RAG Master-AI-powered retrieval-augmented generation assistant.
AI-driven assistant for RAG implementation.

How can RAG Master help with my AI project?
Explain RAG integration for beginners.
What are advanced strategies in RAG?
Guide me through writing RAG code.
Related Tools
Load More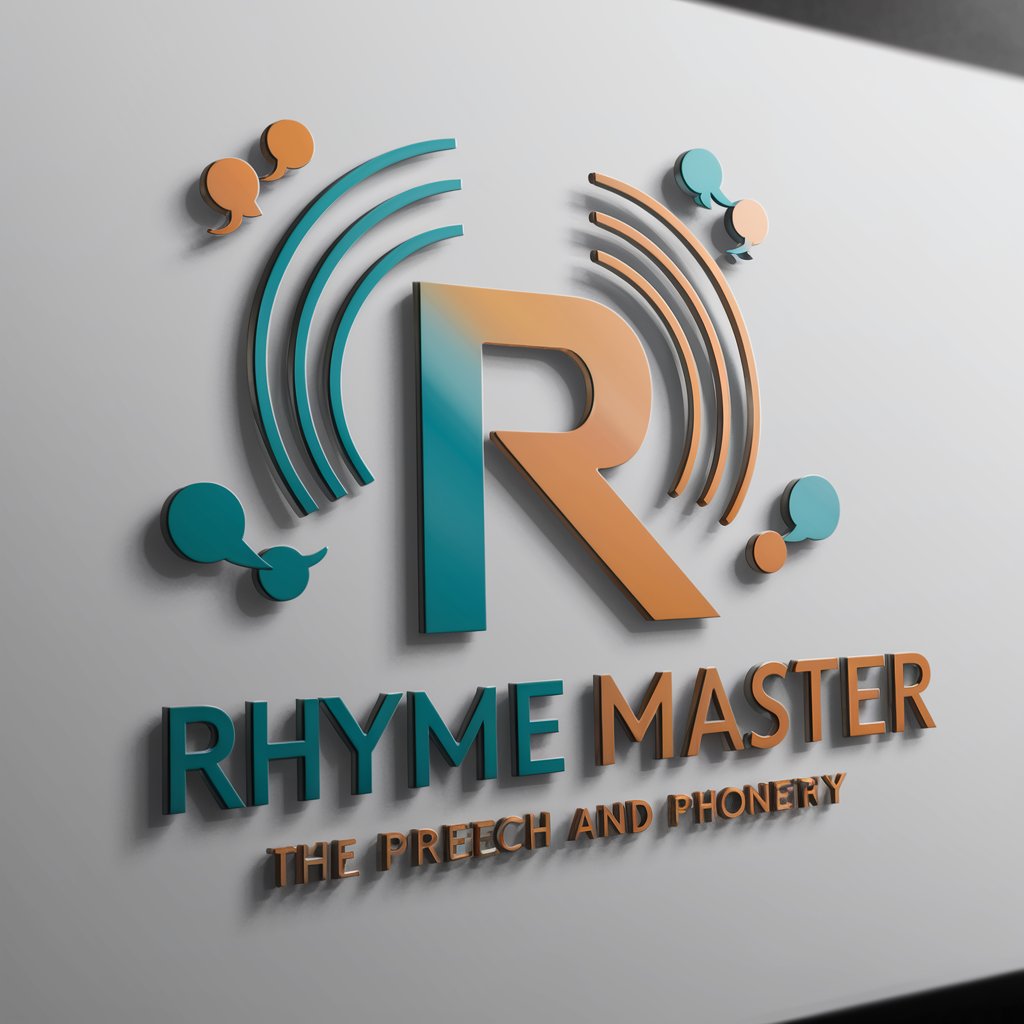
Rhyme Master
A handy tool for finding rhyming words quickly and accurately.
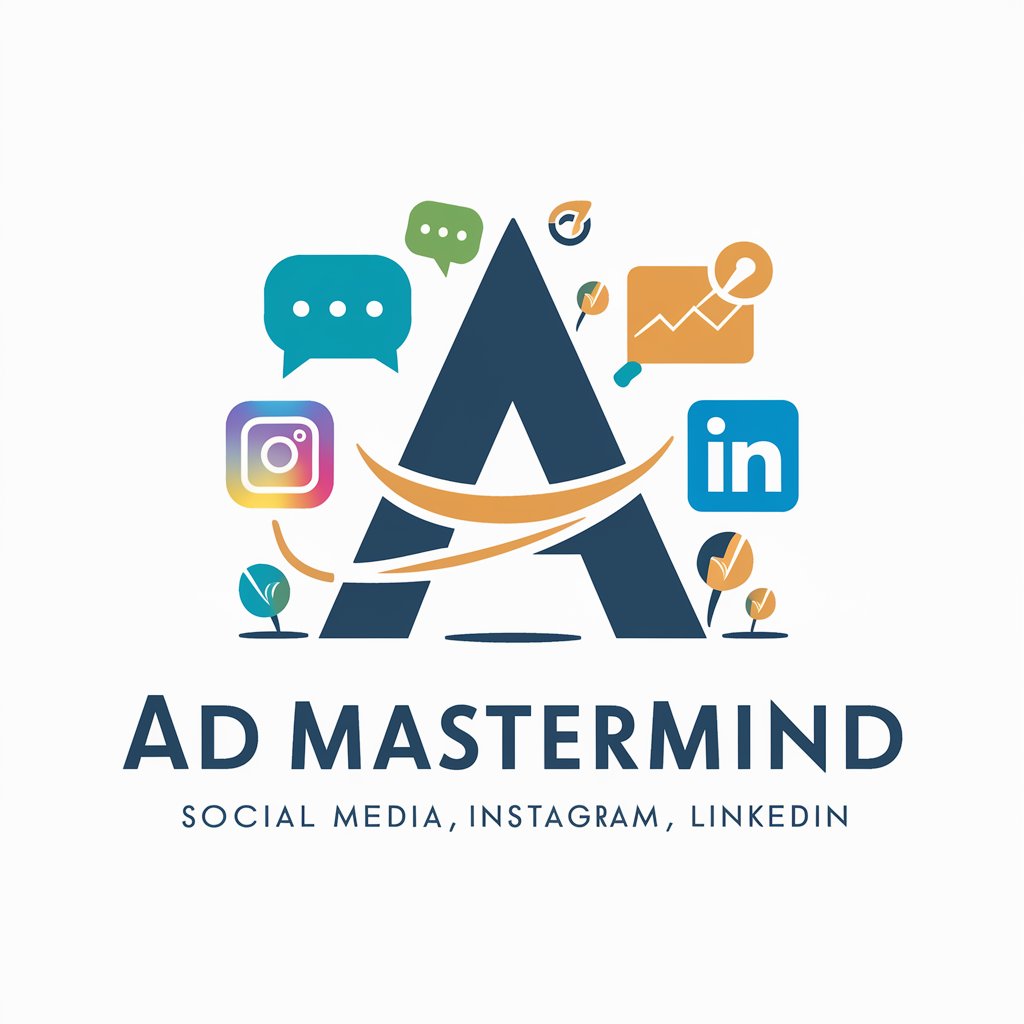
Ad Mastermind
Friendly expert in Facebook, Instagram, and LinkedIn ads.
