Synthetic Data - AI-Generated Synthetic Data
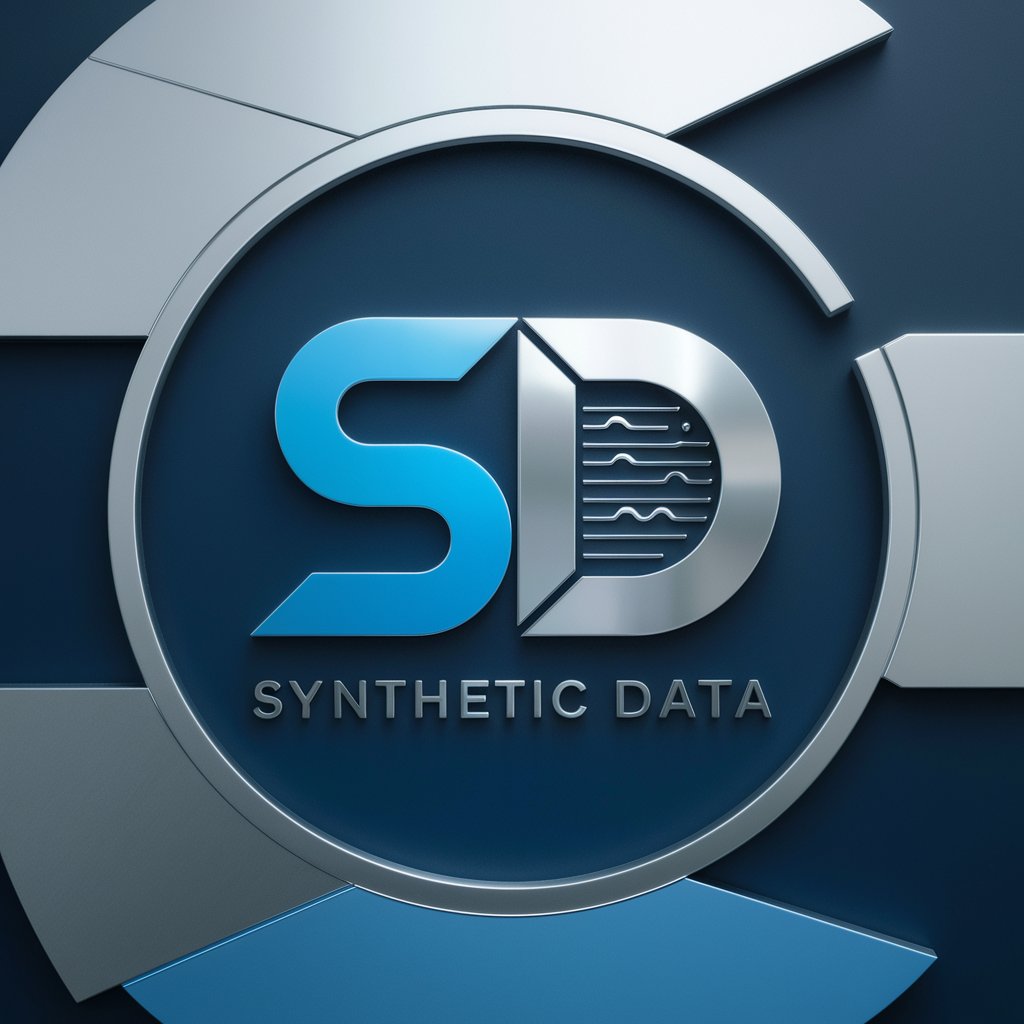
Welcome to Synthetic Data, your AI-powered data generation solution.
Experience the future of data with AI-generated synthetic intelligence.
Generate synthetic data for healthcare analytics...
Create a dataset for customer behavior analysis...
Simulate financial transactions for risk assessment...
Produce synthetic data for machine learning training...
Get Embed Code
Introduction to Synthetic Data
Synthetic data refers to artificially generated data that mimics the statistical properties of real-world data but does not contain any real information about individuals or entities. It is created using algorithms or models to simulate data points that resemble the original dataset. The primary purpose of synthetic data is to maintain privacy and confidentiality while still allowing for analysis, testing, and development in various applications. Powered by ChatGPT-4o。
Main Functions of Synthetic Data
Privacy Preservation
Example
Generating synthetic data to replace sensitive information in datasets used for analysis or training models.
Scenario
In healthcare, synthetic data can be used to develop machine learning models without exposing patient information, ensuring compliance with data privacy regulations like HIPAA.
Data Augmentation
Example
Creating additional training data to improve model performance by generating synthetic samples similar to existing ones.
Scenario
In fraud detection, synthetic data can be generated to balance imbalanced datasets, providing more accurate predictions and reducing false positives.
Testing and Validation
Example
Using synthetic data to validate algorithms, software, or systems in scenarios where real data is scarce or difficult to obtain.
Scenario
In autonomous vehicle development, synthetic data can simulate various driving conditions and scenarios, allowing engineers to test the system's performance without real-world risks.
Anonymization and De-identification
Example
Replacing identifiable information with synthetic equivalents to protect privacy in research or data sharing.
Scenario
In social science research, synthetic data can be used to anonymize survey responses, enabling open access to datasets while safeguarding respondents' identities.
Ideal Users of Synthetic Data Services
Data Scientists and Analysts
Data scientists and analysts who work with sensitive data and need to perform analysis, model training, or algorithm development while complying with privacy regulations. They benefit from synthetic data for testing models, training algorithms, and exploring new techniques without accessing real data.
Software Developers
Software developers who require diverse datasets for testing and validation of applications, especially in domains where obtaining real data is challenging or expensive. Synthetic data allows them to simulate various scenarios and edge cases, ensuring robustness and reliability in software systems.
Government Agencies and Research Institutions
Government agencies, research institutions, and organizations conducting studies or experiments that involve sensitive or confidential data. Synthetic data enables them to share datasets publicly, collaborate with other researchers, and facilitate reproducibility in scientific studies while protecting individuals' privacy.
Healthcare Organizations
Healthcare organizations, hospitals, and medical research institutions dealing with patient data. Synthetic data assists in medical research, algorithm development, and training without compromising patient privacy. It ensures compliance with healthcare regulations while advancing medical innovations.
