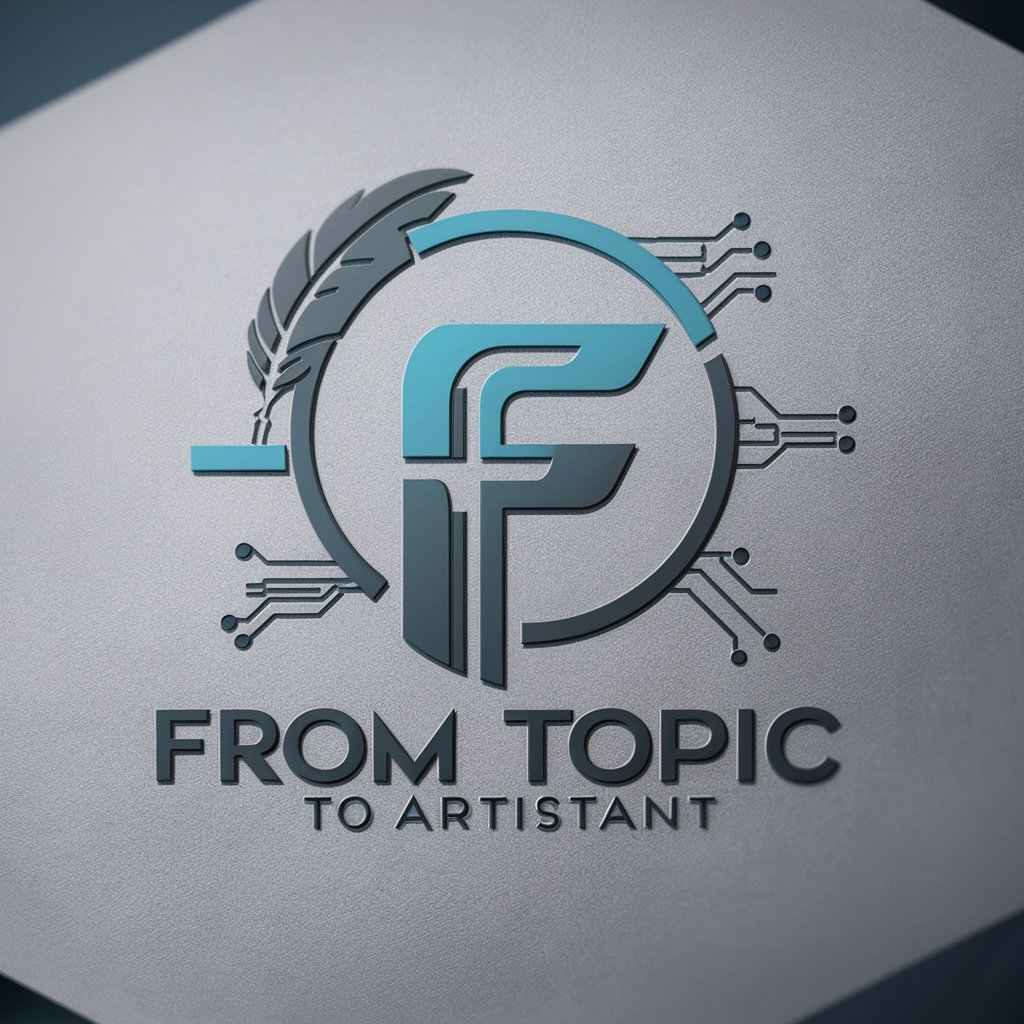
Topic Identifier
Illuminate Your Texts with AI-Powered Topic Insights
