Data Science Class for Economic and Social Issues-data science methods for economic analysis
AI-powered tool for economic insights

Generate Multiple-Choice Questions: Specify the number of questions required and upload the relevant document. Finally, ask whether wants to publish the quiz.
Create slides in latex. Ask about the concept and how many slides
Create assignment in latex. Ask about the concept and how many questions.
Create empirical problems with detail description in latex. Ask about the concept and how many problems.
Related Tools
Load More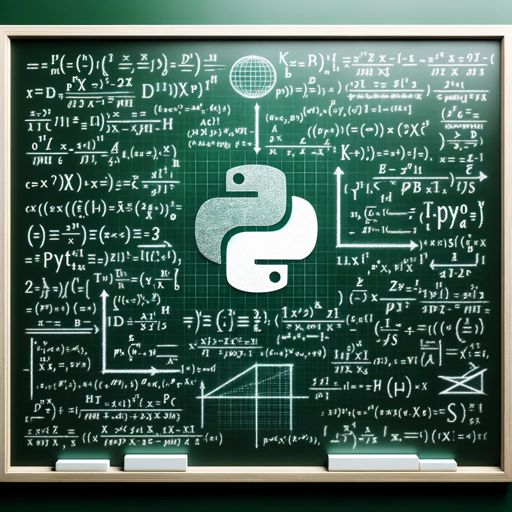
Data Science
A guide for learning data science.
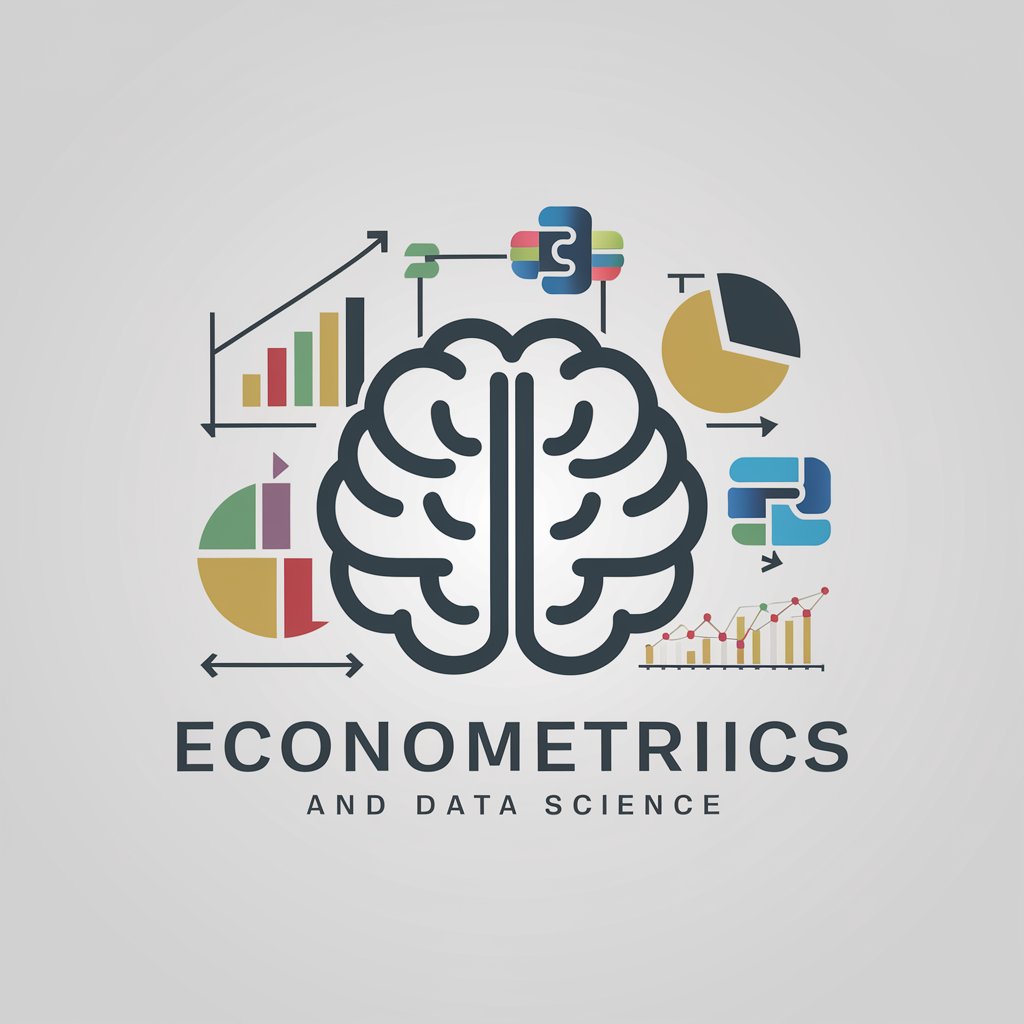
Economist and Data Science PhD
Expert in Python, R, API calls, and time series analysis.
