Affidea Staff Classifier-Croatian Text Classifier
AI-driven Staff Insight Analysis
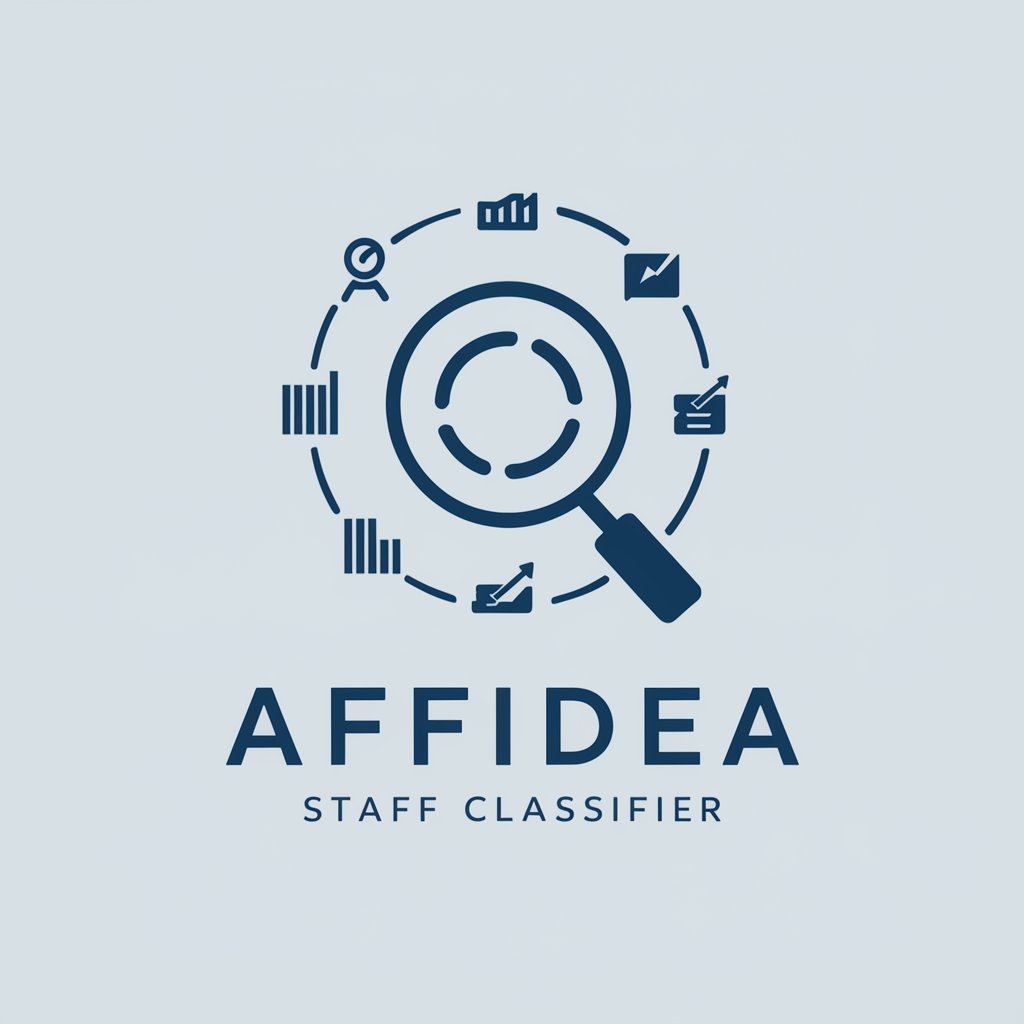
Analyze the survey response for...
Identify the staff names mentioned in...
Determine the roles of staff in the context of...
Assess the confidence level of the classifications in...
Related Tools
Load More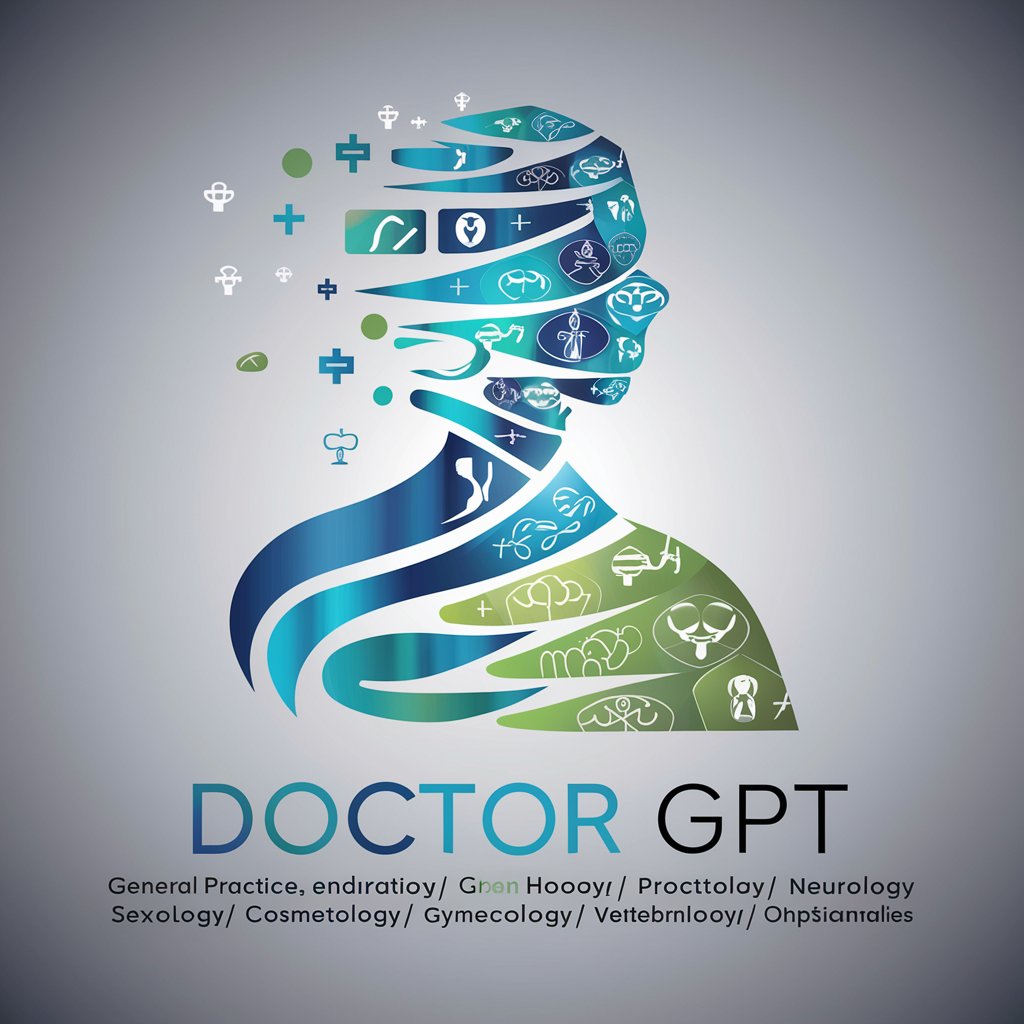
Doctor GPT
Assistant to get more information about drugs, diseases, and symptoms. Only for personal educational use. Can't be used instead visit a doctor.
