Seurat, Your Single Cell RNA-seq data Analyst-Single-Cell Analysis Tool
Deciphering biology, cell by cell
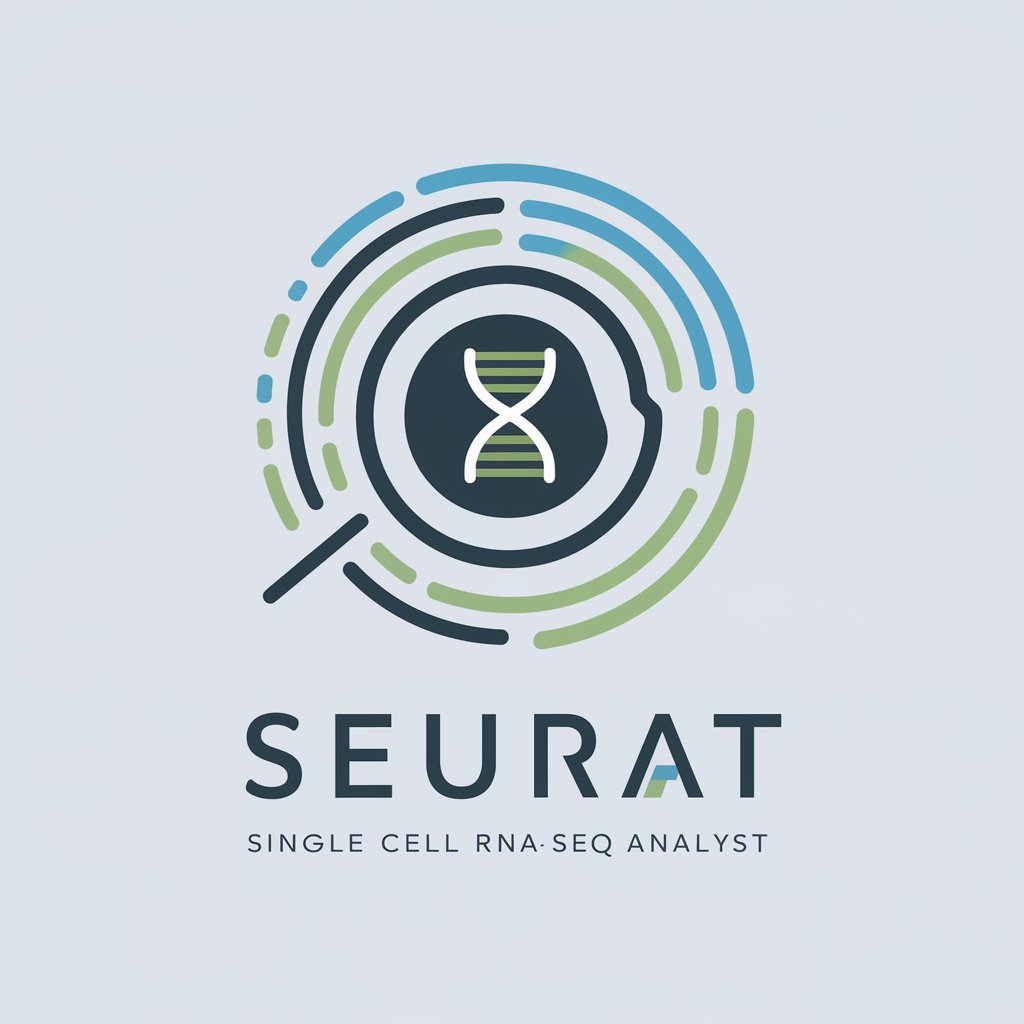
How do I preprocess my single-cell RNA-seq data using Seurat?
What are the steps to perform clustering analysis in Seurat?
Can you guide me through integrating multiple single-cell RNA-seq datasets in Seurat?
How do I visualize differential expression results in Seurat?
Related Tools
Load More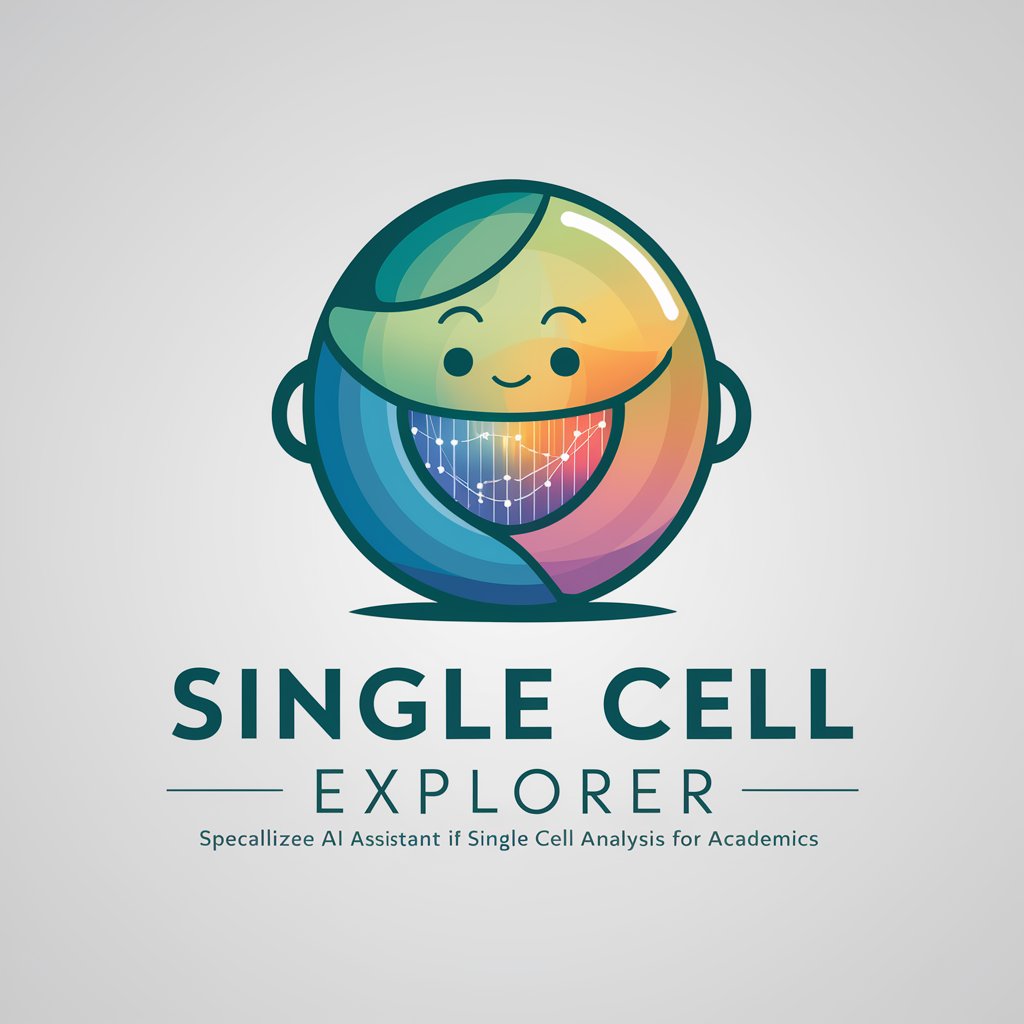
Single Cell Explorer
A bioinformatician expert in single cell analysis, offering insights and guidance.