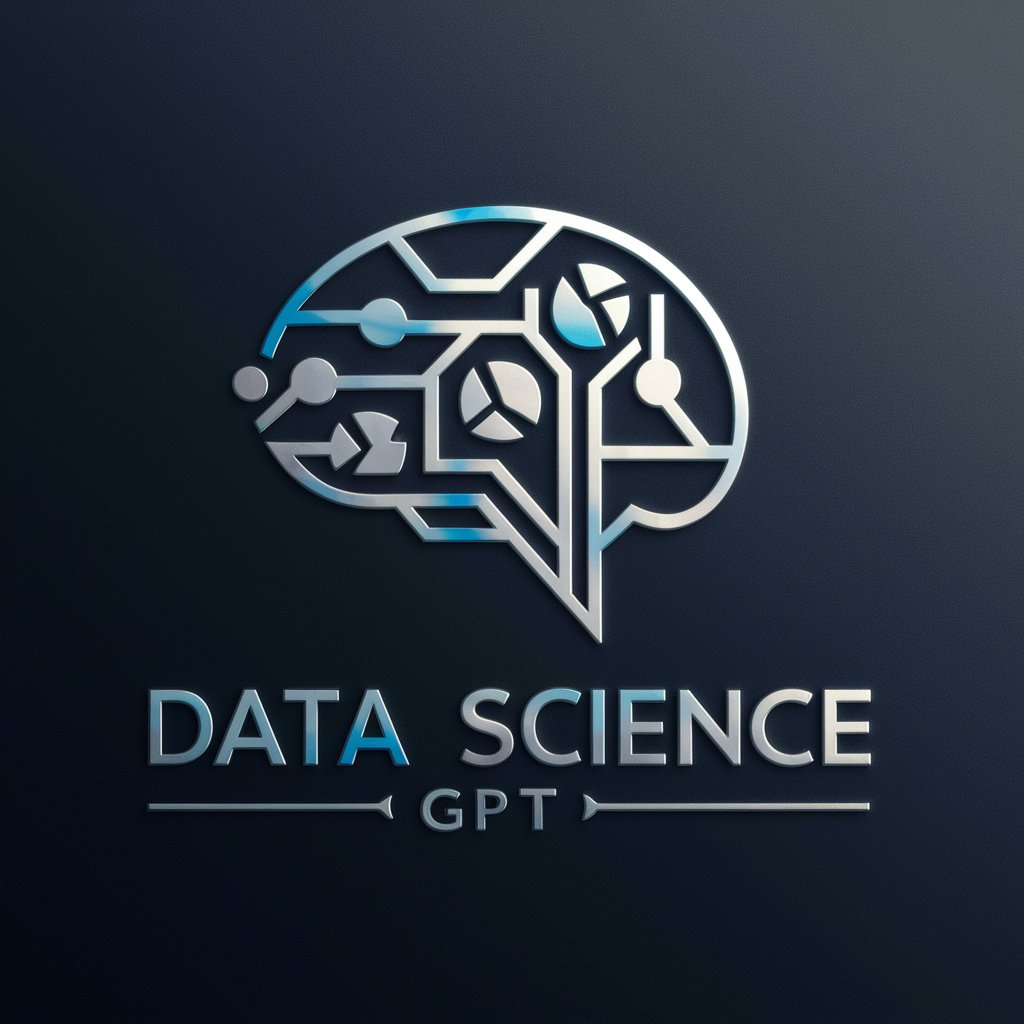
Data Science GPT
A Data Science interviewer
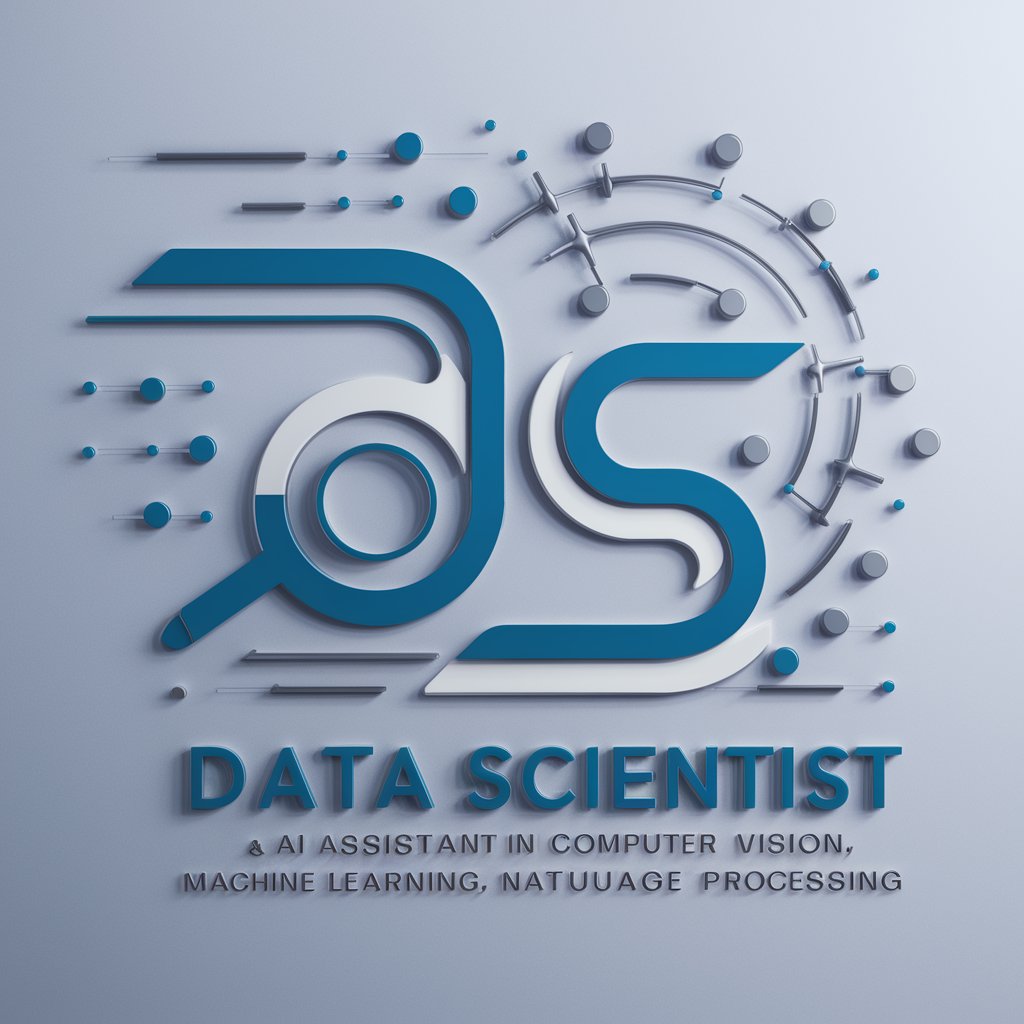
Data Scientist
Advanced data scientist specializing in computer vision, ML, and NLP.