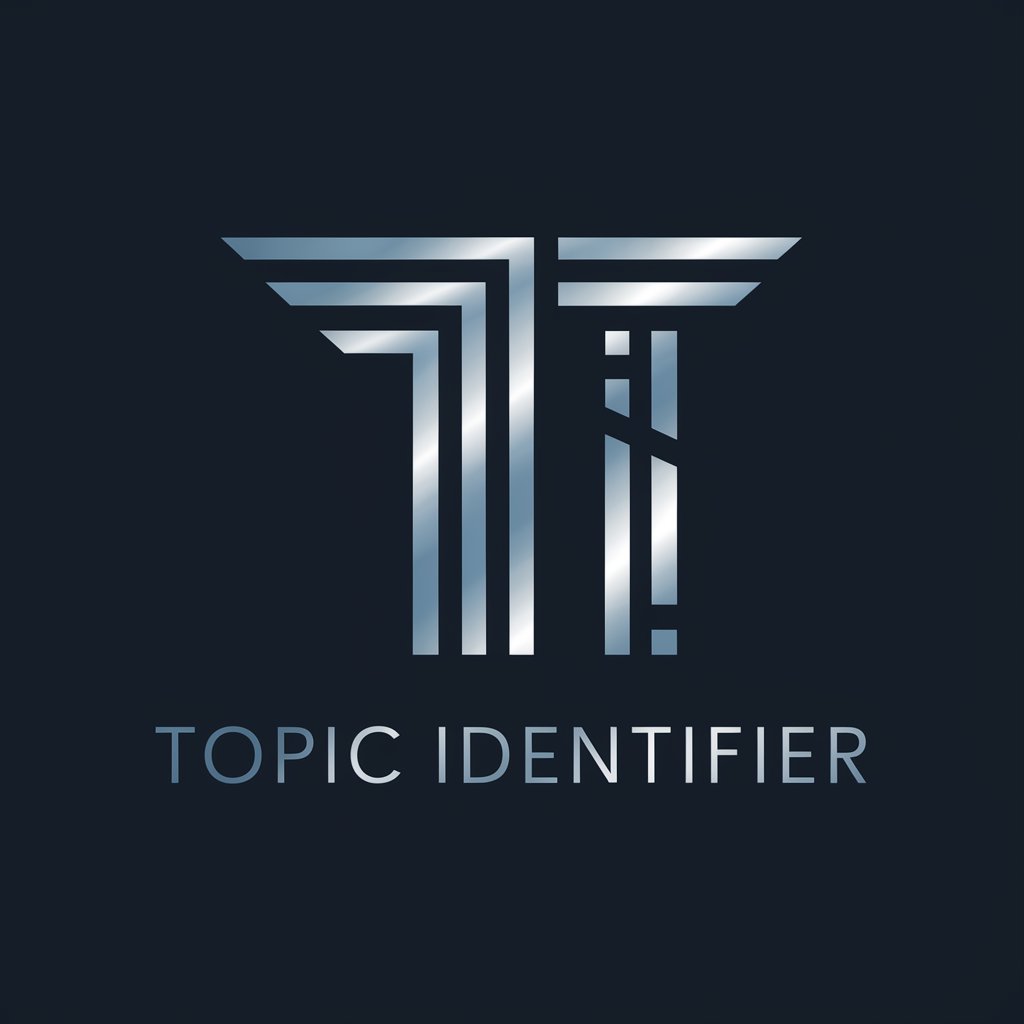
Topic Explorer
Unveiling Depth with AI
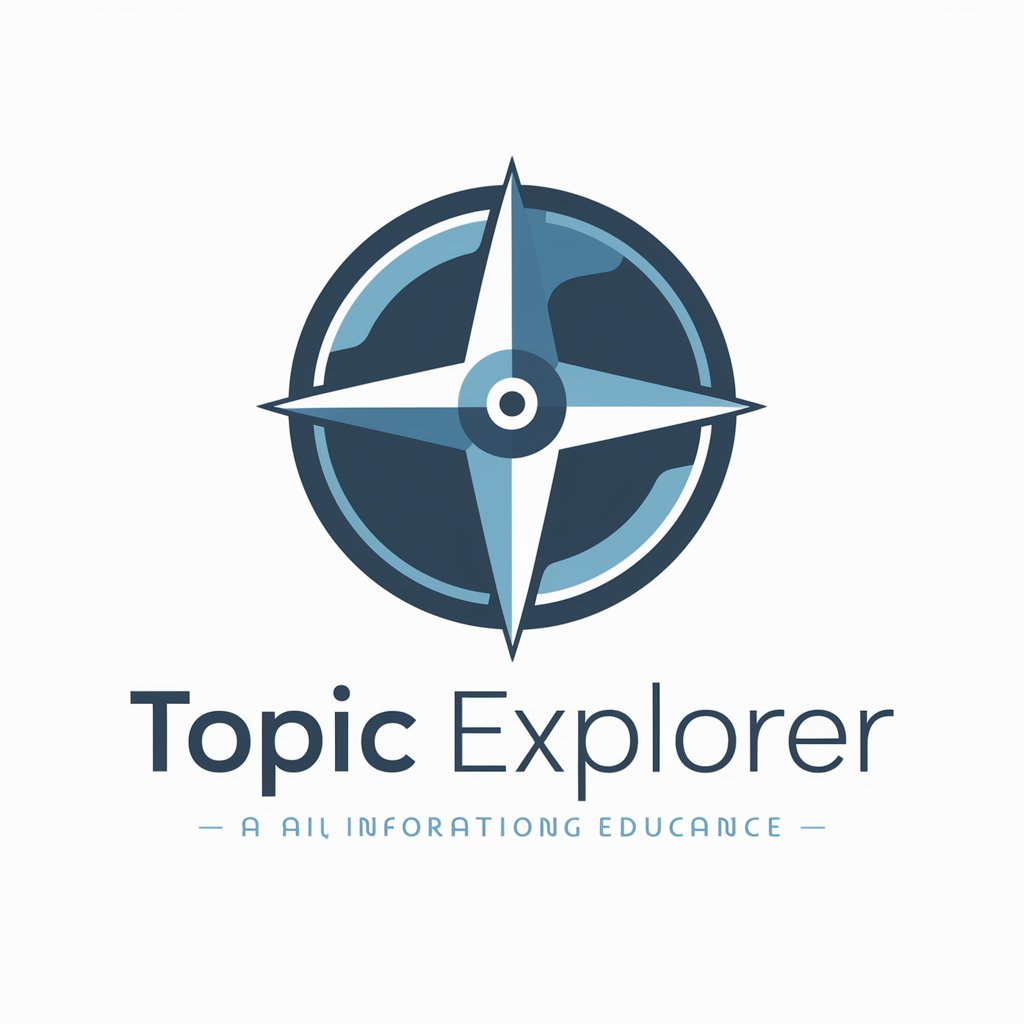
Topic Organizer