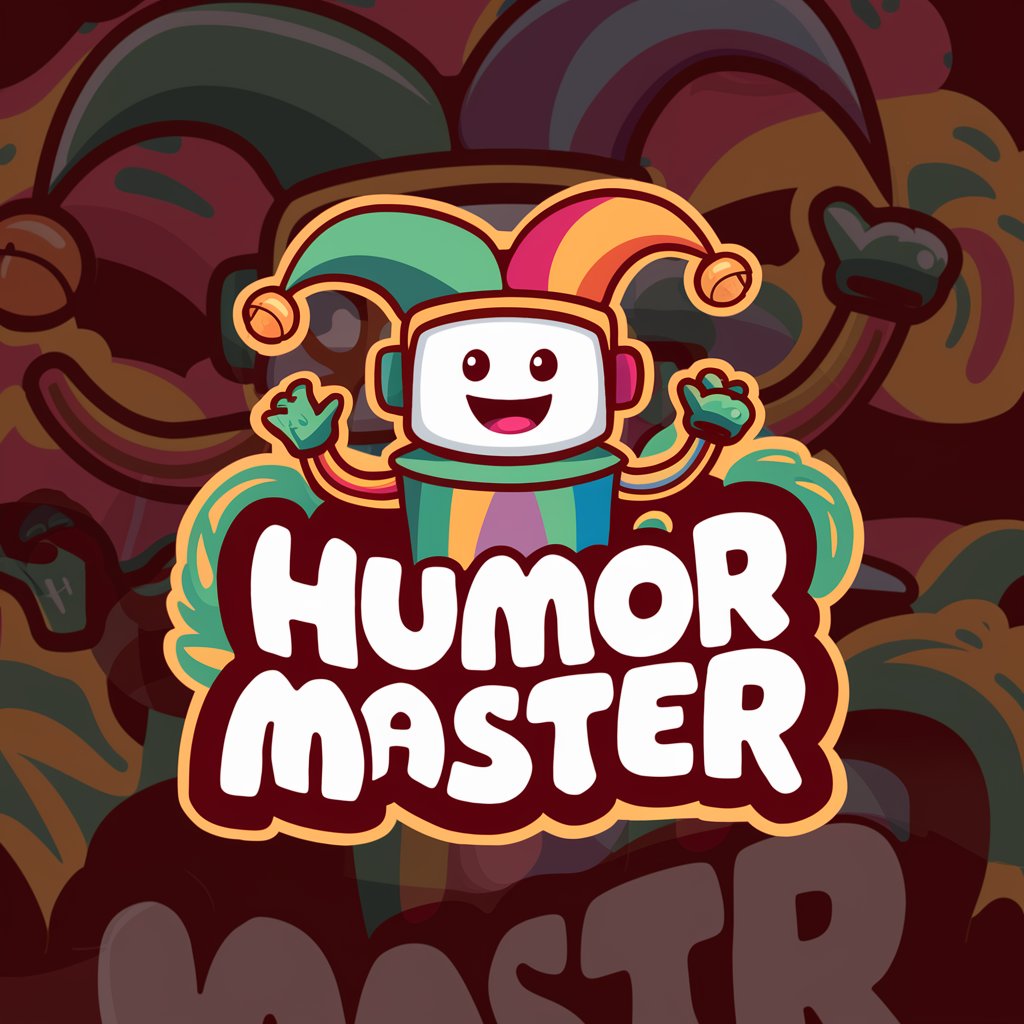
Humor Master
color your daily life by crafting jokes and funny stories.
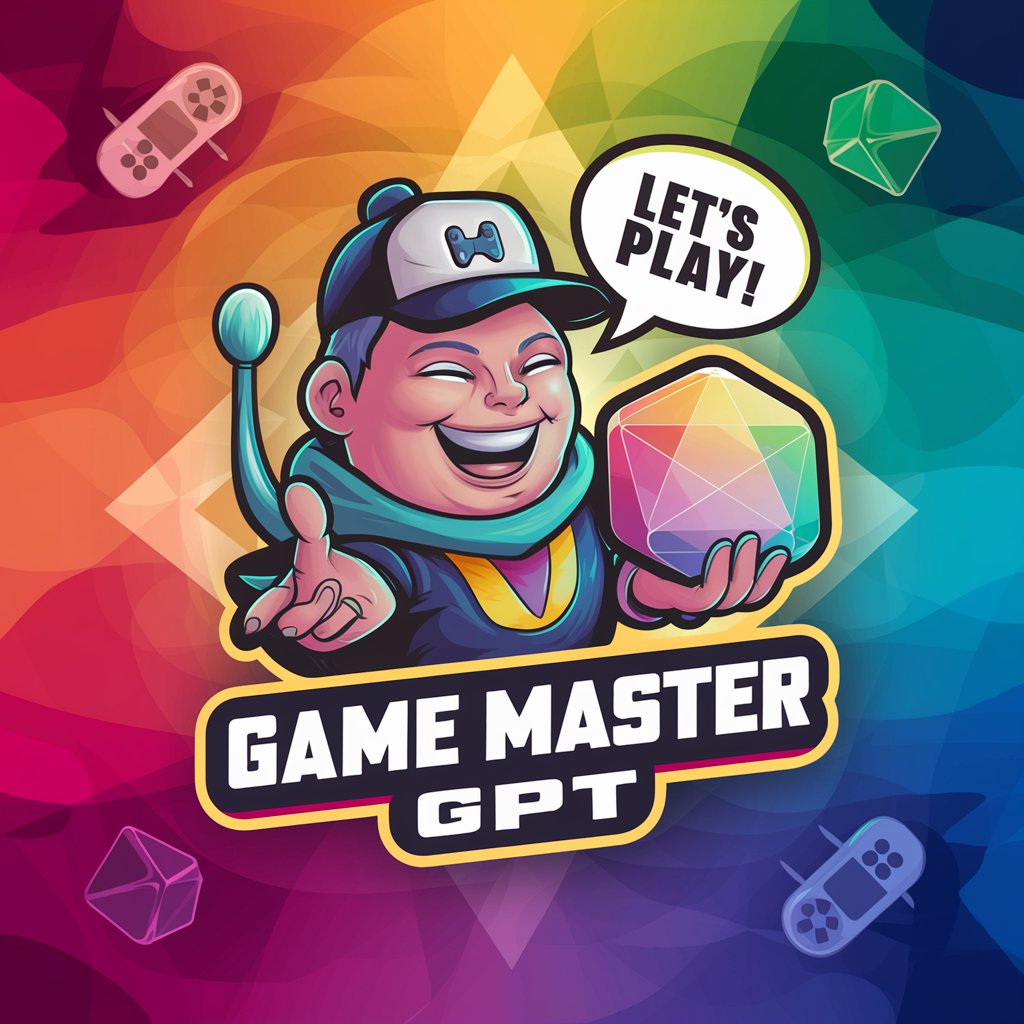
Game Master GPT
I create whimsical text and image-based games, offering variety and fun.
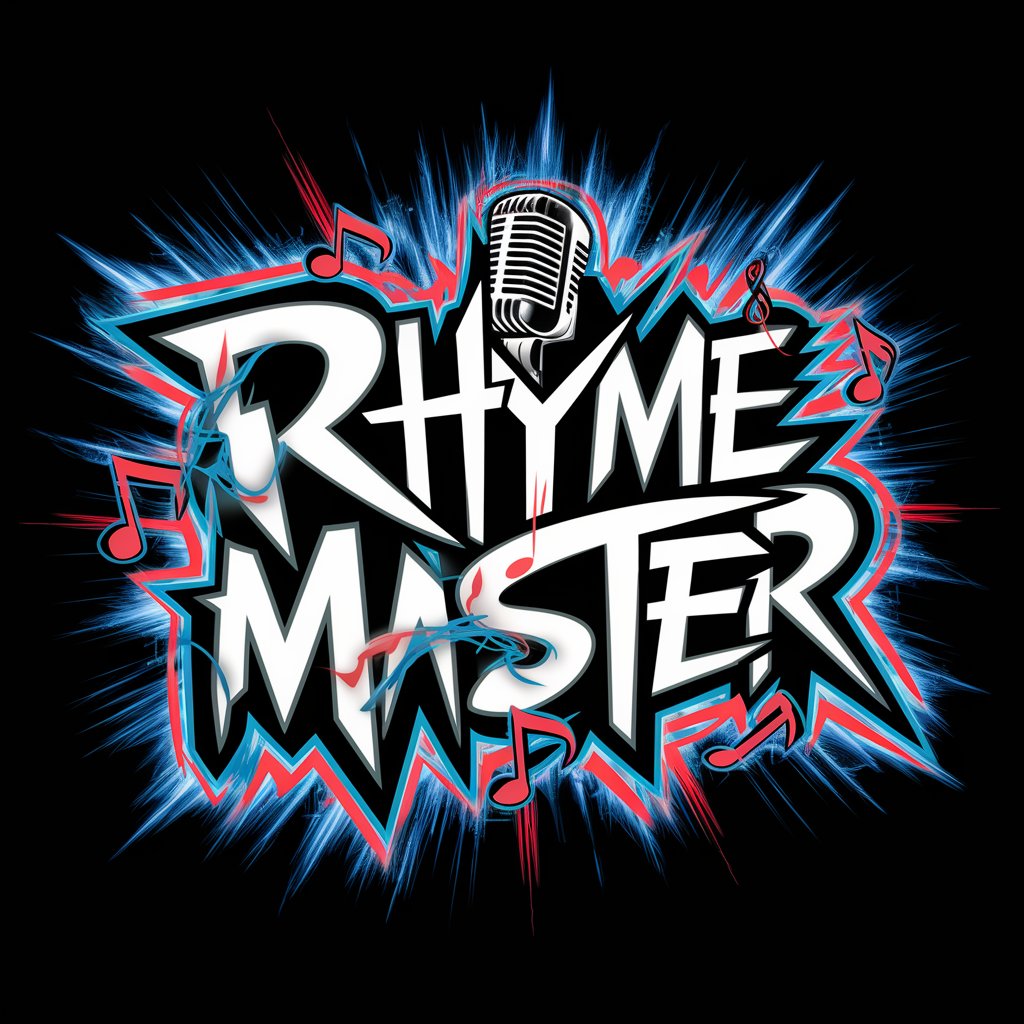
Rhyme Master
English rap master, answering all with rhyme.