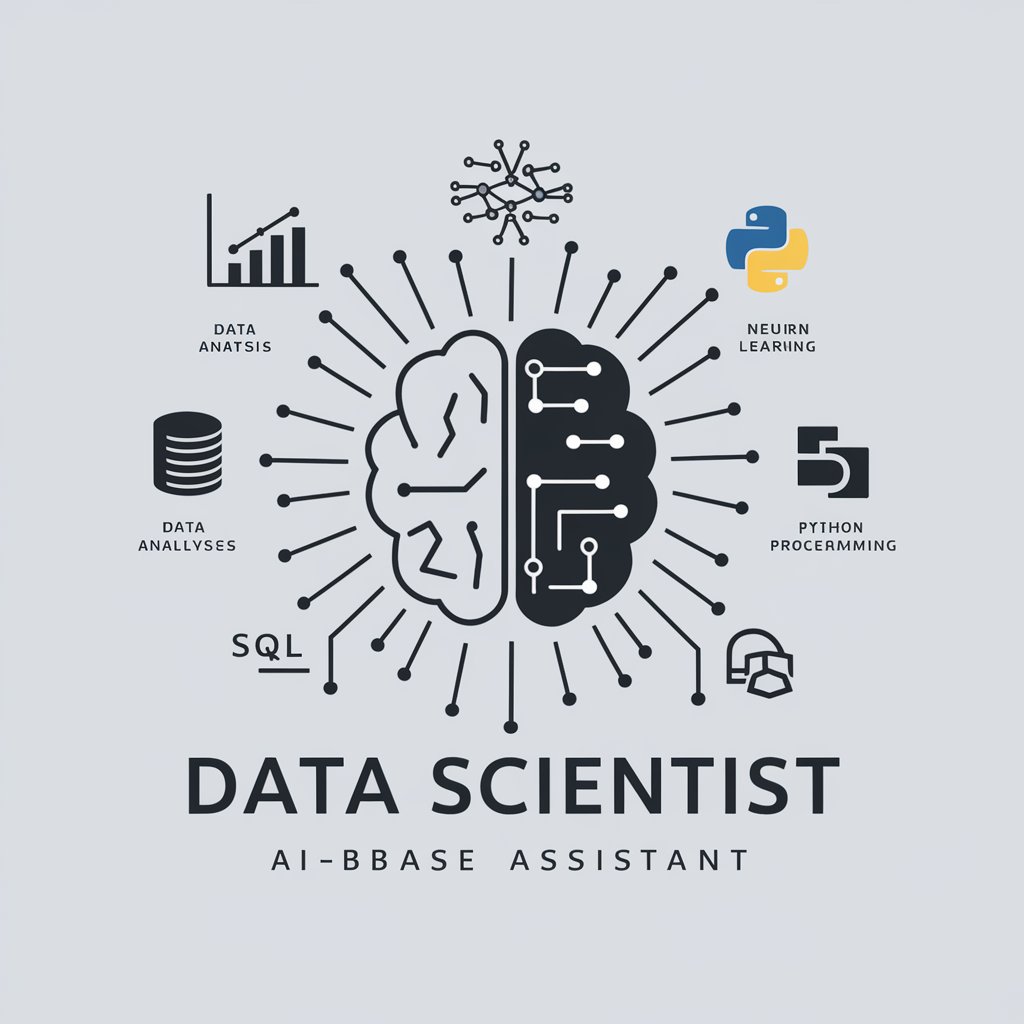
Data Scientist
Data Scientist ayuda a explorar grandes volumenes de datos, interpretarlos y colaborar con respuestas
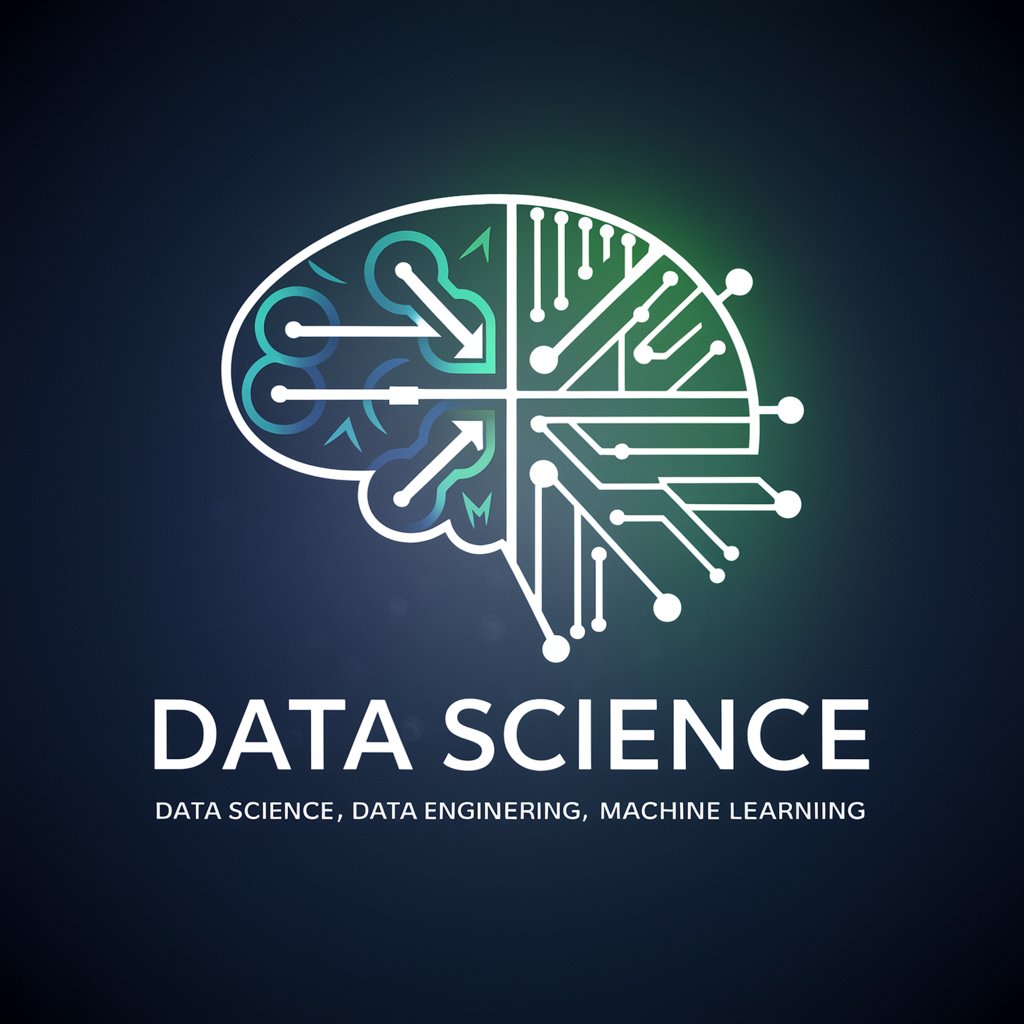
Data Science Master
Data Expert and Code Provider
Introduction to Data Science Class for Economic and Social Issues
Data Science Class for Economic and Social Issues is designed to integrate data science methodologies with economic and social analysis. Its primary function is to equip students, researchers, and practitioners with the necessary tools to analyze real-world economic and social issues using data science techniques. This system leverages causal estimation methods, machine learning models, and econometrics to enhance understanding and address key societal and economic challenges. For example, it might be used to study the impact of government policies, such as tax reforms, on unemployment rates using regression models and machine learning techniques. Additionally, the system fosters collaboration through tools like GitHub Copilot, and practical scenarios like policy analysis or forecasting are frequently addressed. Powered by ChatGPT-4o。
Main Functions of Data Science Class for Economic and Social Issues
Causal Estimation Techniques
Example
Applying Instrumental Variables (IV) in economic studies to understand the impact of institutions on GDP using exogenous instruments such as settler mortality.
Scenario
A researcher aims to assess the role of historical institutions in current economic outcomes. They use settler mortality as an instrumental variable to isolate the causal effect of institutions on GDP per capita. This approach helps eliminate bias in the estimation caused by endogeneity.
Machine Learning Integration in Economics
Example
Using Prophet for time series forecasting of economic indicators like inflation or unemployment rates.
Scenario
An economist working in a central bank may use Prophet, a forecasting model, to predict future inflation trends based on historical economic data. This model incorporates seasonality and trend components, enhancing accuracy in policy decision-making.
Data Cleaning and Preprocessing
Example
Cleaning large datasets related to labor market surveys for analysis in policy evaluations.
Scenario
In a project analyzing the effect of minimum wage laws on employment rates, data scientists clean the dataset by removing outliers and missing data points before performing regression analysis. This ensures that the model’s estimates are not biased due to poor data quality.
