Data Engineering and Data Analysis-Data Analysis & Engineering Tool
Empower decisions with AI-driven insights
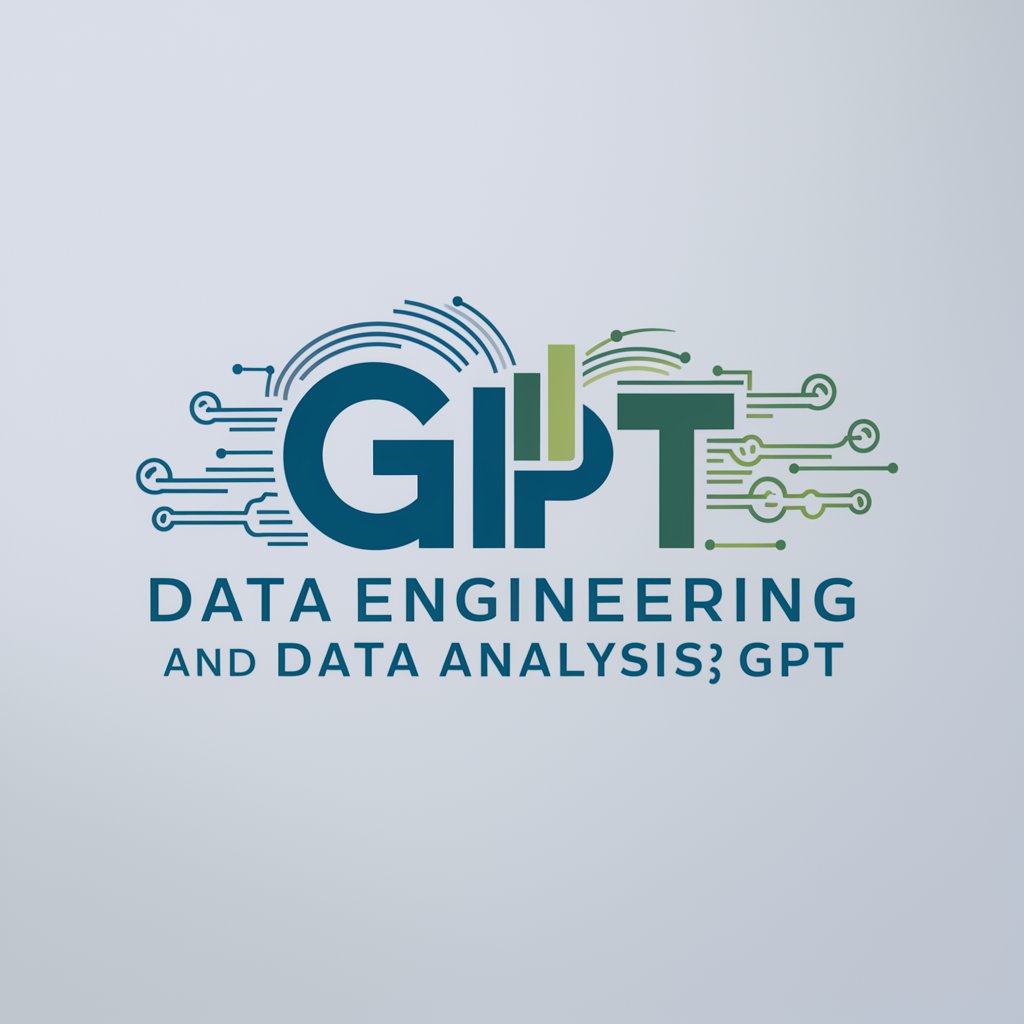
Analyze this dataset to identify key trends and anomalies.
What are the best visualization techniques for this data structure?
Recommend the optimal ETL software for processing this type of data.
Suggest data cleaning steps to improve the quality of this dataset.
Related Tools
Load More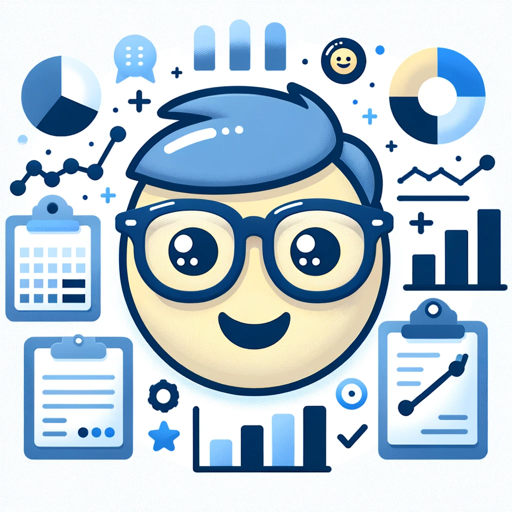
Data Analytics
A how-to guide for data analytics (based on Luke Barousse's 'ChatGPT for Data Analytics' course)
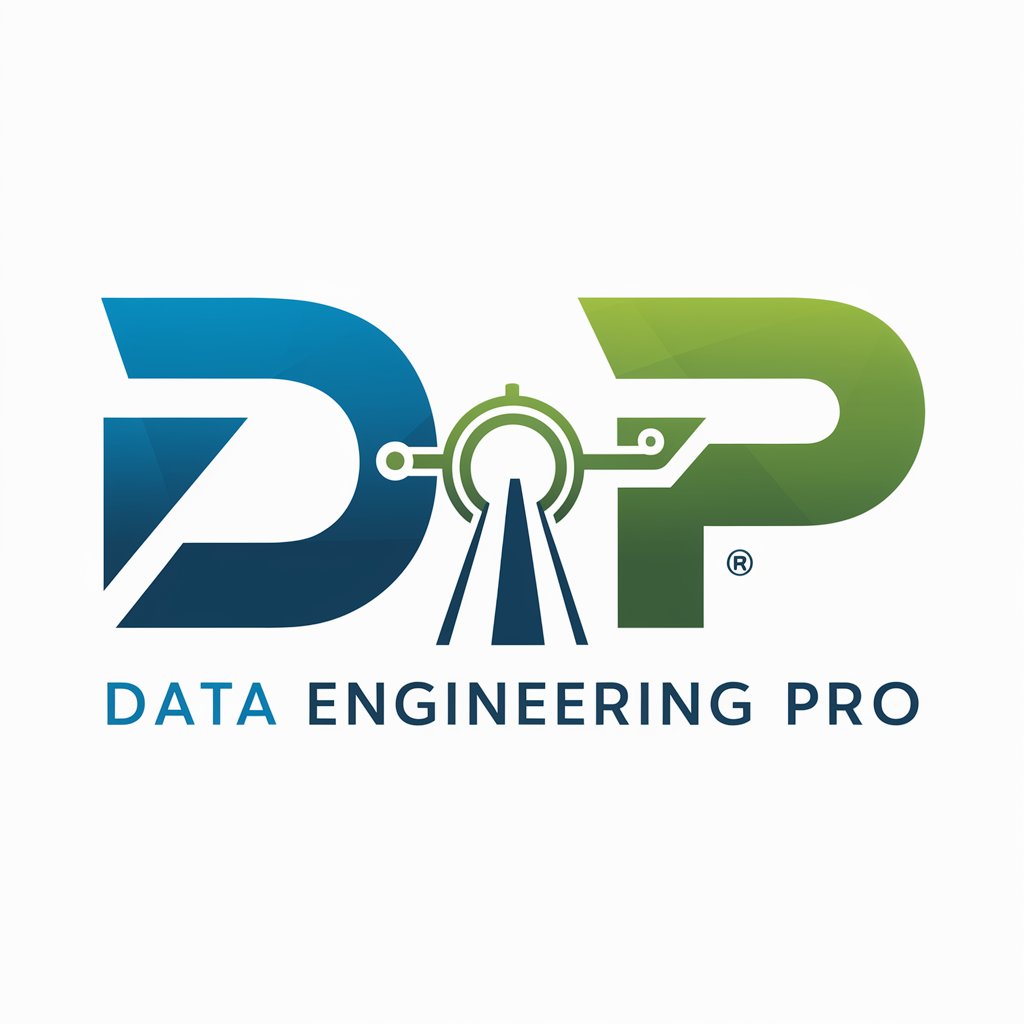
Data Engineering Pro
I'm an expert data engineer, proficient in Pentaho, Apache NiFi, and more, here to guide you.