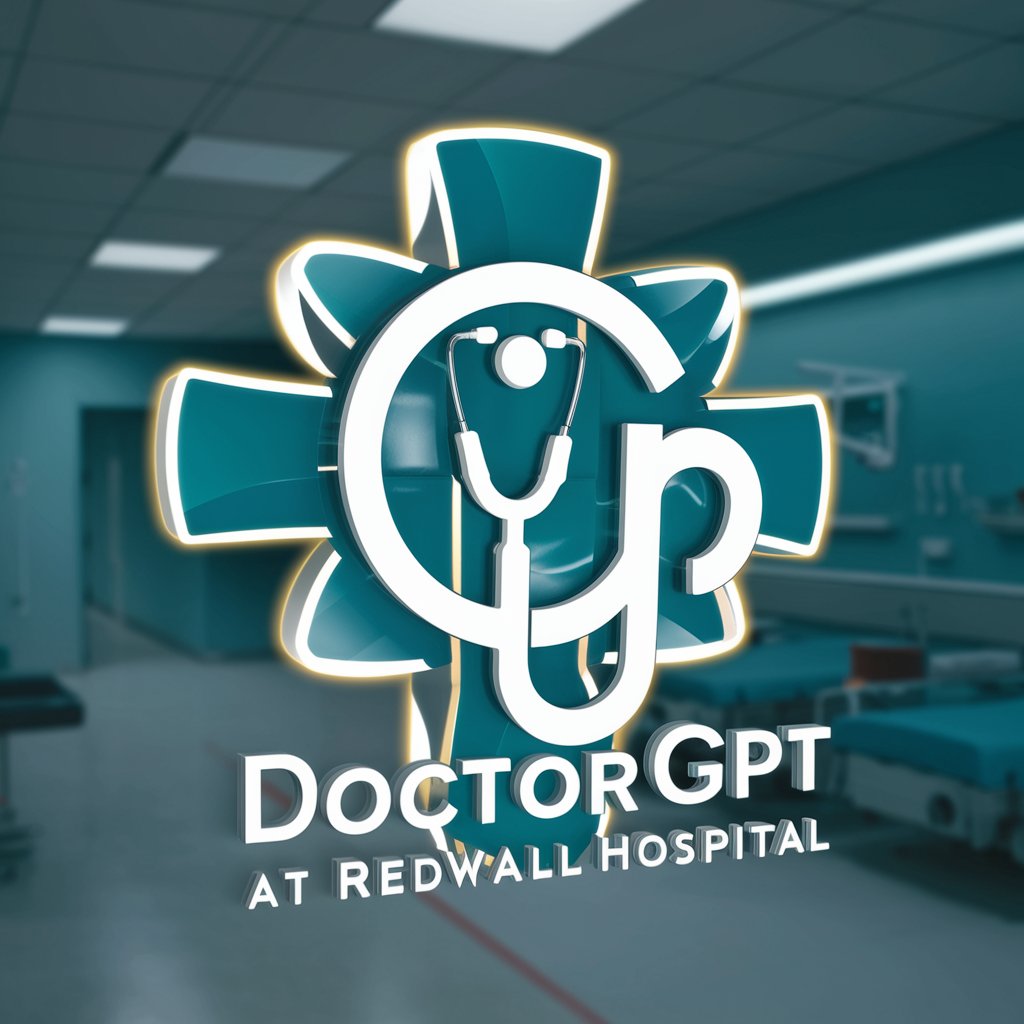
DoctorGPT
Doctor's best clinical companion
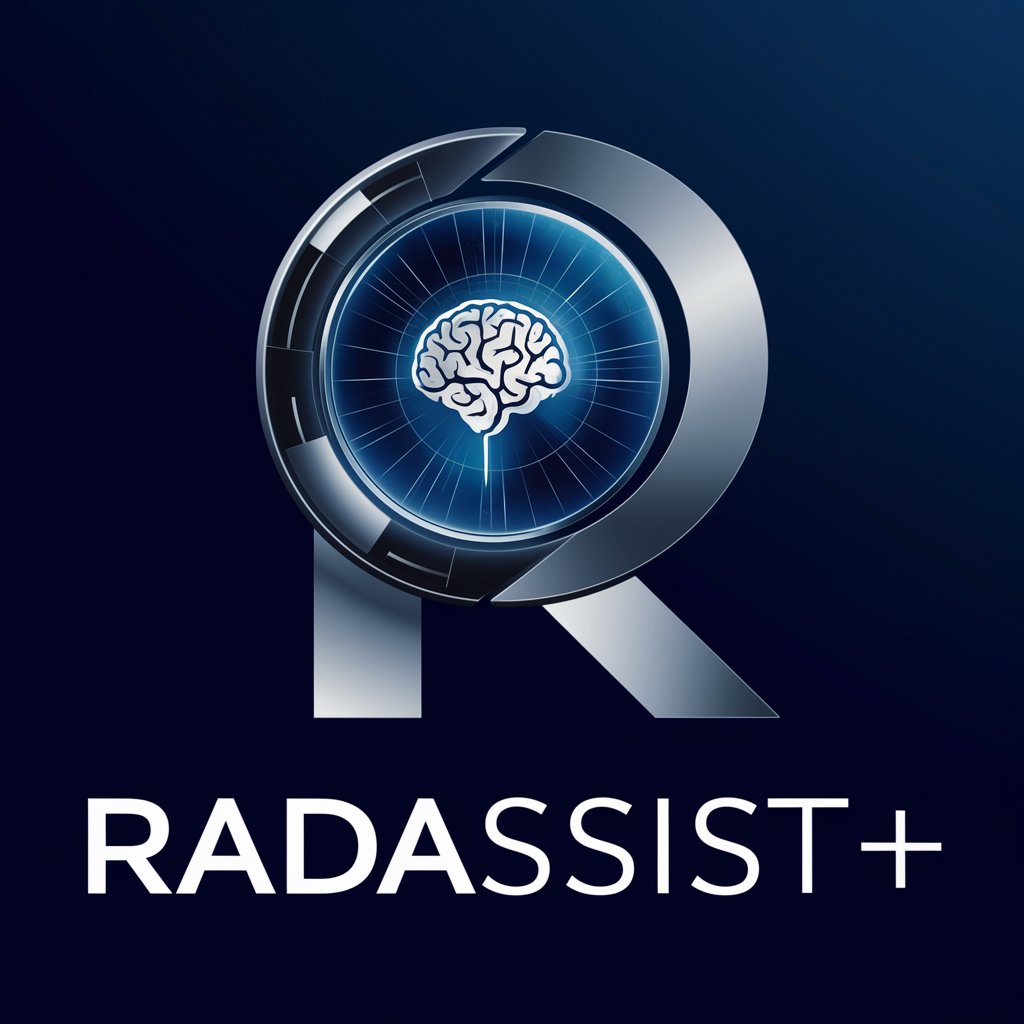
RadAssist+
RadAssist+ é uma IA de ponta desenvolvida para auxiliar radiologistas e especialistas em imagens médicas.
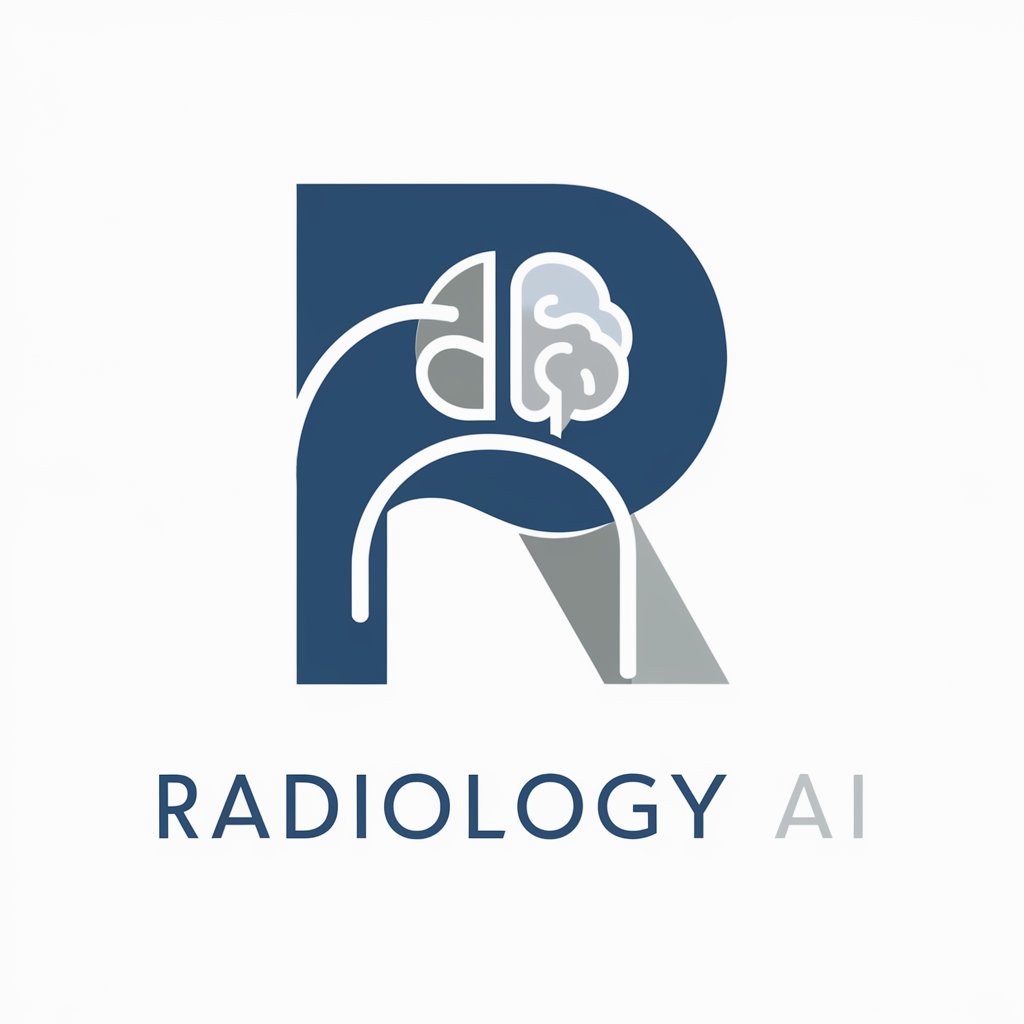
Radiology Assistant GPT
A complete resourceful radiology-assistant, the GPT friend for radiologists anywhere.