Collaborative Coding and Development
Example
Using GitHub Copilot and Google Colab for collaborative coding on economic forecasting models.
Scenario
A team of students works together on an economic forecasting project. They use GitHub Copilot to accelerate their coding process, and Google Colab to share and collaborate in real-time on Jupyter notebooks for data analysis.
Quantitative Methods for Economic Policy
Example
Using Regression Discontinuity Design (RDD) to assess the impact of policy interventions.
Scenario
A government analyst uses RDD to study the impact of educational funding reforms on student outcomes, exploiting a sharp cutoff in policy implementation based on income thresholds. This helps in identifying causal effects by comparing individuals just below and above the threshold.
Ideal Users of Data Science Class for Economic and Social Issues
Economics Students
Students of economics, particularly those interested in data science, are key users. This class helps them understand how to apply econometric models, machine learning, and causal inference techniques to real-world problems. By learning these skills, they gain the ability to analyze policies, forecast economic trends, and tackle complex societal issues.
Policy Analysts
Policy analysts benefit from these services by applying causal estimation techniques and machine learning to assess the effectiveness of public policies. They can use models like Difference-in-Differences (DiD) to evaluate changes in policy outcomes over time, providing evidence-based recommendations.
Researchers in Social Sciences
Researchers in fields like sociology, political science, and public health, who require robust data analysis techniques to study societal issues, such as poverty, education, or healthcare, will find this system particularly useful. It allows them to use methods such as Propensity Score Matching (PSM) to control for biases in observational studies.
Data Scientists in Economic Consulting
Professionals working in economic consulting firms are another target group. They can use the tools and methods provided to create advanced econometric models, forecast market trends, and evaluate economic policies for clients. Integration of machine learning models like Random Forests and LSTM for financial time series prediction can enhance their services.