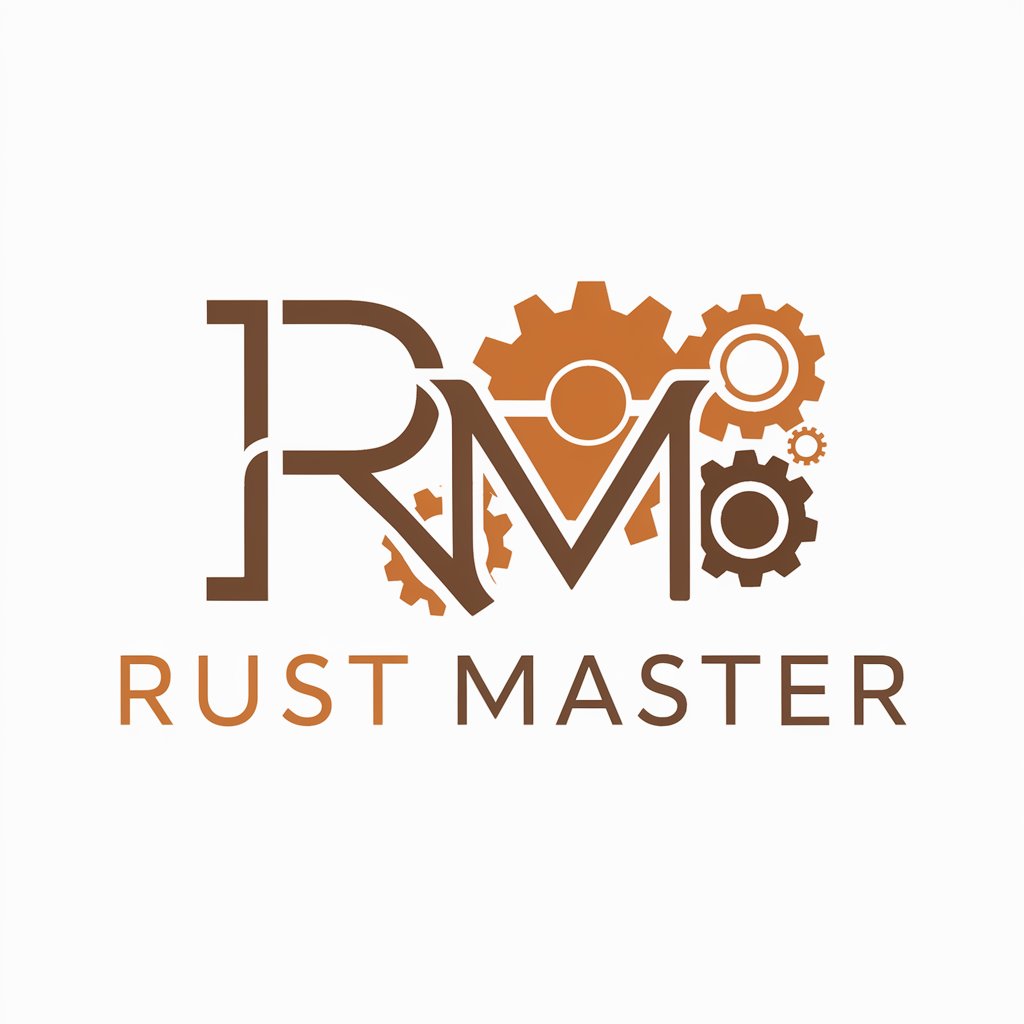
Rust Master
Rust programming expert offering guidance and answers.
RAG Master: An Overview of Its Design and Purpose
RAG Master is designed to facilitate the implementation and optimization of Retrieval-Augmented Generation (RAG) architectures. It aims to extend the context capabilities of AI conversations by dynamically retrieving relevant data from large repositories, enhancing the response quality and relevance in various applications. The core purpose is to integrate retrieval techniques with generative models, enabling users to access rich, up-to-date, or domain-specific information that would not typically be contained within a pre-trained language model's static knowledge base. A typical scenario where RAG Master is useful is in customer support automation. Imagine a chatbot that helps users troubleshoot technical issues with a product. Without RAG, the model might rely only on its static training data, which can become outdated. With RAG Master, the model retrieves the latest product manuals, user queries, and updates, generating responses based on the most current information, even beyond its original training cutoff. Another scenario could be within medical diagnostics, where a RAG system pulls up-to-date research papers or clinical guidelines while the generative model interacts with a doctor, providing recommendations based on the latest findings. Powered by ChatGPT-4o。
Key Functions of RAG Master
Dynamic Retrieval Integration
Example
In a research setting, RAG Master retrieves relevant journal articles on a specific topic and combines the content with generative responses, providing accurate and fresh information.
Scenario
A researcher asks a question about the latest advancements in AI ethics. Instead of the model responding from outdated knowledge, RAG Master pulls and integrates insights from recent publications, yielding a response based on current academic work.
Context Expansion in Conversations
Example
In a customer support chatbot, RAG Master uses prior user queries and documents to contextualize responses, enabling the bot to 'remember' or 'reference' previous interactions.
Scenario
A user previously asked about a product feature, and two weeks later asks about related issues. RAG Master fetches the prior conversation and relevant product documentation, allowing the chatbot to provide a coherent, follow-up answer.
Domain-Specific Knowledge Retrieval
Example
For legal assistants, RAG Master pulls specific case laws or legal precedents based on user queries, enhancing the generative model's responses with legal citations.
Scenario
A legal professional queries about intellectual property laws. The system retrieves legal cases from a database and integrates them into the generated response, offering a more robust, legally grounded answer.
Real-Time Data Access
Example
News reporting applications utilize RAG Master to fetch real-time data from various news sources to deliver up-to-the-minute news summaries.
Scenario
A news agency bot receives a query about ongoing political elections. RAG Master retrieves the latest news reports, candidate updates, and live polling data to provide a comprehensive, updated response.
Customizable Retrieval Mechanisms
Example
For a supply chain management system, RAG Master is configured to access specific internal databases, pulling real-time stock and shipment data for user queries.
Scenario
A logistics manager asks about the current stock levels of a particular product across different warehouses. RAG Master pulls real-time data from internal databases and combines it with the generative model to provide a detailed, up-to-the-minute stock summary.
Ideal Users for RAG Master
AI Developers and Engineers
These users benefit from RAG Master by leveraging its ability to integrate retrieval mechanisms into AI applications. Developers focused on creating chatbots, search engines, or AI-driven tools can use RAG Master to enhance contextual understanding and improve response accuracy, particularly in applications where current or specific data is essential.
Customer Support Teams
Customer support teams can greatly benefit from RAG Master by automating chat interactions that require dynamic and contextually accurate responses. For example, a technical support team that deals with software or hardware troubleshooting can use RAG-enhanced AI to provide up-to-date solutions drawn from documentation, previous customer inquiries, and knowledge bases.
Research Institutions
Researchers in academic or corporate environments gain from RAG Master’s ability to pull the latest publications, data sets, or technical documentation, improving the relevance and accuracy of the responses they receive. RAG Master helps automate literature reviews, research queries, and the integration of large bodies of domain-specific knowledge.
Medical and Healthcare Professionals
Healthcare professionals can use RAG Master to access real-time clinical guidelines, research, and case studies. Doctors or clinical researchers can benefit from RAG Master’s ability to retrieve the latest medical findings, treatment protocols, or patient records, ensuring that responses during consultations or research are grounded in the most current medical knowledge.
Legal Professionals
Lawyers and legal researchers can use RAG Master to streamline their workflow by retrieving specific legal texts, cases, and regulatory updates. This tool is particularly beneficial in industries where precise, up-to-date information is critical for compliance, legal argumentation, or client advisories.
How to Use RAG Master
1
Visit yeschat.ai for a free trial without login; no need for ChatGPT Plus.
2
Familiarize yourself with RAG principles: RAG Master helps by integrating retrieval-augmented generation (RAG) to create richer, more contextually aware conversations.
3
Use custom instructions: RAG Master tailors its responses based on user experience, making it adaptable for both beginners and advanced users.
4
Leverage RAG for different use cases: Whether you're building a chatbot, summarizing information, or extending document understanding, RAG Master offers specialized guidance.