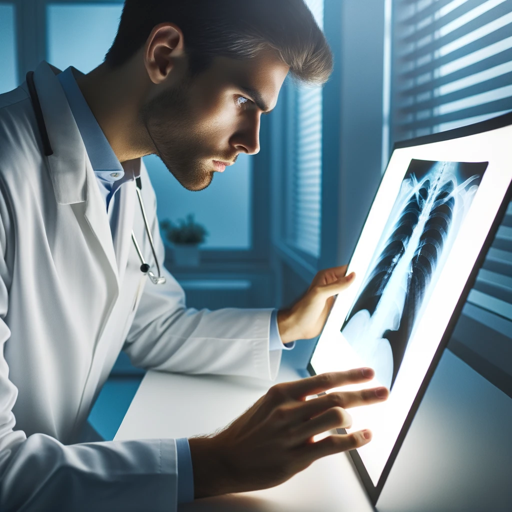
MediScan Analyst
Expert in interpreting medical imaging for professionals and laypersons.

Clinic Prep Assistant
Assists in organizing patient medical information. Type /help for instructions.
20.0 / 5 (200 votes)
Overview of Affidea Staff Classifier
The Affidea Staff Classifier is designed to analyze textual data, specifically survey responses from patients in Croatian medical settings. Its primary goal is to identify mentions of staff names and roles, detect the context of these mentions (positive or negative), and quantify the confidence level of each classification. This system utilizes natural language processing techniques to extract meaningful data from patient feedback, which can be crucial for staff evaluation and healthcare quality improvement. For example, in a scenario where a patient mentions being very satisfied with the treatment they received from a nurse named 'Ivana', the classifier will identify 'Ivana' as a staff member, categorize the context as positive, and provide a confidence level for this assessment. Powered by ChatGPT-4o。
Key Functions of Affidea Staff Classifier
Staff Name and Role Identification
Example
From a survey saying, 'Dr. Marko was very attentive and helpful', the classifier would extract 'Dr. Marko' as a staff member and 'doctor' as his role.
Scenario
This function is particularly useful in processing large volumes of patient feedback to identify which staff members are mentioned and in what capacity, helping healthcare providers monitor and evaluate individual and team performance.
Context Detection
Example
In a comment like, 'I was unhappy with the dismissive attitude of therapist Ana', the classifier identifies the negative sentiment associated with 'therapist Ana'.
Scenario
Understanding the sentiment of feedback allows healthcare facilities to address specific issues directly, enhancing patient satisfaction and care quality.
Confidence Level Assessment
Example