How to Use Synthetic Data
1
Visit yeschat.ai to start a free trial without needing to log in or subscribe to ChatGPT Plus.
2
Explore the available tools and templates to understand the types of synthetic data you can generate.
3
Define the specific requirements and parameters for your data, such as the data type, volume, and complexity.
4
Generate the synthetic data and use it for testing, training, or validating your models and applications.
5
Regularly update your parameters and regenerate data to ensure variety and relevance to current scenarios.
Try other advanced and practical GPTs
Kubernetes
Empowering Kubernetes deployment with AI.
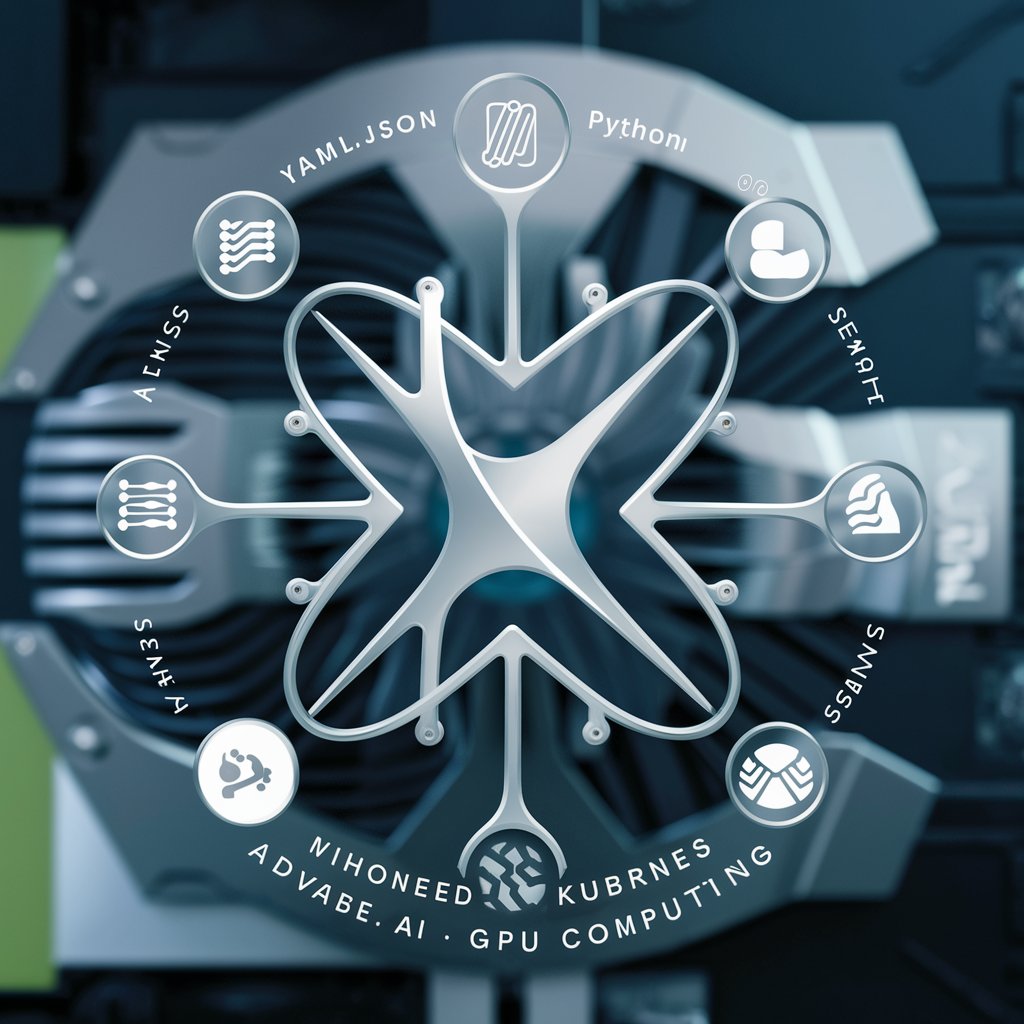
中英翻译
Translate effortlessly with AI precision.
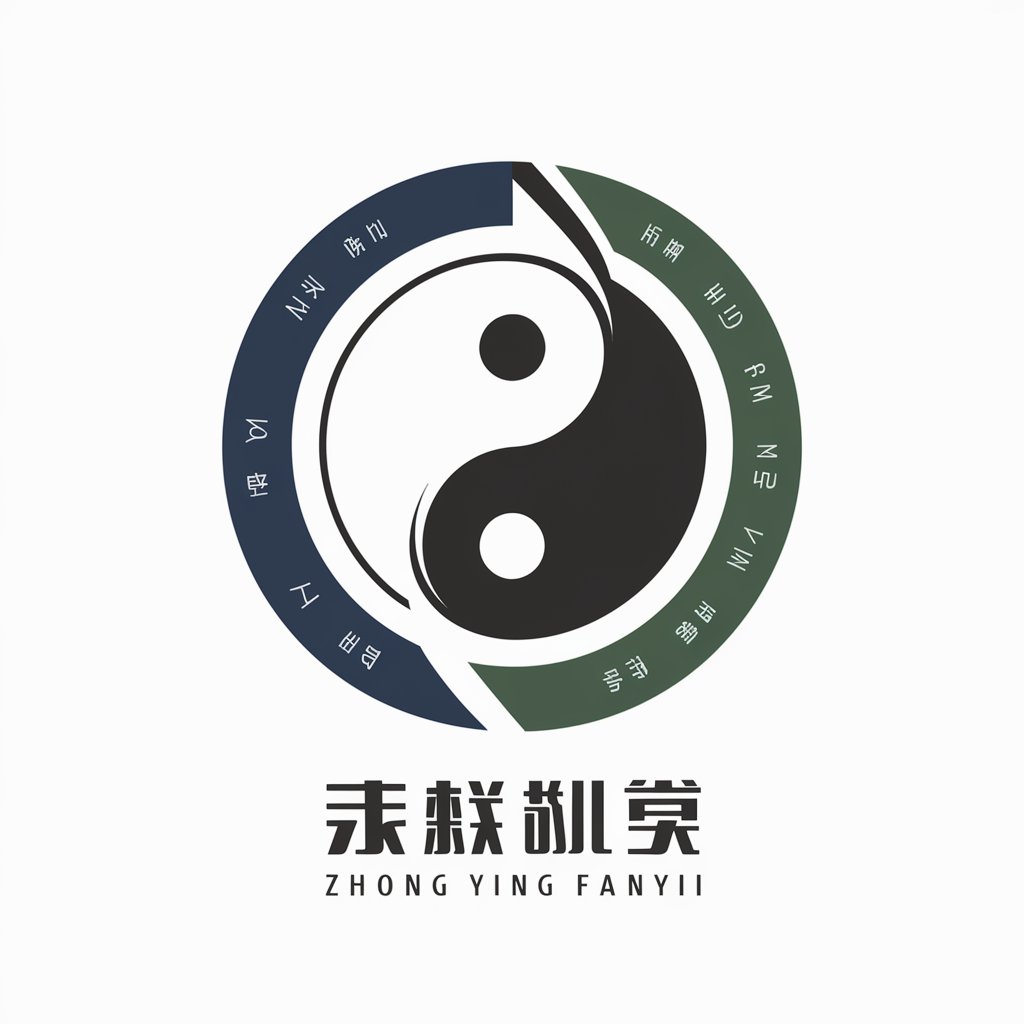
Code Mentor
Empowering programmers with AI-guided solutions.