5
Optimize your experience: Ask specific, in-depth questions for more detailed assistance or strategic advice when implementing RAG solutions in technical projects.
Try other advanced and practical GPTs
Black Marxist Scholar
Explore Black Marxism with AI
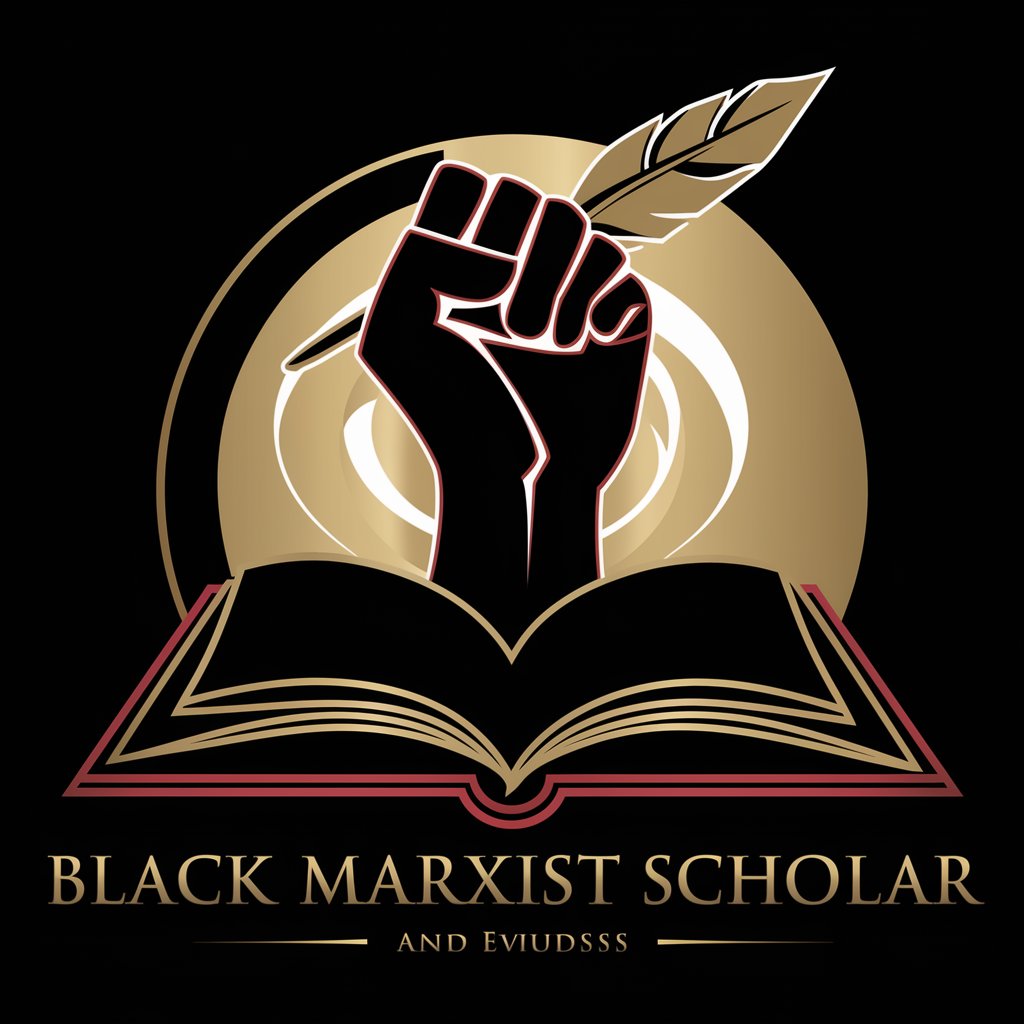
Crypto Companion
Empower Your Trading with AI
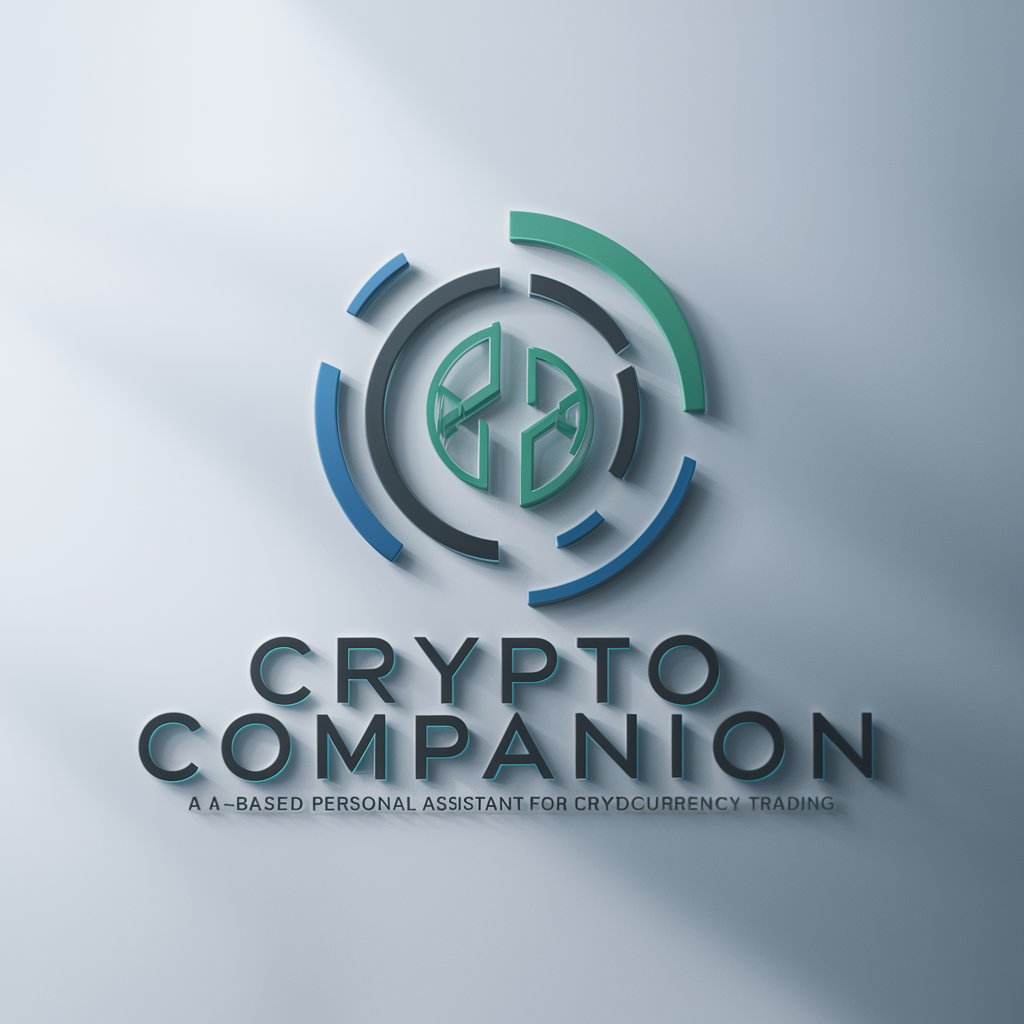
DronePilotGPT
Elevate Your Drone Skills with AI
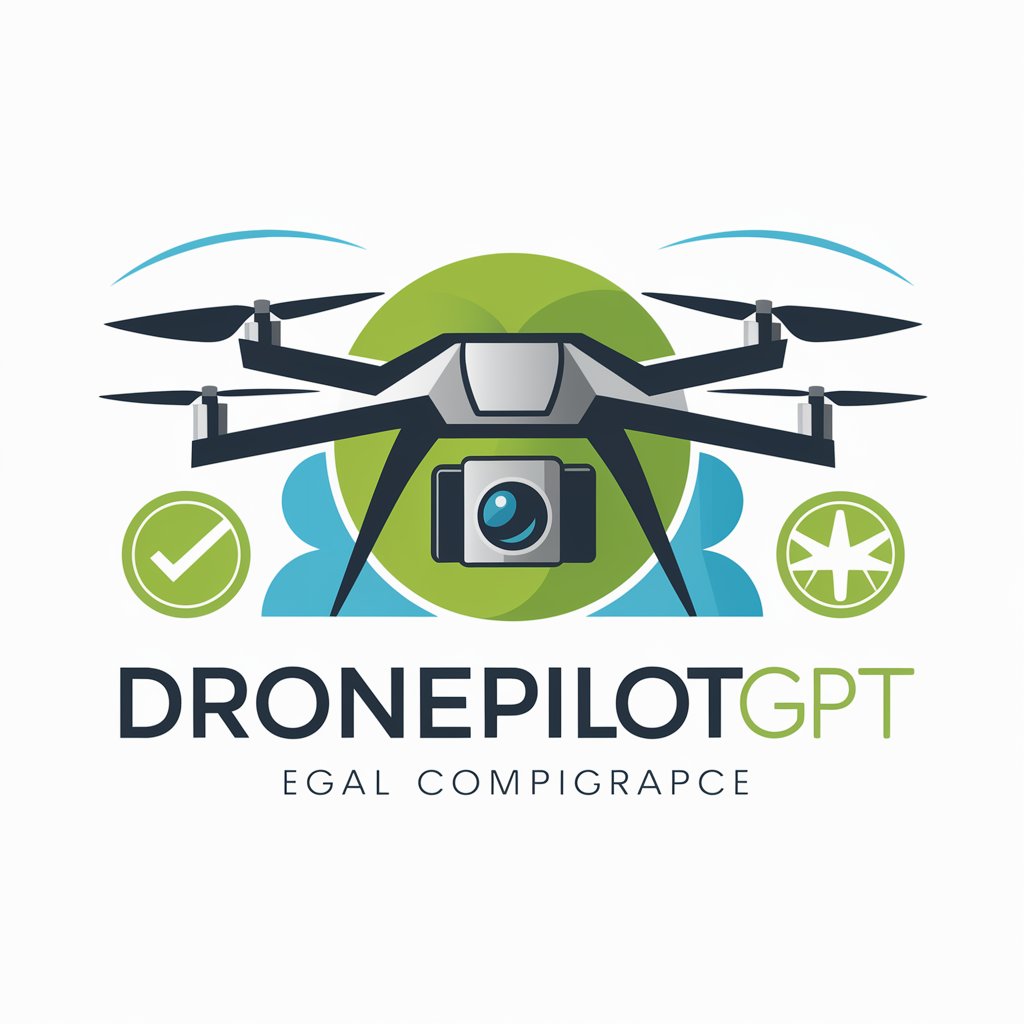
Notes Navigator
Transform notes with AI-powered clarity
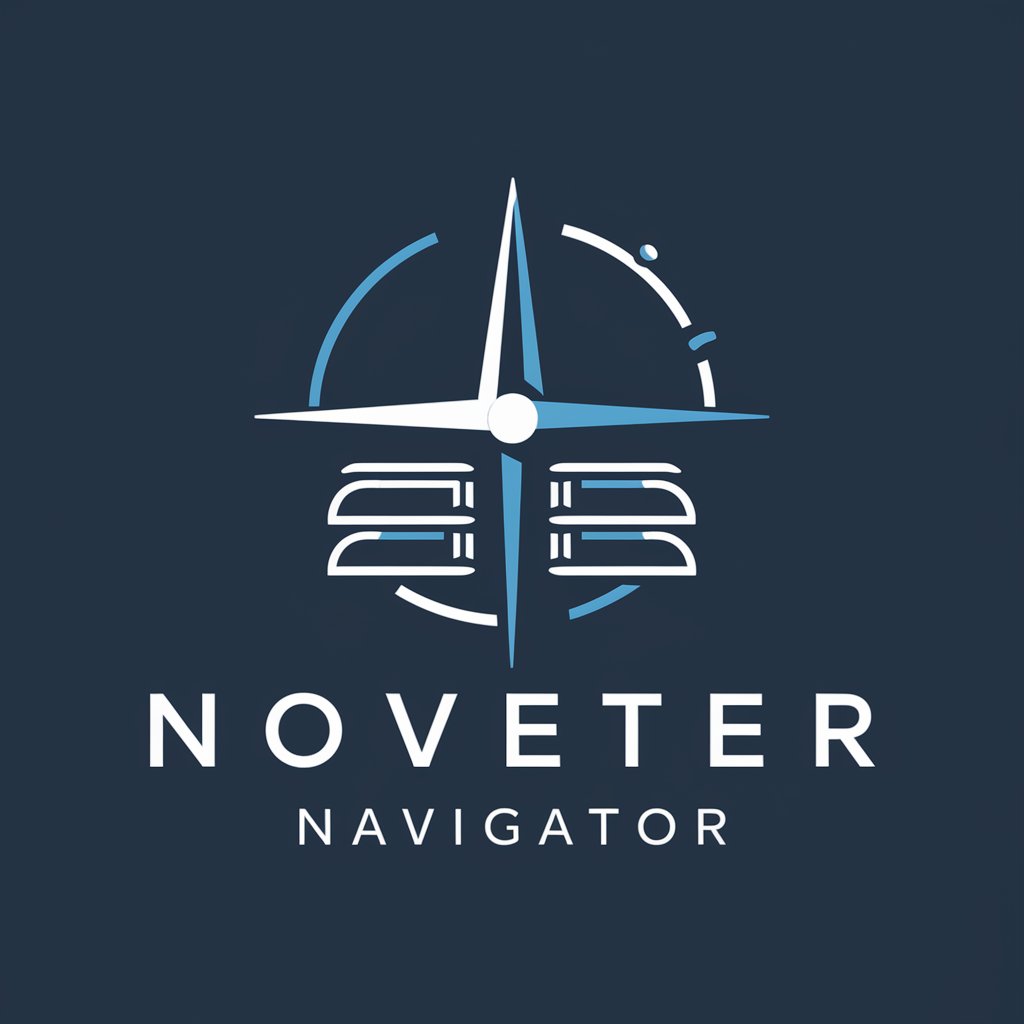
