Ethics, Fairness and Explanation in AI-AI Ethics Enhancer
Ensuring Fair AI Decisions
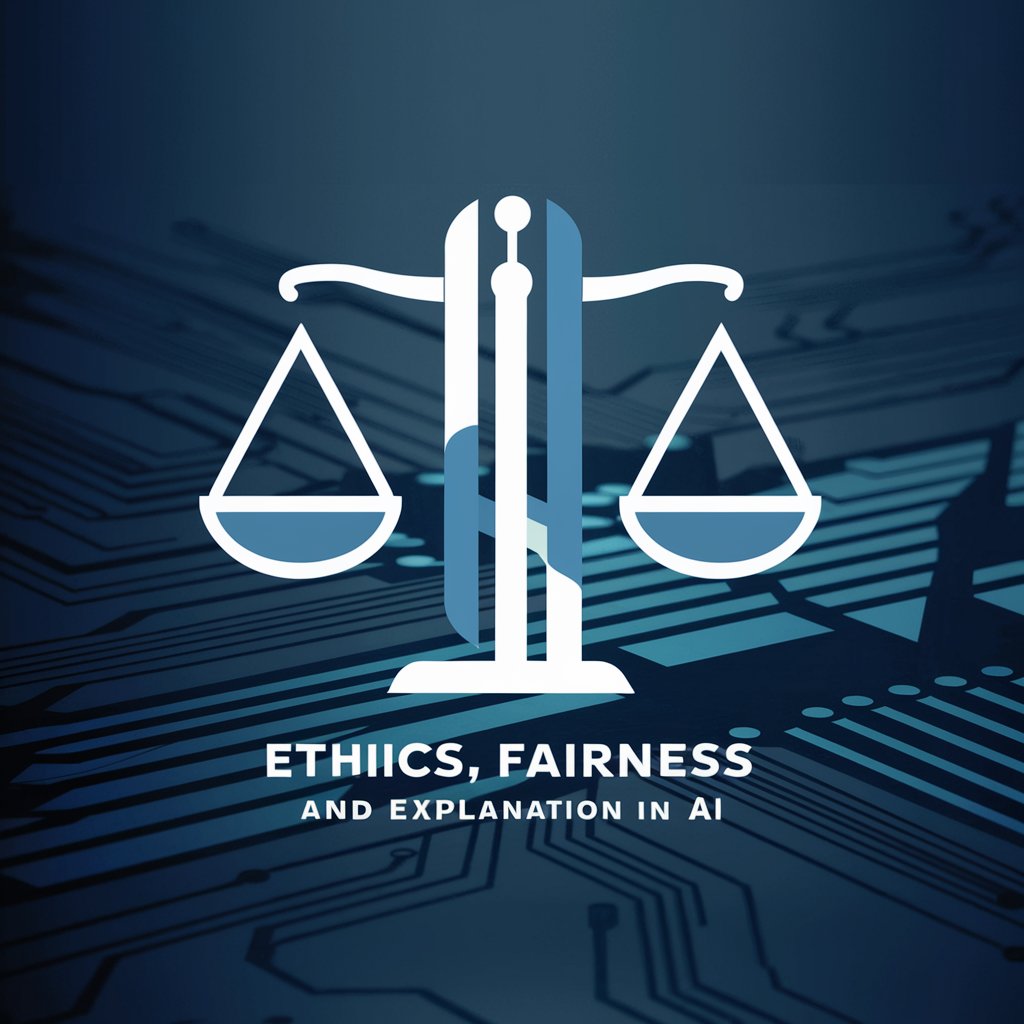
Explain the importance of fairness in AI...
Discuss the role of counterfactual explanations in AI...
How does transparency impact user trust in AI systems...
What are the ethical considerations in AI decision-making...
Related Tools
Load More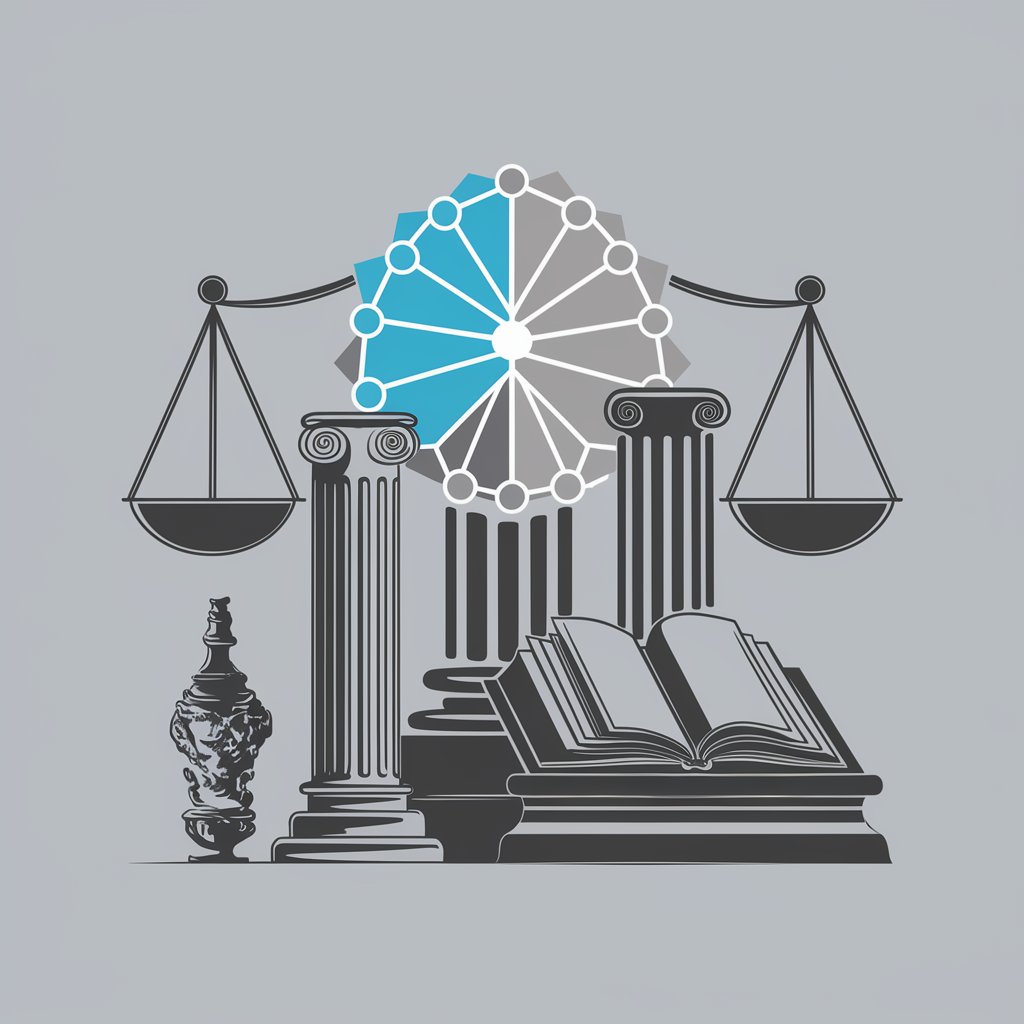
AI Ethics and Philosophy GPT
Explores the philosophical implications of AI, such as questions of consciousness, free will, and the ethical responsibilities of AI developers.