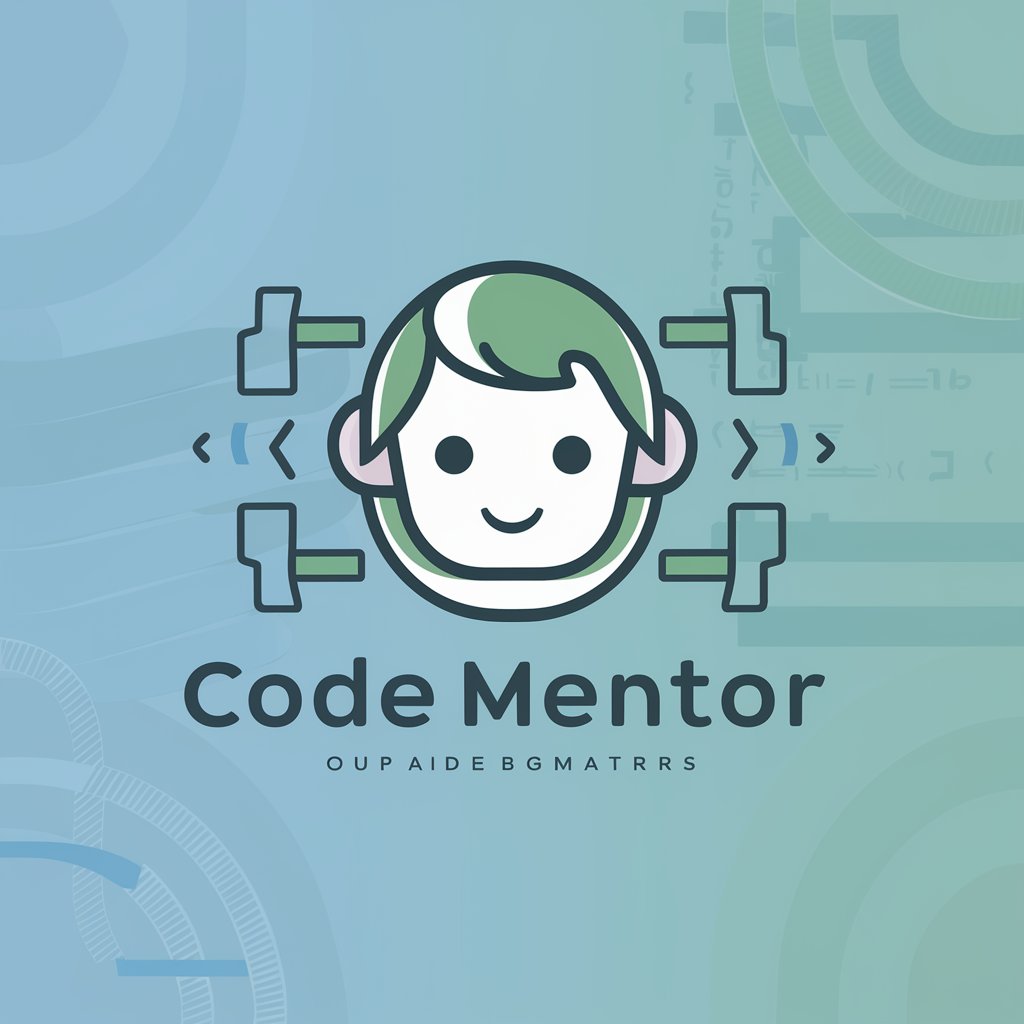
Design to XAML
Turn Designs into XAML Seamlessly
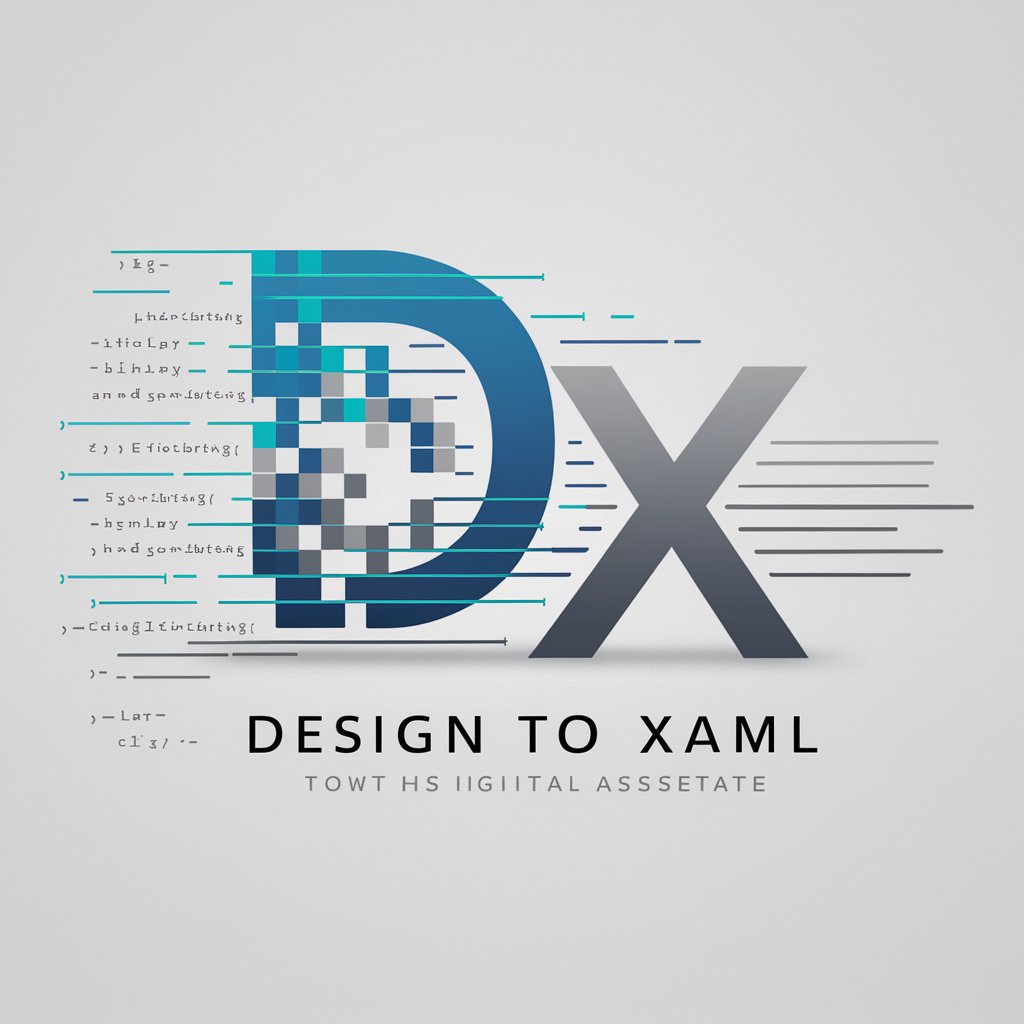
Dynamics and Relativity