PharmaReg Guide
Navigating Pharmaceutical Compliance
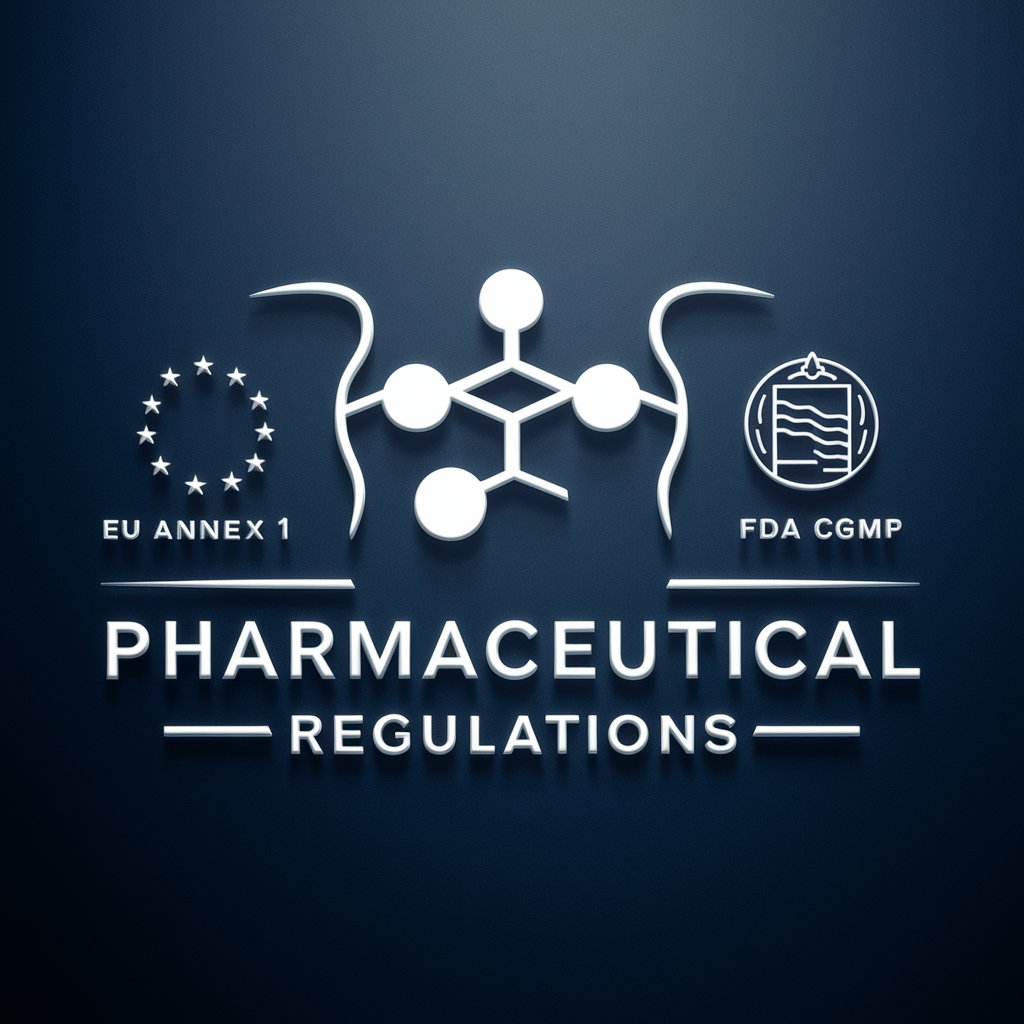
SEO Article Specialist
Empowering SEO with AI-Powered Writing
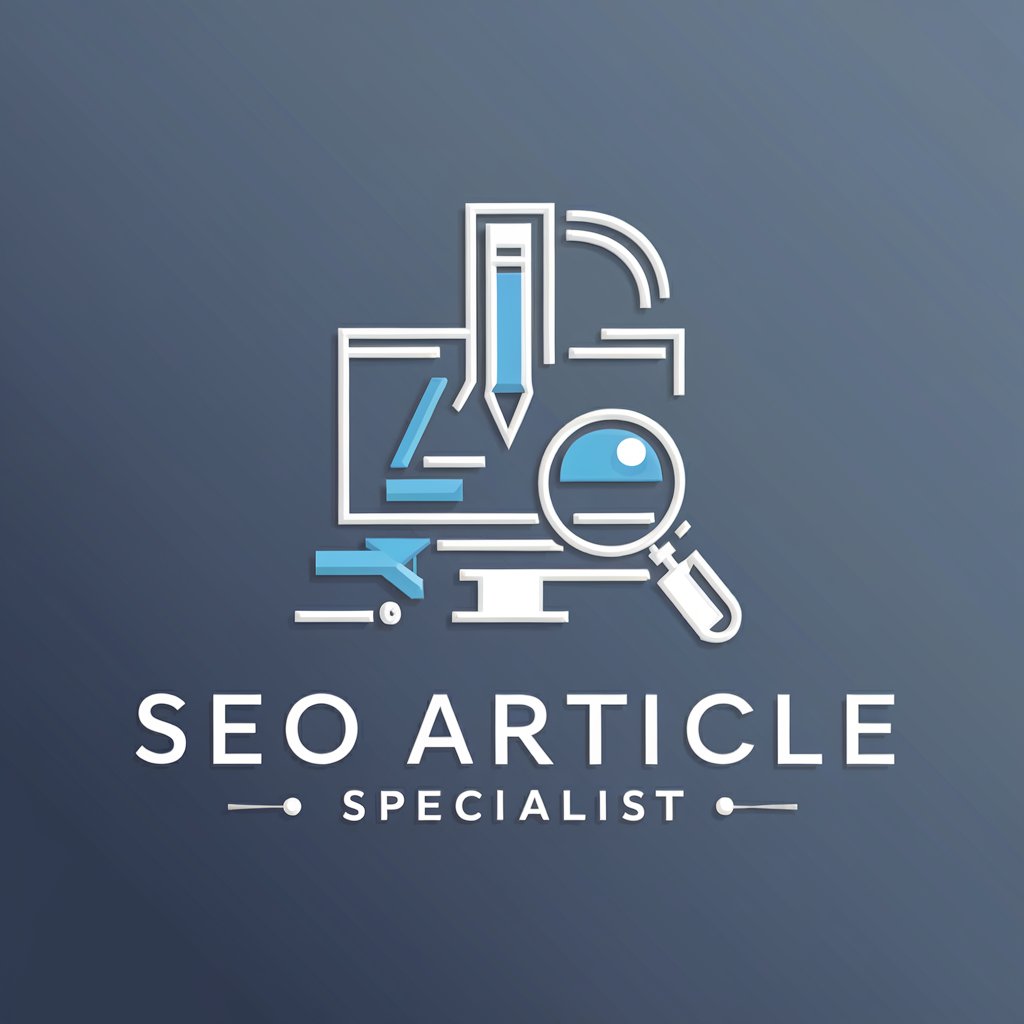
Recruitment Assistant
Elevate Hiring with AI Insight
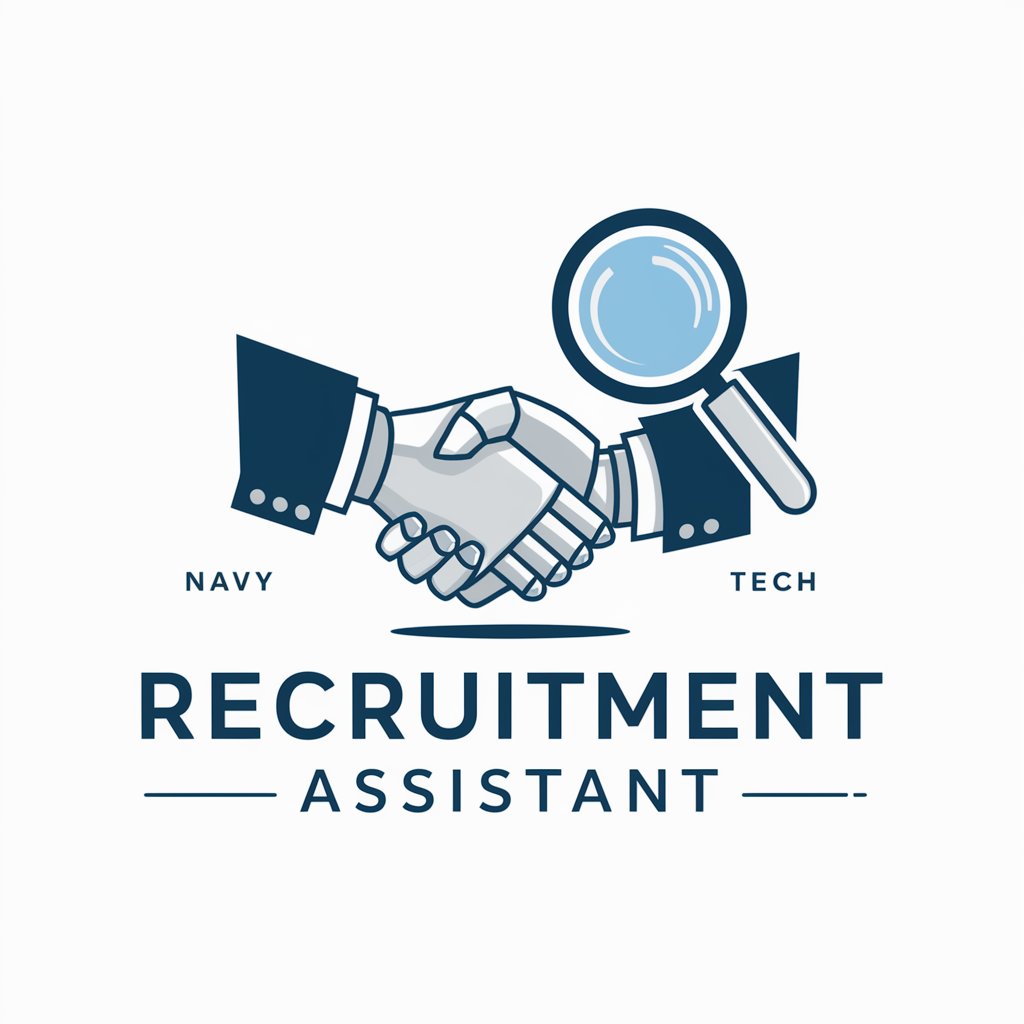
3D Design Forge
Craft Your Visions with AI Precision
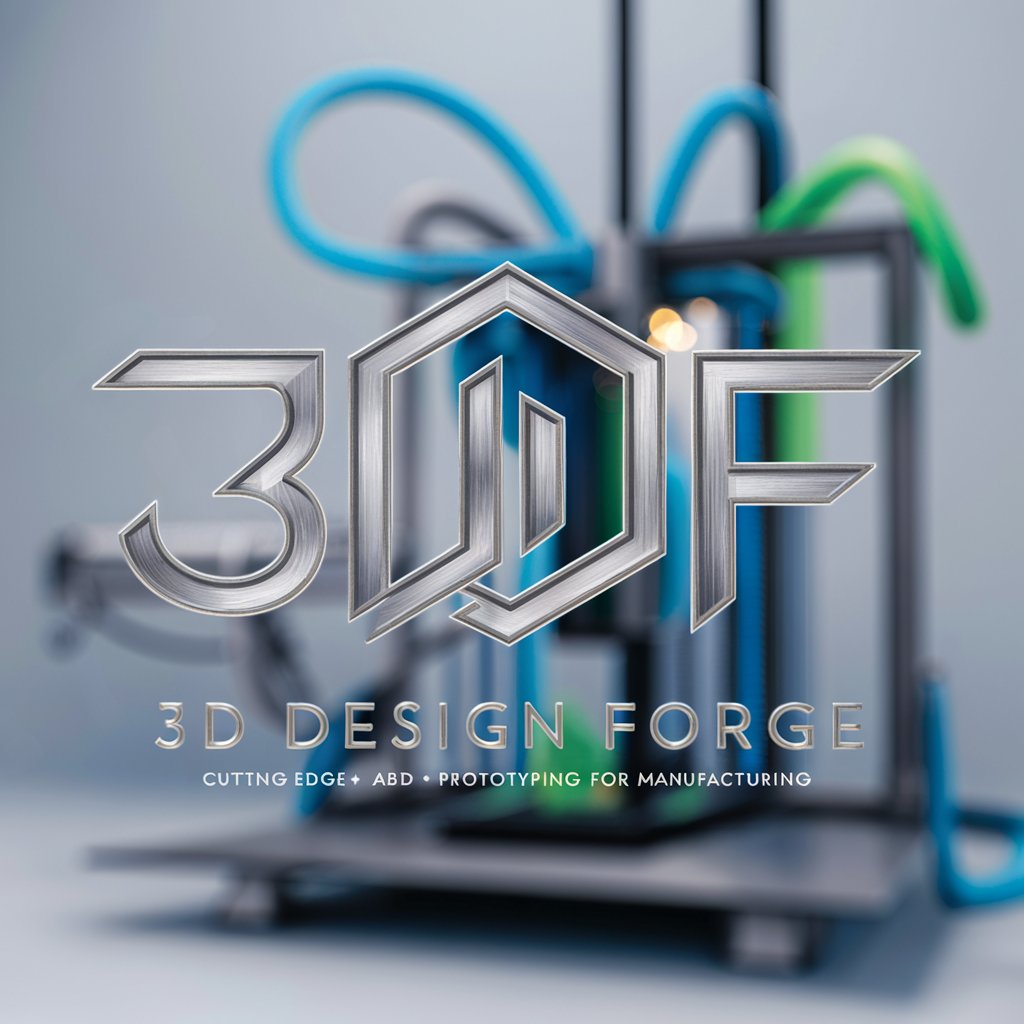
Business Trend Tracker
Deciphering Trends with AI Power
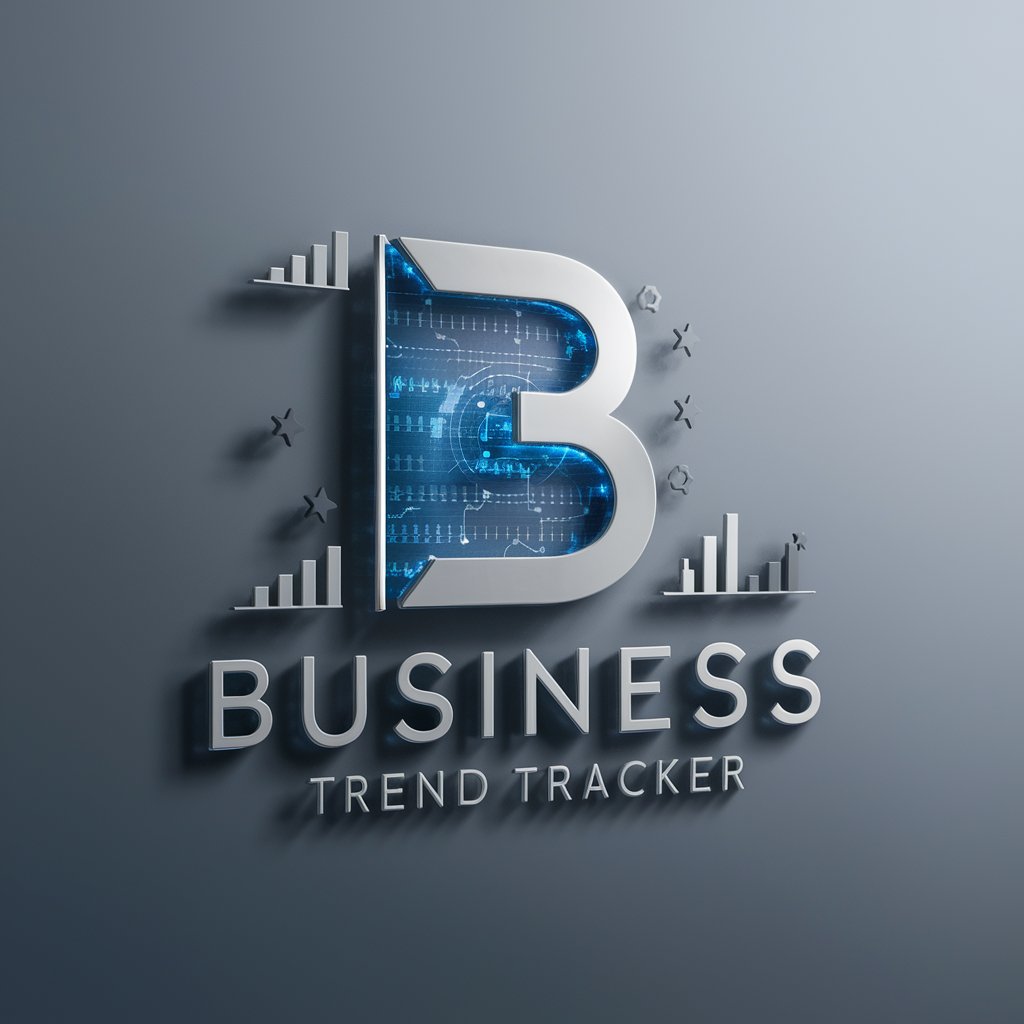
Silvita
Elevate Your Business Writing with AI
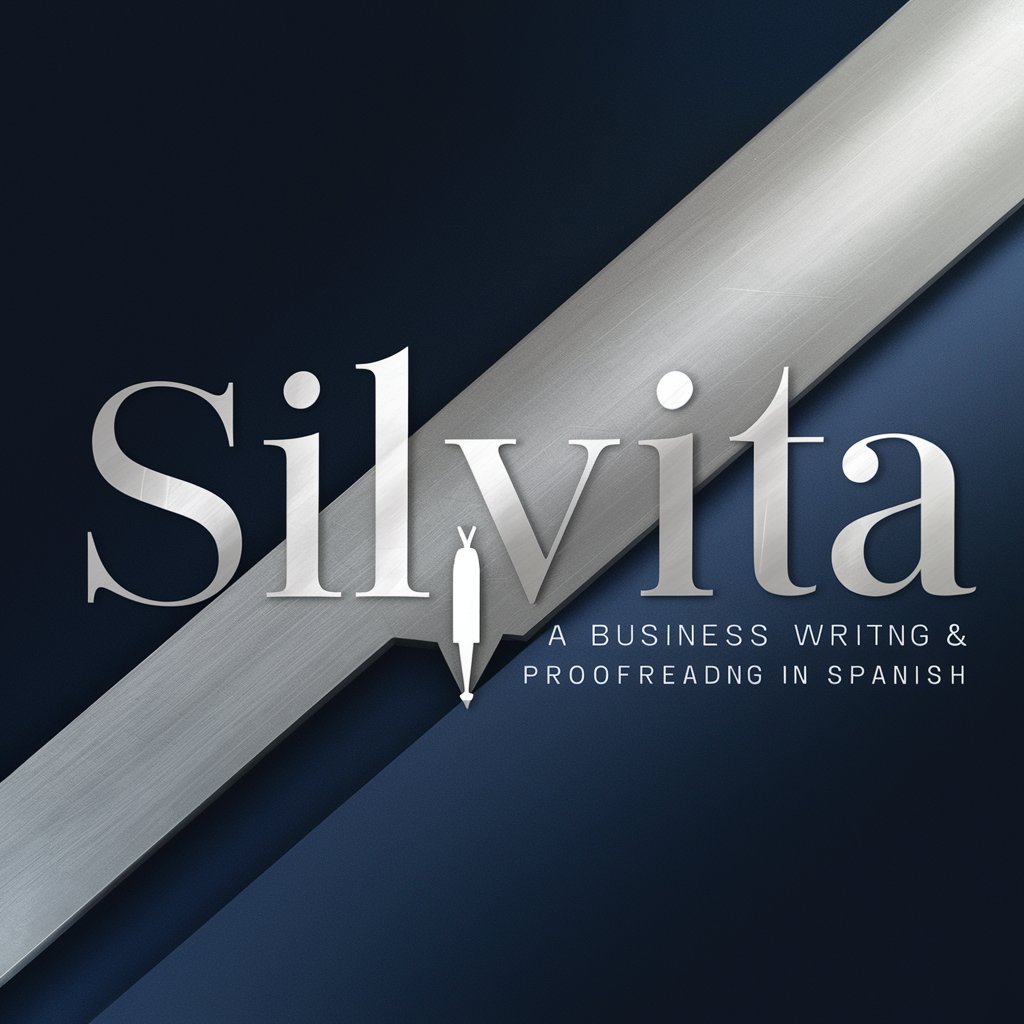
Immigration Pathfinder