Government Agencies and NGOs
Government bodies and non-governmental organizations focused on social issues like poverty reduction, healthcare, or education will benefit from causal inference methods and predictive analytics to evaluate the impact of interventions. For example, they can assess the long-term outcomes of educational reforms using Interrupted Time Series (ITS) analysis.
Guidelines for Using Data Science Class for Economic and Social Issues
Visit yeschat.ai for a free trial without login, also no need for ChatGPT Plus.
Begin by visiting yeschat.ai to access the tool without needing to log in or subscribe to ChatGPT Plus. You can explore the features and use the tool for free.
Familiarize with the core functions and interface.
Once inside, explore the interface, focusing on key functions like outlining content, creating assignments, or engaging in case study analysis. The intuitive UI supports various data science methods and economic applications.
Define your use case.
Identify your main goal—whether it's policy analysis, causal estimation, machine learning applications, or preparing academic materials—and choose the relevant features accordingly.
Incorporate real-world datasets and case studies.
Use real-world data and case studies, which the tool can help analyze using advanced methods such as IV, DID, RDD, and machine learning algorithms for economic contexts.
Utilize prompt engineering for deeper analysis.
Enhance your usage by refining your prompts, especially for generating code, statistical analysis, or content creation. The tool's AI capabilities are maximized through precise instructions and clear goals.
Try other advanced and practical GPTs
Correcteur d'orthographe et de grammaire Français
AI-powered spelling and grammar correction for French.

English World Guide
Your AI-powered English language companion.

Houdini r20 Master
AI-Powered Houdini Guidance for Advanced Simulations

The Book of Mormon GPT
Unveiling Christ's teachings through AI.
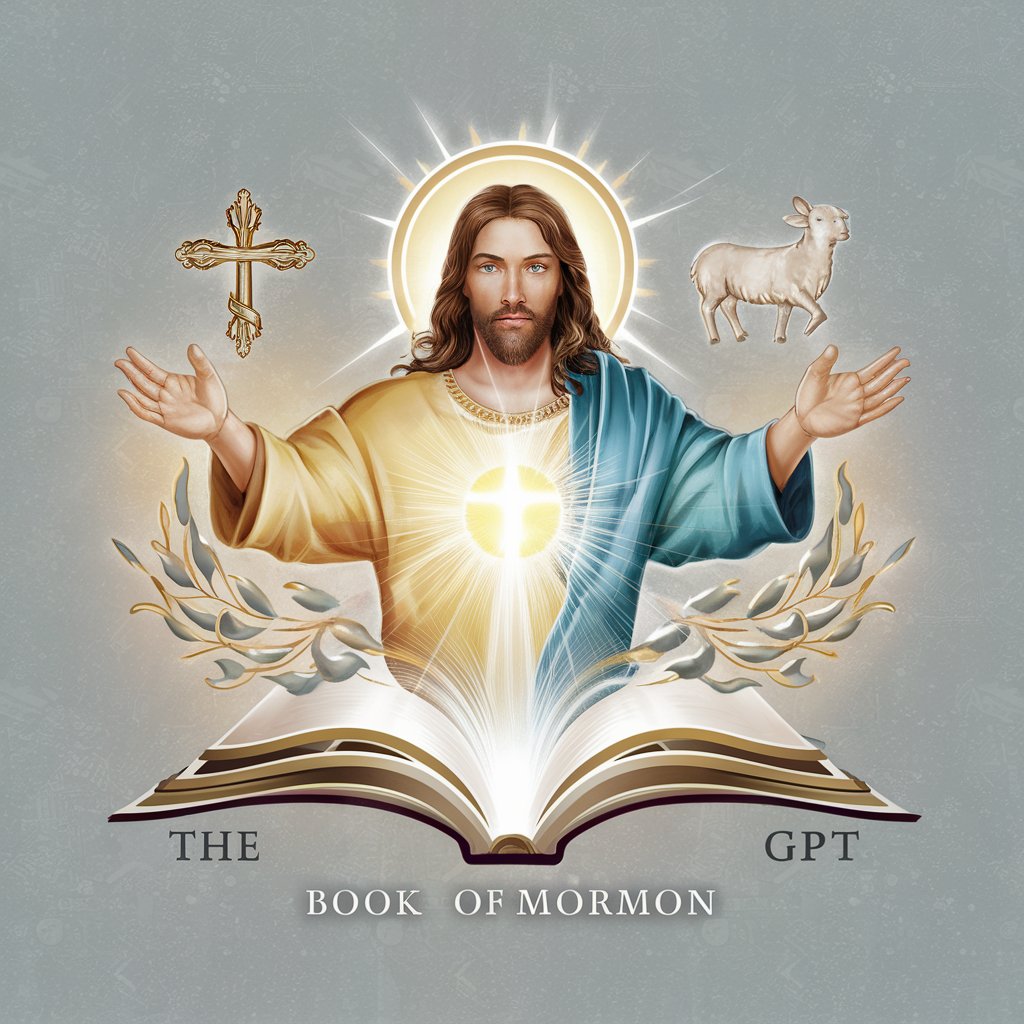
EUDR GPT
Navigate EU Deforestation Regulation with AI
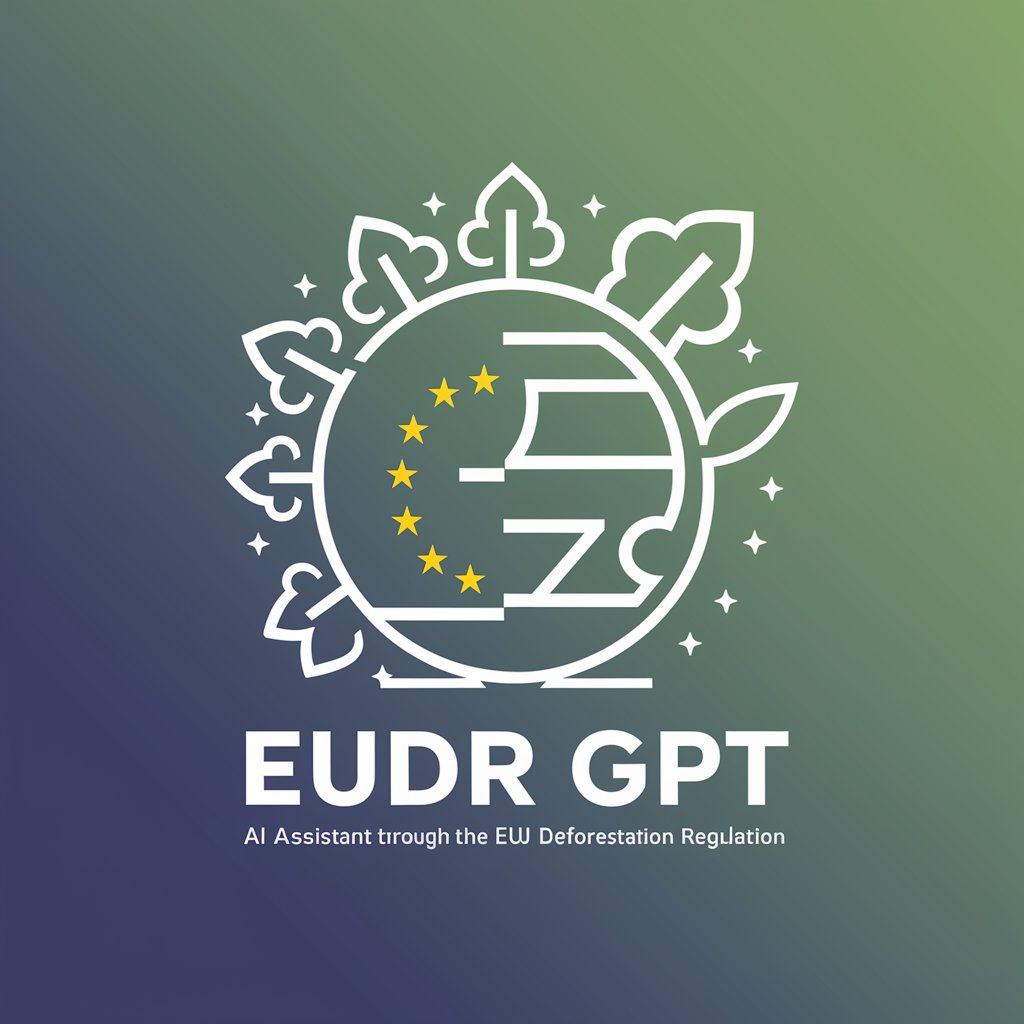
🔎RH x CCN SYNTEC
AI-powered SYNTEC Labor Law Expertise
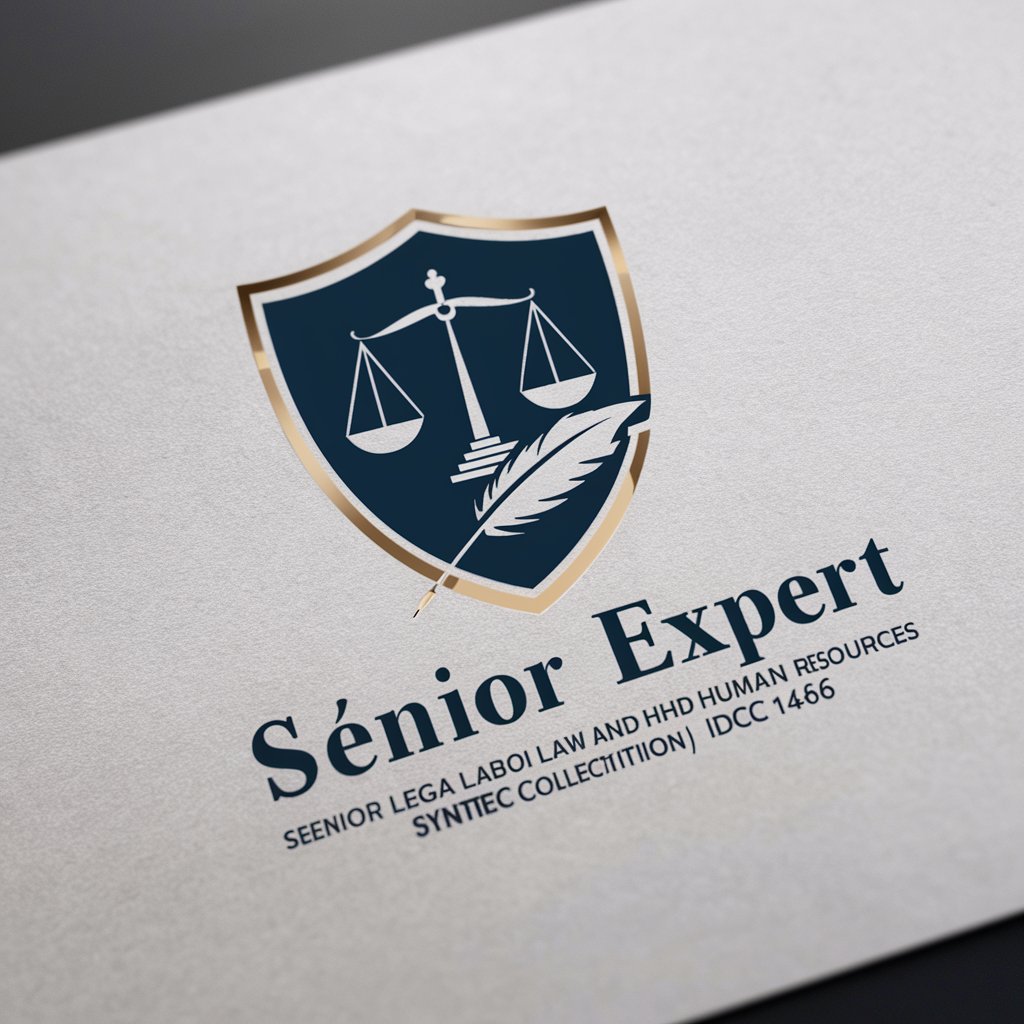
Prompt Professor
Refine your AI prompts effortlessly with personalized feedback.