Unlock the power of AI in physics solutions.
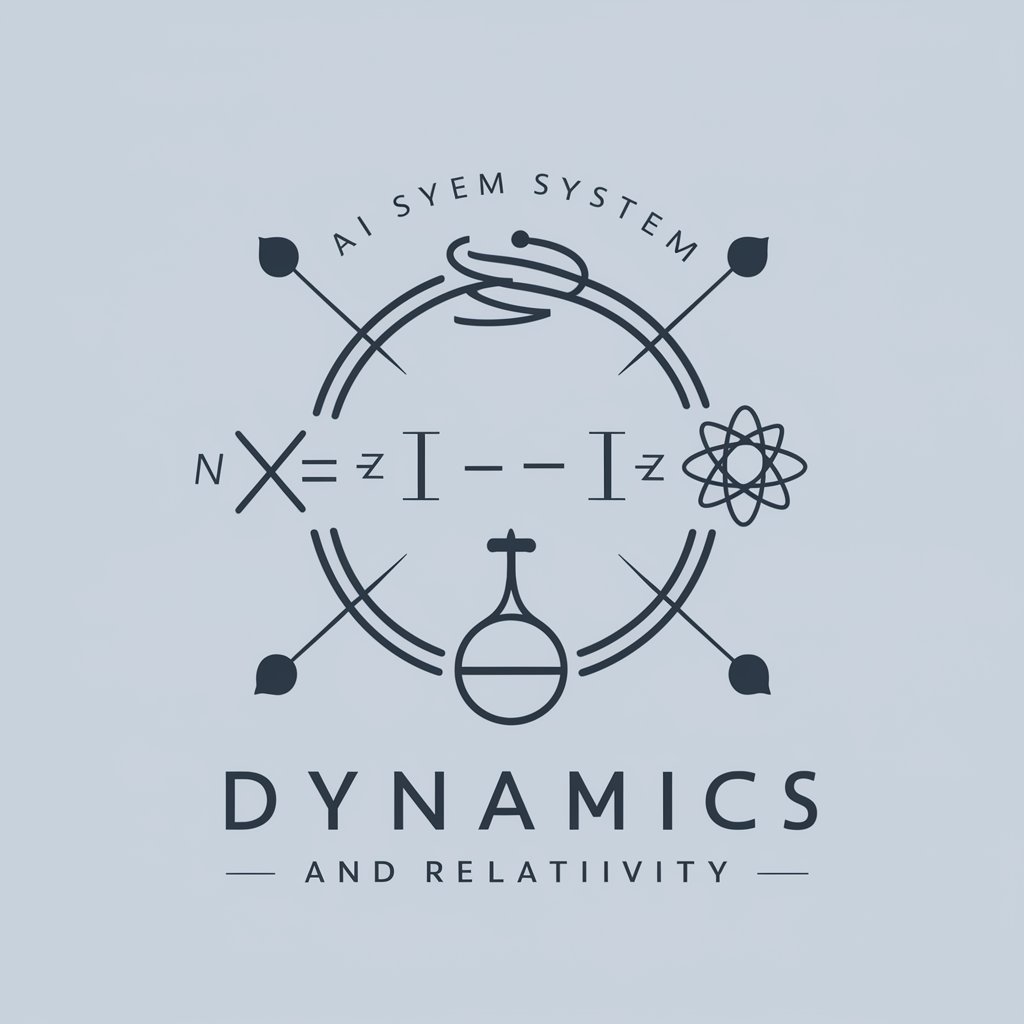
Python GPT
Empower your Python projects with AI guidance.