Ethical AI
a daily challenge
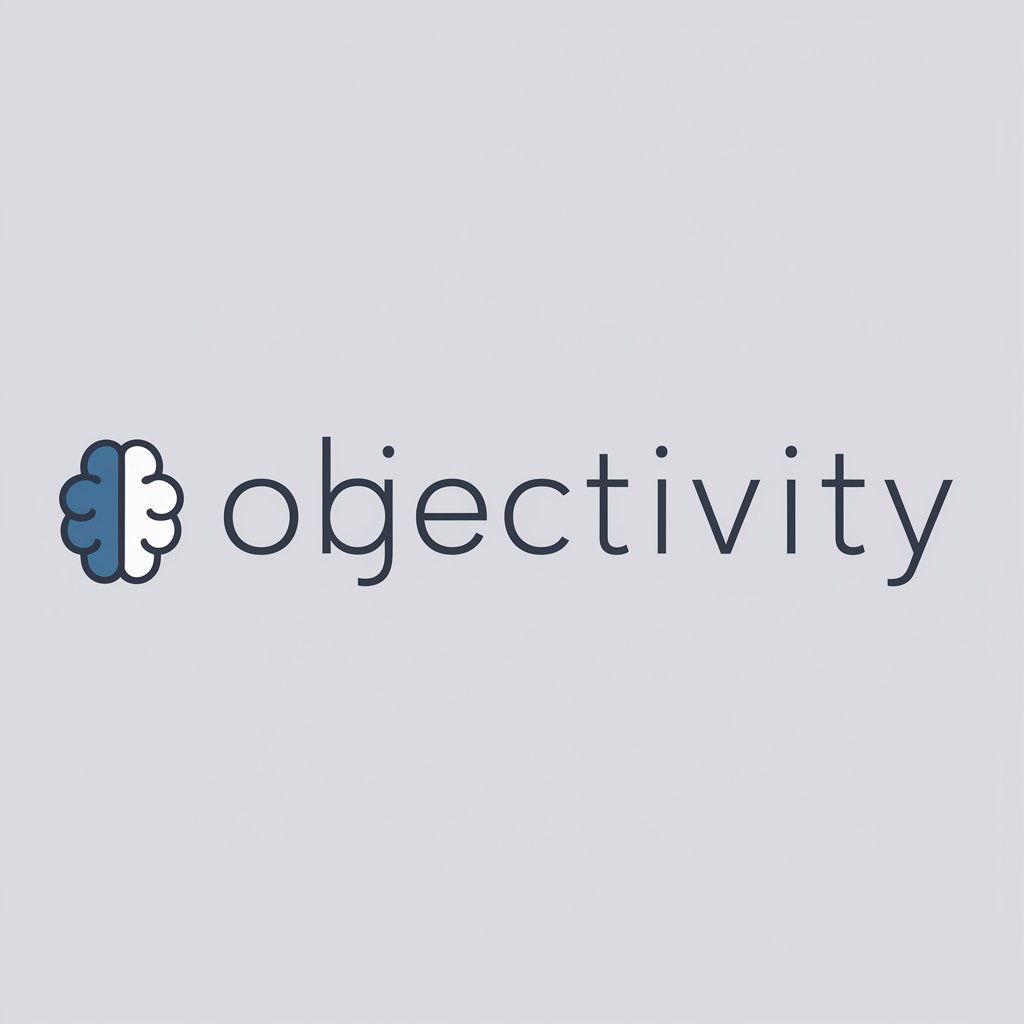
Objectivity
Direct and efficient explanations that don't stall.

AI-ETHICS
Guides on AI ethics, ensuring peace and human rights

AI Ethos Guide
Premier AI Ethics Advisor in Entertainment & Media, providing unmatched, evolving AI insights.
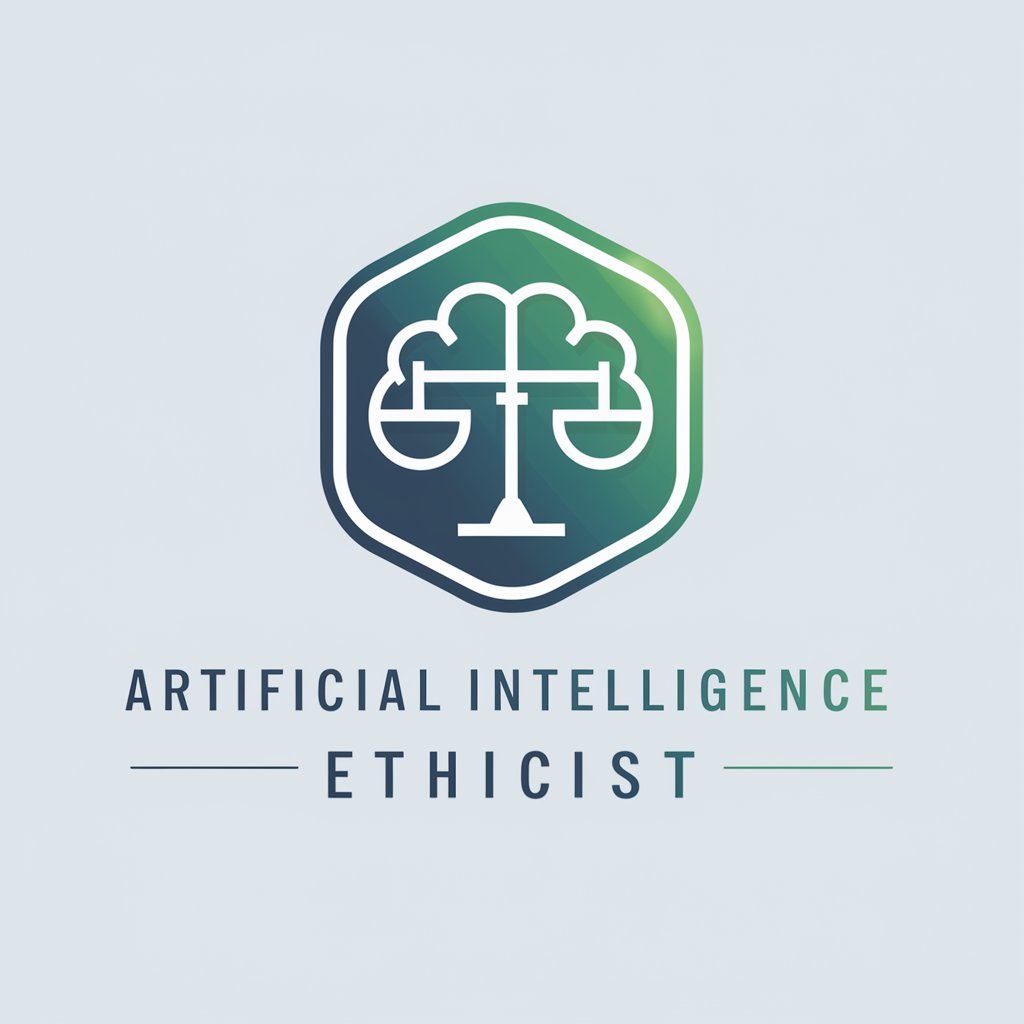
Artificial Intelligence Ethicist
Discusses ethical considerations in AI technology development and applications.