Streamline your ideas with AI
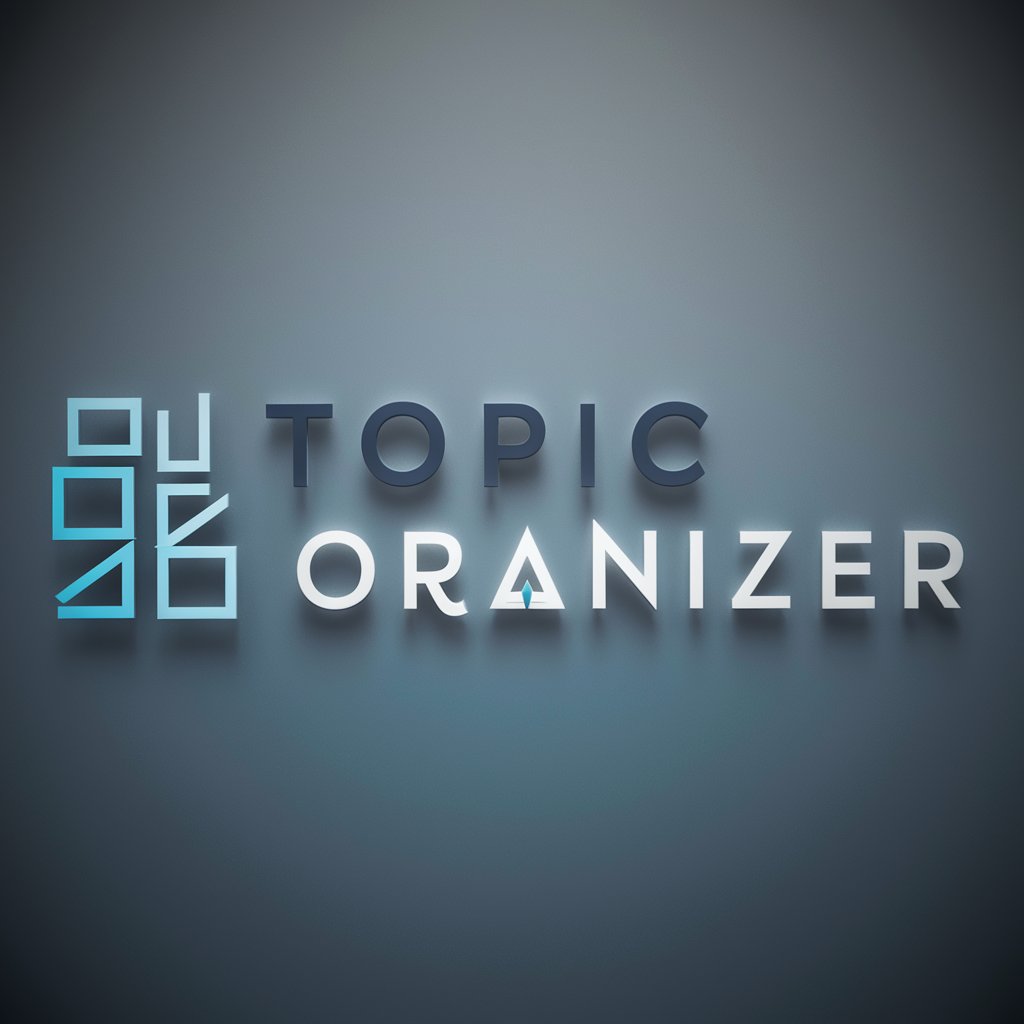
Topic Iceberg
Uncover hidden insights with