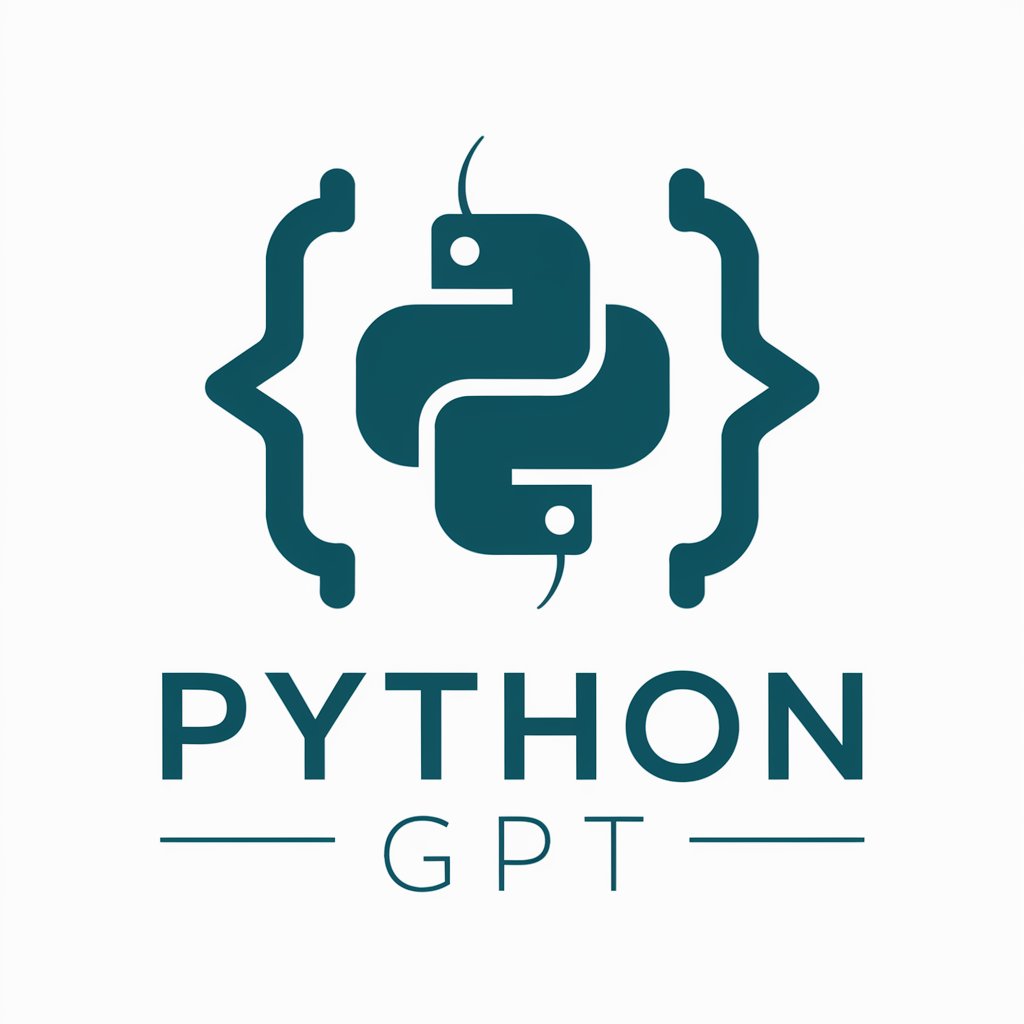
TypeGPT
Empower your TypeScript development with AI.
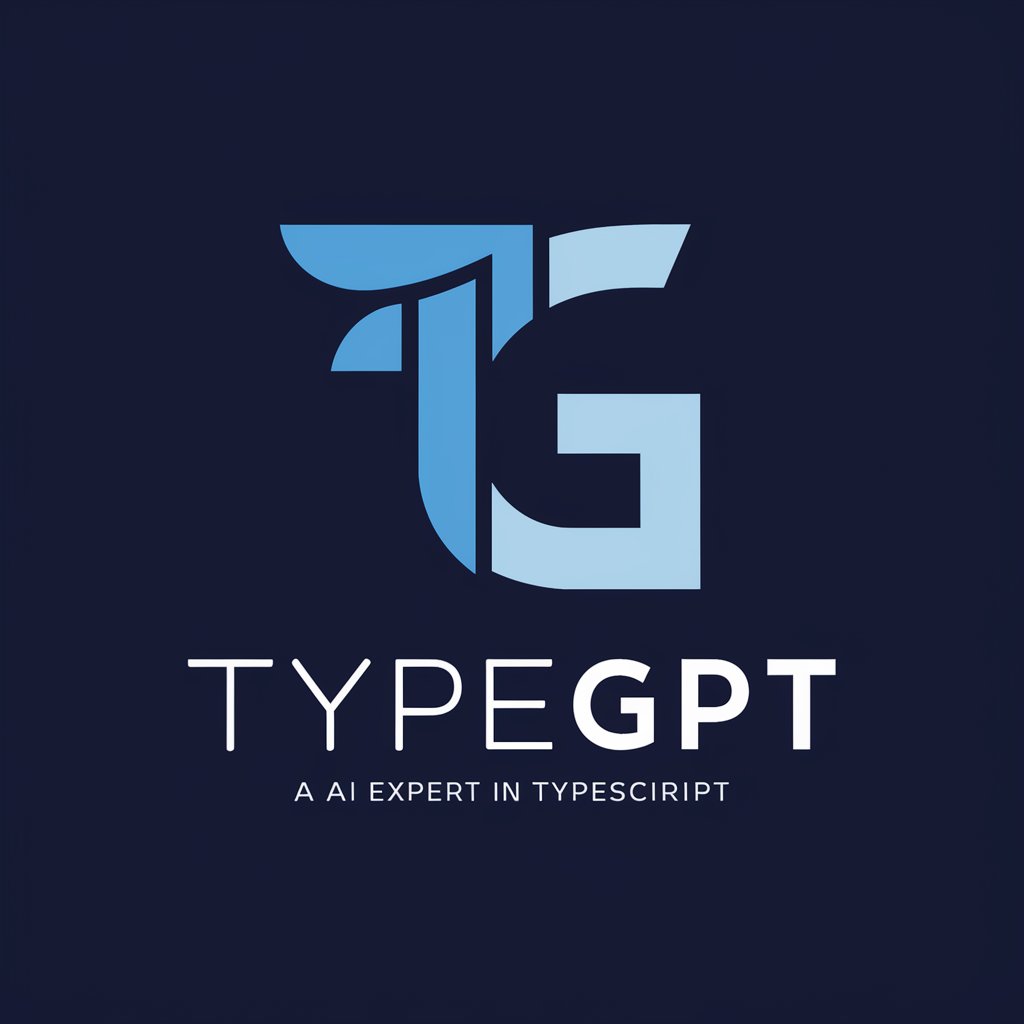
Study with RV
Unlock the power of AI for smarter studying.