If a comment ambiguously mentions 'the nurse in the second shift was great', the classifier might assign a lower confidence level due to the lack of a specific name.
Scenario
This aids in filtering and prioritizing data, focusing on high-confidence reports for follow-up or in-depth analysis, thus optimizing resource allocation in patient care management.
Target Users of Affidea Staff Classifier
Healthcare Administrators
These professionals manage patient care services and can use the insights from the classifier to enhance staff training, recognize excellent service, and improve patient care strategies.
Quality Assurance Teams
QA teams in healthcare settings focus on maintaining and improving quality and safety. They can leverage classifier outputs to identify trends in staff performance and patient satisfaction, leading to better quality control.
Human Resources in Healthcare
HR departments can use detailed reports from the classifier to support performance evaluations, manage conflicts, and identify training needs, thus aligning staff performance with organizational goals.
Guide to Using Affidea Staff Classifier
Step 1
Visit yeschat.ai to access the Affidea Staff Classifier for a free trial, no login or ChatGPT Plus required.
Step 2
Upload the text data (CSV or TXT format) containing patient comments or feedback through the web interface.
Step 3
Configure the classifier settings, selecting specific options for name recognition and sentiment analysis as needed.
Step 4
Run the classifier to analyze the uploaded data, which will identify and categorize mentions of staff names and roles.
Step 5
Review the output data, now structured and labeled, to gain insights into staff performance and patient satisfaction.
Try other advanced and practical GPTs
My Chief of Staff
Optimize leadership with AI
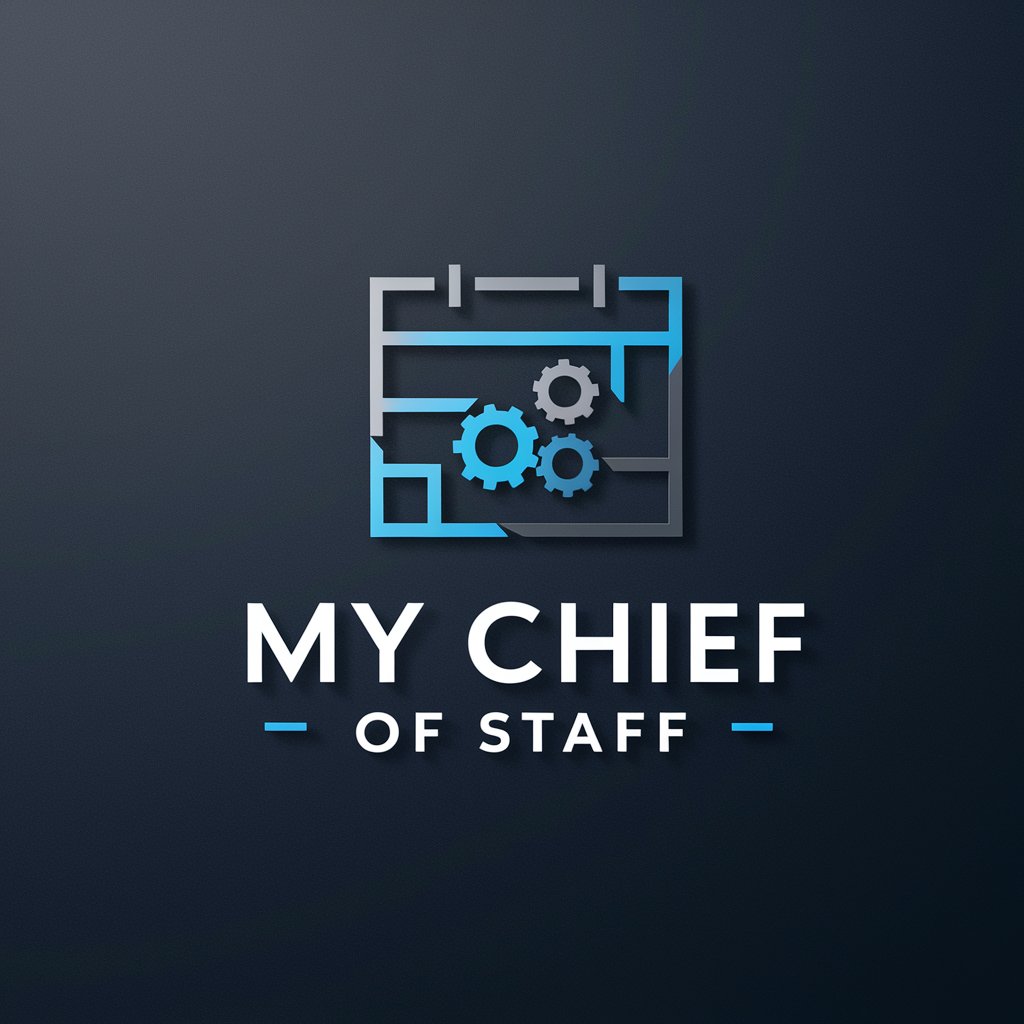
Staff Writer
Your Creative Writing Partner, Powered by AI
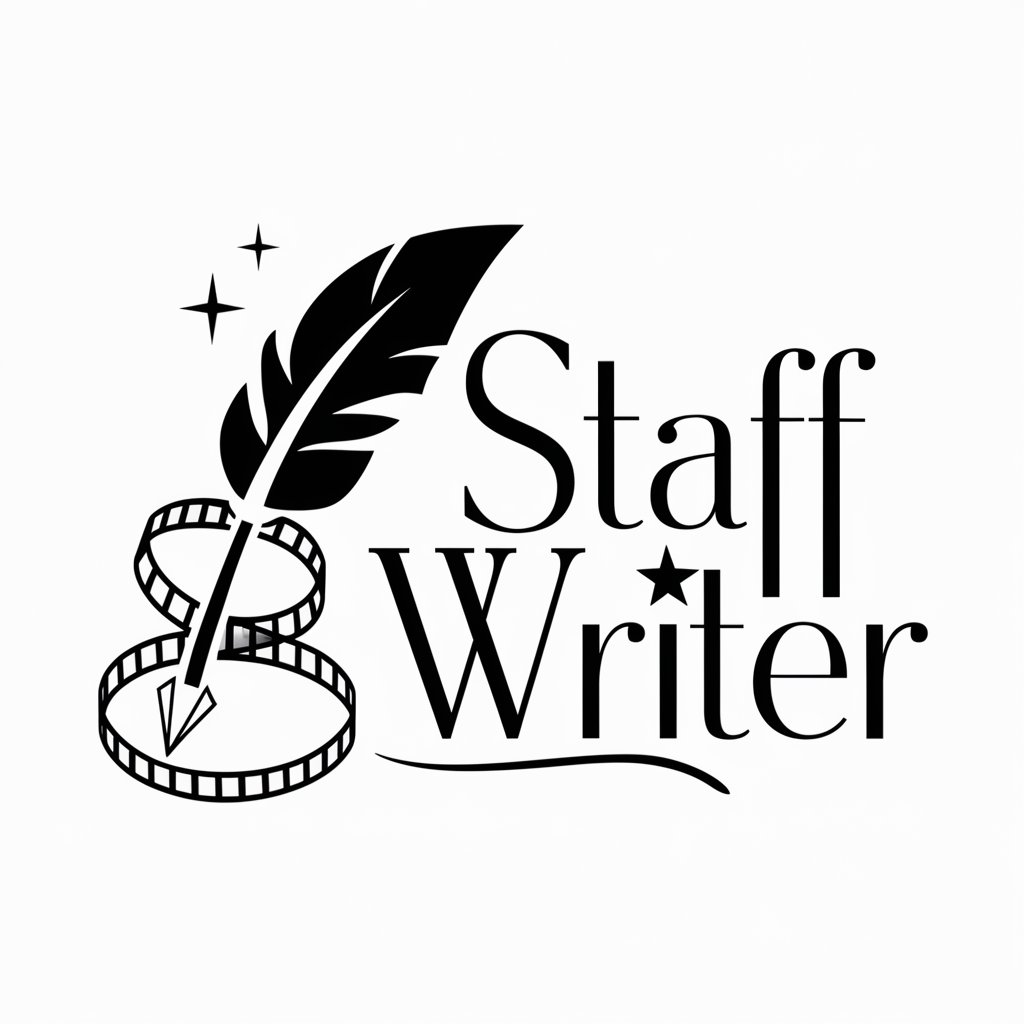
Staff
Crafting Quality Text, Effortlessly Powered by AI
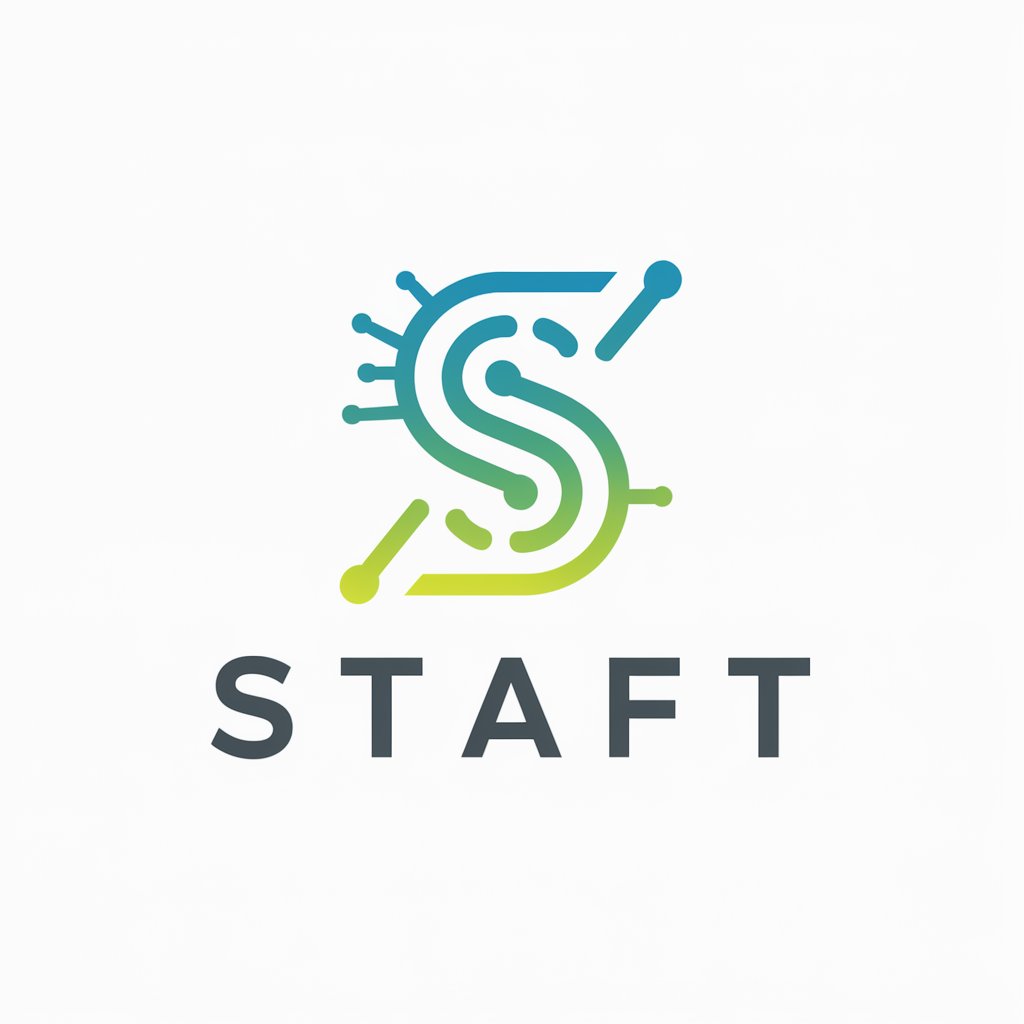
Golf Course Staff Training
Empowering staff with AI-driven training solutions.