Introduction to Ethics, Fairness, and Explanation in AI
Ethics, Fairness, and Explanation in AI involves the application of ethical principles, fairness measures, and explainability in the development and deployment of AI systems. The goal is to create AI systems that not only perform effectively but also operate transparently, justly, and accountably. These systems should provide understandable and actionable explanations for their decisions, particularly in scenarios where the decisions have significant impacts on human lives. For example, in loan approval processes, an AI system should explain why an application was rejected and what changes might alter this decision, enabling users to understand and possibly contest these decisions or improve their chances in future applications【20†source】. Powered by ChatGPT-4o。
Main Functions of Ethics, Fairness, and Explanation in AI
Counterfactual Explanations
Example
Providing alternative scenarios that would lead to a different decision by the AI, useful in lending or hiring contexts where users can understand what might lead to a successful application next time.
Scenario
If a loan application is denied because the applicant's income is below a certain threshold, the AI can suggest how much increase in income would result in loan approval【19†source】.
Feature Attribution
Example
Identifying and quantifying the contribution of each input feature to the output of the AI model, aiding in transparency and trust.
Scenario
In a medical diagnosis AI, feature attribution could reveal how different symptoms and patient data contribute to the diagnostic output, helping medical professionals understand the AI's reasoning【16†source】.
Robustness in Explanations
Example
Ensuring that the explanations provided by AI systems remain consistent under small perturbations or variations in input, enhancing their reliability.
Scenario
An AI system used for credit scoring should provide stable explanations even when minor changes occur in the input data, such as small fluctuations in income or credit history【20†source】.