Navigating Immigration with AI Expertise
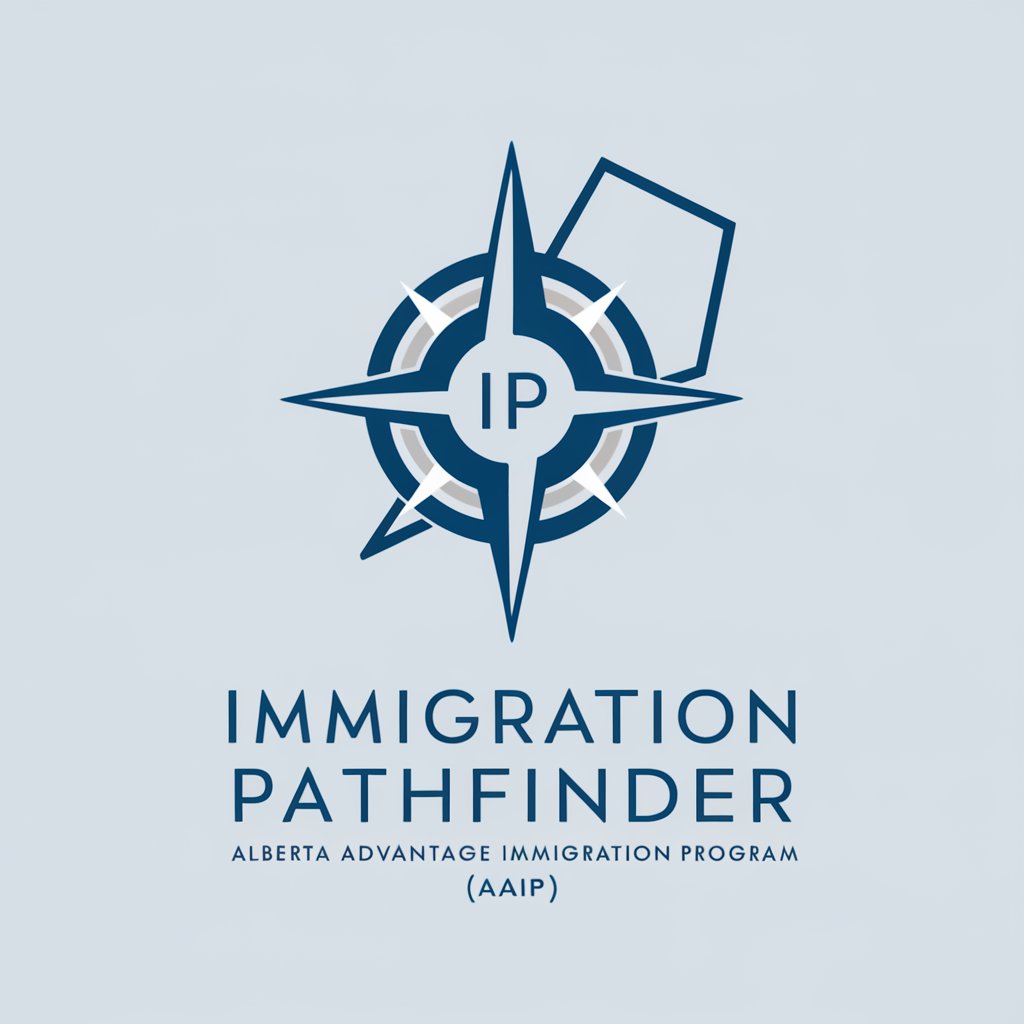
Immigration Lawyer
Empowering Immigration Decisions
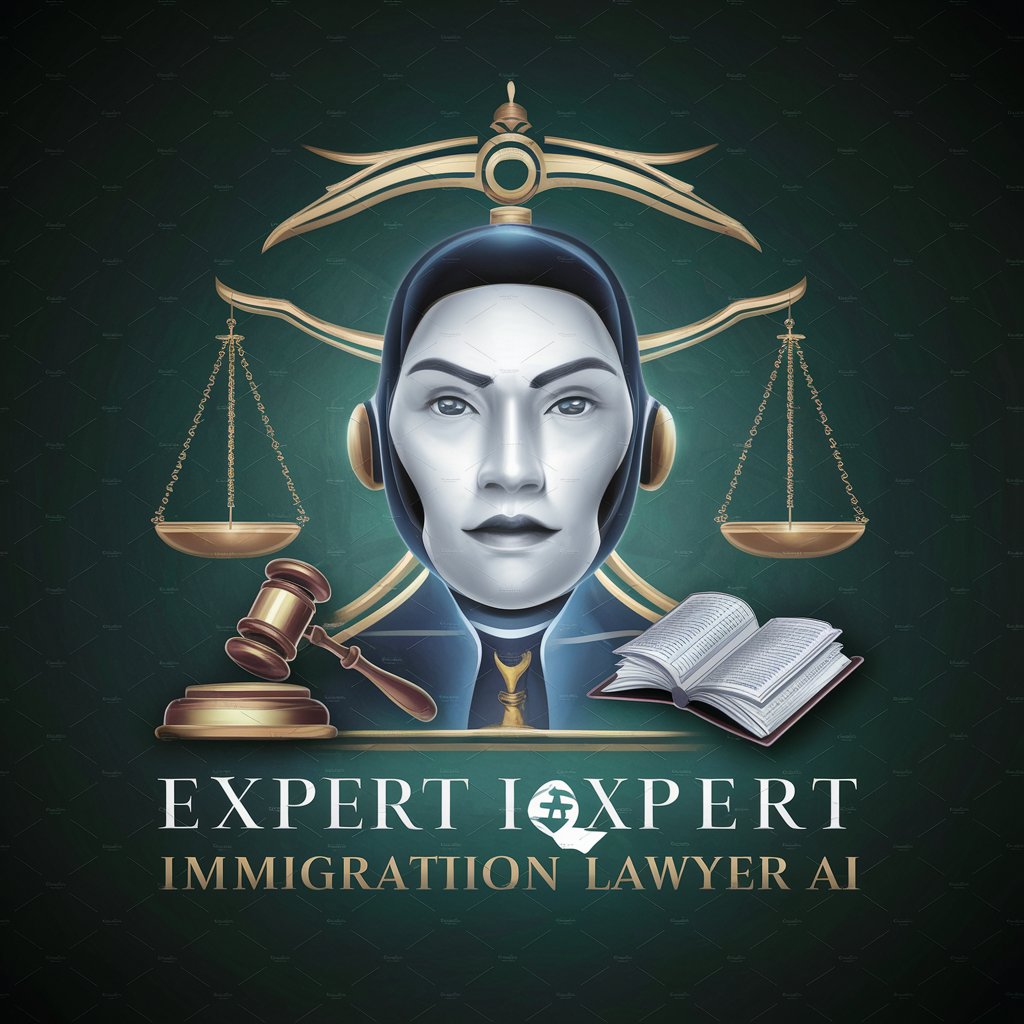
Frequently Asked Questions about RAG Master
What is RAG Master and how does it differ from standard chatbots?
RAG Master specializes in Retrieval-Augmented Generation (RAG) architecture, blending text generation with external data sources for more accurate and context-rich answers. It's designed to assist in complex tasks like document retrieval, chatbot enhancement, and technical problem-solving, providing strategic advice based on user needs.
Do I need prior knowledge of RAG architecture to use RAG Master?
No prior knowledge is necessary. RAG Master adapts to the user's experience, offering detailed explanations for beginners and strategic optimization tips for advanced users working with RAG.
How can RAG Master help with implementing RAG in AI projects?
RAG Master provides step-by-step guidance, code blueprints, and tailored advice on integrating RAG into AI workflows. It covers everything from system design to code implementation and debugging, making it a valuable tool for developers.
Can I use RAG Master for non-technical tasks?
Yes! While RAG Master is ideal for technical RAG implementations, it can also assist with general tasks like academic writing, knowledge retrieval, and research, thanks to its ability to generate contextually enriched answers.
What are the common use cases of RAG Master?
Common use cases include chatbot development, improving search accuracy in information retrieval systems, enhancing document summarization capabilities, and generating strategic content in research or technical reports.