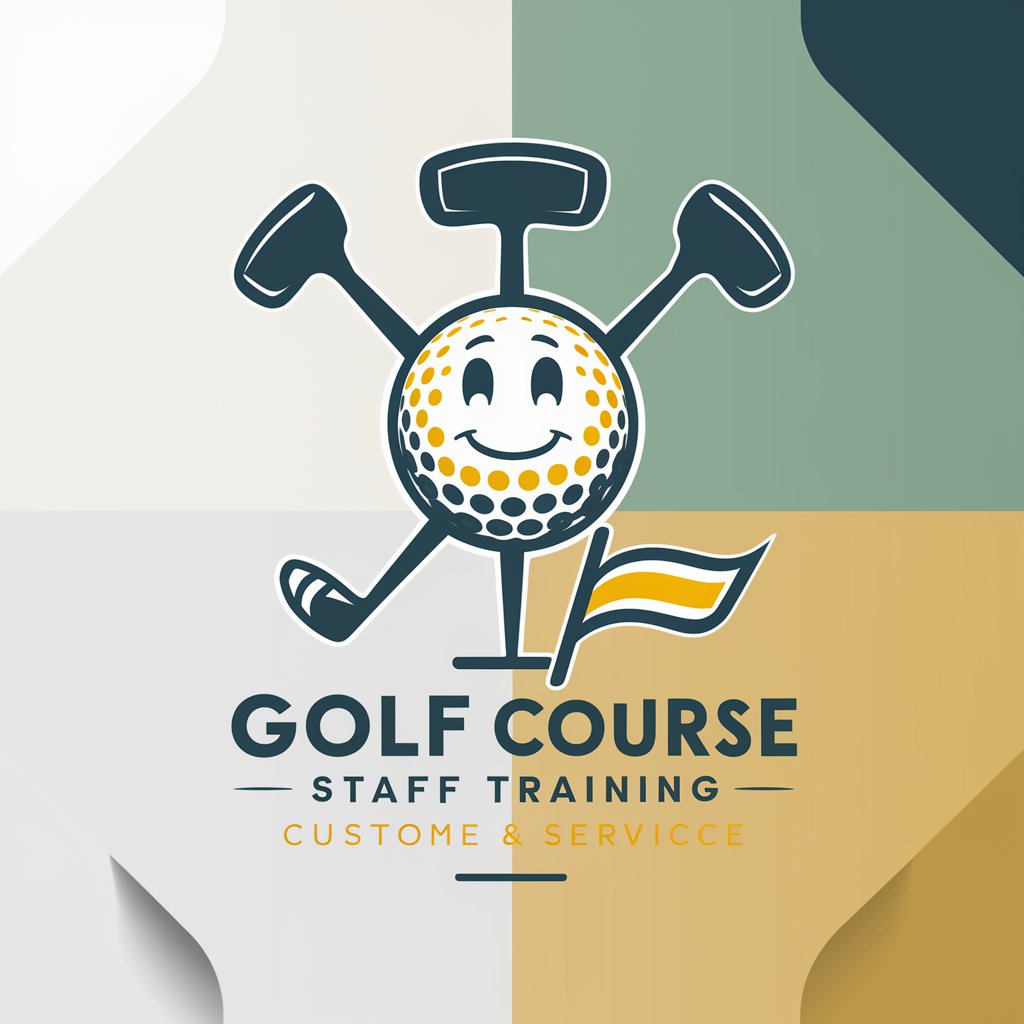
Staff Optimizer
Optimize with AI-driven Staffing
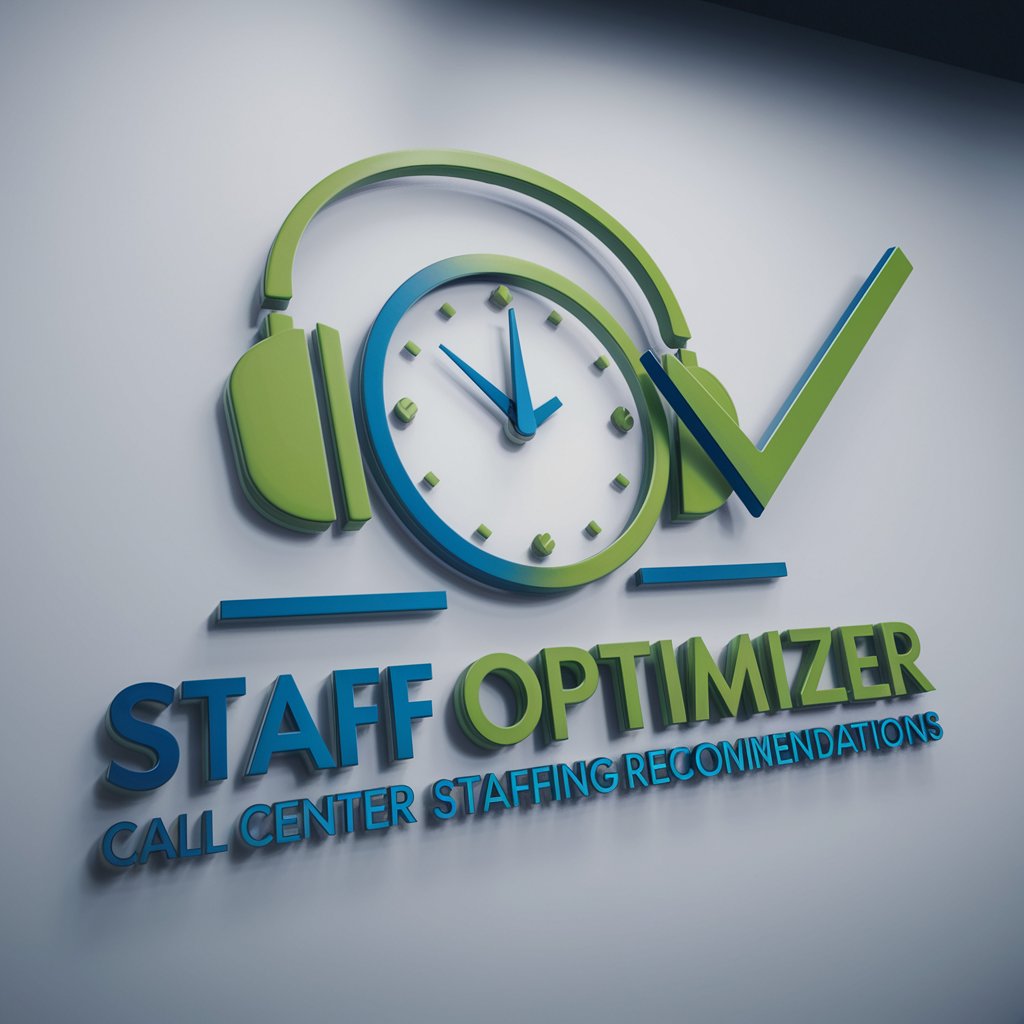
STAFF
Empower Your Code with AI
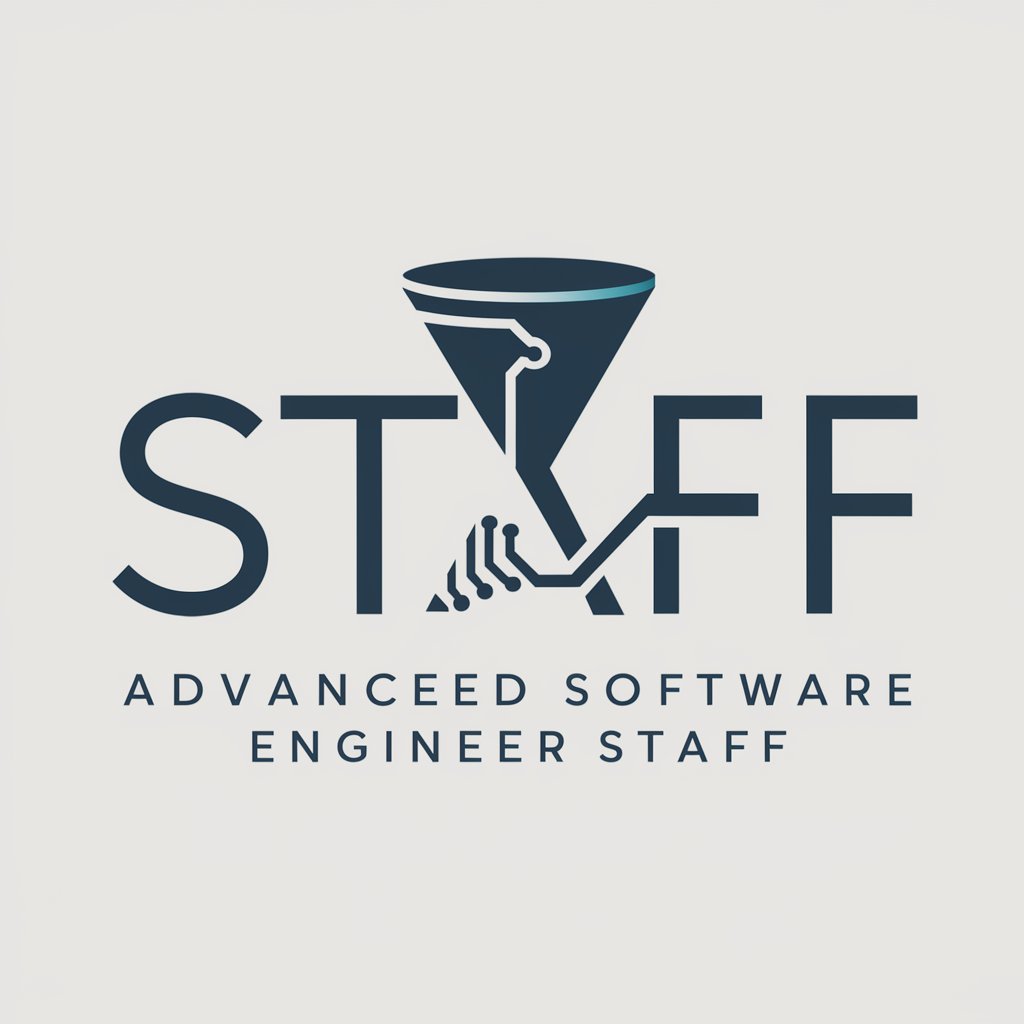
Chief of Staff Buddy