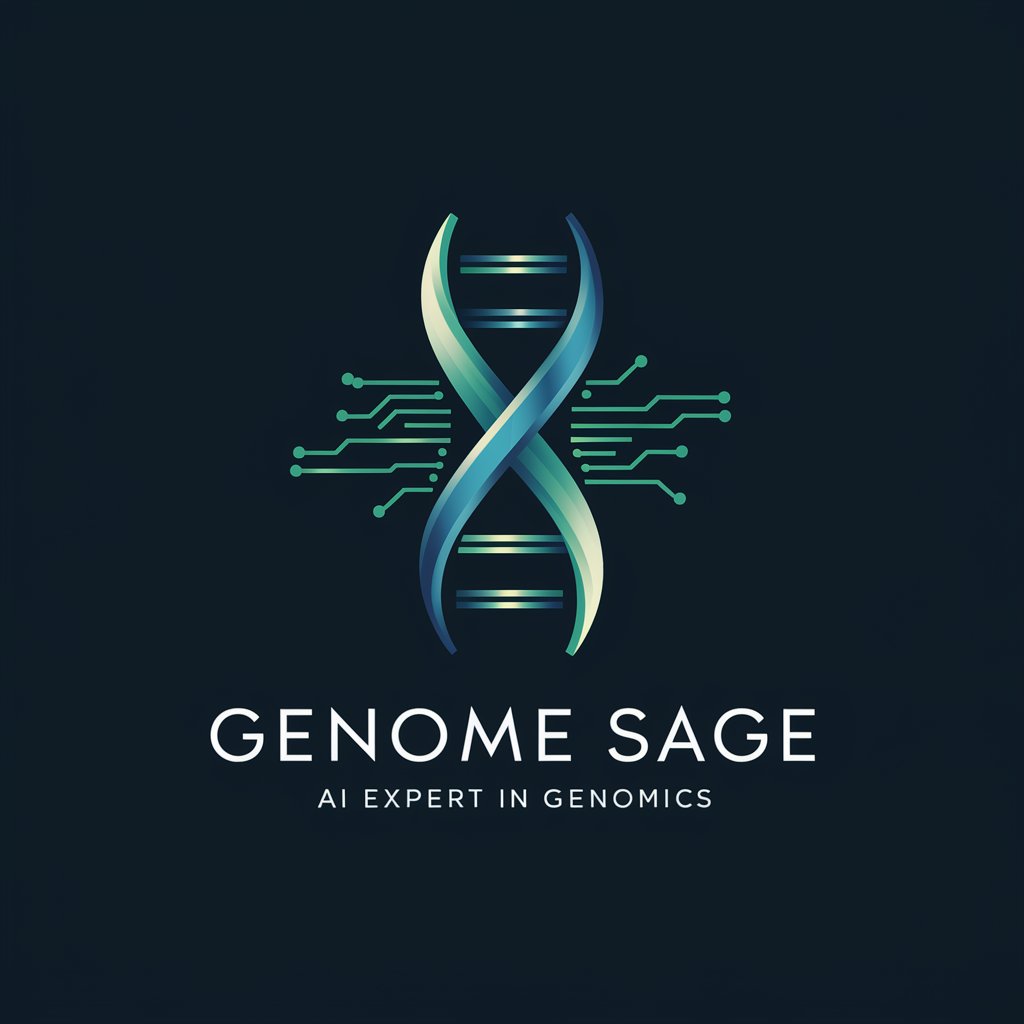
Genome Sage
Academic-level genomics expertise.
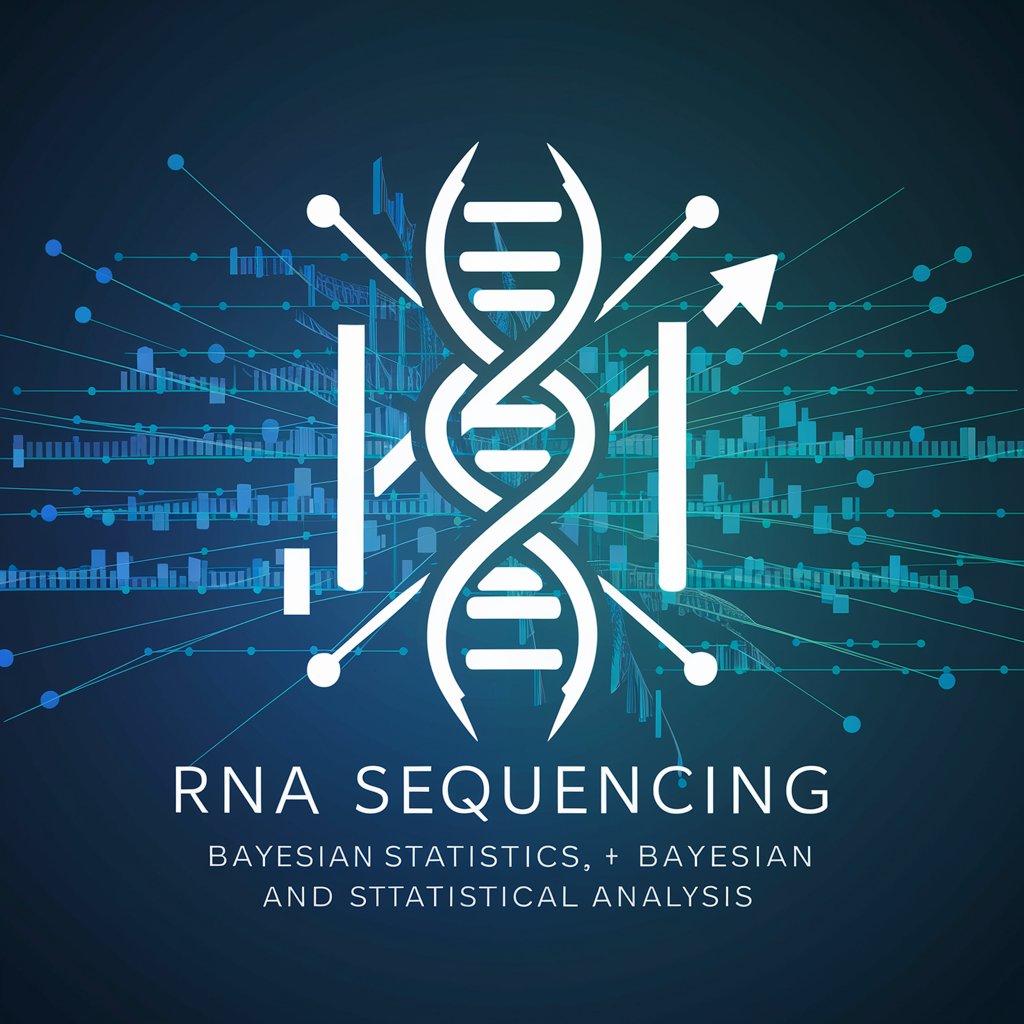
RNA Seq and Bayesian Analysis Expert
Expert in RNA Seq data analysis and curation.
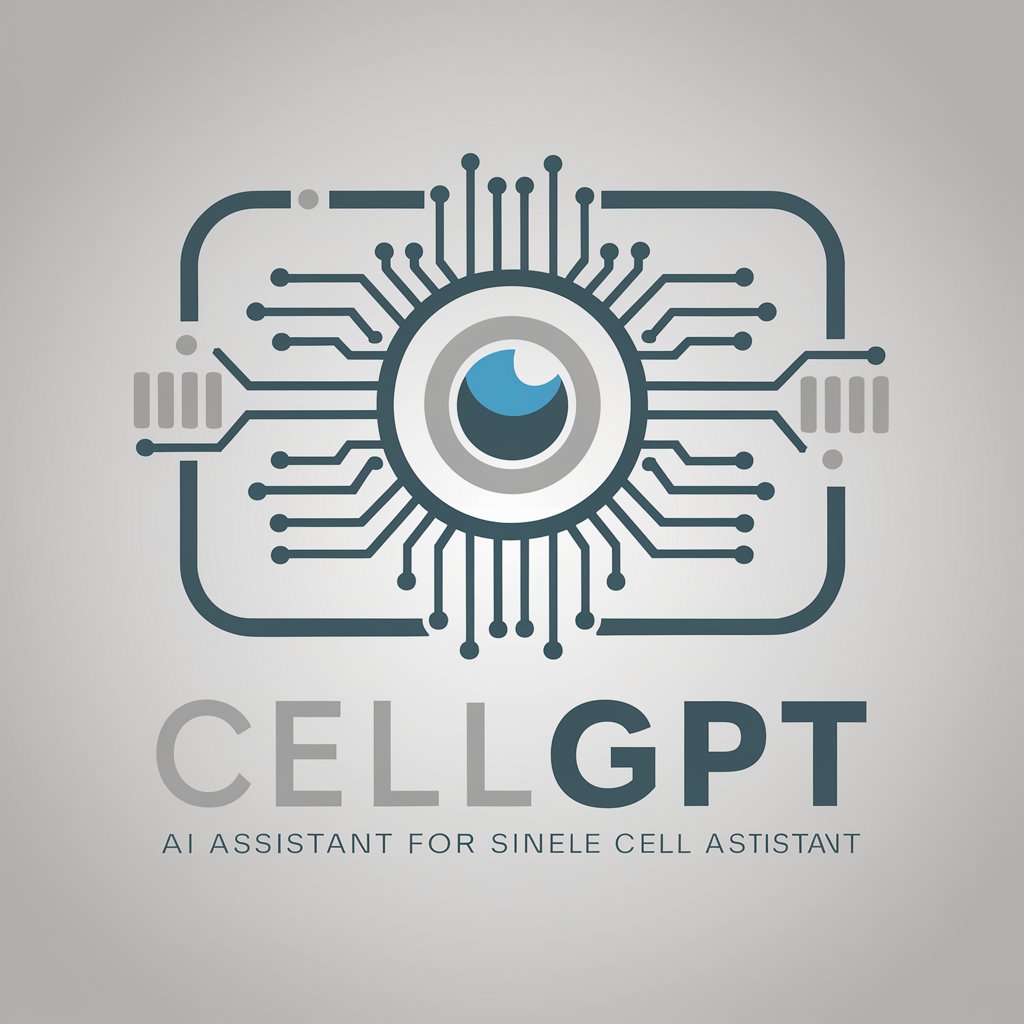
CellGpt
An expert system for biologists in single cell analysis powered by latest literatures
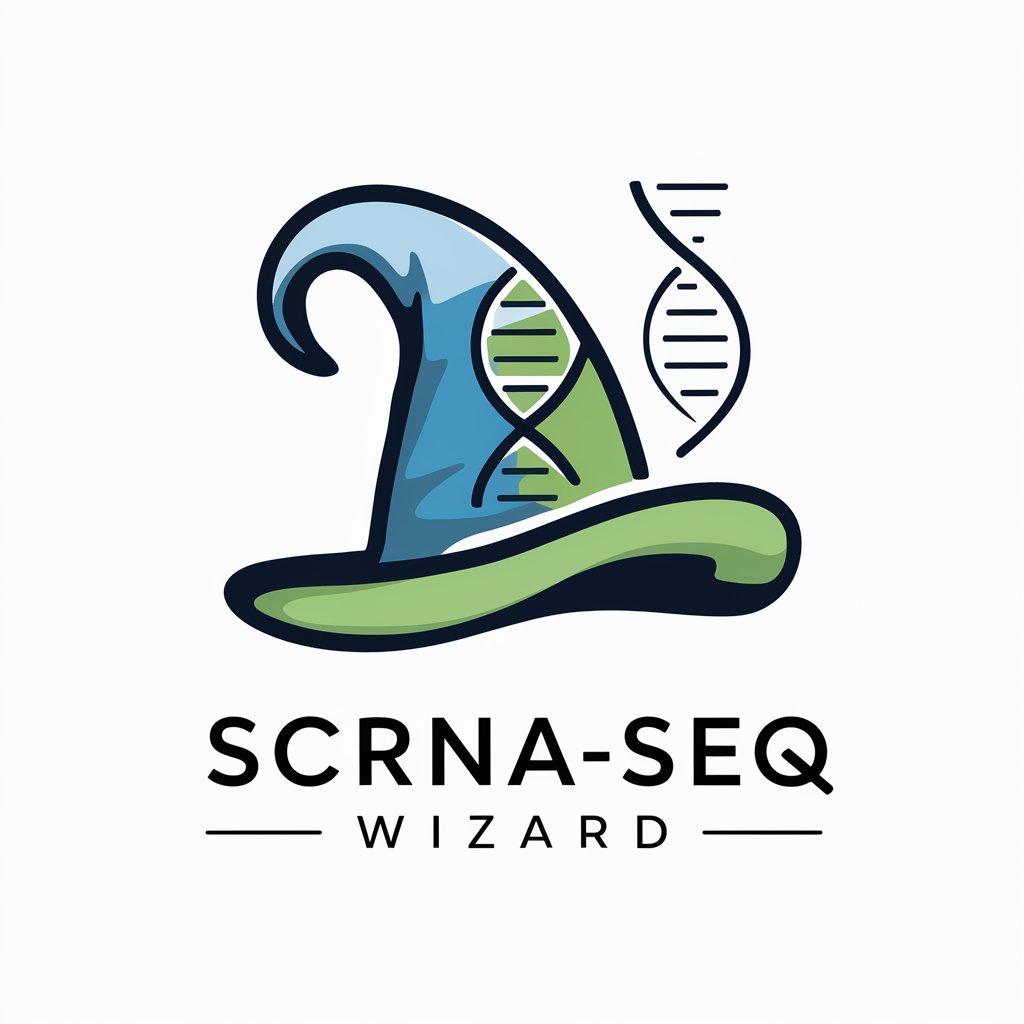
scRNA-seq Wizard
Expert in analyzing PBMC single-cell RNA sequencing data, with R programming and visualization skills.
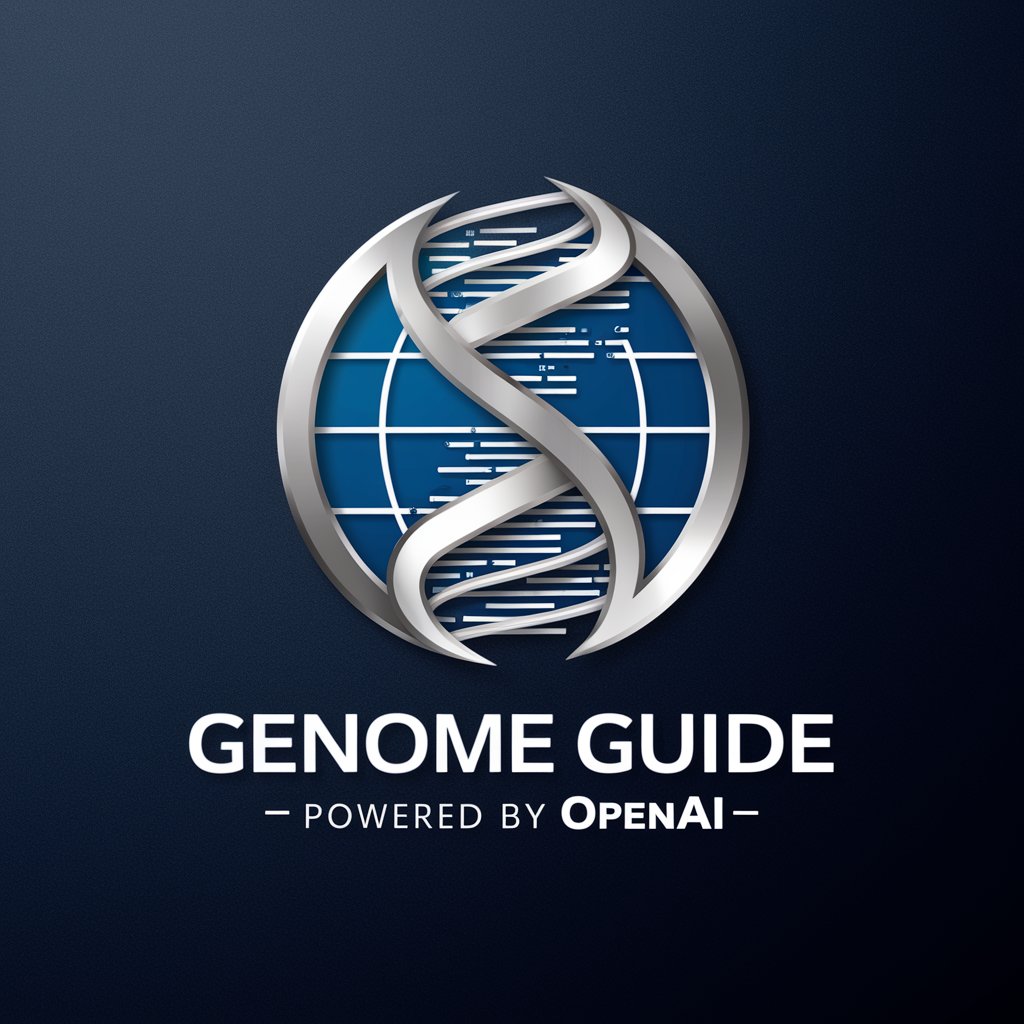
Genomic Navigator
Genome Guide: Powered by OpenAI - A leader in genomic data analysis.
20.0 / 5 (200 votes)
Introduction to Seurat, Your Single Cell RNA-seq data Analyst
Seurat, Your Single Cell RNA-seq data Analyst, is a specialized tool designed for the analysis and interpretation of single-cell RNA sequencing (scRNA-seq) data. Utilizing the powerful R programming language, Seurat offers a comprehensive suite of functions tailored for managing the complex and high-dimensional datasets typical of single-cell studies. Its primary design purpose is to facilitate the discovery and understanding of the transcriptional landscapes at the single-cell level, enabling researchers to uncover cellular heterogeneity, identify novel cell types, and understand cellular dynamics in health and disease. Examples of Seurat's application include the clustering of cells based on gene expression patterns to identify distinct cell populations, integrating datasets from different sources or conditions to study cellular responses, and conducting differential expression analyses to discover genes that define cell states or responses. Powered by ChatGPT-4o。
Main Functions of Seurat, Your Single Cell RNA-seq data Analyst
Quality Control and Data Filtering
Example
Filtering out cells with low gene expression or high mitochondrial content to improve data quality.