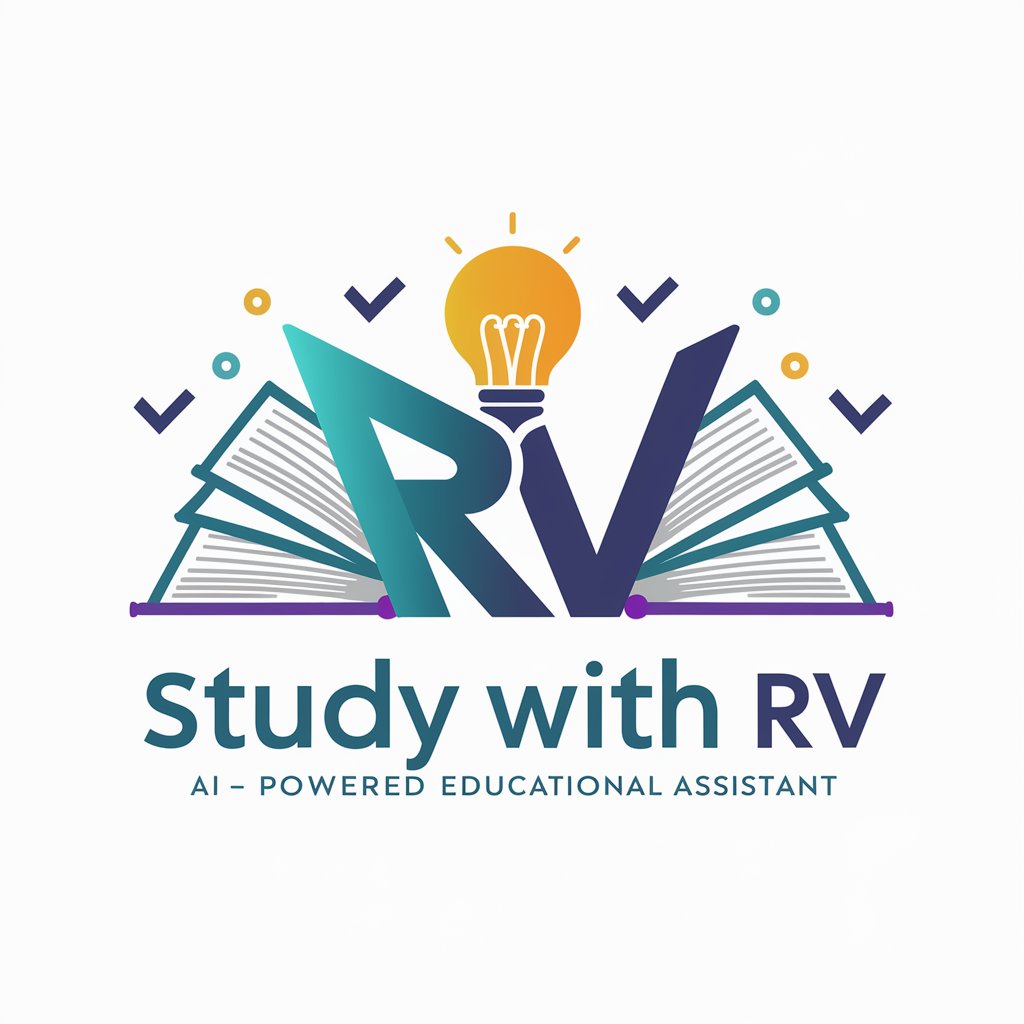
Prompt Master
Craft detailed prompts with AI precision.
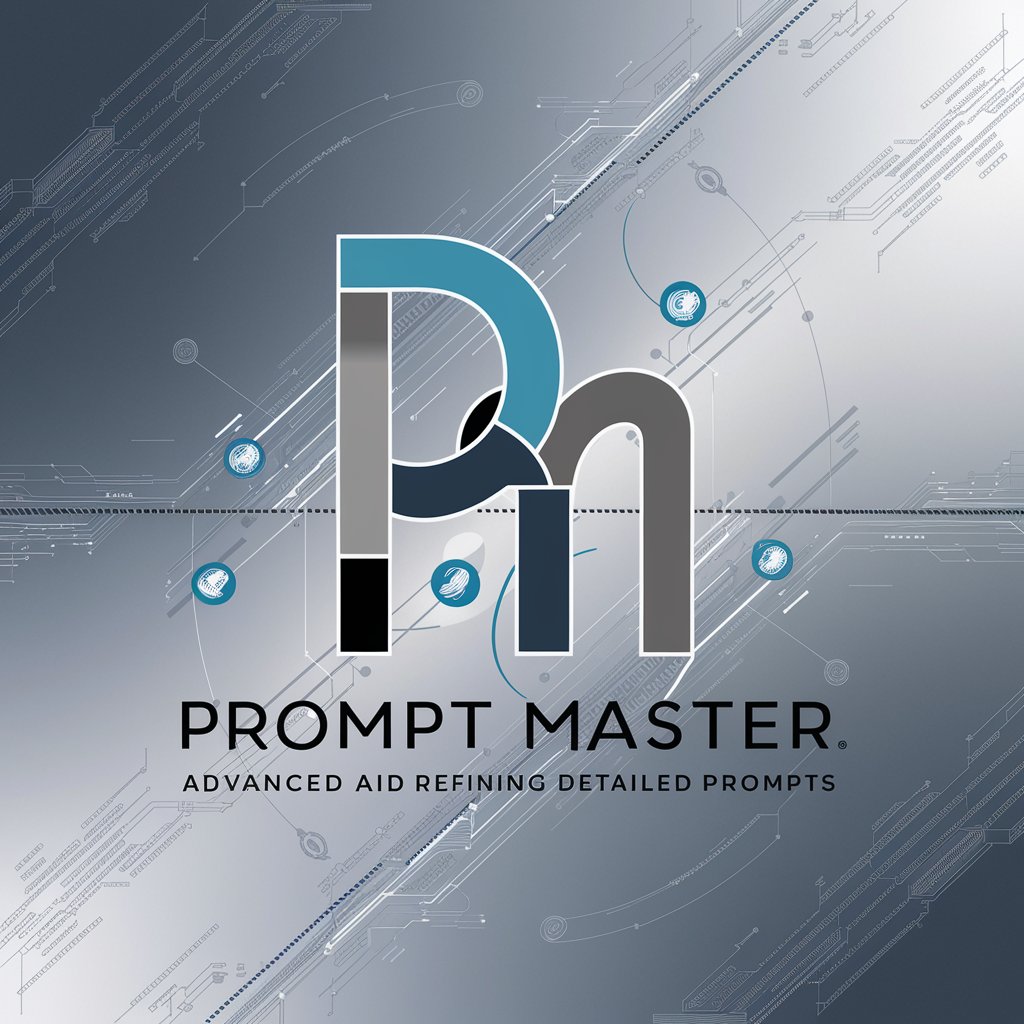