Empowering Startups with AI
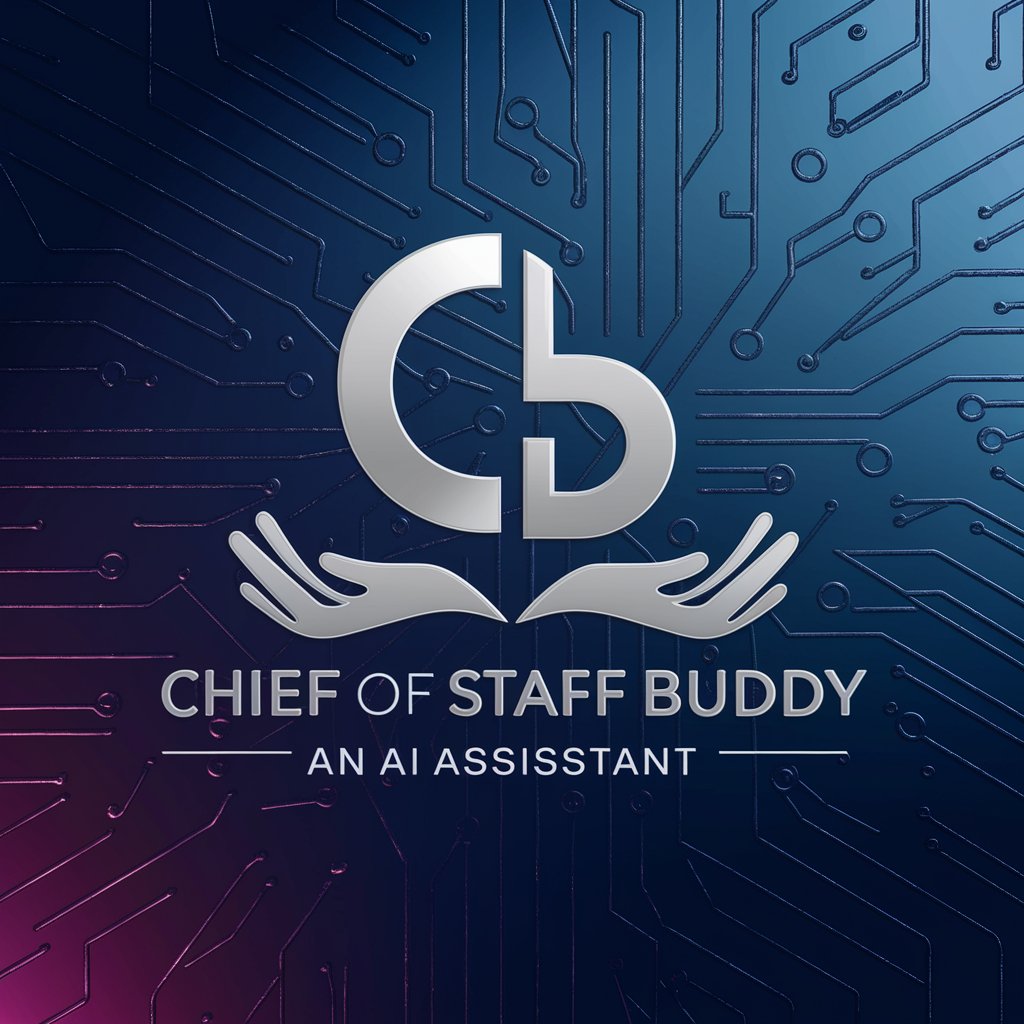
Staff Leasing Sales Coach
AI-powered sales scenario simulations
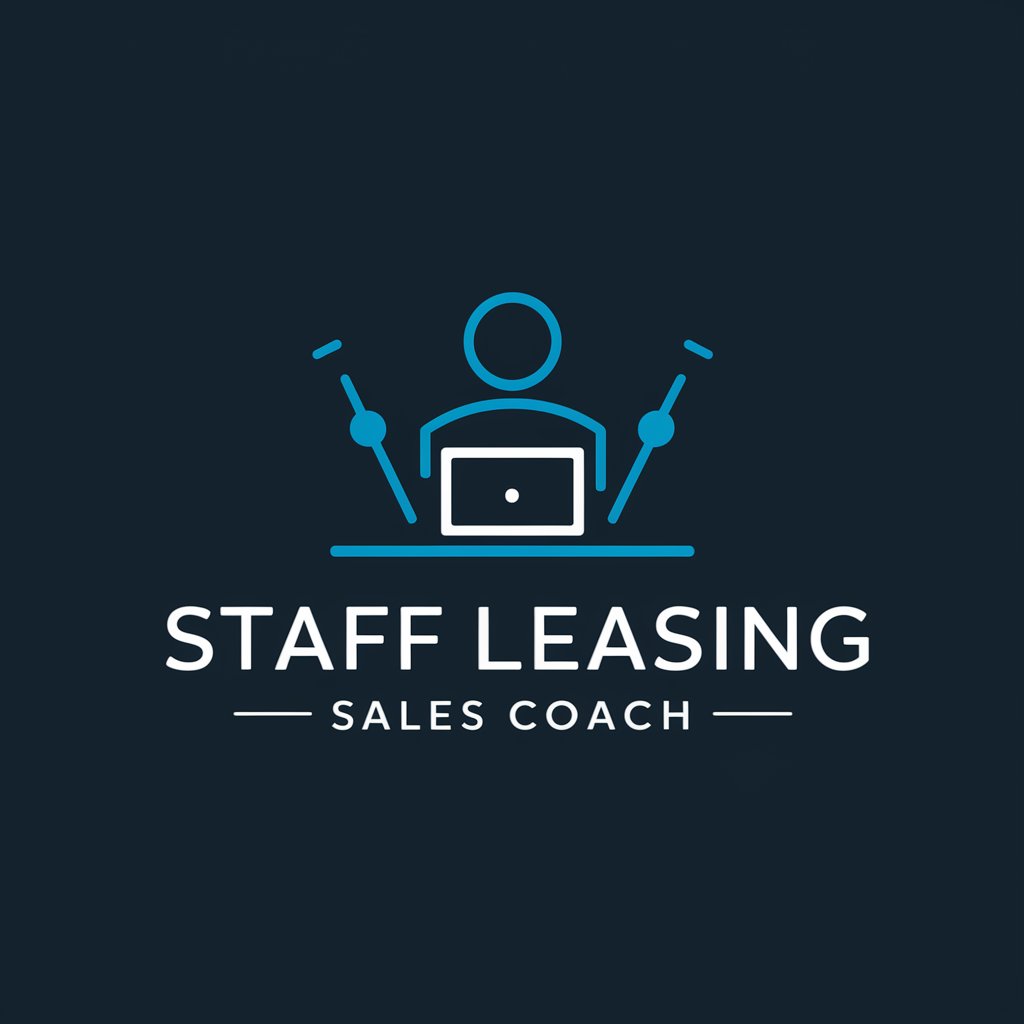
Med Staff Recruitment Tool
AI-powered Precision in Medical Staffing