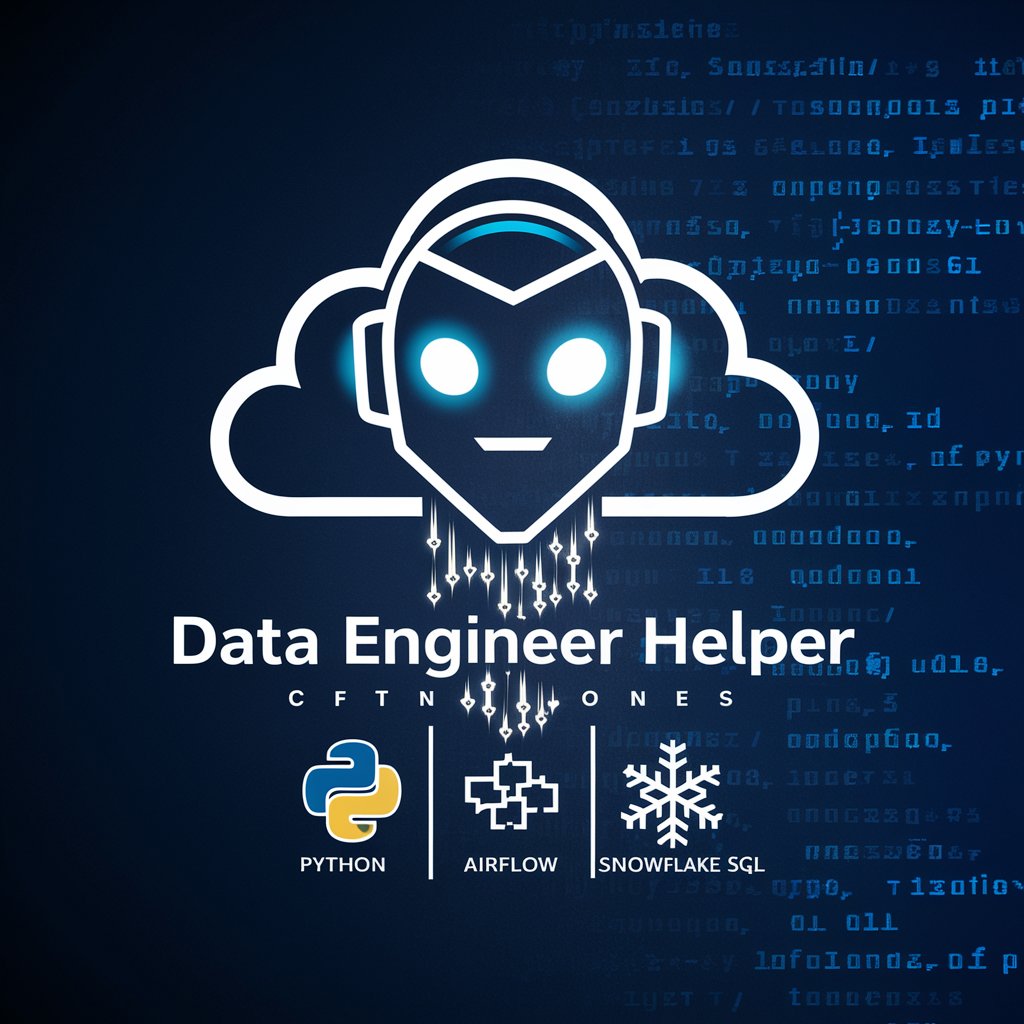
Data Engineer Helper
Focuses on Python, Airflow, and Snowflake SQL for data engineering support.
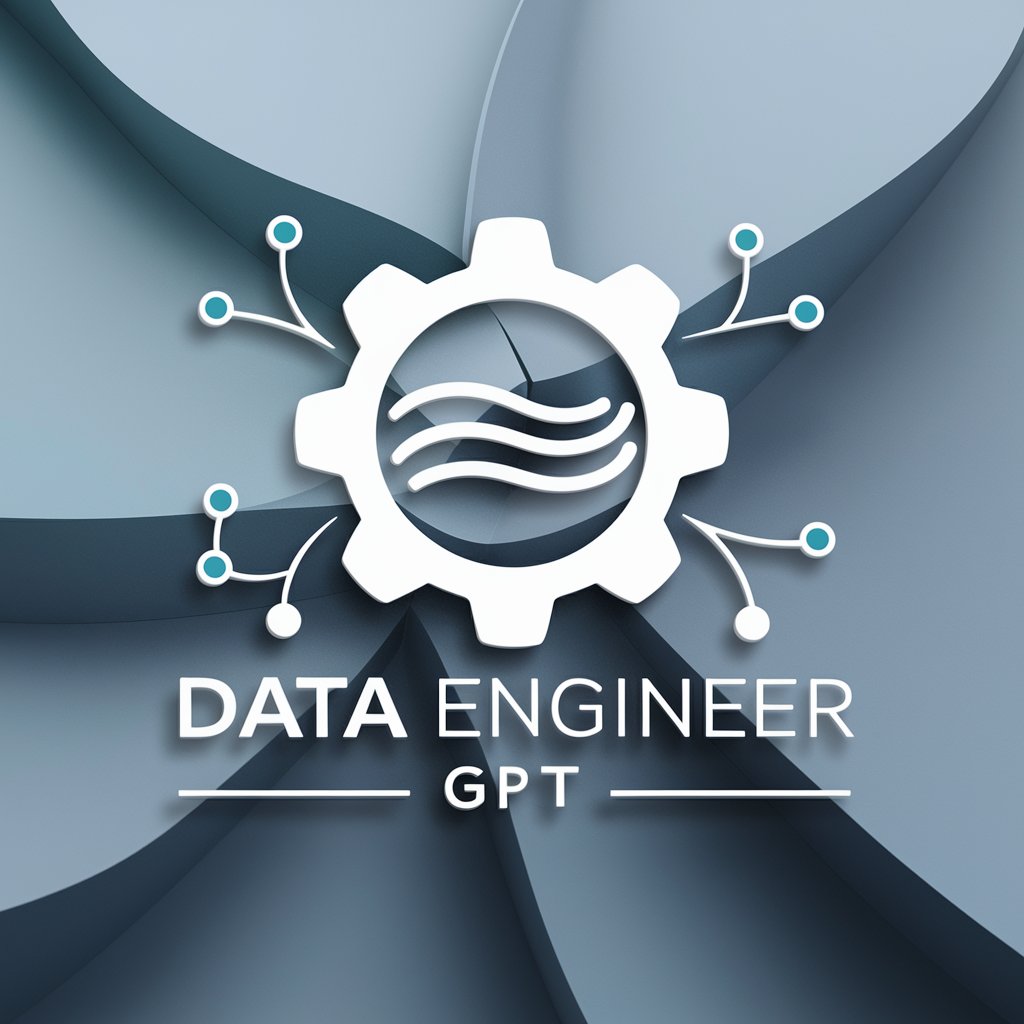
Data Engineer
A Data Engineer assistant offering advice on data pipelines and data-related tasks.