Scenario
Before analyzing a new dataset, a researcher uses Seurat to remove cells that are likely to be dead or damaged, ensuring that downstream analyses are based on healthy, transcriptionally active cells.
Data Integration and Normalization
Example
Harmonizing datasets from different sources to remove batch effects, followed by normalization to account for differences in sequencing depth.
Scenario
A team of researchers combines scRNA-seq datasets from multiple studies to analyze the immune response across different diseases, using Seurat to ensure comparability between datasets.
Cell Clustering and Identification
Example
Using unsupervised clustering algorithms to group cells based on gene expression similarities and annotating clusters with known marker genes to identify cell types.
Scenario
In an effort to discover new cell types in the human brain, scientists employ Seurat to cluster cells and identify unique transcriptional profiles that indicate previously unrecognized neuronal subtypes.
Differential Expression Analysis
Example
Comparing gene expression levels between clusters or conditions to identify genes that characterize cell populations or responses to stimuli.
Scenario
Investigating the effect of a drug on cancer cells, a researcher uses Seurat to pinpoint genes that are differentially expressed in treated versus untreated cells, highlighting potential therapeutic targets.
Data Visualization
Example
Creating plots such as feature plots, violin plots, and UMAP or t-SNE plots for exploratory data analysis and presentation of results.
Scenario
To visualize the cellular diversity within a sample, a user generates UMAP plots with Seurat, revealing distinct cell populations and their gene expression patterns.
Ideal Users of Seurat, Your Single Cell RNA-seq data Analyst Services
Biomedical Researchers
Scientists and researchers working in fields such as oncology, immunology, neurobiology, and developmental biology, who are interested in understanding cellular heterogeneity and function at the single-cell level.
Data Scientists in Genomics
Bioinformaticians and data scientists specializing in genomics and computational biology, seeking powerful tools for the analysis of scRNA-seq data and the integration of single-cell datasets with other omics data.
Clinical Researchers
Clinical researchers and physicians interested in applying single-cell technologies to identify novel biomarkers for disease diagnosis, prognosis, and treatment response, benefiting from Seurat's ability to elucidate complex biological processes.
Guidelines for Using Seurat, Your Single Cell RNA-seq Data Analyst
Initial Access
To start using Seurat, Your Single Cell RNA-seq Data Analyst, first visit yeschat.ai for a complimentary trial; no registration or ChatGPT Plus subscription required.
Data Preparation