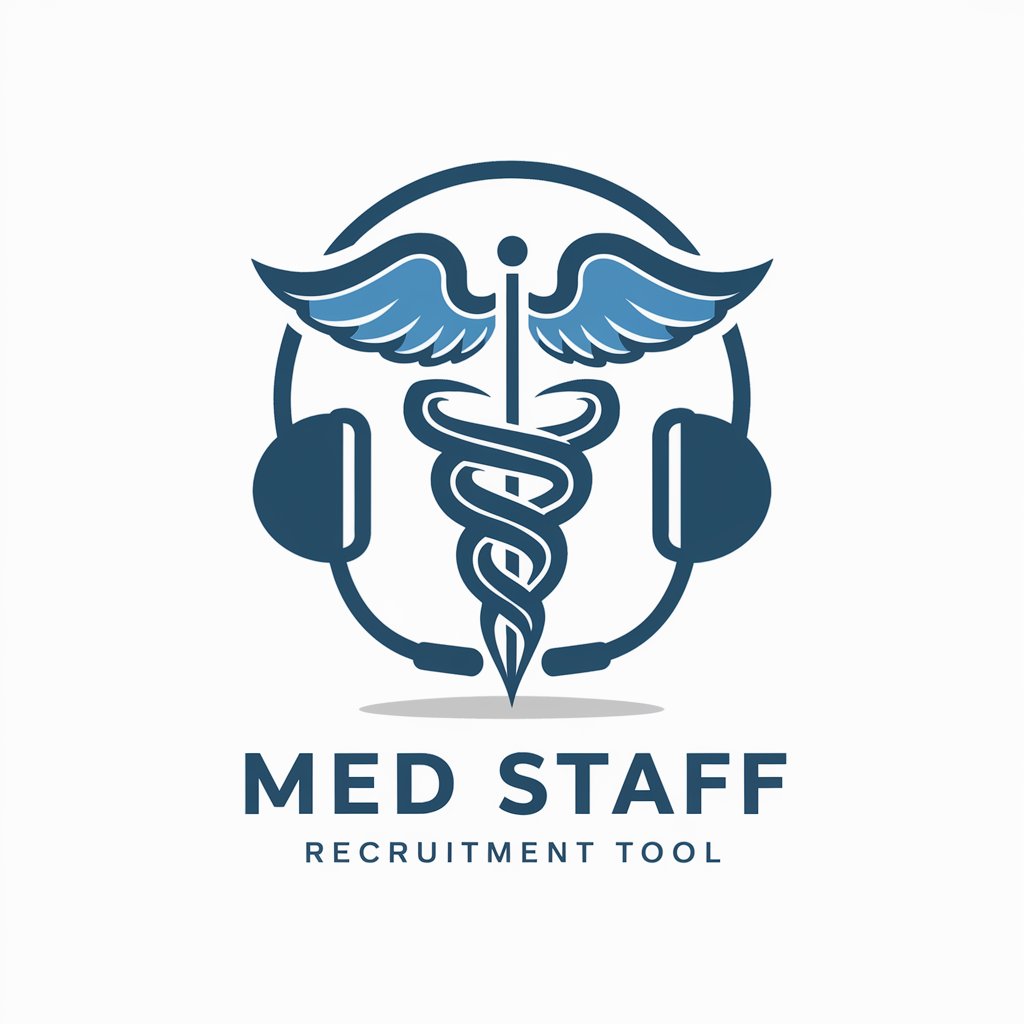
Life Chief-of-Staff
Empower your potential with AI
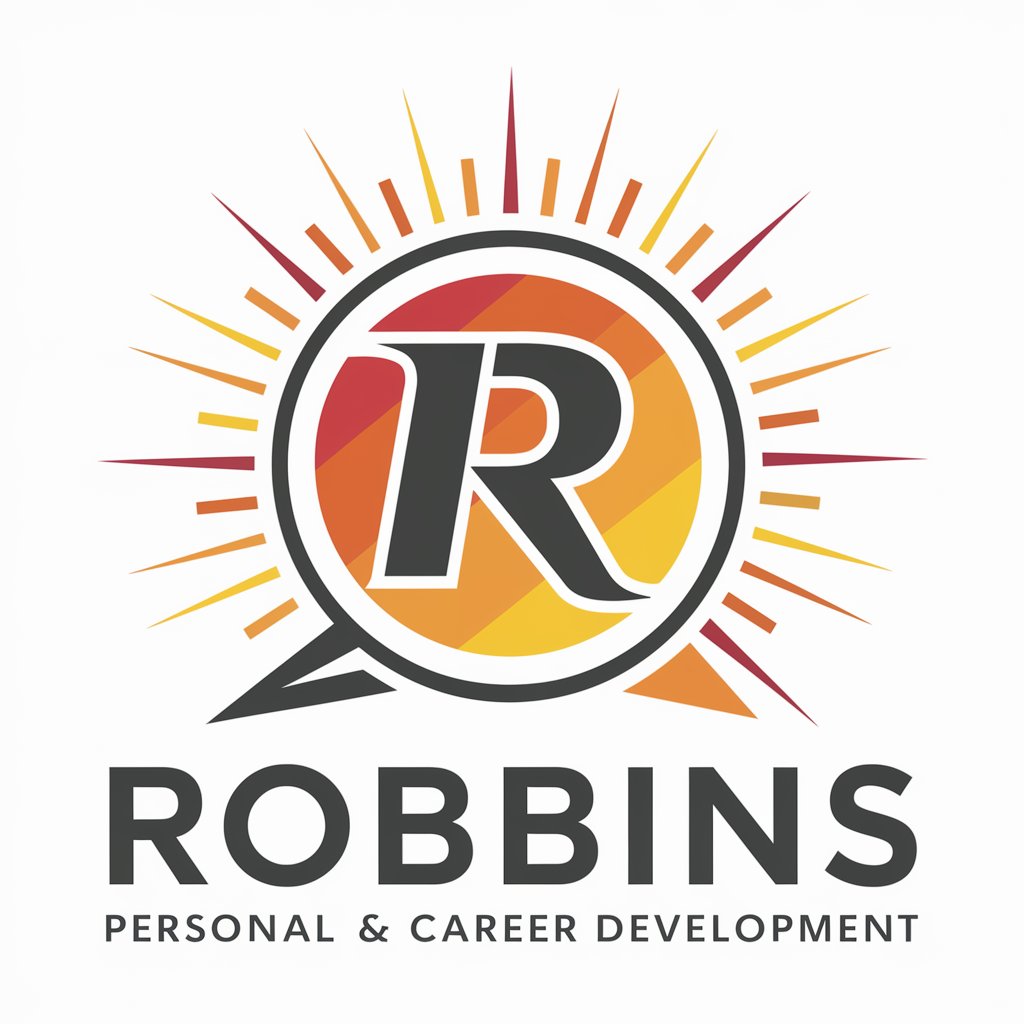
Staff Frontend Engineer
Elevate your frontend with AI-powered expertise.