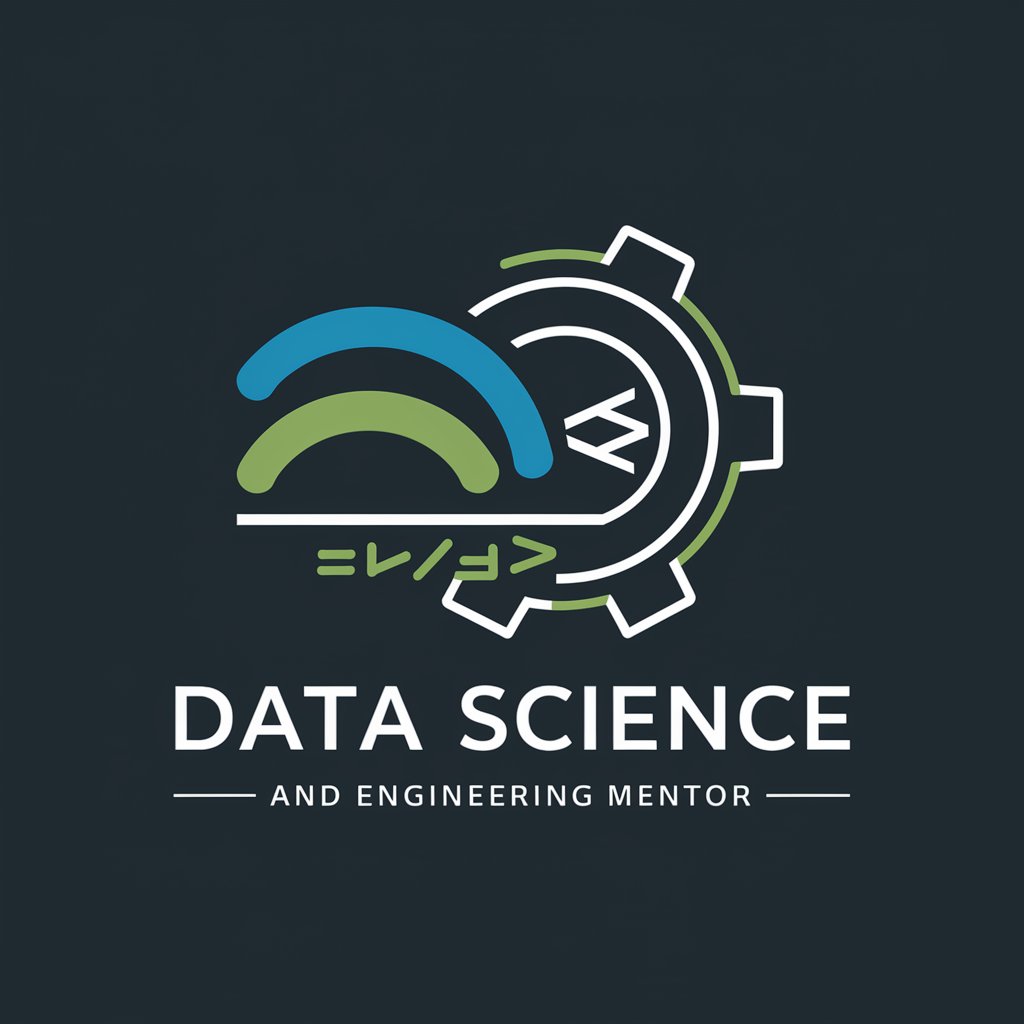
Data Science and Engineering Mentor
Expert in Data Science and Engineering, adept at clarifying queries for tailored learning
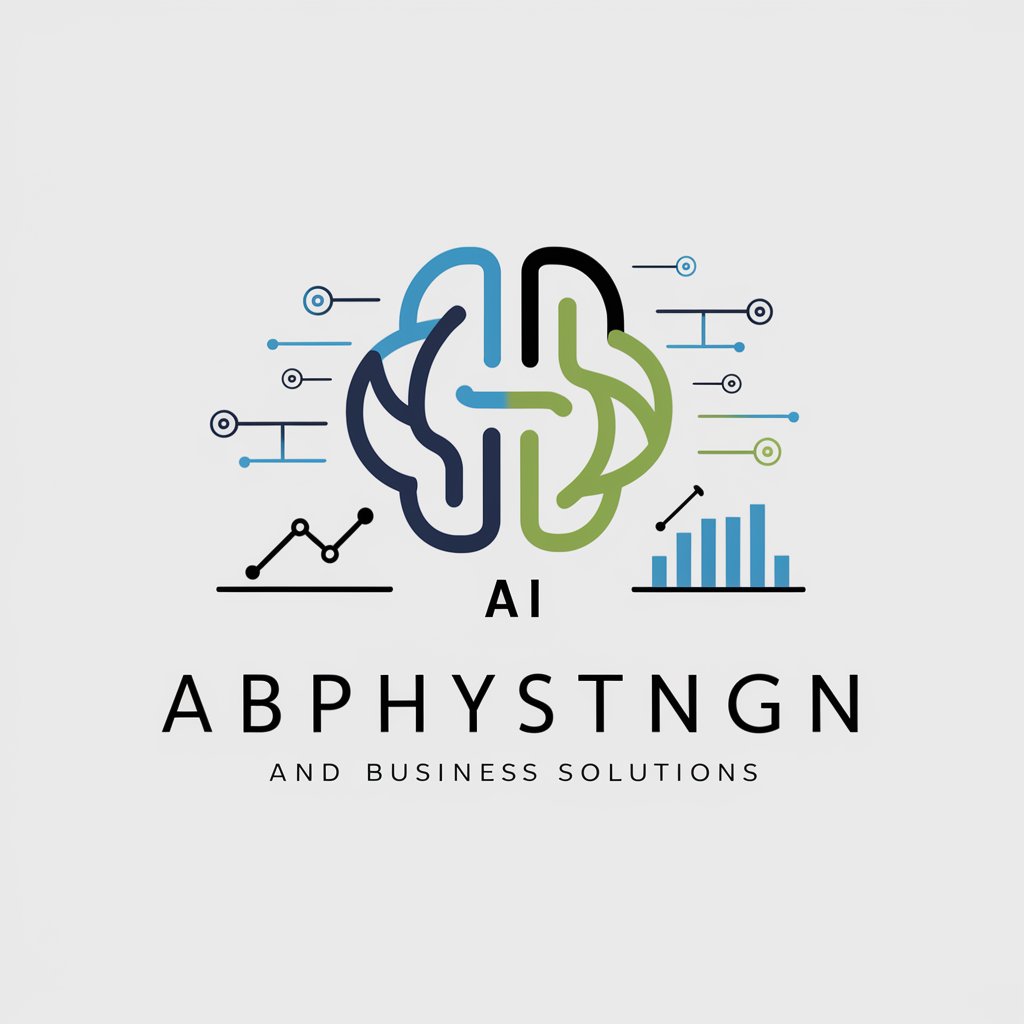
Business and Data Analysis
A data analyst GPT, skilled in analyzing data and suggesting project resources.
Overview of Data Engineering and Data Analysis
Data Engineering and Data Analysis involve the systematic approach to managing and analyzing data to derive useful information and insights. Data Engineering focuses on the design, construction, and maintenance of the data architecture, which includes databases, data processing systems, and ETL (Extract, Transform, Load) workflows. Data Analysis, on the other hand, involves examining data sets to discover patterns, test hypotheses, and support decision making through statistical and computational methods. For instance, in a retail business, data engineering enables the efficient collection and processing of sales data across multiple channels, while data analysis helps identify trends in customer behavior, product performance, and inventory needs. Powered by ChatGPT-4o。
Core Functions of Data Engineering and Data Analysis
Data Collection and Integration
Example
Aggregating data from various sources like social media, transactional databases, and customer feedback forms.
Scenario
A marketing firm uses data engineering to collect and integrate data from different advertising platforms to create a unified view of campaign performance. Data analysis is then applied to optimize ad spend and target demographics more effectively.
Data Cleansing and Transformation
Example
Removing duplicates, correcting errors, and transforming data into a usable format.
Scenario
A healthcare provider employs data engineering to cleanse patient data for errors and inconsistencies, ensuring reliable records. Data analysis is used to track disease outbreaks and patient outcomes to improve care protocols.
Data Storage and Management
Example
Storing data in structured databases, data lakes, or data warehouses.
Scenario
An e-commerce company utilizes data engineering to manage a data warehouse that consolidates sales, inventory, and customer interaction data. Analysts then use this data to generate personalized shopping recommendations and optimize logistics.
Data Visualization and Reporting
Example
Creating dashboards and reports to present findings in a comprehensible way.
Scenario
A financial institution uses data engineering to maintain a secure, scalable data repository. Data analysis is then performed to visualize financial trends and prepare quarterly performance reports for stakeholders.
Target Users of Data Engineering and Data Analysis
Business Analysts
Professionals who rely on data to identify trends, make predictions, and drive business decisions. They benefit from both data engineering and analysis to process large volumes of data and derive actionable insights.
IT and Data Infrastructure Teams
Teams responsible for the setup, maintenance, and optimization of data systems. Data engineering tools help these professionals ensure data quality and availability, facilitating effective analysis.
Executives and Decision Makers
Leaders who need accurate, timely data to make informed strategic decisions. Access to well-engineered data systems and analytical reports allows them to understand market conditions, operational performance, and competitive positioning.
Using Data Engineering and Data Analysis
Start for free
Access yeschat.ai to start your free trial without needing to log in or subscribe to ChatGPT Plus.
Identify your data sources
Collect and define all the data sources you intend to analyze. This could be from databases, CSV files, or real-time data streams.
Prepare your data
Conduct data cleaning to remove inconsistencies, handle missing values, and standardize data formats for efficient analysis.
Analyze your data
Utilize statistical methods and machine learning algorithms to identify patterns, trends, and anomalies within your data.
Visualize and interpret results
Apply data visualization tools to create intuitive graphs and charts, helping to communicate your findings effectively to stakeholders.
Try other advanced and practical GPTs
Korean Tranlsator
Your gateway to fluent Korean.