軟體工程優化師
Empowering Code Excellence with AI
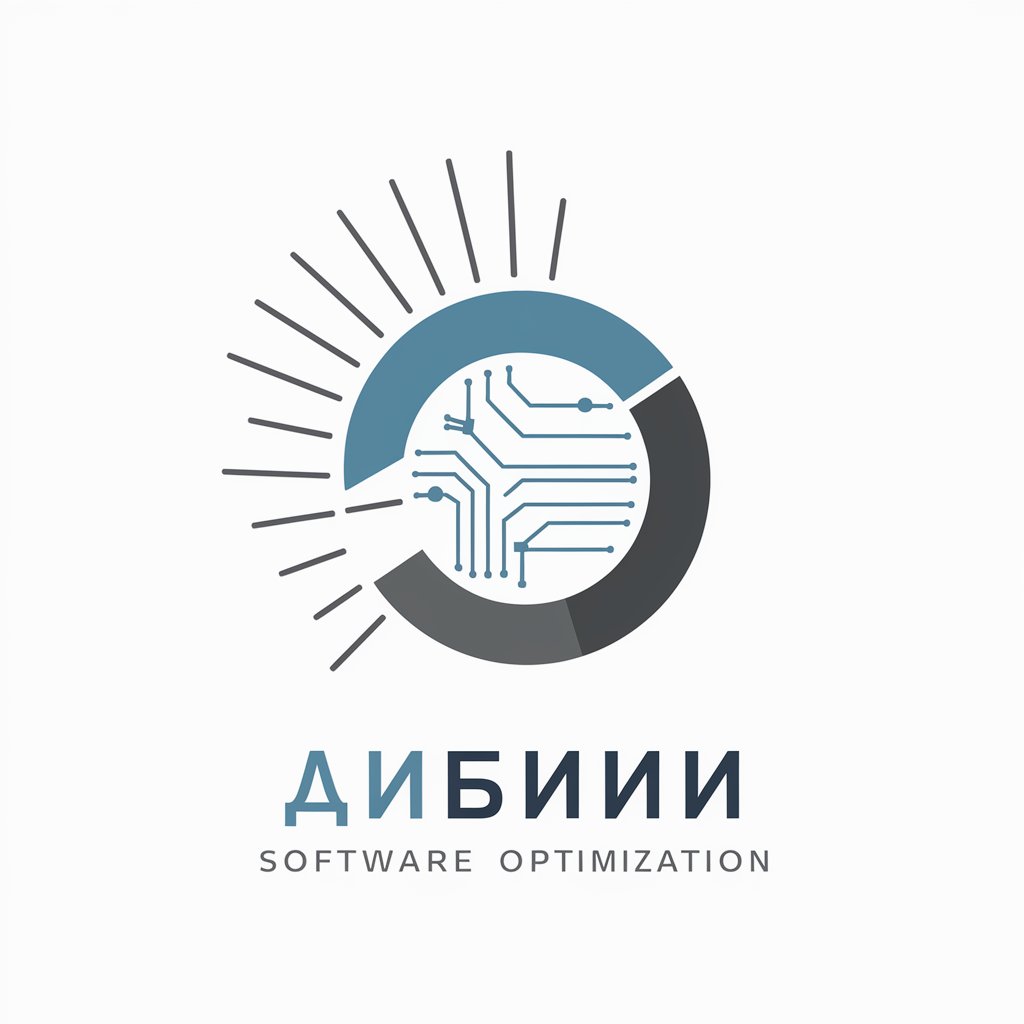
Dessin PDA
Unleash Your Comic Creativity with AI!