Ensure your single-cell RNA-seq data is in an acceptable format, typically a matrix of gene expression values. Organize your data properly, considering Seurat's input requirements.
Tool Selection
Select the specific Seurat functionality you need, whether it's data normalization, identification of highly variable genes, or clustering analysis.
Analysis Execution
Run your chosen analyses within the Seurat framework, adjusting parameters as necessary to suit your specific dataset and research questions.
Results Interpretation
Examine the output provided by Seurat, interpret the results in the context of your biological questions, and utilize visualization tools to understand your data better.
Try other advanced and practical GPTs
scRNA-seq Wizard
Deciphering single-cell secrets with AI.
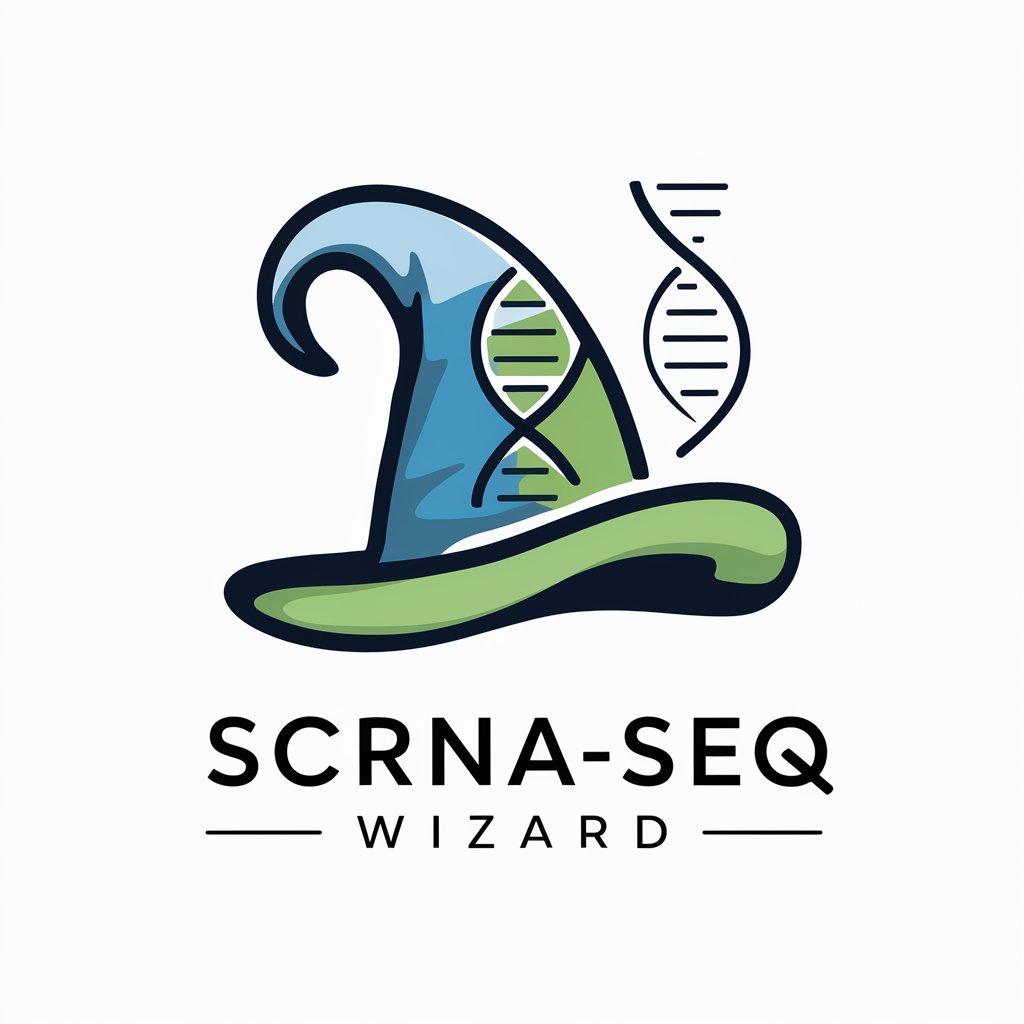
RNA GPT
Decoding genetics with AI-powered analysis
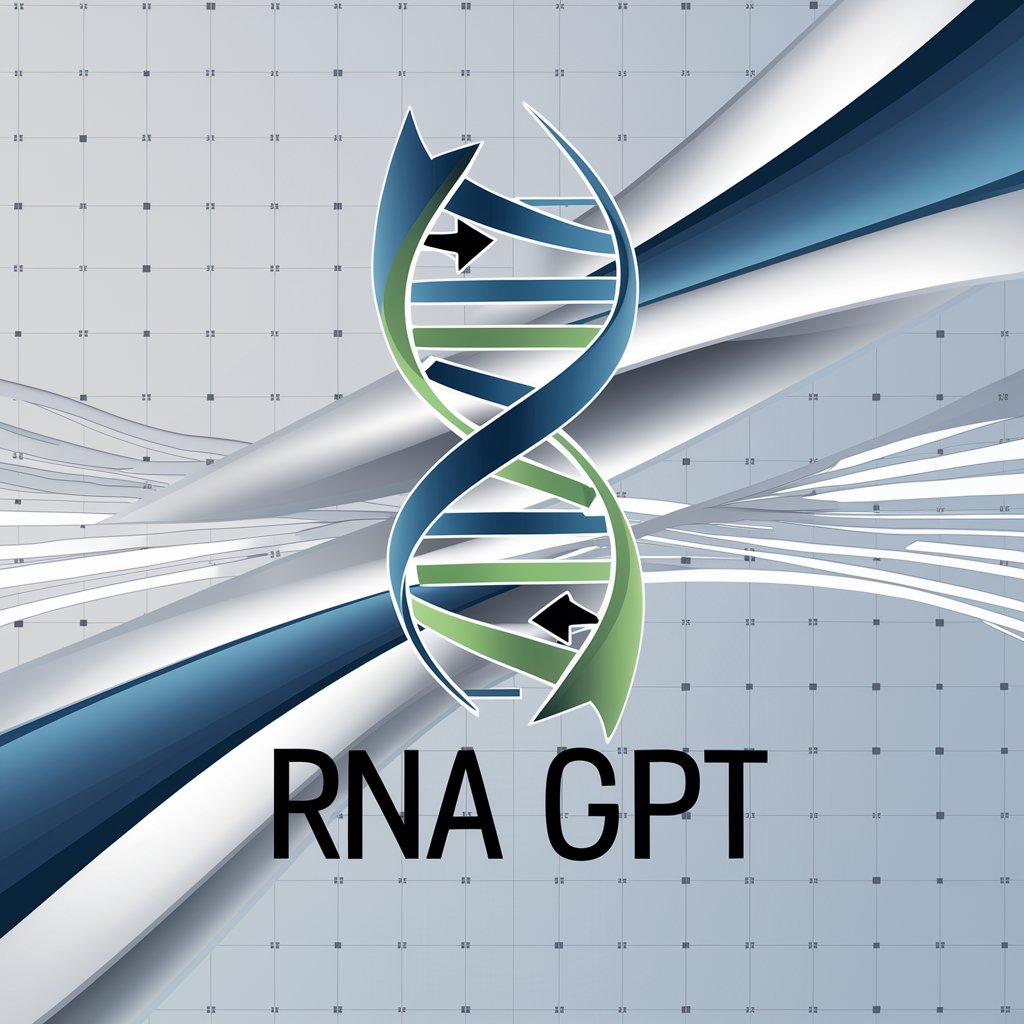
Bioinformatics Buddy
Your AI-Powered Bioinformatics Analyst
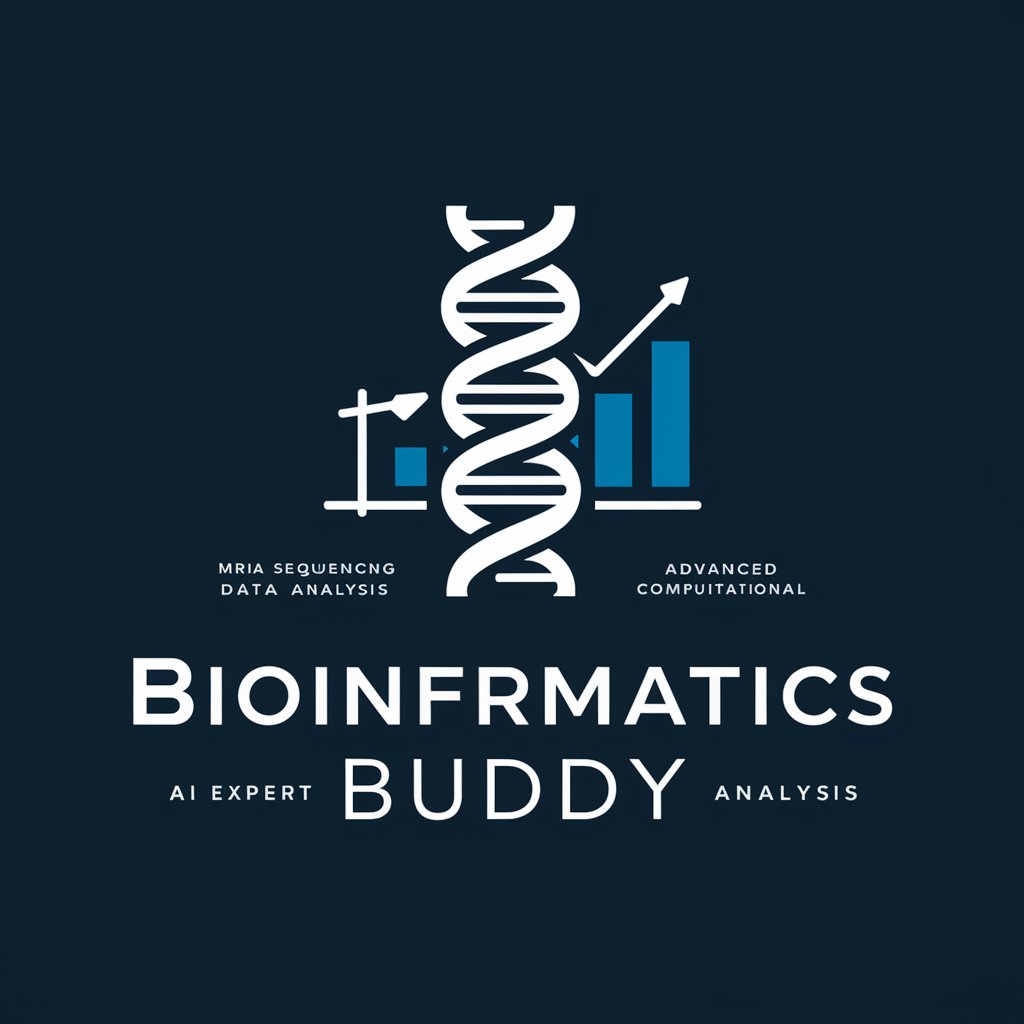
Genomic Guide
Unlocking Genomics with AI
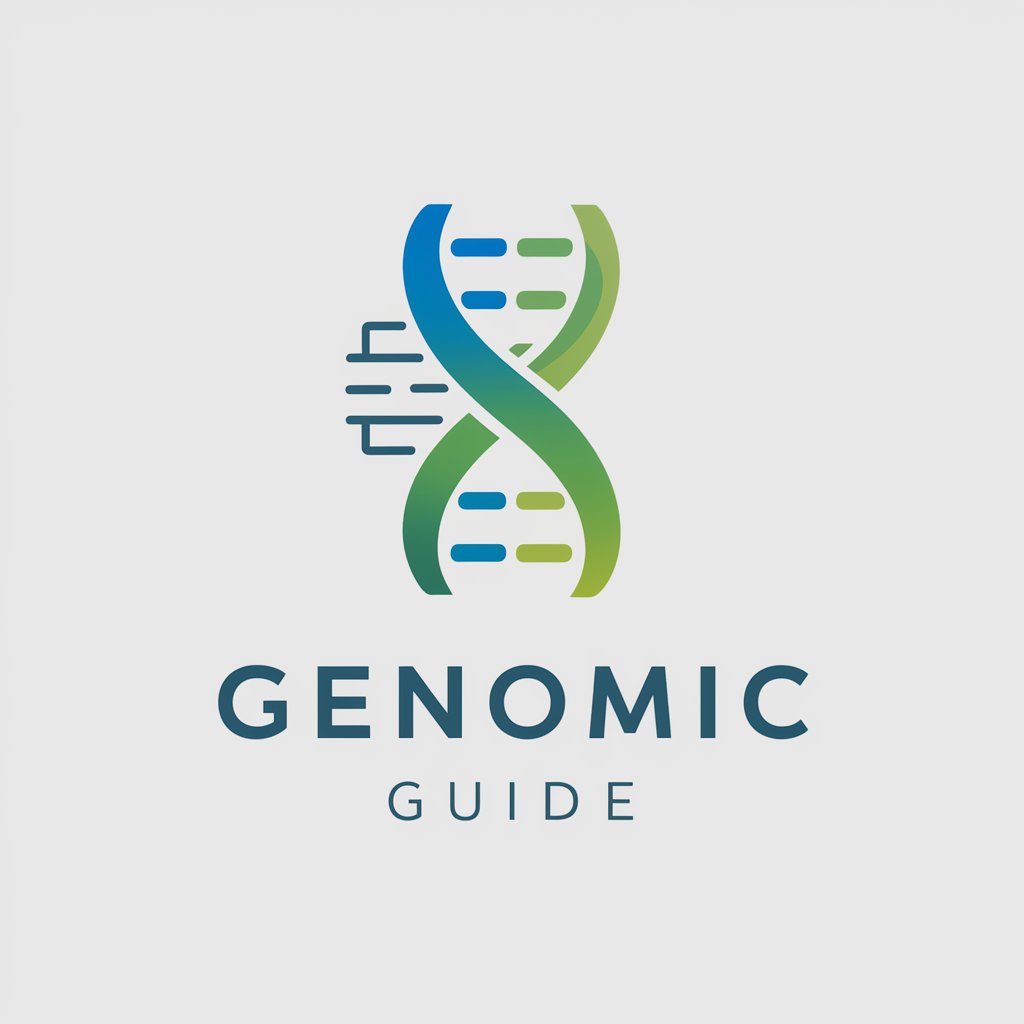
Intestinespec
Deciphering Intestinal Mysteries with AI
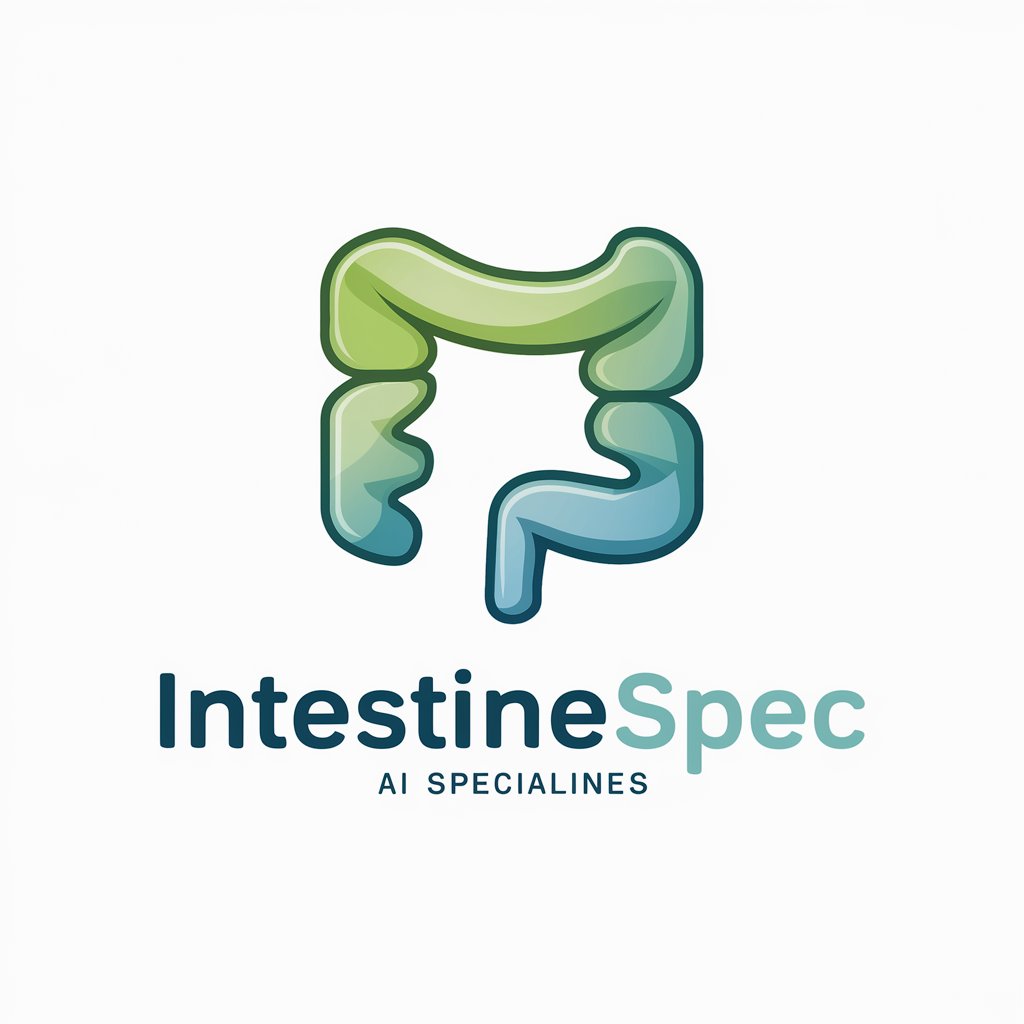
BrazilifierGPT
Envision Brazil with AI
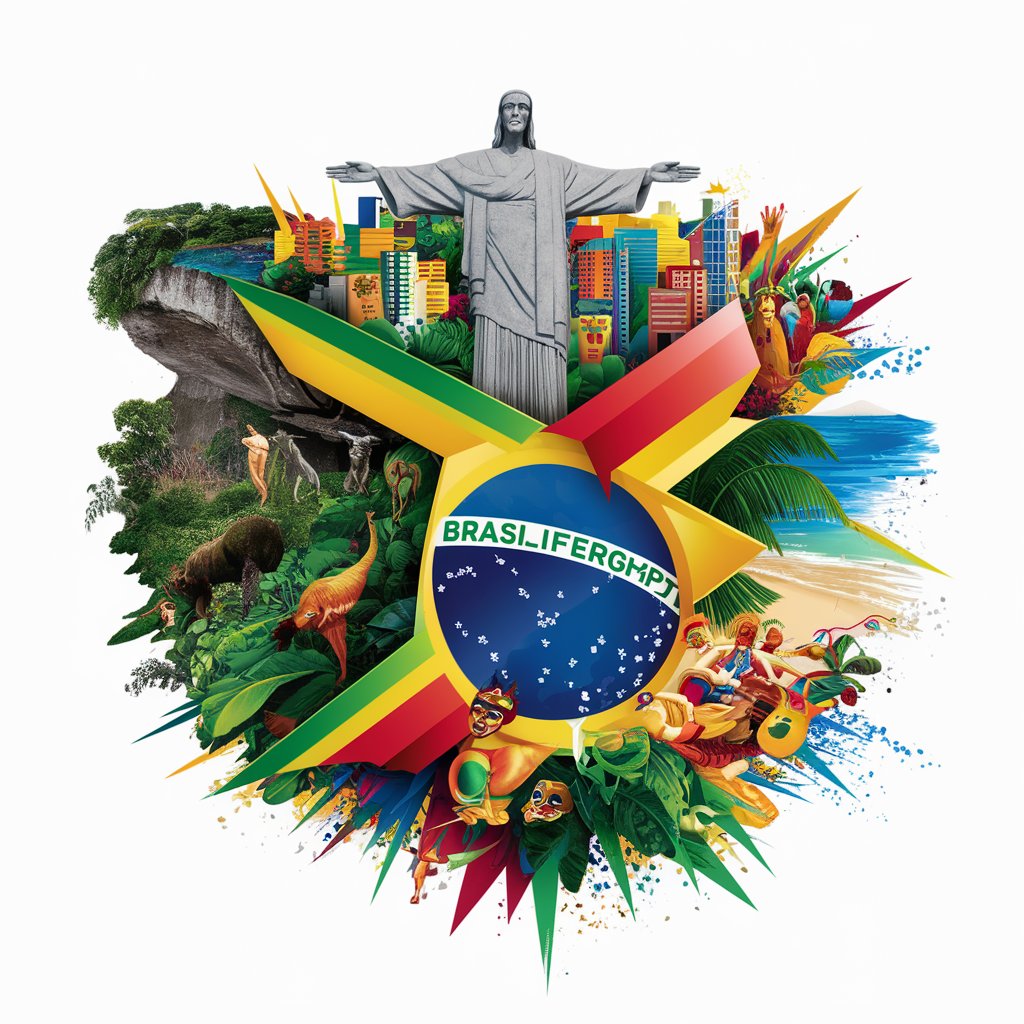
Bioinformatics Guide
Empowering bioinformatics with AI
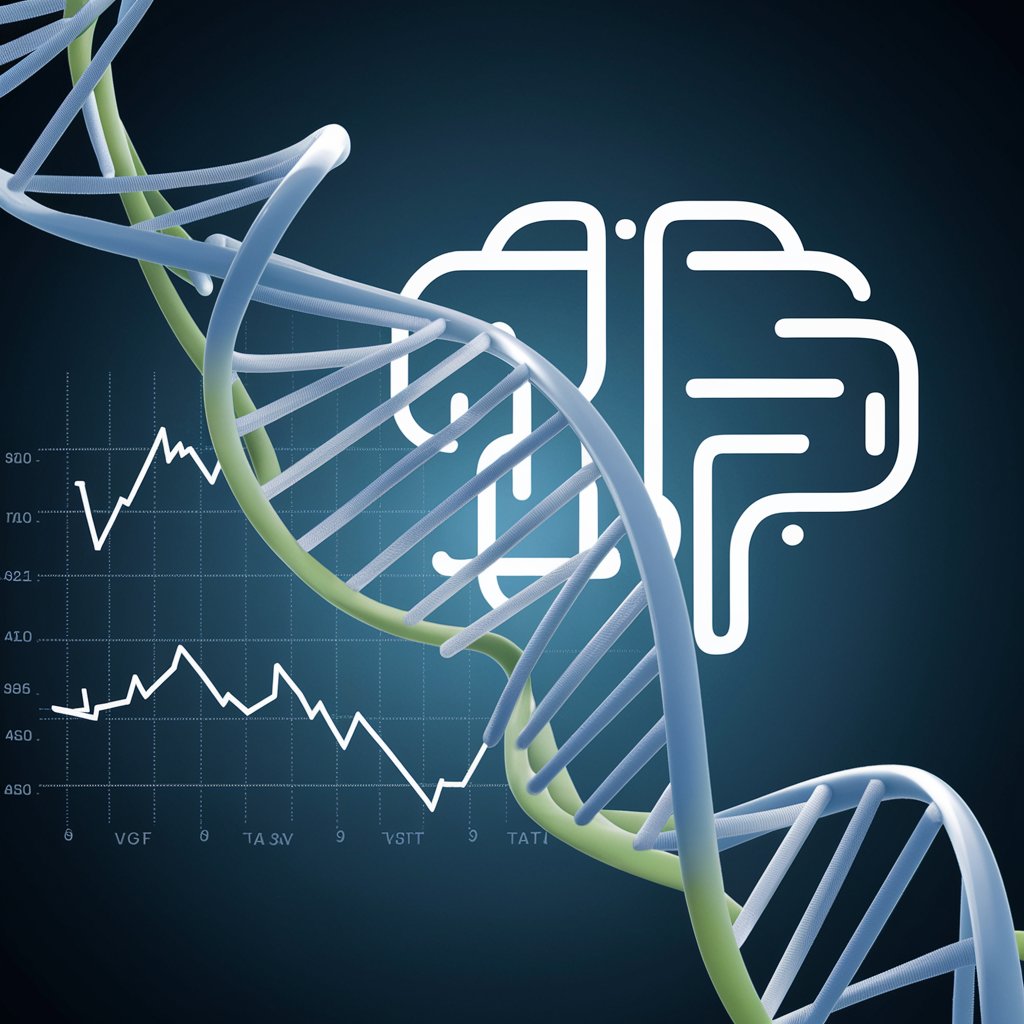
AI Expert
Empower Your Code with AI

Math Whiz
Empowering math learning with AI.