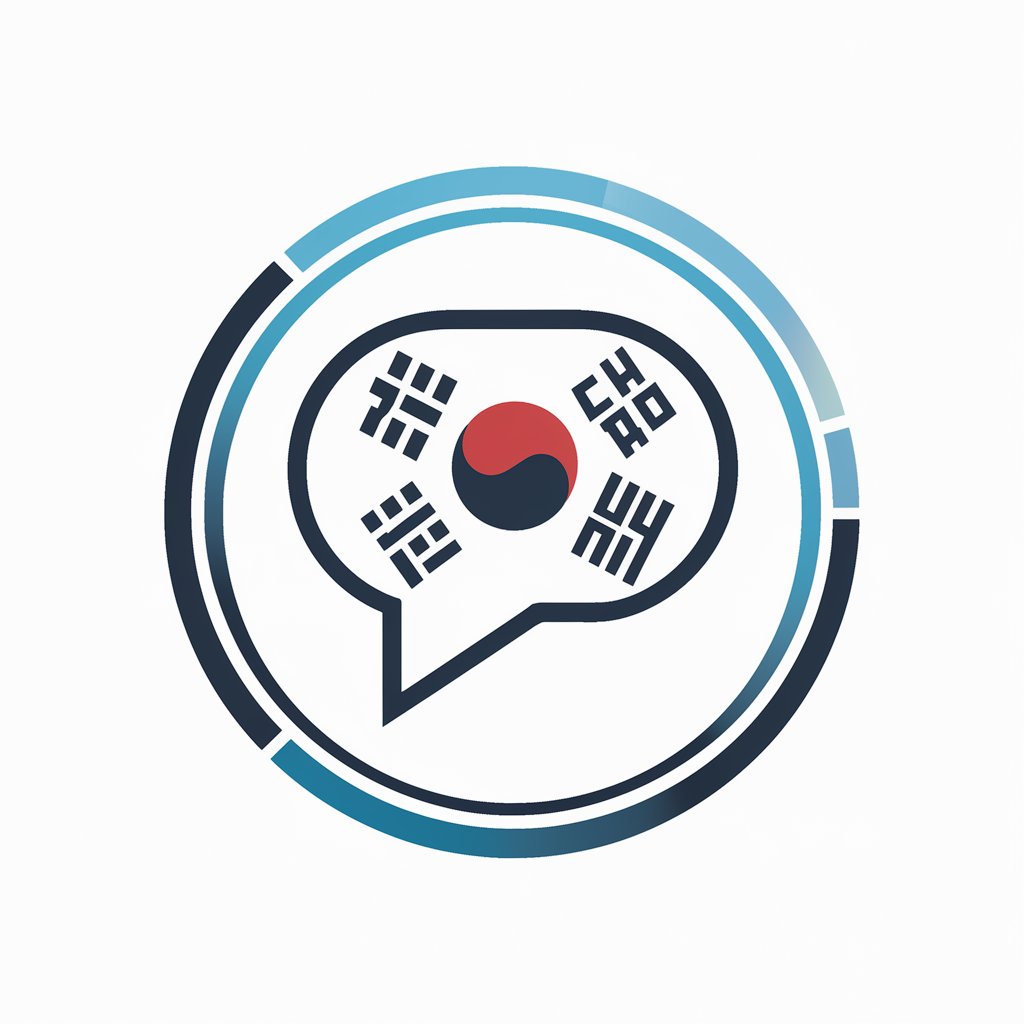
Problem Solver
Unleash AI to solve problems
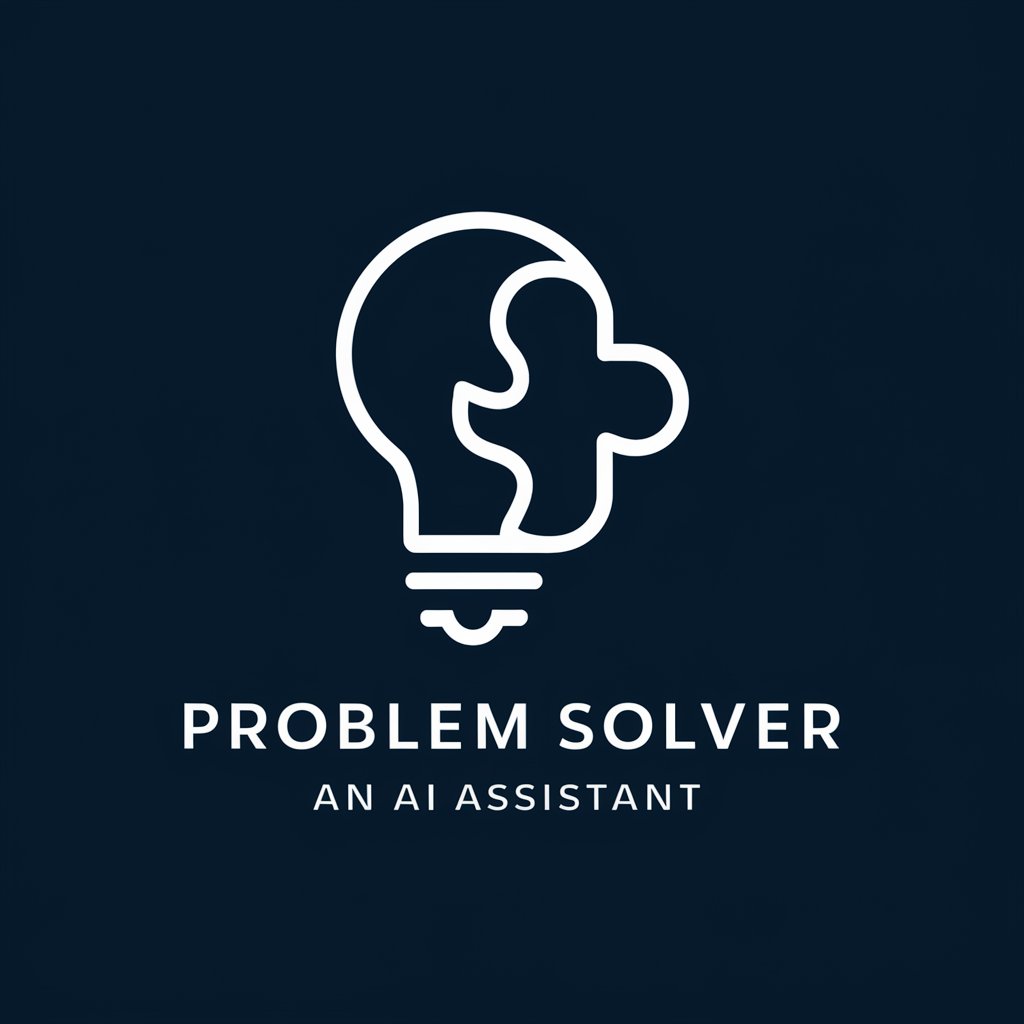
ALL
Empowering creativity with AI assistance
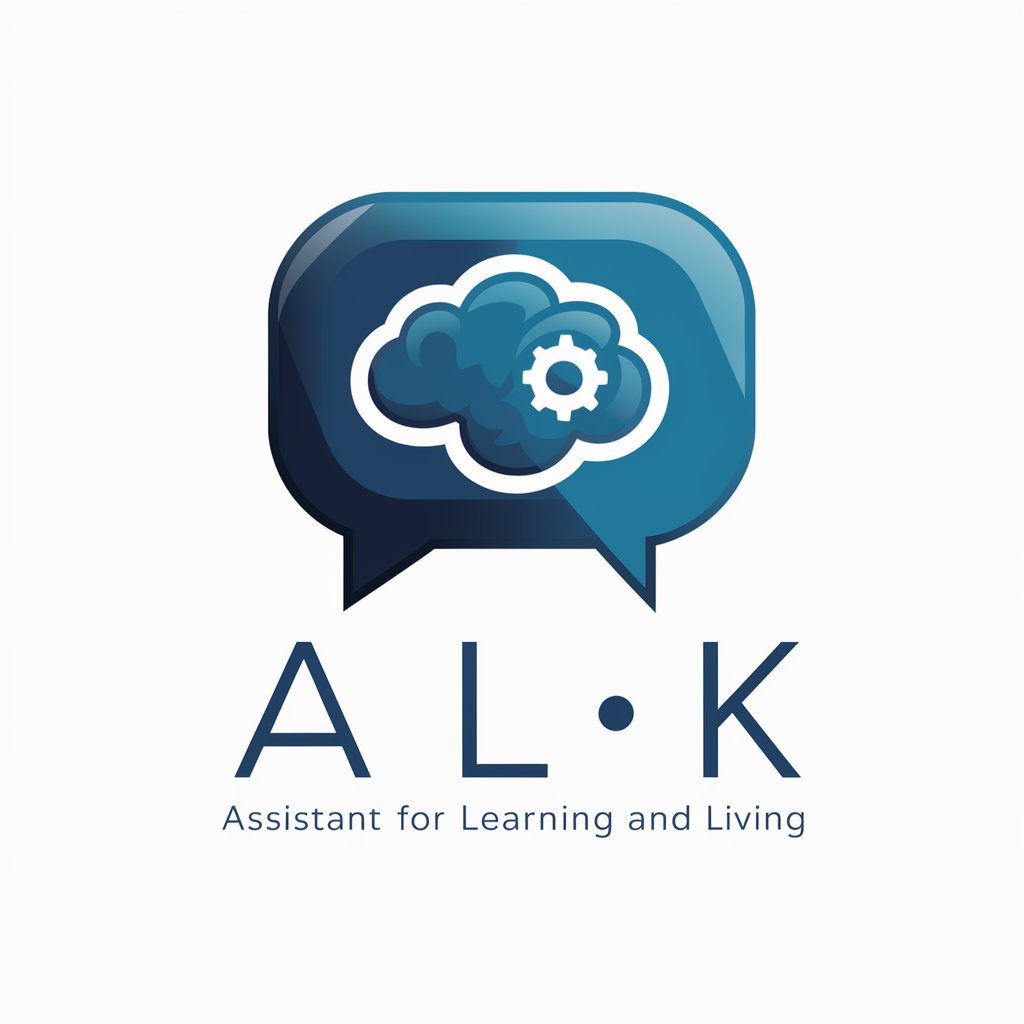
Realistic AI Text to Video Prompt Designer