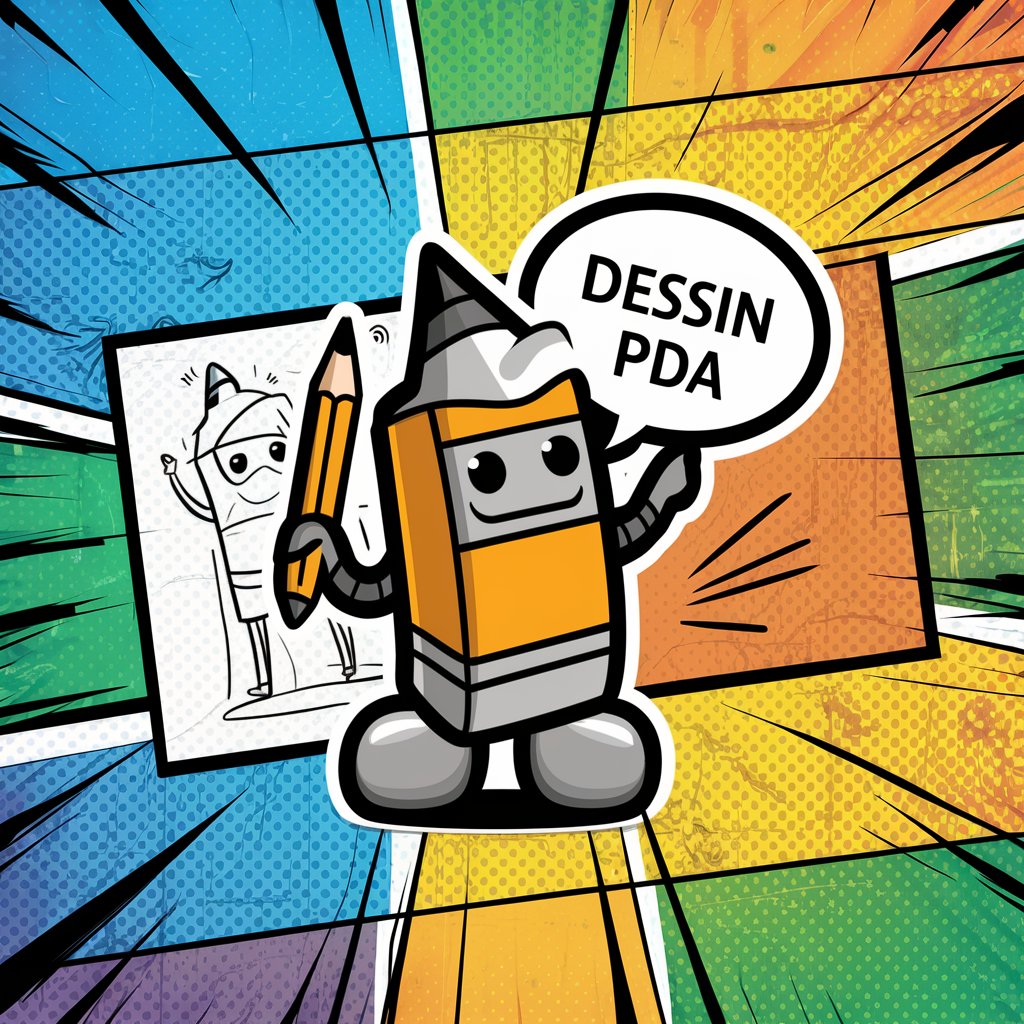
Lalaloopsy/Super Smash Dolls RP Chat Room
Immerse in AI-powered crossover role-playing.
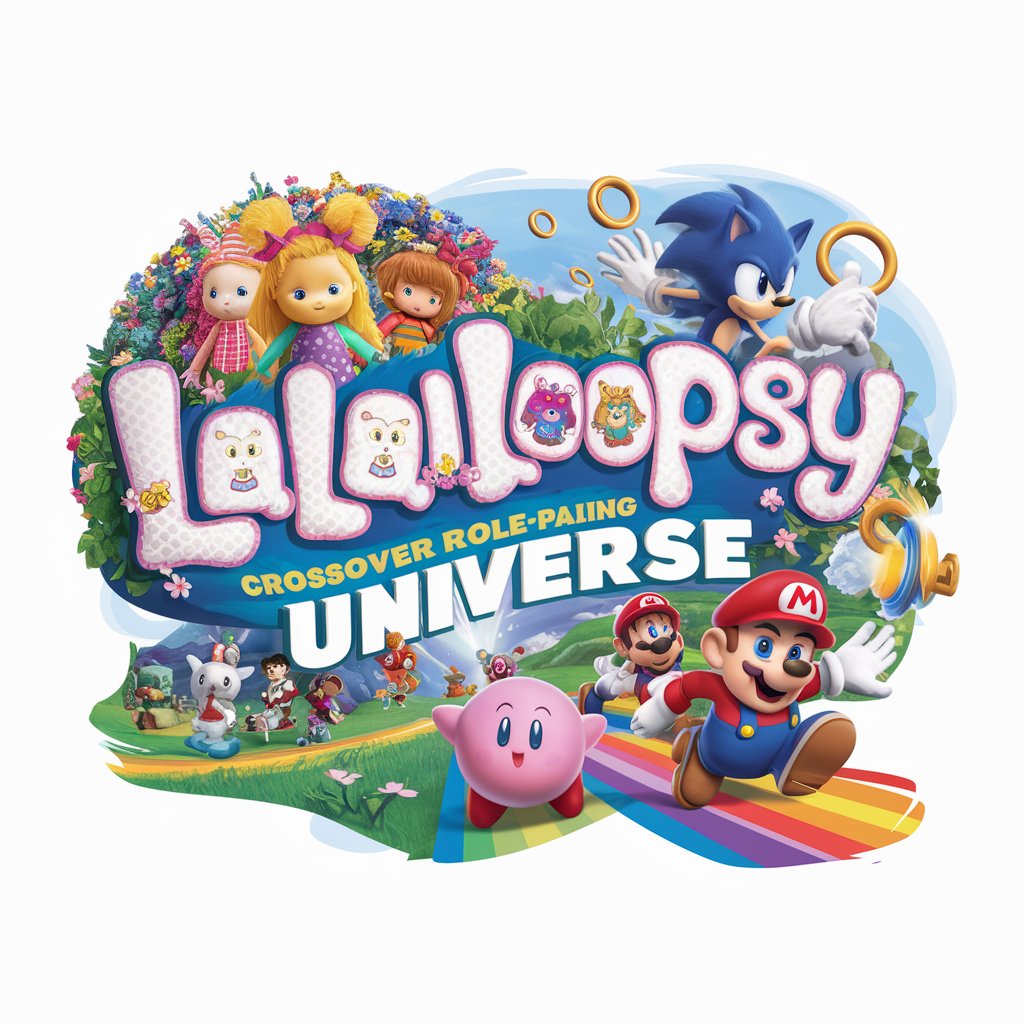
Frequently Asked Questions About Synthetic Data
What is synthetic data?
Synthetic data is artificially generated data that mimics real-world data but does not contain any real, sensitive information. It's used to train machine learning models where actual data is scarce or sensitive.
How does synthetic data help in machine learning?
It provides a high volume of diverse, annotated data which can be used to train and improve machine learning models without the privacy risks associated with real data.
Can synthetic data replace real data?
While synthetic data is useful for augmenting datasets and initial training phases, it cannot completely replace real data due to potential biases and the complexity of real-world scenarios it might not capture.
What are the risks of using synthetic data?
Potential risks include the introduction of biases if the synthetic data generation algorithms are not properly calibrated, and the data might not accurately reflect real-world variations.
How can one ensure the quality of synthetic data?
Quality can be ensured by using advanced generation techniques that include realistic variability, and by continuously validating the synthetic data against real-world outcomes and metrics.