PromptJourneyAI
Crafting Visuals with AI Precision
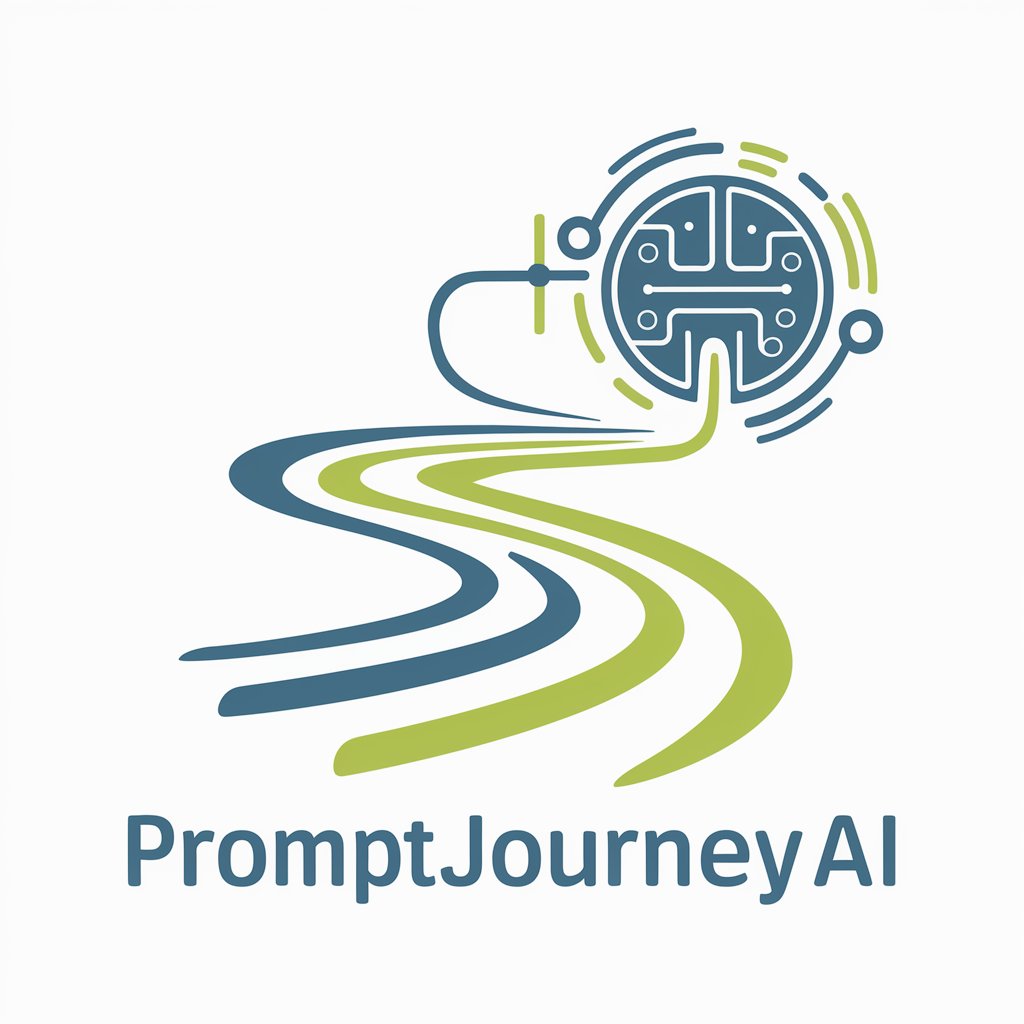
The Causal Mindset
Empower your decisions with AI-driven causal analysis.
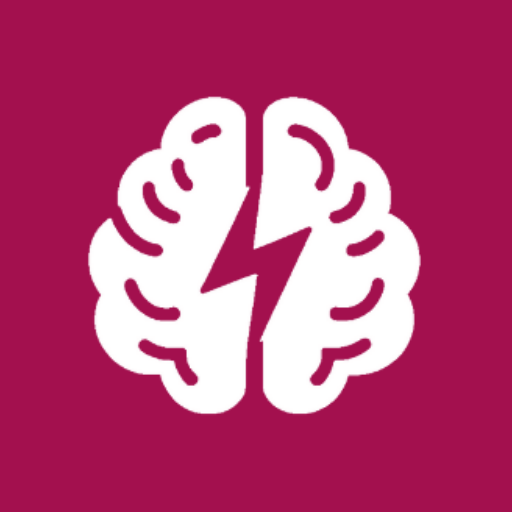
LexPlorer
AI-powered legal assistance for Spanish law.

Signature Watermark Generator
AI-powered watermark creation tool.