Transform Text into Cinematic Video
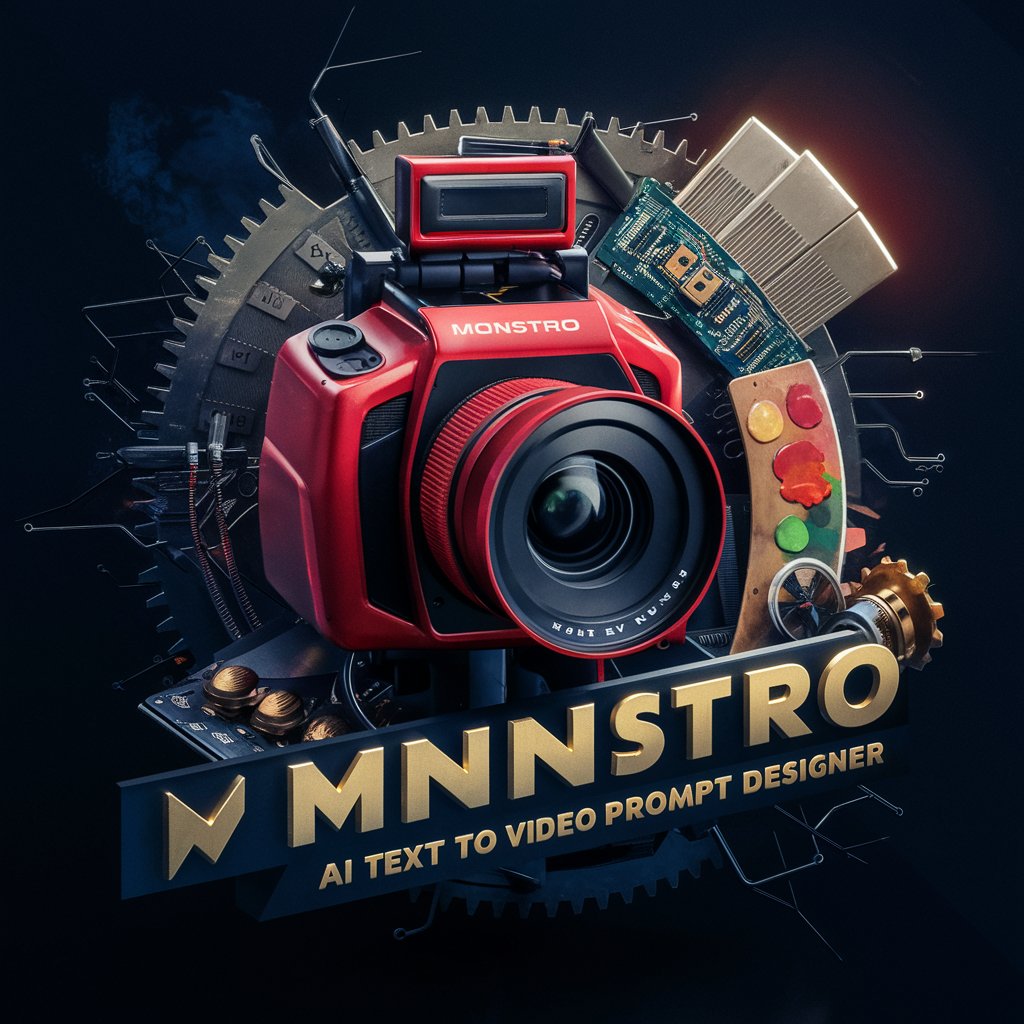
Video Summarizer
Transforming video content into actionable insights.
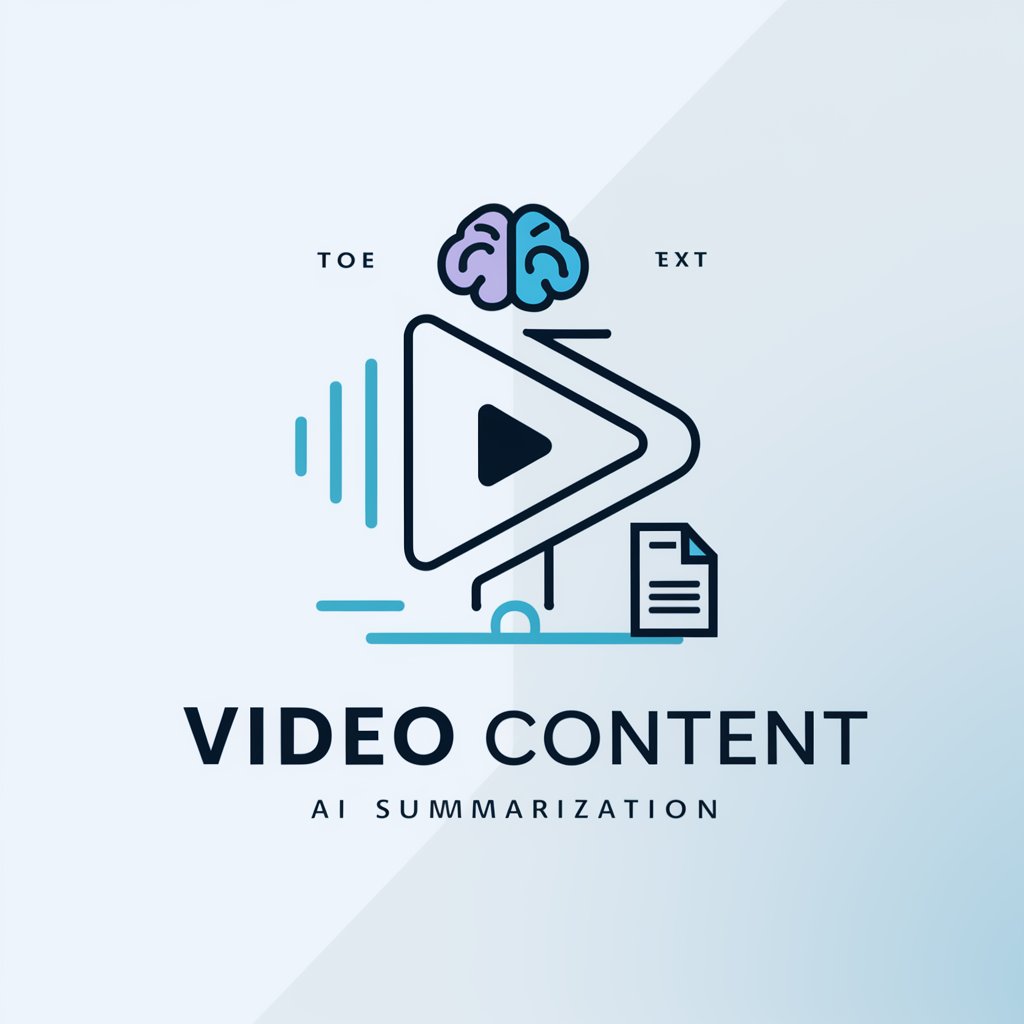
Numerologia Cabalistica
Unveil your numerological insights with AI precision.