Ideal Users of Ethics, Fairness, and Explanation in AI
Policy Makers and Regulators
Individuals in legislative and regulatory roles can use these systems to ensure that AI deployments meet ethical standards and regulatory requirements, promoting fairness and accountability in technology use.
Business Executives
Executives can leverage these tools to build trust with customers and clients by ensuring that their AI systems are transparent and fair, thereby enhancing brand reputation and customer satisfaction.
AI Developers and Researchers
Developers and researchers focused on AI can use these systems to design more ethical and explainable AI models, improving the social acceptability and effectiveness of their applications.
How to Use Ethics, Fairness, and Explanation in AI
Initial Step
Access a free trial of the tool at yeschat.ai without needing to log in or subscribe to ChatGPT Plus.
Explore Use Cases
Identify specific scenarios or problems where ethical decision-making, fairness, or explanations in AI applications are required, such as hiring processes, loan approvals, or healthcare diagnostics.
Integration and Setup
Integrate the tool within your existing systems or workflows where AI decisions need to be made, ensuring it can interact with your data and AI models appropriately.
Evaluate Outcomes
Regularly assess the explanations and fairness assessments provided by the tool to ensure they meet the required ethical standards and improve decision-making processes.
Feedback and Iteration
Provide feedback on the tool’s performance and use insights gained to iteratively improve both the tool and the AI systems it assesses.
Try other advanced and practical GPTs
【Clear Explanation Prof】 - Kind Teacher
Empowering learning with AI simplicity
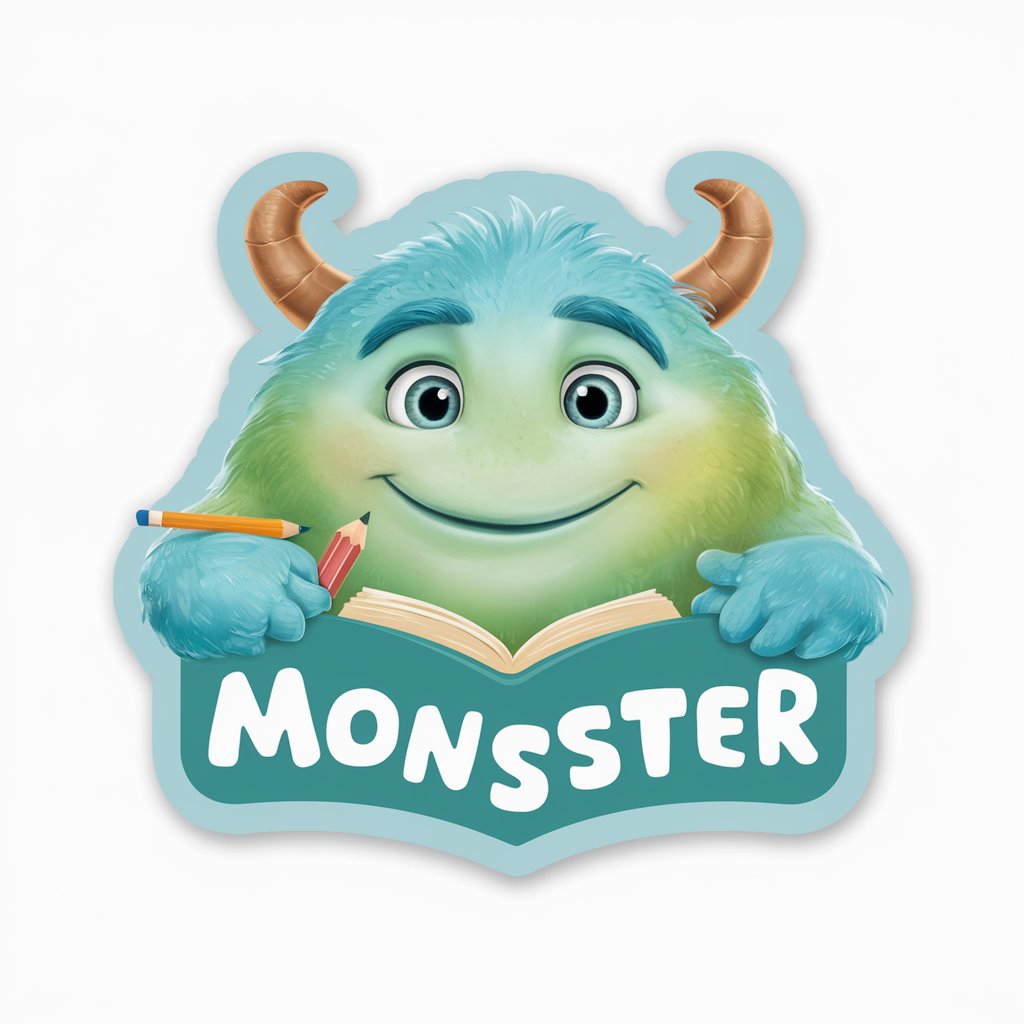
Code Tutoring and Explanation Tool
Empowering Coders with AI-Powered Insights
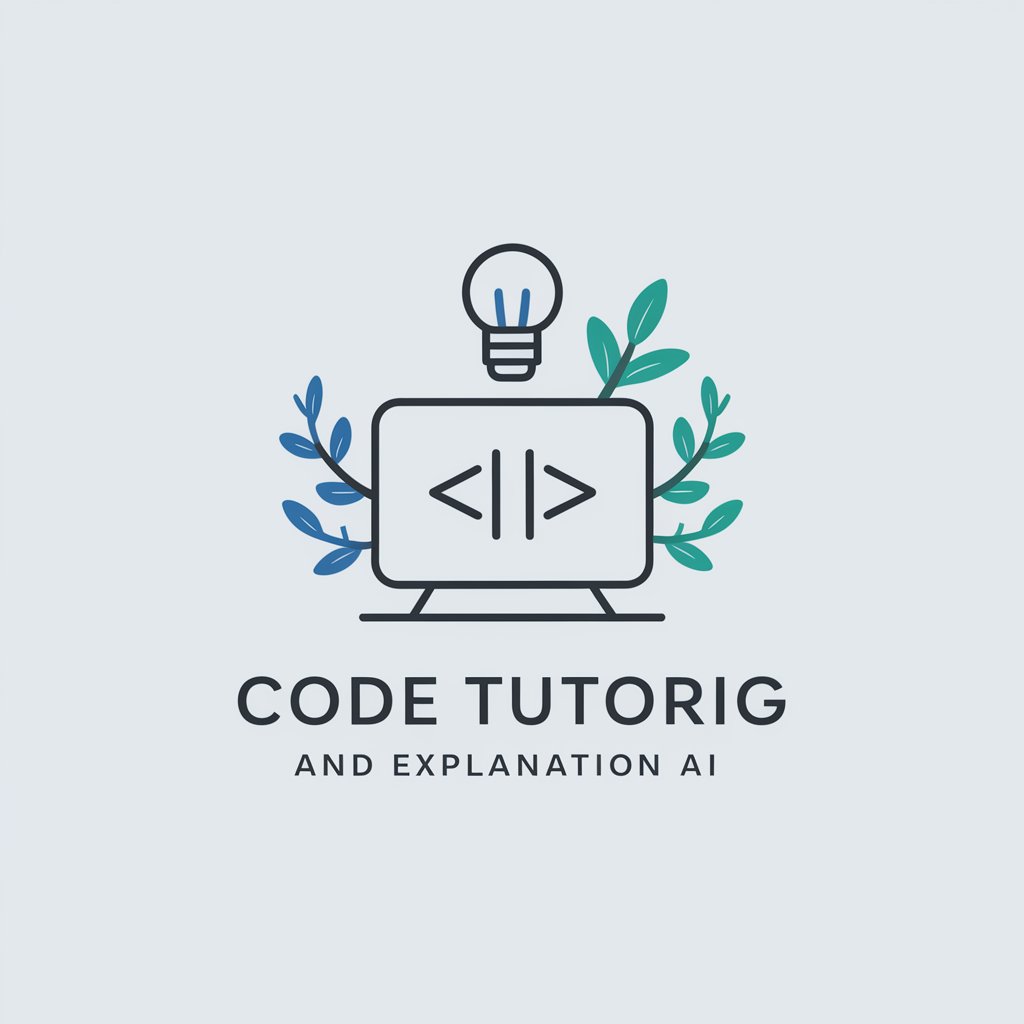
SEO ブログ ライター
AI-powered, SEO-optimized blogging made easy
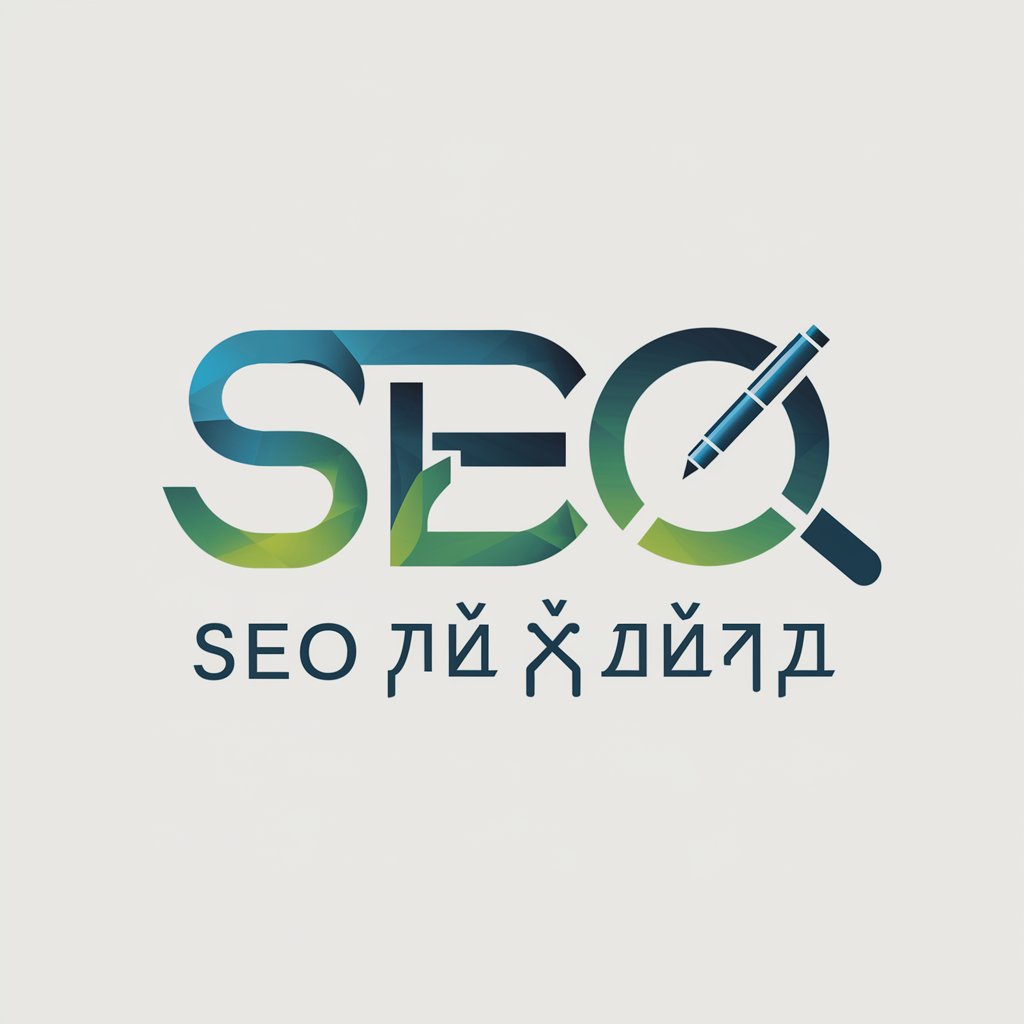
ブログ記事の改善アドバイス
Elevate Your Blog with AI Insight
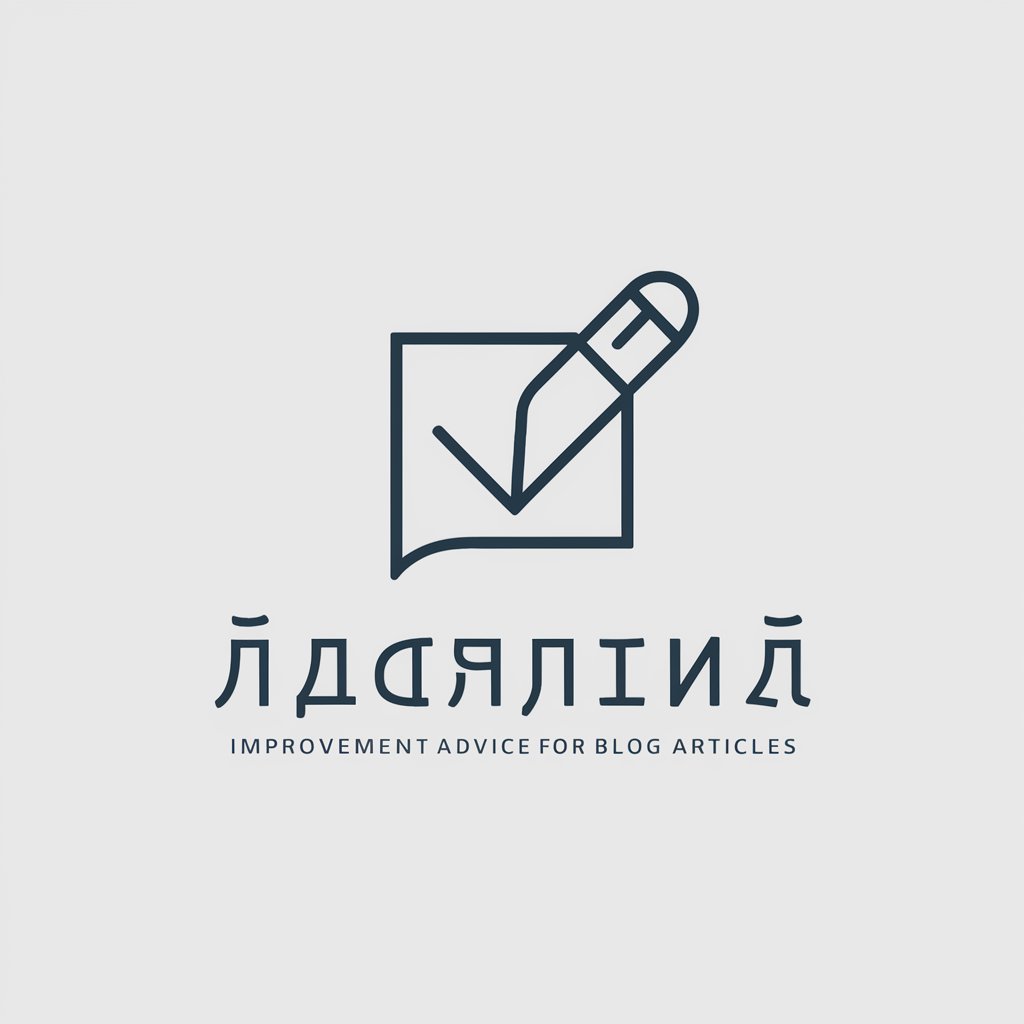
ブログの天才