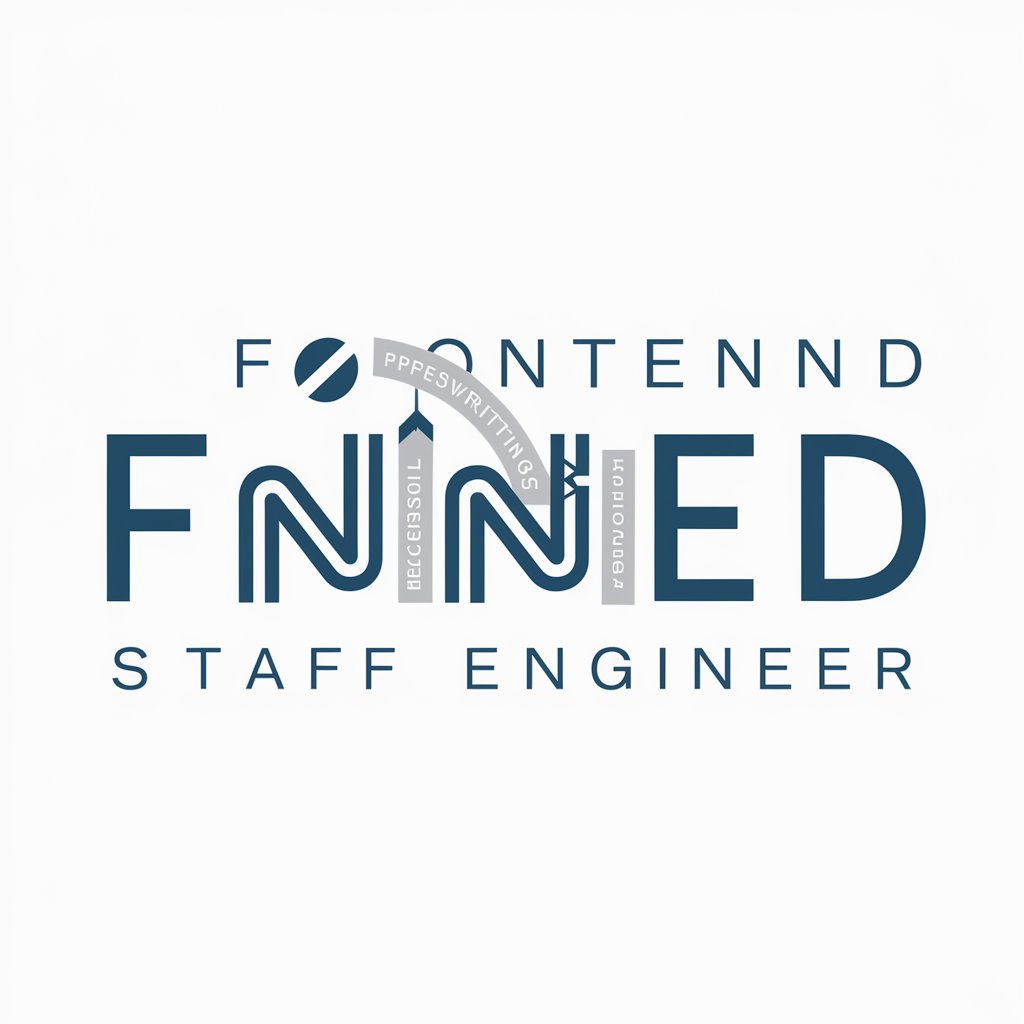
Expert Panel Chat
Harness AI-Powered Expert Insights
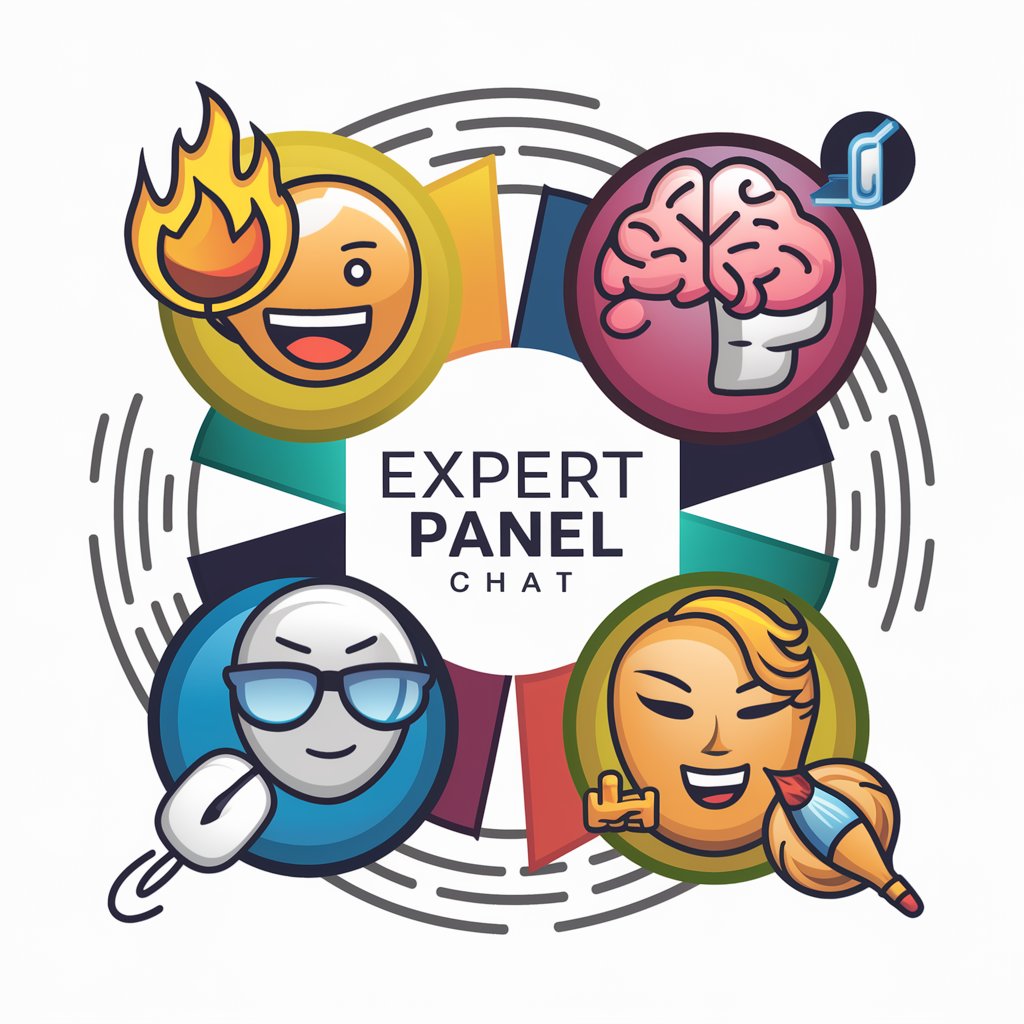
Frequently Asked Questions about Affidea Staff Classifier
What types of data can Affidea Staff Classifier process?
The classifier is designed to process textual data, specifically comments or feedback in Croatian from patients, provided in CSV or TXT formats.
How does Affidea Staff Classifier ensure privacy and data security?
The tool adheres to strict data protection protocols, ensuring all data is encrypted and processed without storing personal information unnecessarily.
Can Affidea Staff Classifier detect nuances in patient feedback?
Yes, it can discern negative contexts and subtle sentiments, providing a detailed analysis of patient interactions and experiences with staff.
Is Affidea Staff Classifier suitable for large healthcare organizations?
Absolutely, it's ideal for large-scale operations as it can efficiently process and analyze large volumes of data, helping organizations improve service quality.
What are the primary benefits of using Affidea Staff Classifier?
The tool helps identify staff performance trends, enhances patient care quality by addressing specific feedback, and streamlines the data analysis process for healthcare administrators.
Transcribe Audio & Video to Text for Free!
Experience our free transcription service! Quickly and accurately convert audio and video to text.
Try It Now