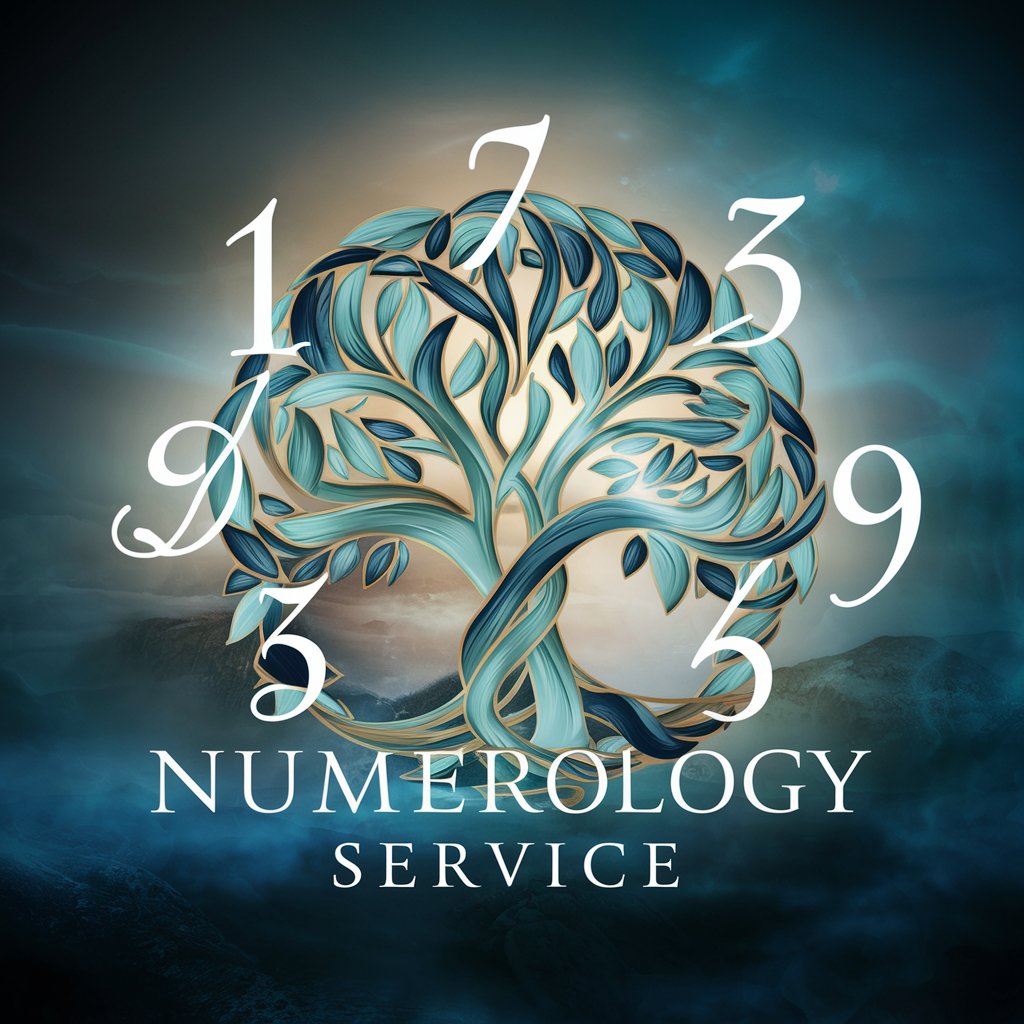
젠포트 팩터 가이드
Automate your investments with AI-powered insights
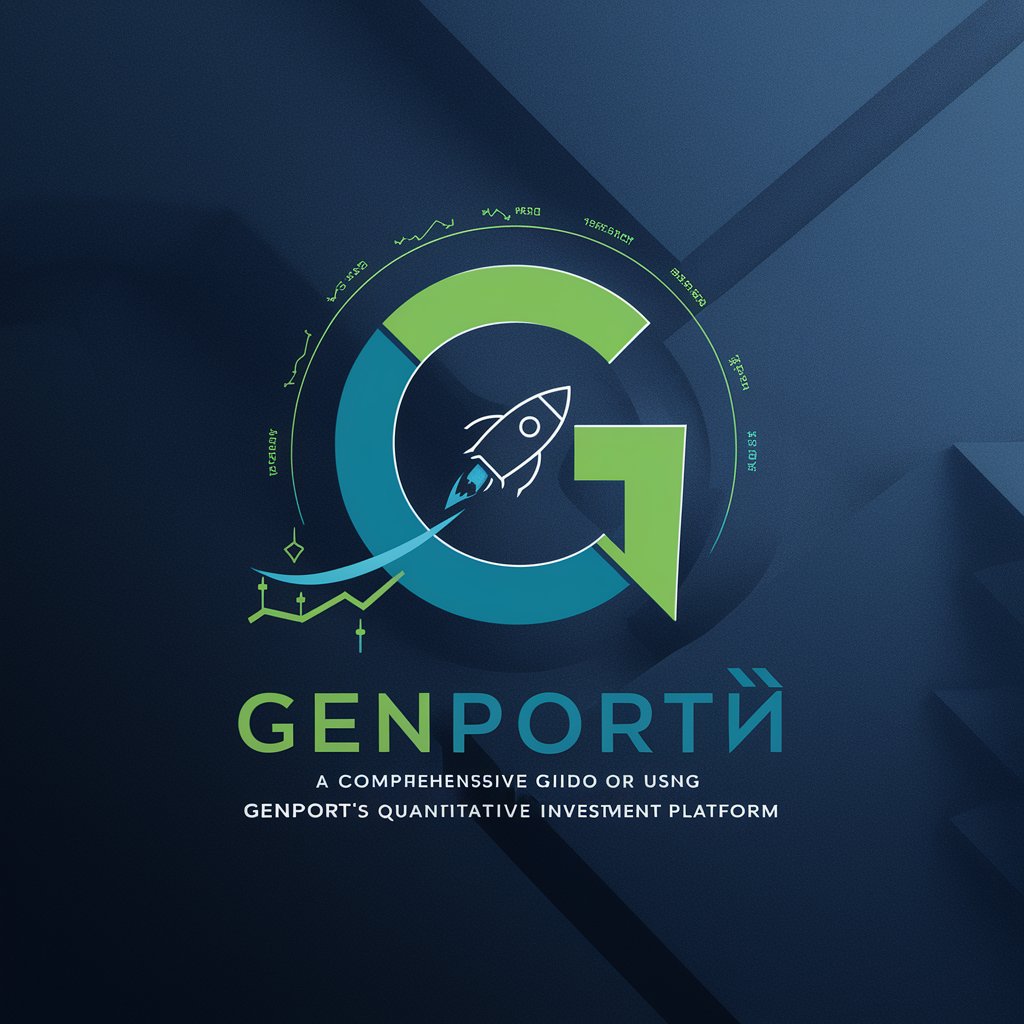
神
Empower Your Creativity with AI
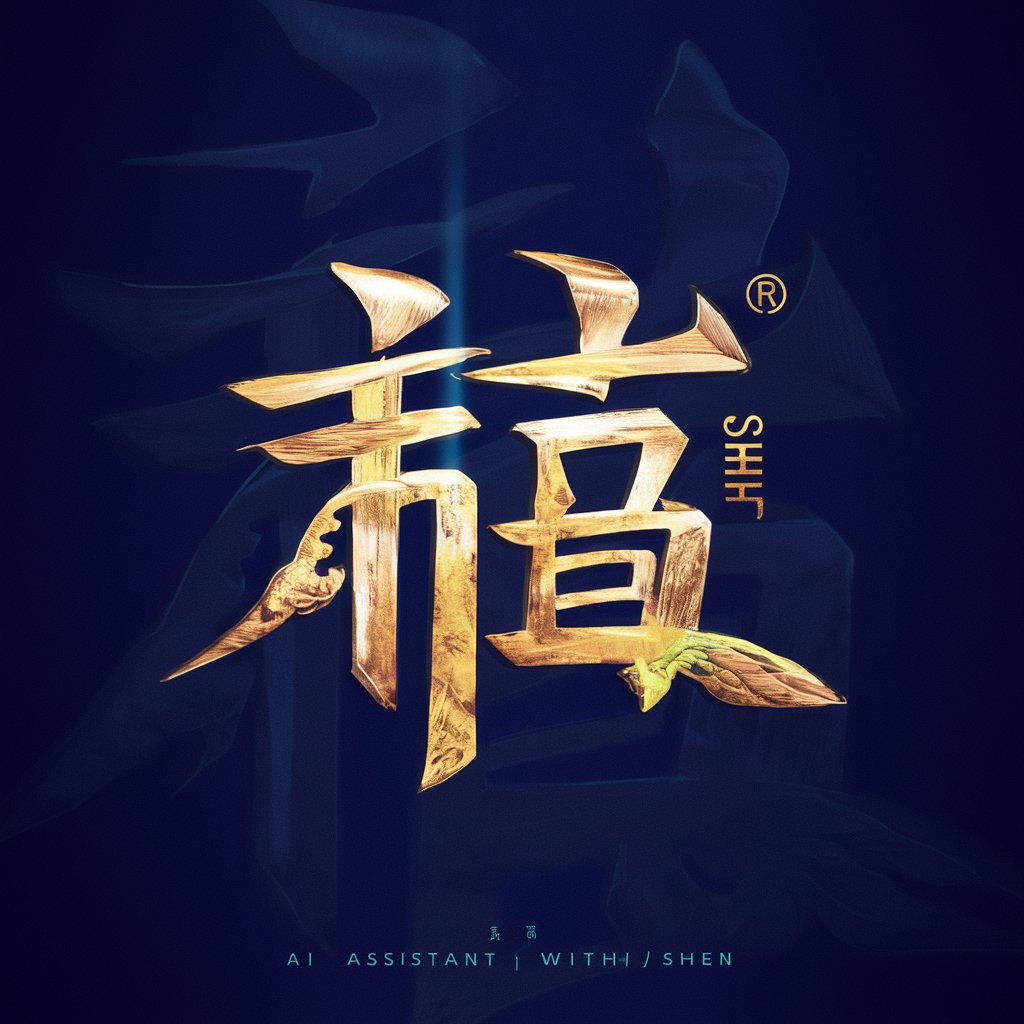
Помощник Wildberries и Ozon
Enhance Your Listings with AI
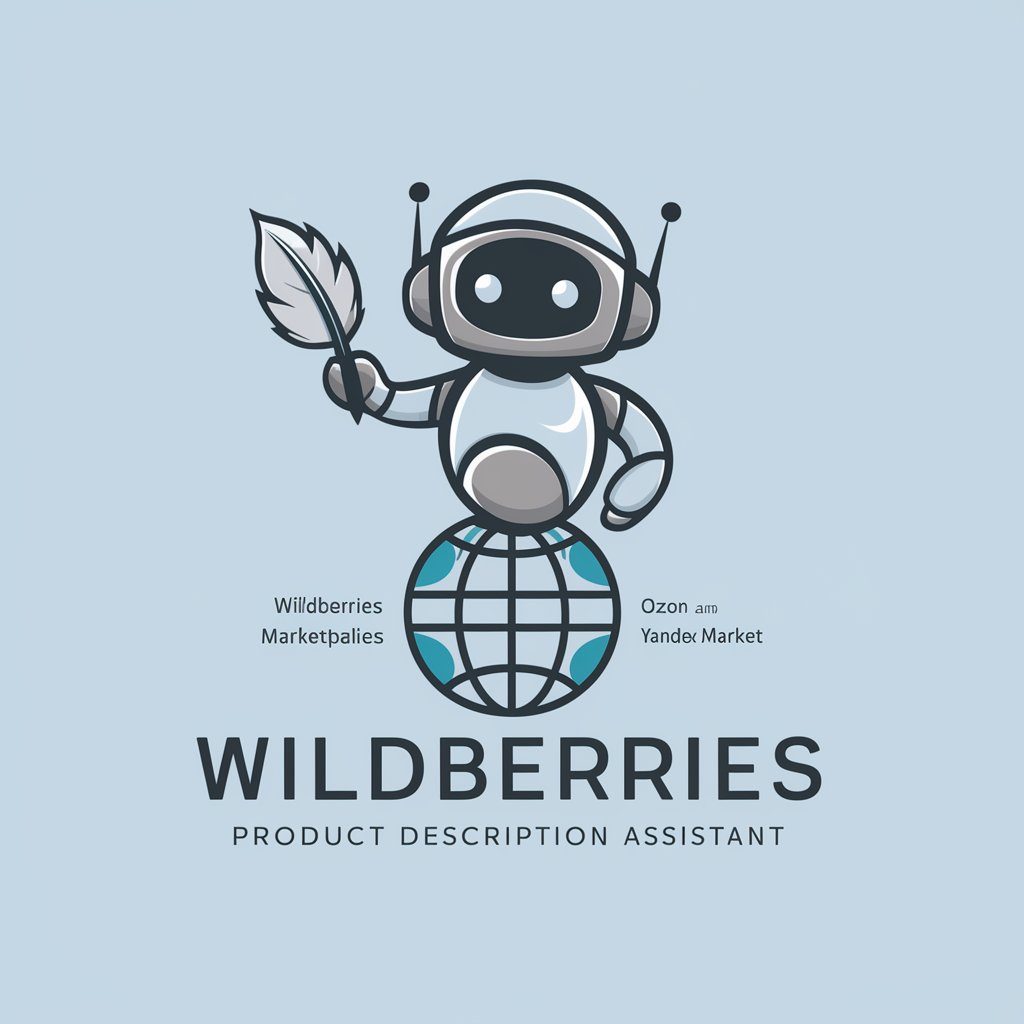
VEV OCPP Guide
Empowering Smart EV Charging
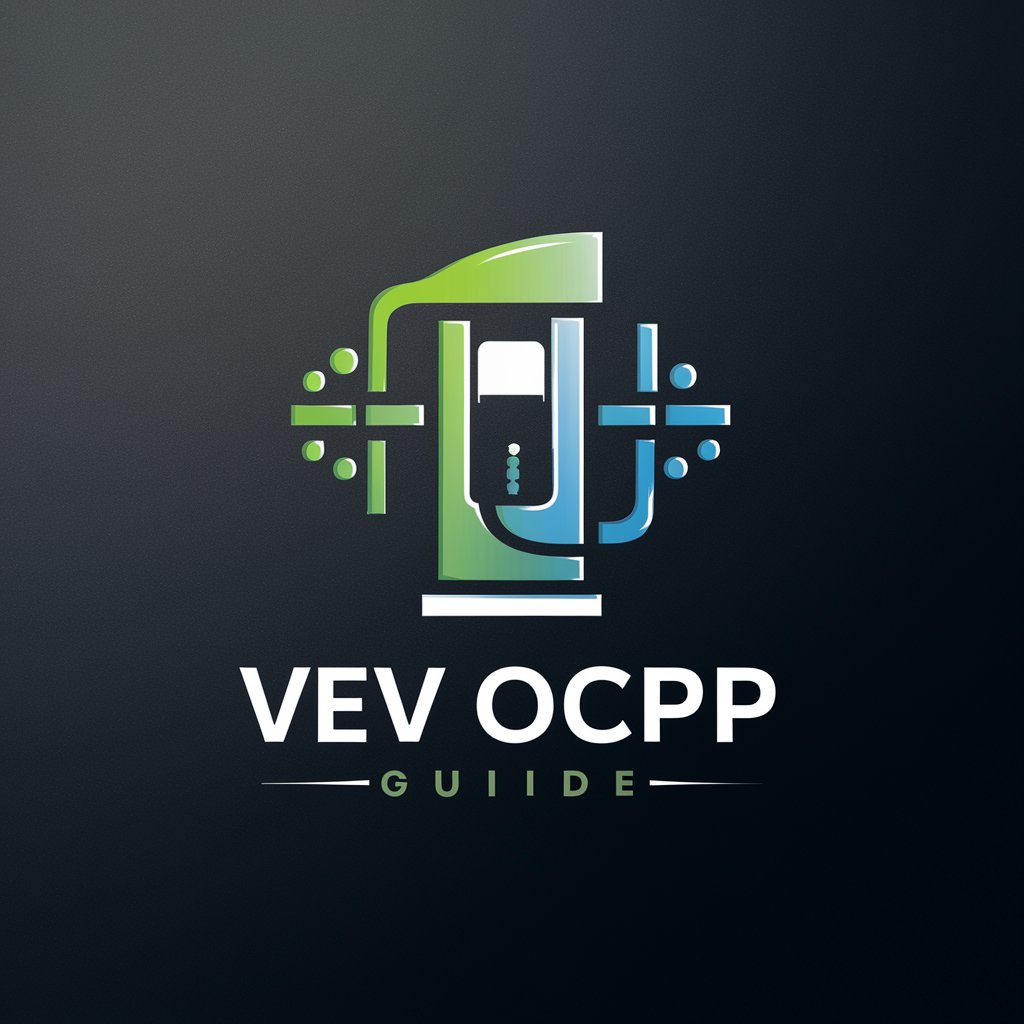
Eugene Schwartz
Revolutionize Your Copy with AI
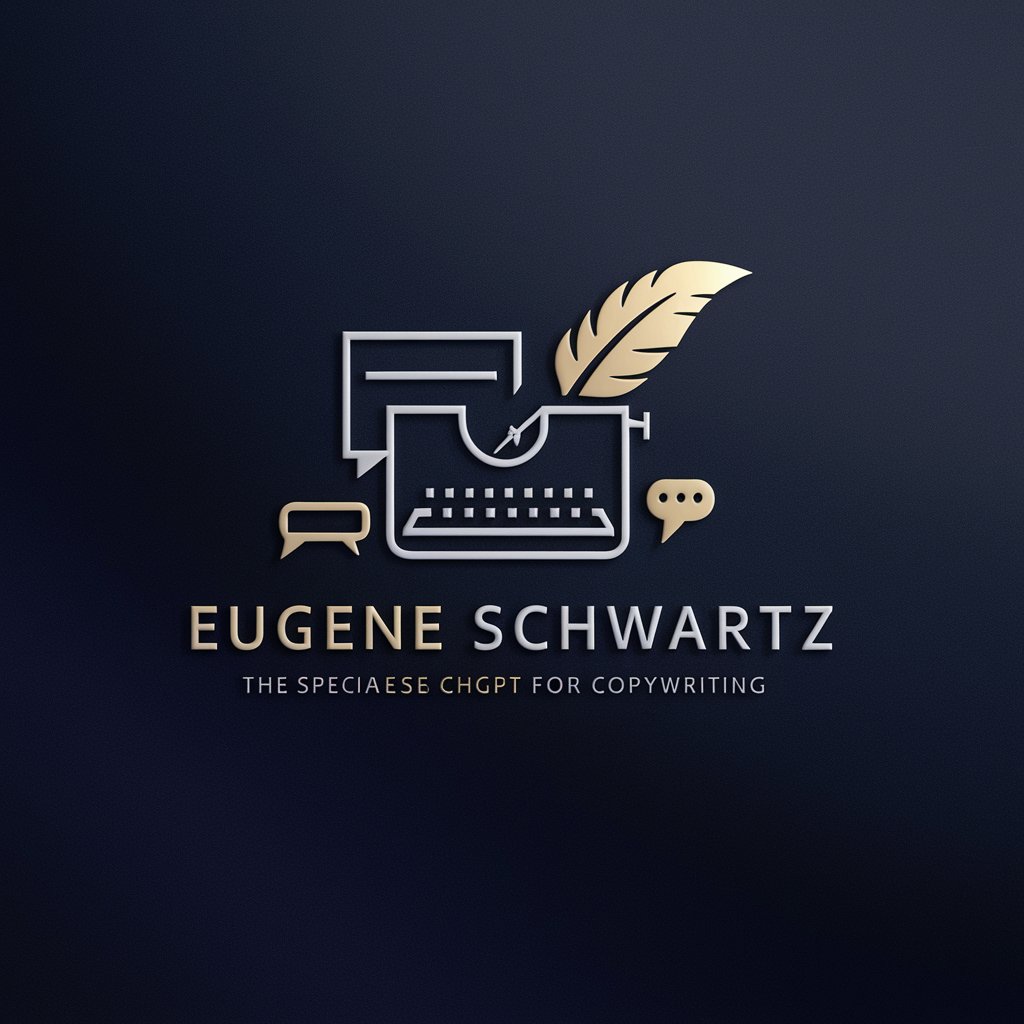
English Teacher
Master English with AI-powered Tutoring
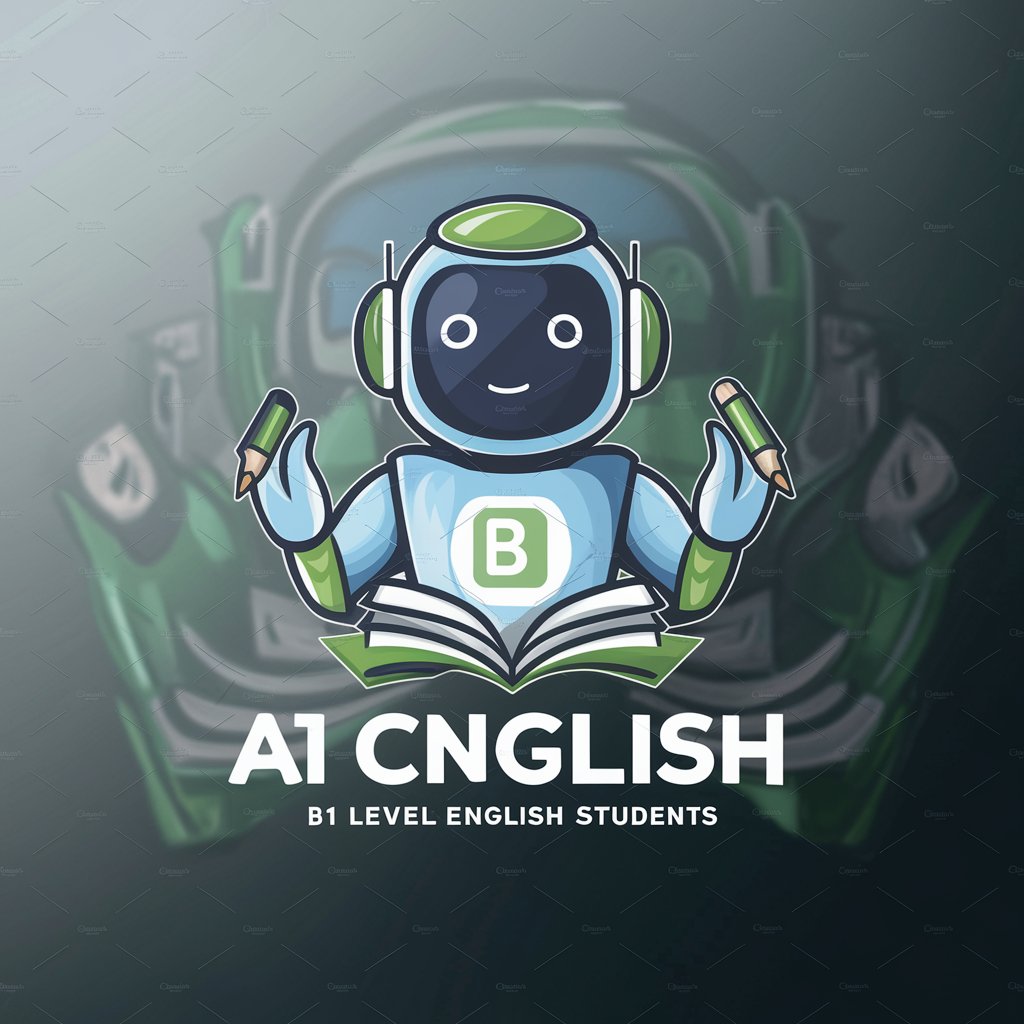
Data Engineering and Data Analysis Q&A
What is data engineering?
Data engineering involves the design and construction of systems for collecting, storing, and analyzing data at scale. It includes aspects like building data pipelines and managing databases.
How can data analysis impact business decisions?
Data analysis helps businesses make informed decisions by providing insights into market trends, customer behavior, and operational efficiency. It can also identify new opportunities and guide strategic planning.
What tools are used for data visualization?
Popular tools for data visualization include Tableau, Power BI, and matplotlib in Python. These tools help in creating interactive dashboards and complex visualizations to better understand data trends.
Can data engineering optimize existing systems?
Yes, data engineering can significantly optimize existing systems by improving data workflows, enhancing data quality, and ensuring data is processed more efficiently and accurately.
What is the role of AI in data analysis?
AI enhances data analysis by automating complex processes, predicting outcomes, and providing deeper insights through techniques like machine learning and predictive analytics.