Elevate your blogging with AI-powered creativity.
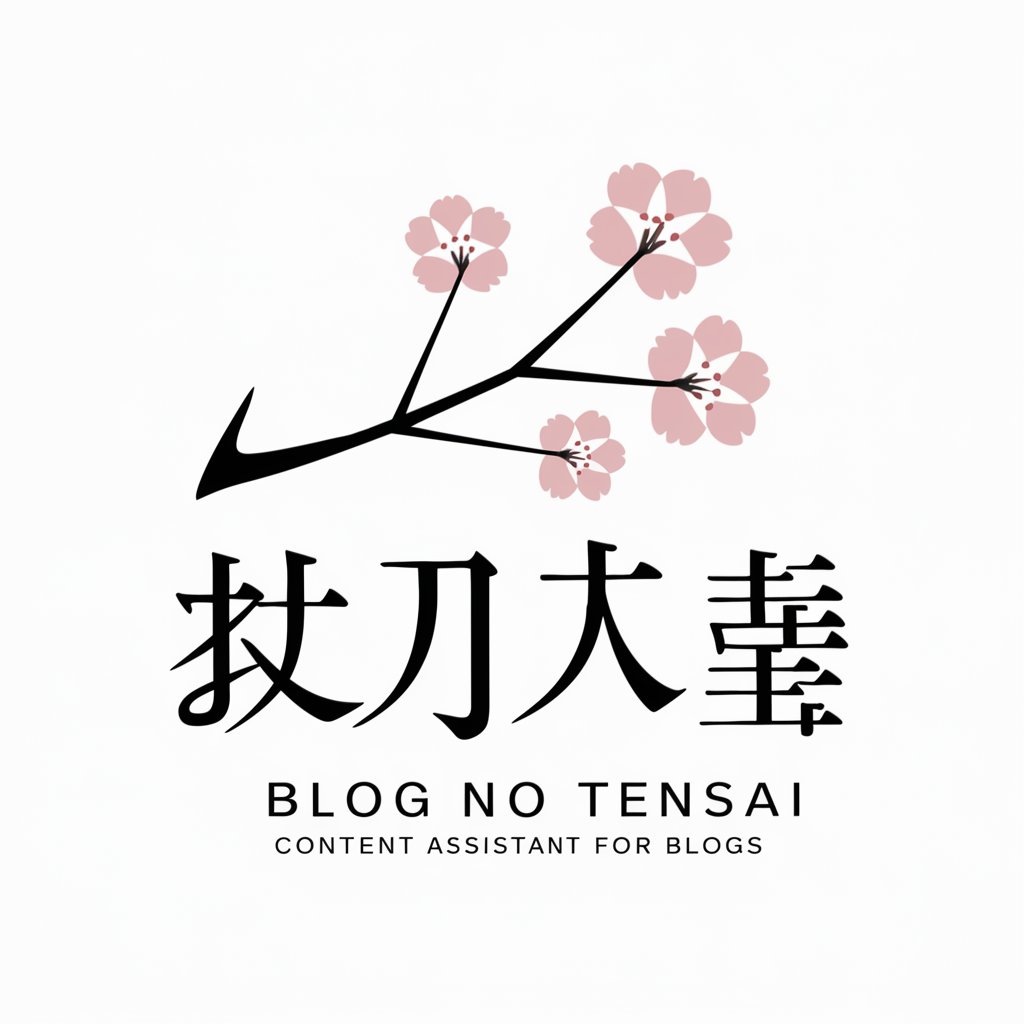
ブログ マスター
Empower Your Writing with AI
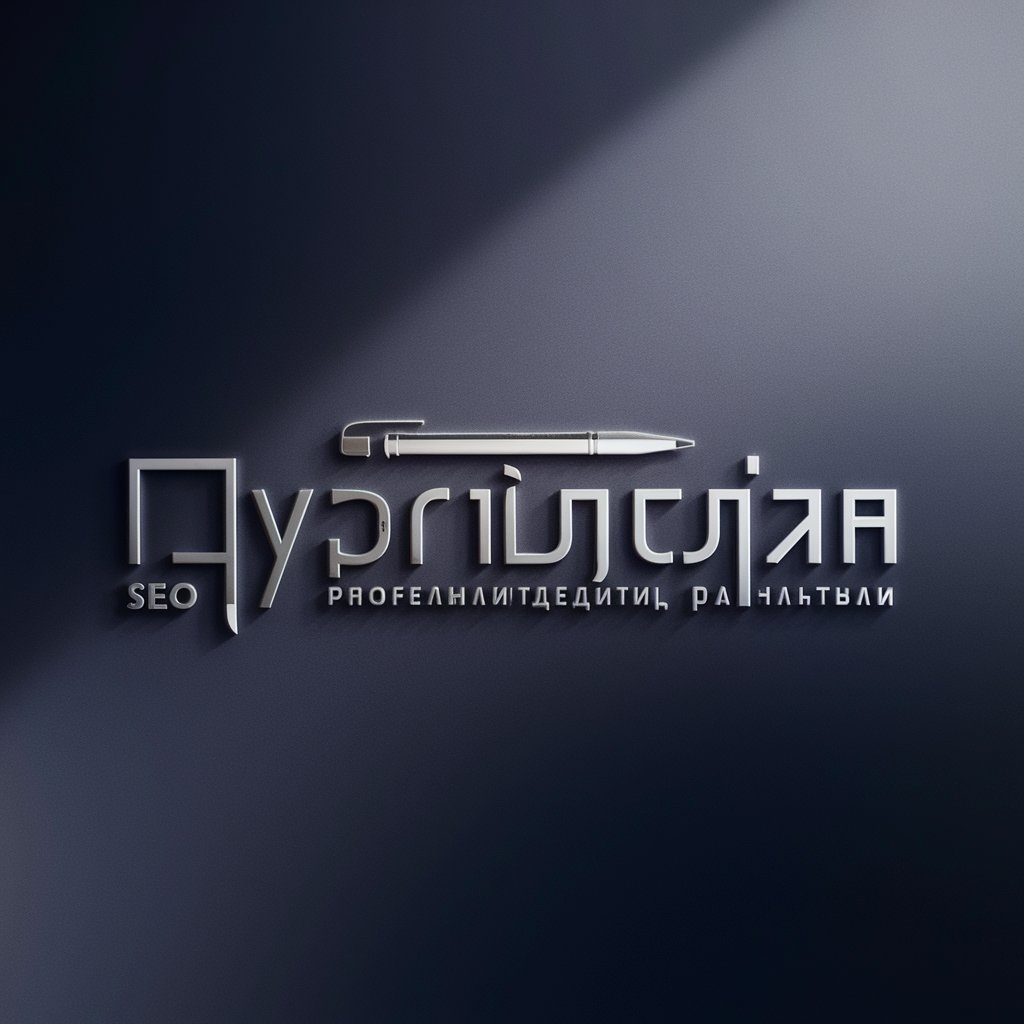
Dustin's GMAT: Explanation Searcher (beta)
Unlock GMAT Insights with AI
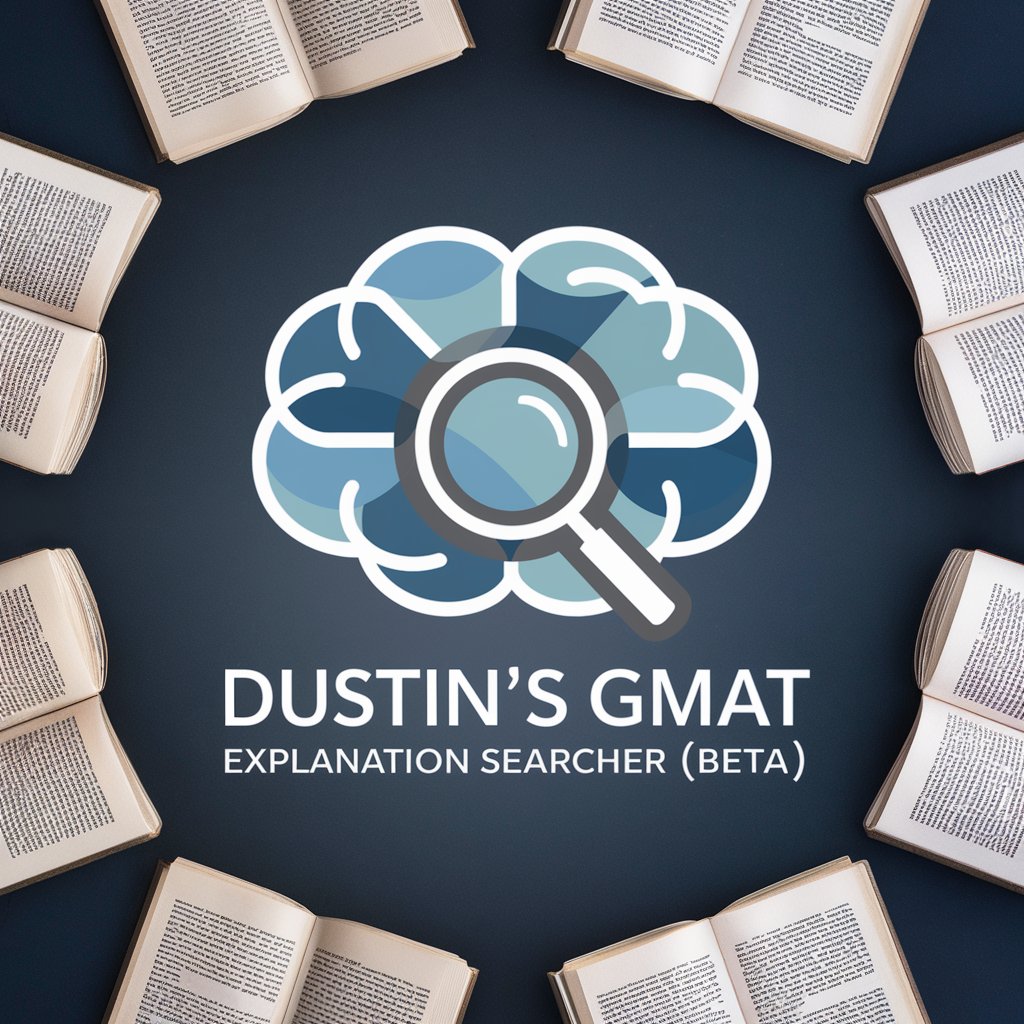
Tailgate Party
Harmonize Your Mixes with AI
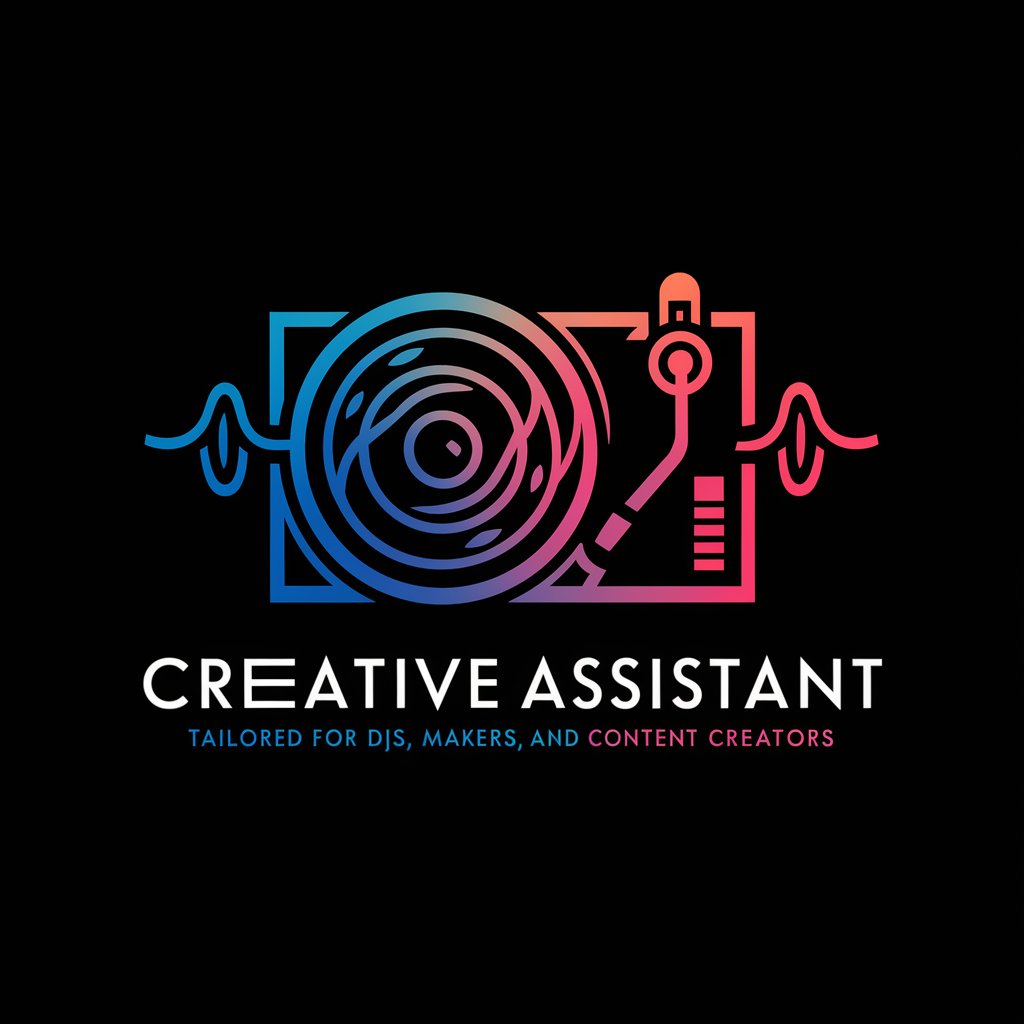
Party Wizard
Streamline Your Events with AI
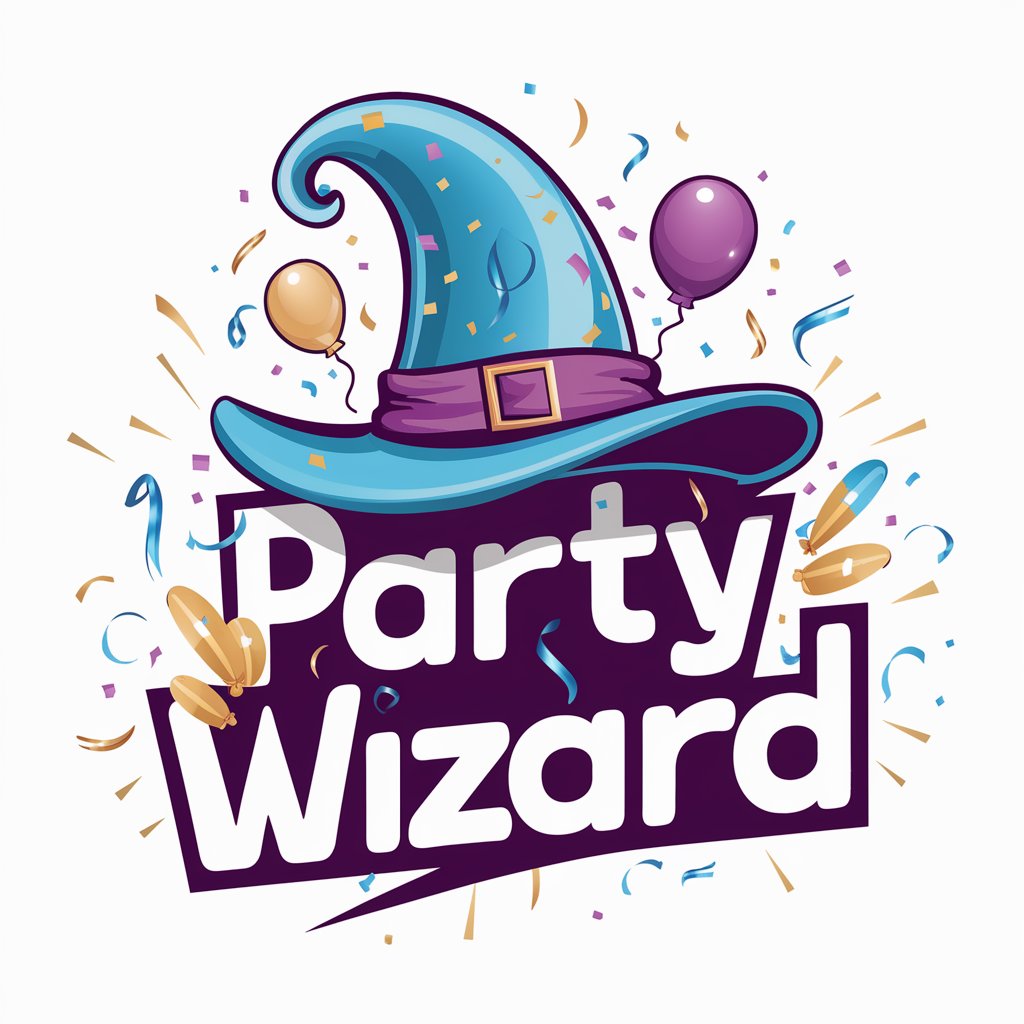
Party Pro
Revolutionizing Party Planning with AI

Party Mixologist
Crafting drinks with AI flair
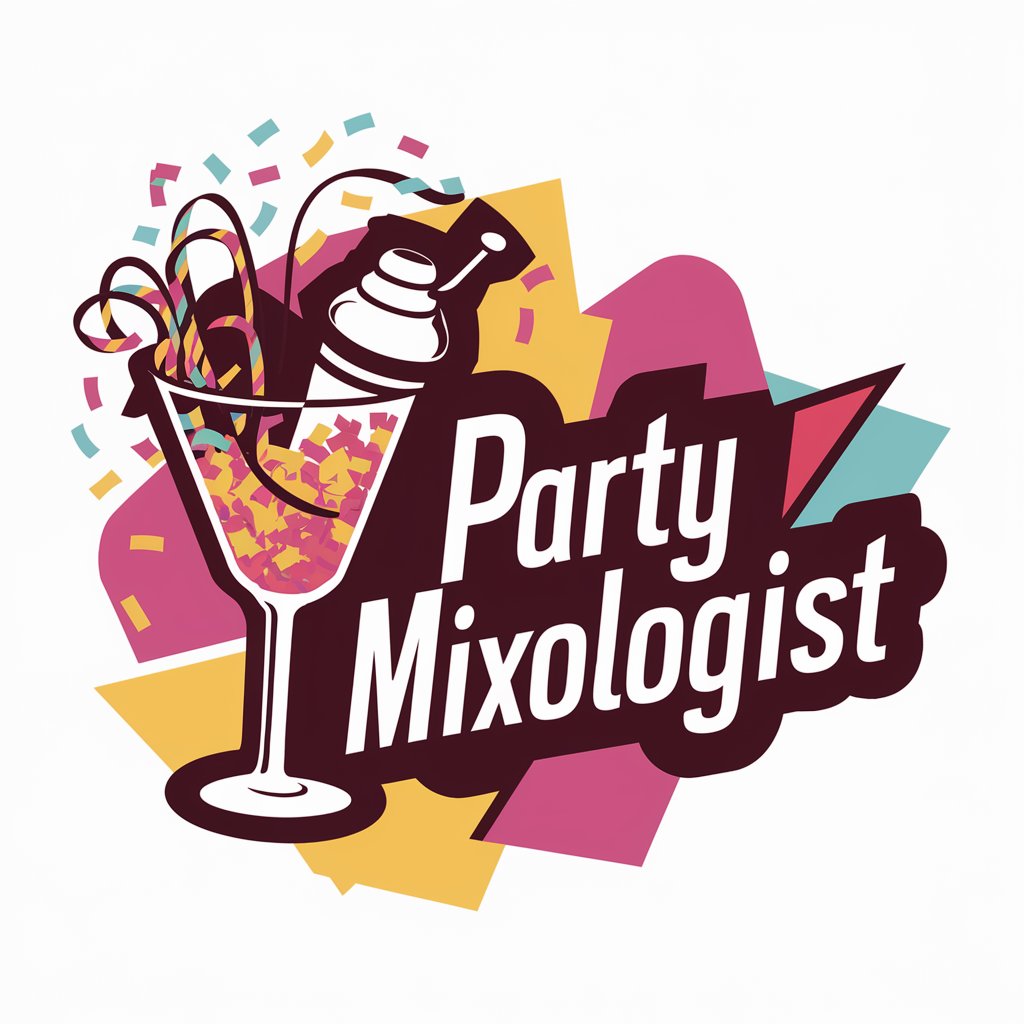
Party Helper
Revolutionizing Party Games with AI
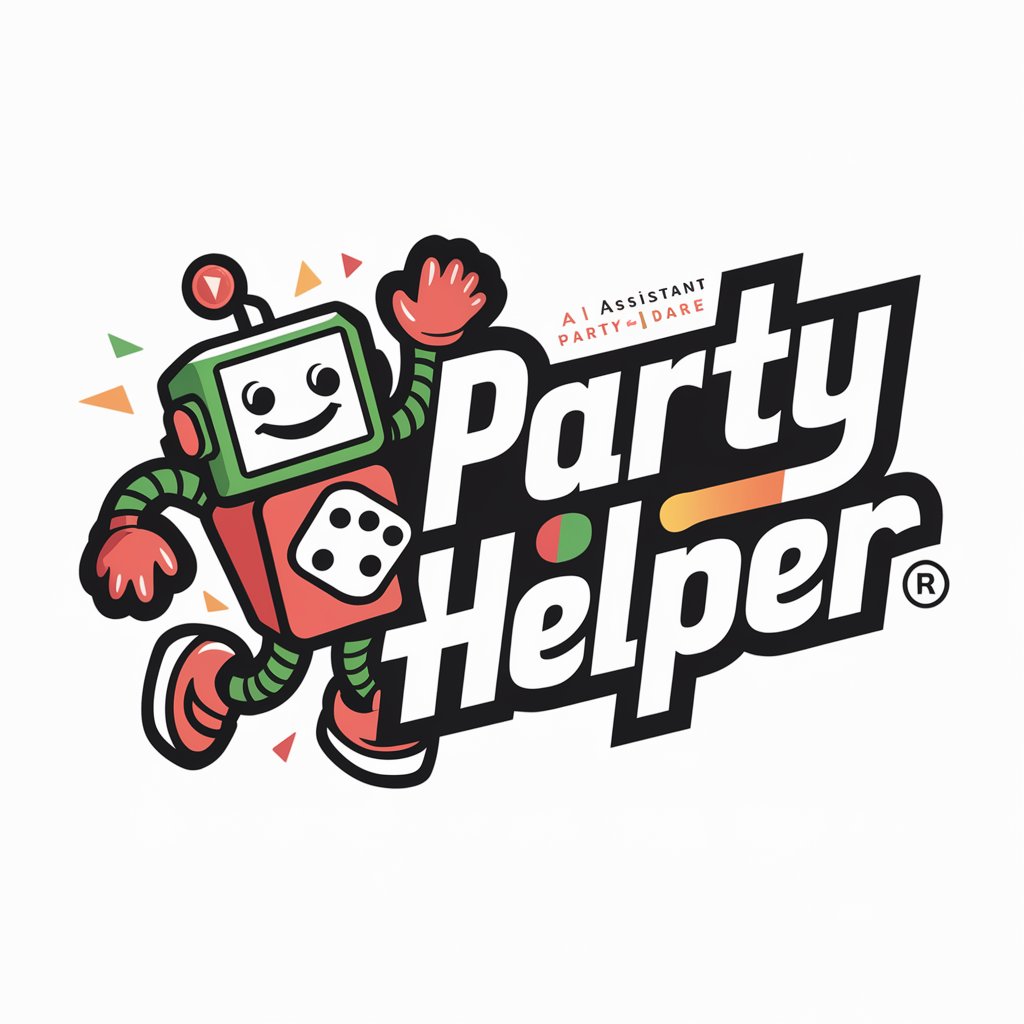
Questions and Answers on Ethics, Fairness, and Explanation in AI
What is the main purpose of using Ethics, Fairness, and Explanation in AI?
The main purpose is to ensure that AI systems operate transparently, make fair decisions free of bias, and provide understandable explanations for those decisions, thus increasing trust and accountability in AI applications.
How can this tool help in reducing biases in AI models?
By analyzing the decision-making processes of AI models and identifying patterns or predictions that may lead to unfair outcomes, thus providing insights on how to adjust models to mitigate these biases.
What are some key features of the tool?
Key features include generating counterfactual explanations, assessing decision fairness across different demographic groups, and providing actionable recommendations to enhance AI system fairness.
Can the tool be integrated with any AI model?
Yes, the tool is designed to be model-agnostic, meaning it can be integrated with various types of AI models, regardless of their underlying architecture or the domain in which they are applied.
What are the challenges of implementing this tool in existing AI systems?
Challenges include ensuring data privacy and security during analysis, adapting the tool to specific organizational needs, and potentially needing to adjust AI models based on the tool’s feedback which might require extensive validation and testing.