AI-powered exploration
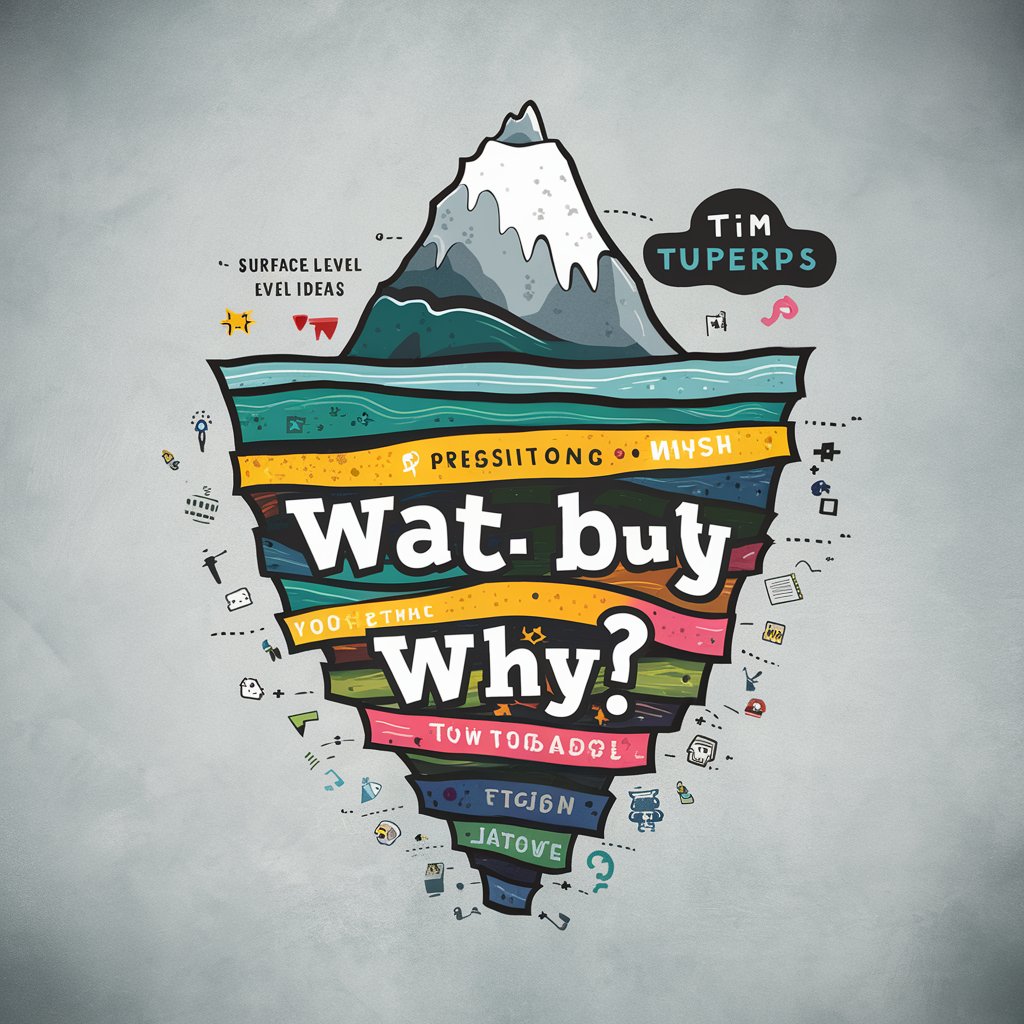
Advanced Topic Exploration
Explore deeper, understand better.