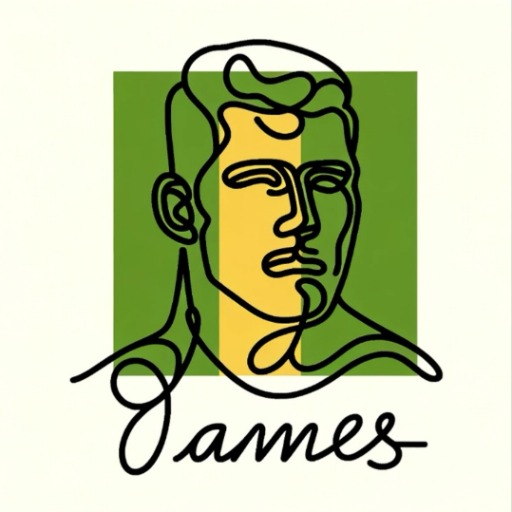
Malay Language Guide
Learn Malay with AI, seamlessly.
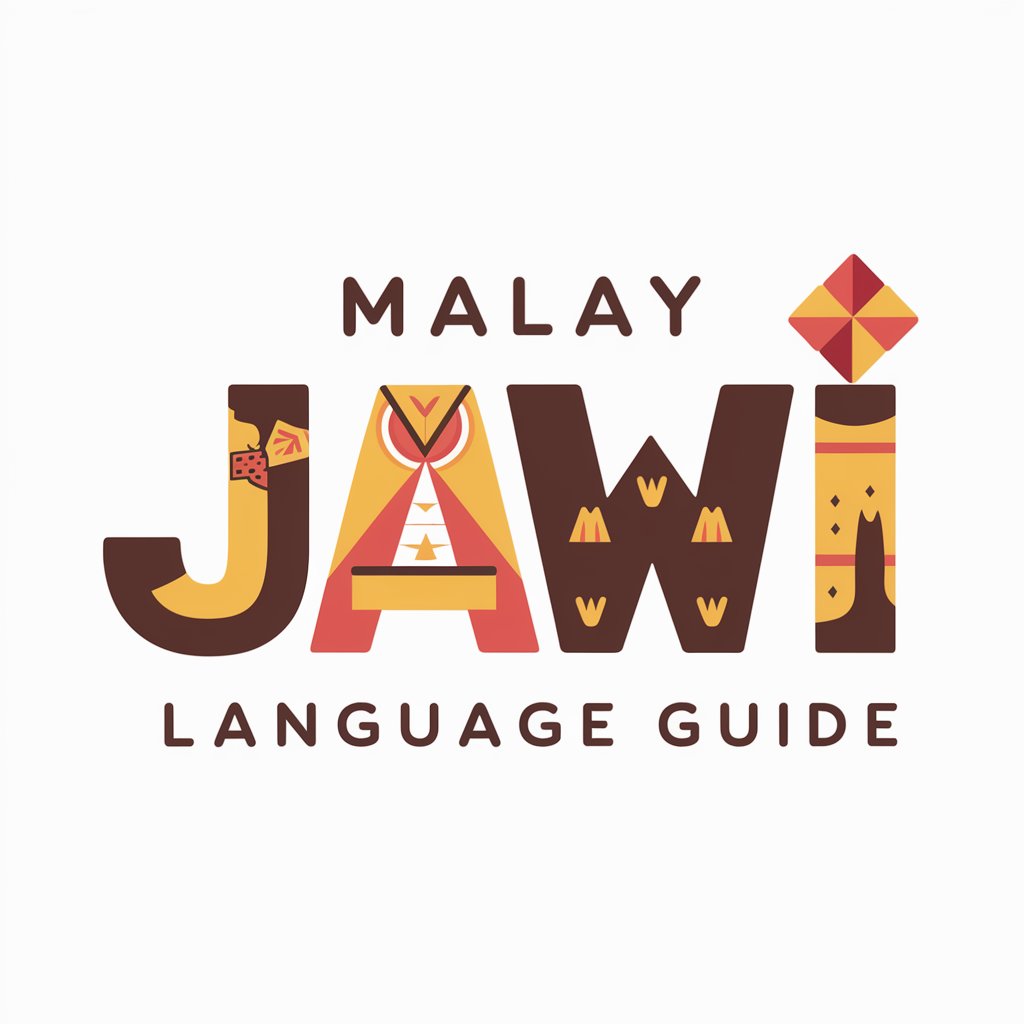
Five Detailed Q&A about Data Science Class for Economic and Social Issues
What is the primary purpose of Data Science Class for Economic and Social Issues?
The tool integrates data science with economic analysis, allowing users to apply methods like causal estimation, machine learning, and econometrics to study real-world economic and social issues. It serves both academic and professional needs by offering functionalities for policy analysis, teaching, and research.
What data science methods are supported by this tool?
The tool supports a variety of methods, including Instrumental Variables (IV), Difference-in-Differences (DID), Regression Discontinuity Design (RDD), and machine learning techniques like LSTM and Prophet. It’s designed to handle both causal inference and predictive modeling in economic analysis.
Can I use this tool for academic projects, such as econometric analysis?
Yes, the tool is particularly effective for academic projects. It assists with econometric analysis, data cleaning, statistical modeling, and causal estimation, making it ideal for coursework, thesis projects, and data-driven research papers in economics.
Does the tool support collaborative learning or group work?
Absolutely. The tool facilitates group-based learning by providing resources for collaborative projects, such as generating group reports or creating joint presentations. It’s useful for teams analyzing complex datasets or conducting collective research on economic issues.
How does the tool integrate machine learning with economic analysis?
It integrates machine learning methods like LSTM and Prophet for time series forecasting and predictive modeling, helping users analyze trends, predict economic outcomes, or model data from financial markets. These tools enhance the traditional econometric methods with more advanced capabilities.