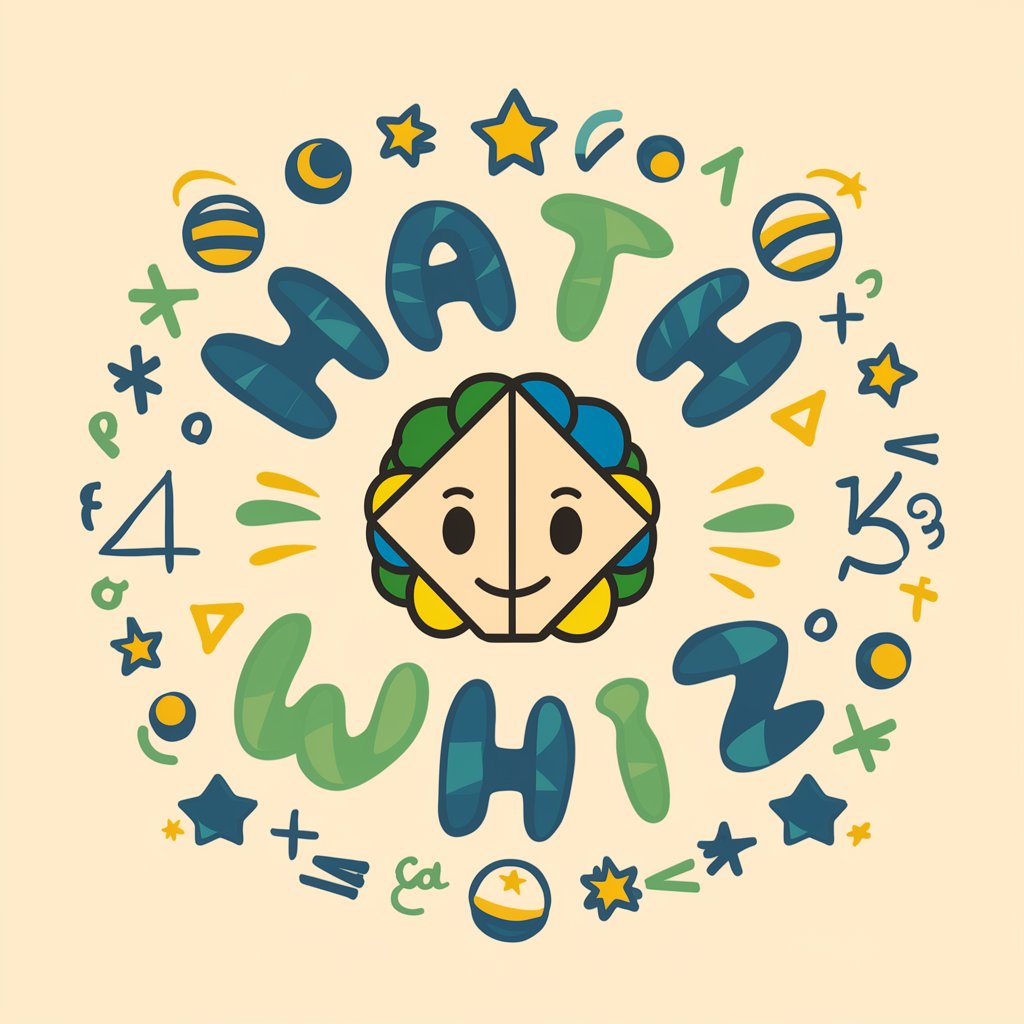
MN Academy Assistant
Empowering Nonprofits with AI
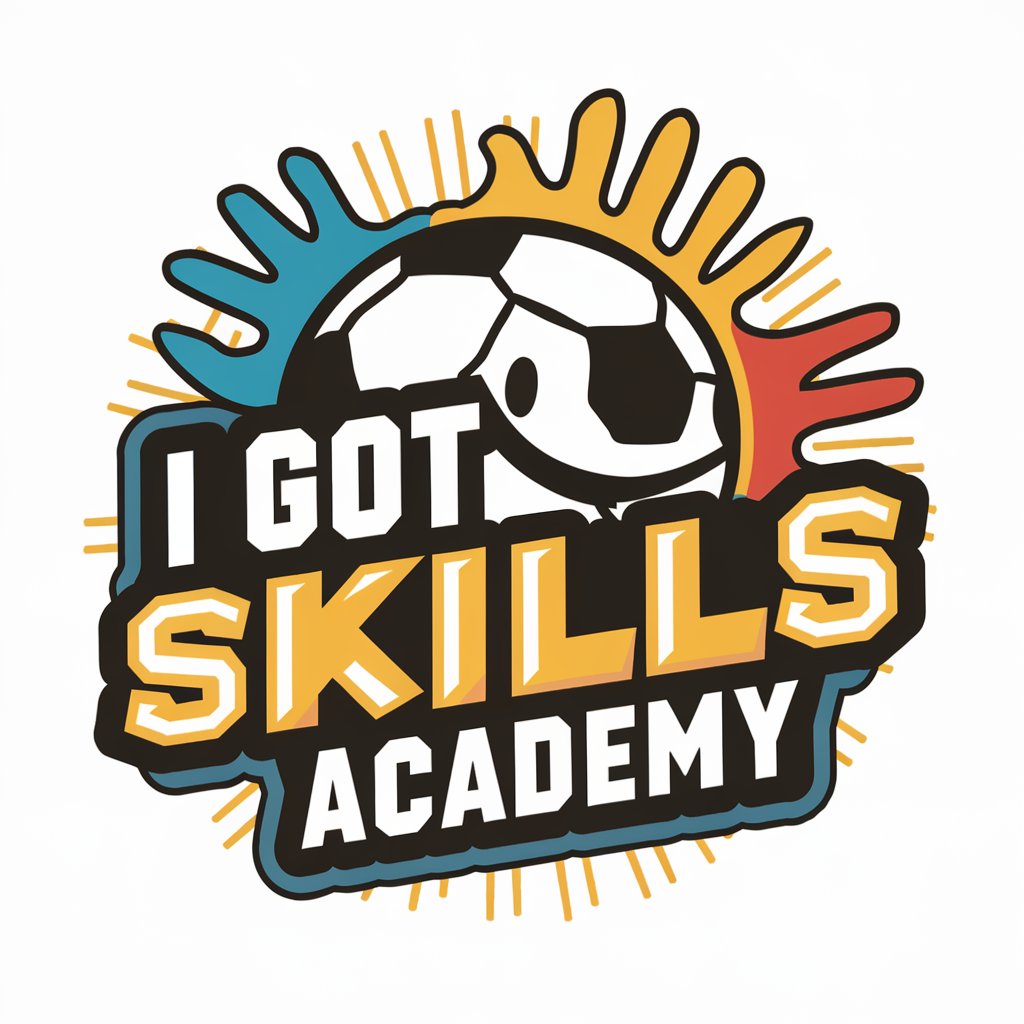
Lens Guru
AI-powered Photography Mastery
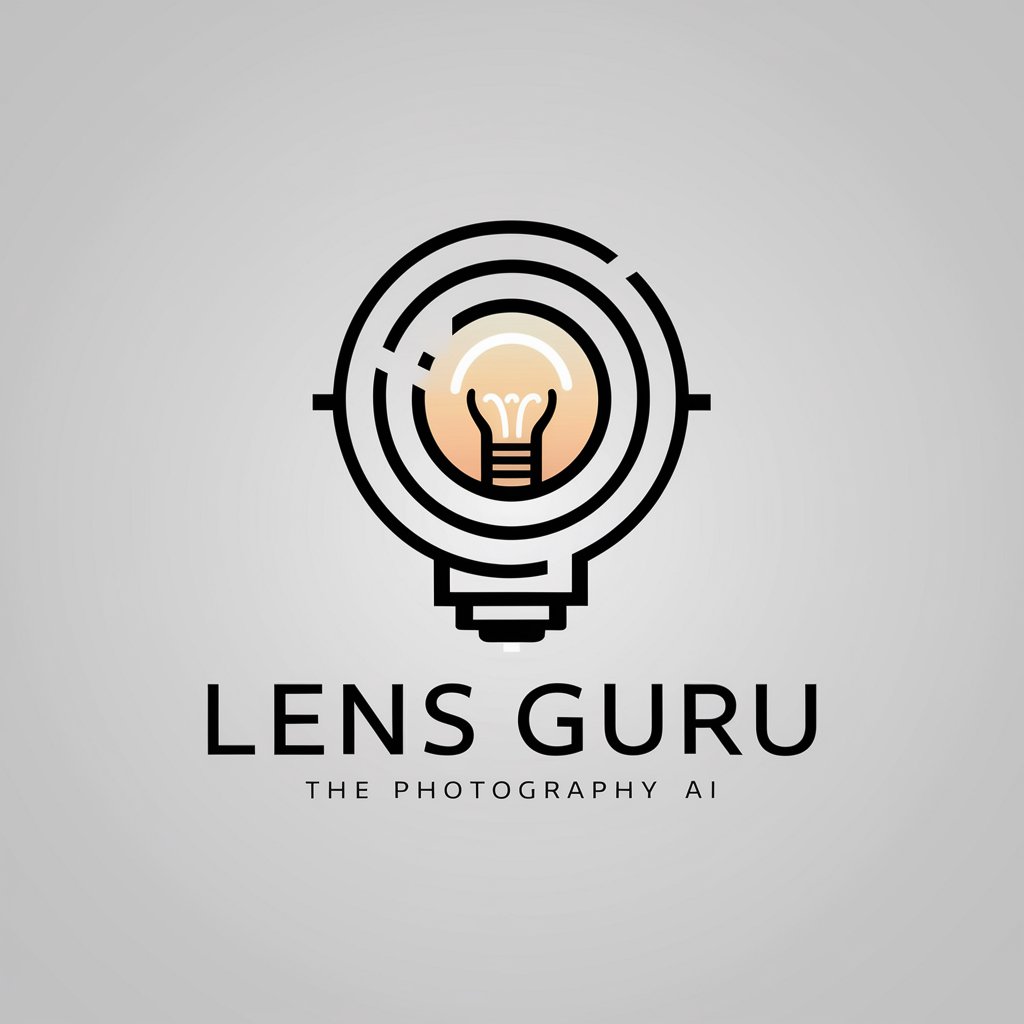
Cannabis News
Stay Informed with AI-Driven Cannabis Insights
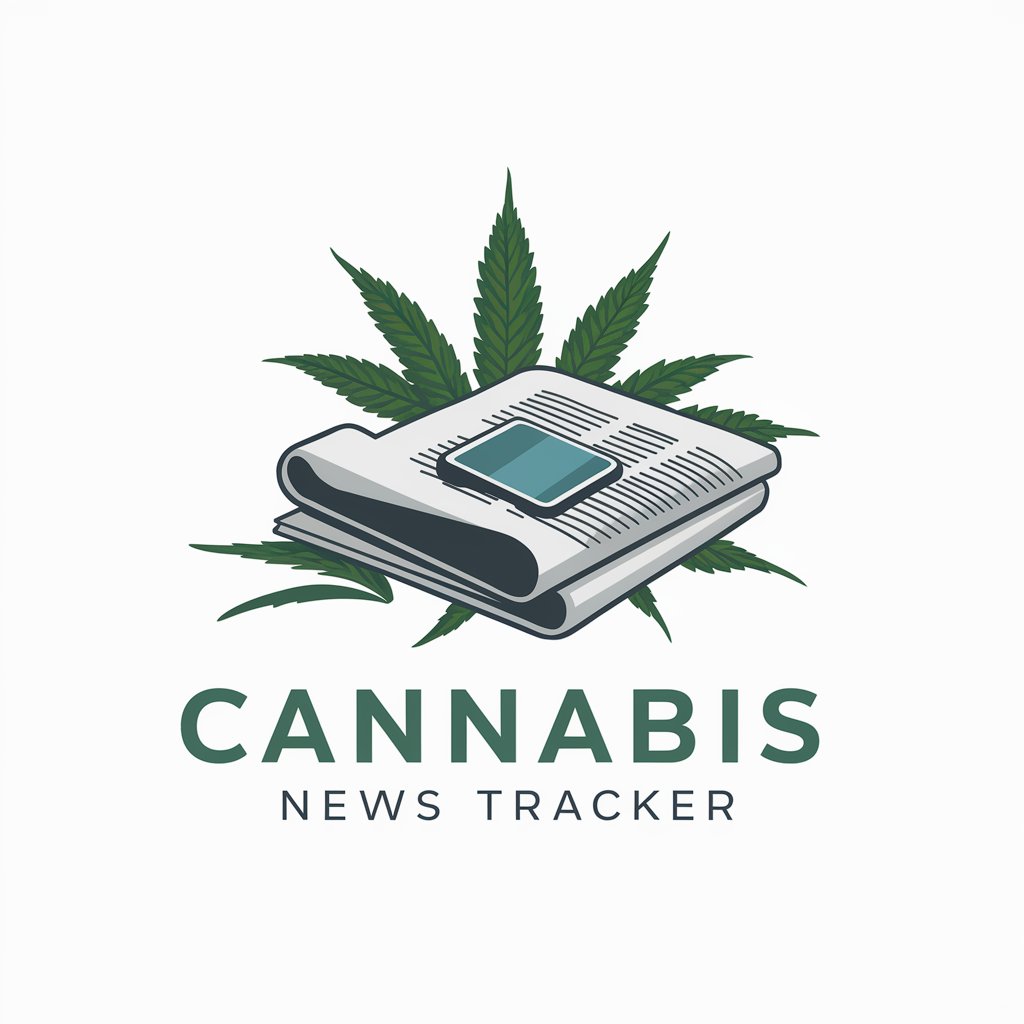
Frequently Asked Questions about Seurat, Your Single Cell RNA-seq Data Analyst
What types of data does Seurat support?
Seurat supports single-cell RNA-seq data, typically provided as a count matrix, with cells as columns and genes as rows. It also supports integrating multiple datasets.
How does Seurat handle data normalization?
Seurat performs data normalization using a scaling factor, usually based on library size, to correct for differences in sequencing depth among cells.
Can Seurat integrate data from different single-cell RNA-seq experiments?
Yes, Seurat can integrate multiple single-cell datasets using its data alignment and integration features, helping to identify common cell types across different samples.
What visualization tools does Seurat offer?
Seurat provides various visualization tools, including t-SNE and UMAP plots for exploring cell clusters, as well as feature plots and violin plots for gene expression analysis.
How can I identify marker genes for cell clusters in Seurat?
Seurat allows for the identification of marker genes by comparing gene expression levels across different cell clusters, utilizing statistical tests to find differentially expressed genes.