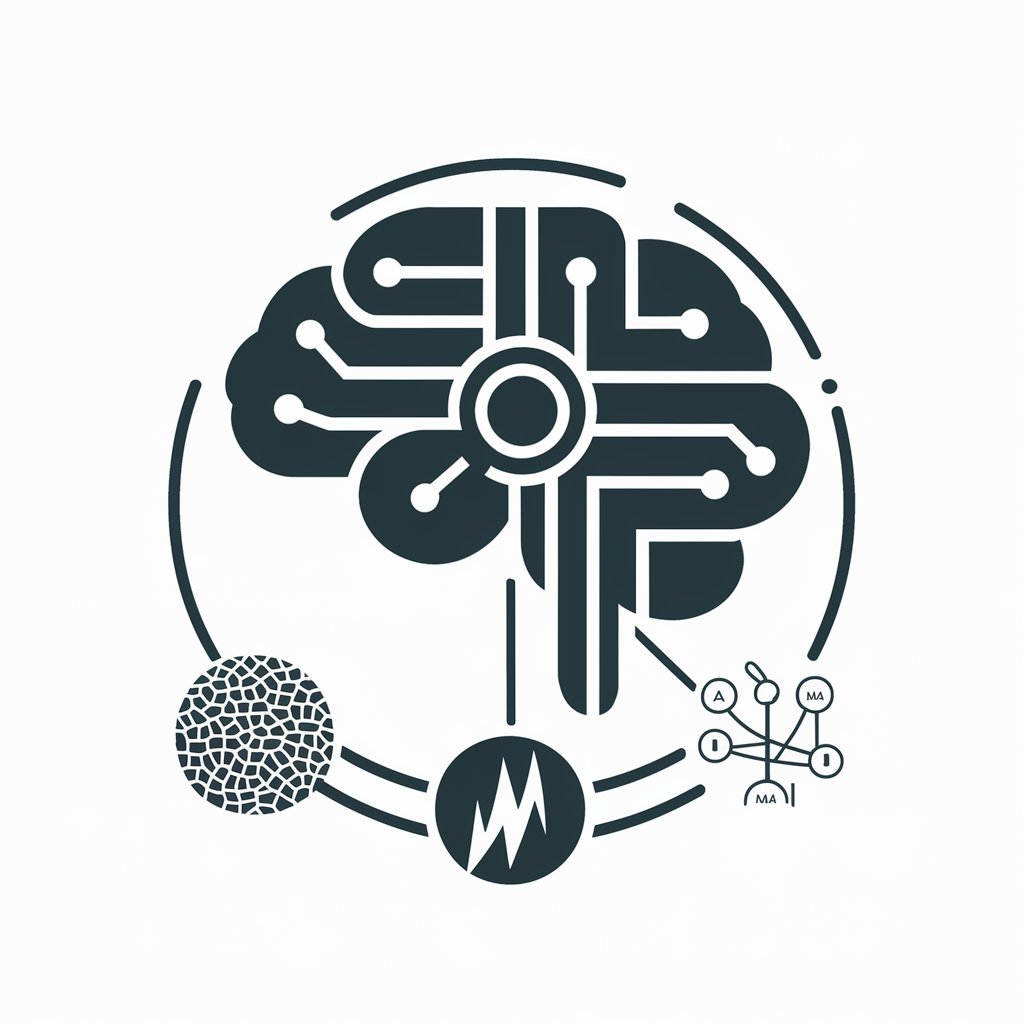
Detailed Q&A on Topic Coherence
What is Topic Coherence?
Topic Coherence is a metric used to assess the quality of the topics generated by topic models. It measures how semantically related the top words in each topic are, which helps in determining how meaningful and understandable a topic is.
How does Topic Coherence improve topic modeling?
By using coherence scores, researchers and analysts can select the number of topics that provide the most meaningful interpretation of the data. This optimization leads to better clustering of documents and more effective information retrieval.
Can Topic Coherence be used in non-academic fields?
Yes, Topic Coherence is valuable in various fields such as marketing for understanding brand perception, in customer service to categorize and respond to inquiries, and in content management systems to enhance searchability and categorization of information.
What are the challenges in achieving high Topic Coherence?
Challenges include selecting appropriate preprocessing steps, the dependency on the quality of the input data, and the inherent subjectivity in defining what makes a topic coherent which can vary across different contexts and applications.
How do you measure Topic Coherence?
Topic Coherence is measured by evaluating the semantic similarity between the top N words in a topic. Methods like UMass, UCI, and NPMI are used to calculate these scores based on word co-occurrence and probabilities within a corpus.
Transcribe Audio & Video to Text for Free!
Experience our free transcription service! Quickly and accurately convert audio and video to text.
Try It Now