4 GPTs for Material Simulation Powered by AI for Free of 2024
AI GPTs for Material Simulation are advanced computational tools powered by Generative Pre-trained Transformers that offer specialized solutions in the field of material science and engineering. These tools leverage the capabilities of GPTs to analyze, predict, and simulate the properties and behaviors of various materials under different conditions. They are particularly valuable for their ability to process and generate complex datasets, providing insights that assist in material design, innovation, and testing. By integrating AI with material science, these tools offer a transformative approach to discovering new materials, optimizing material properties, and solving engineering challenges.
Top 4 GPTs for Material Simulation are: architecture render engine gpt,Texture Generator,Material Intellect Nexus,Quantum Computing Problem Solver
architecture render engine gpt
Bringing Architectural Concepts to Life with AI
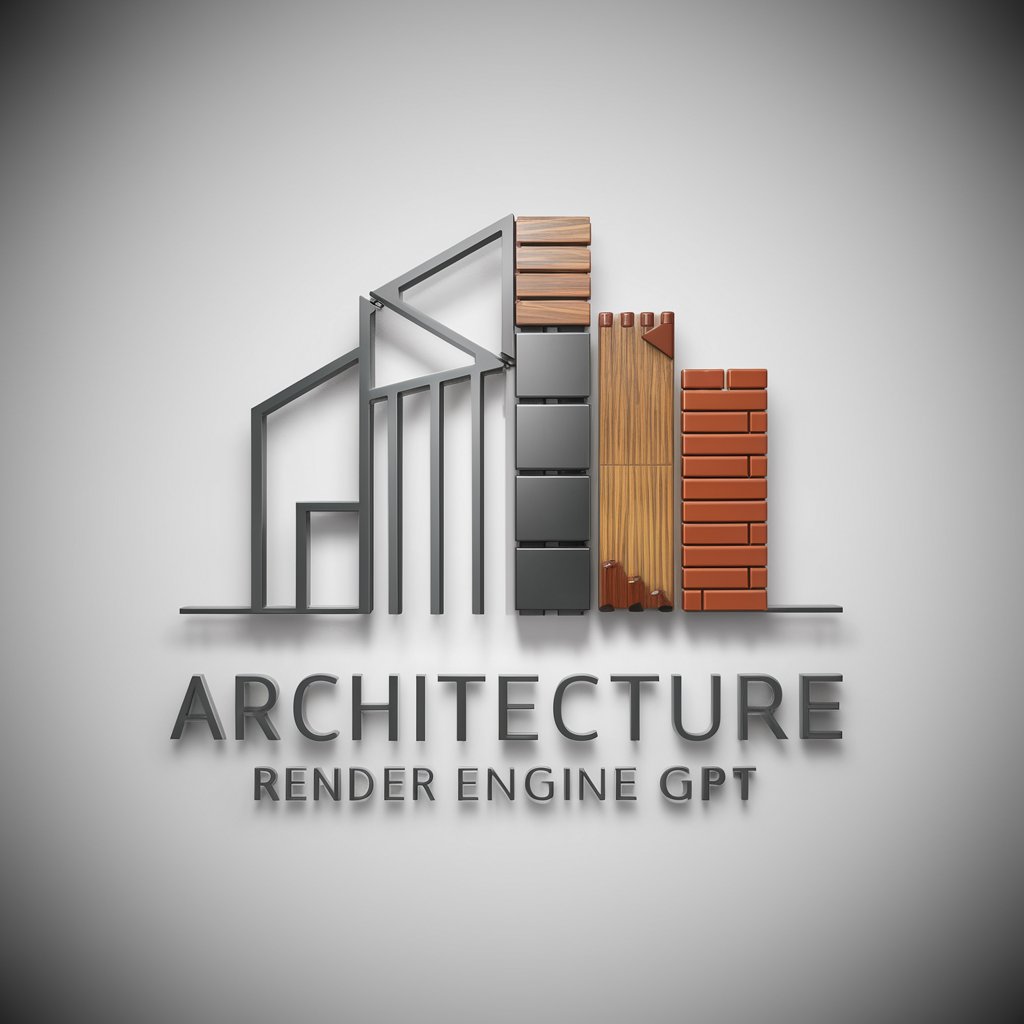
Texture Generator
Crafting Realism with AI-Powered Textures
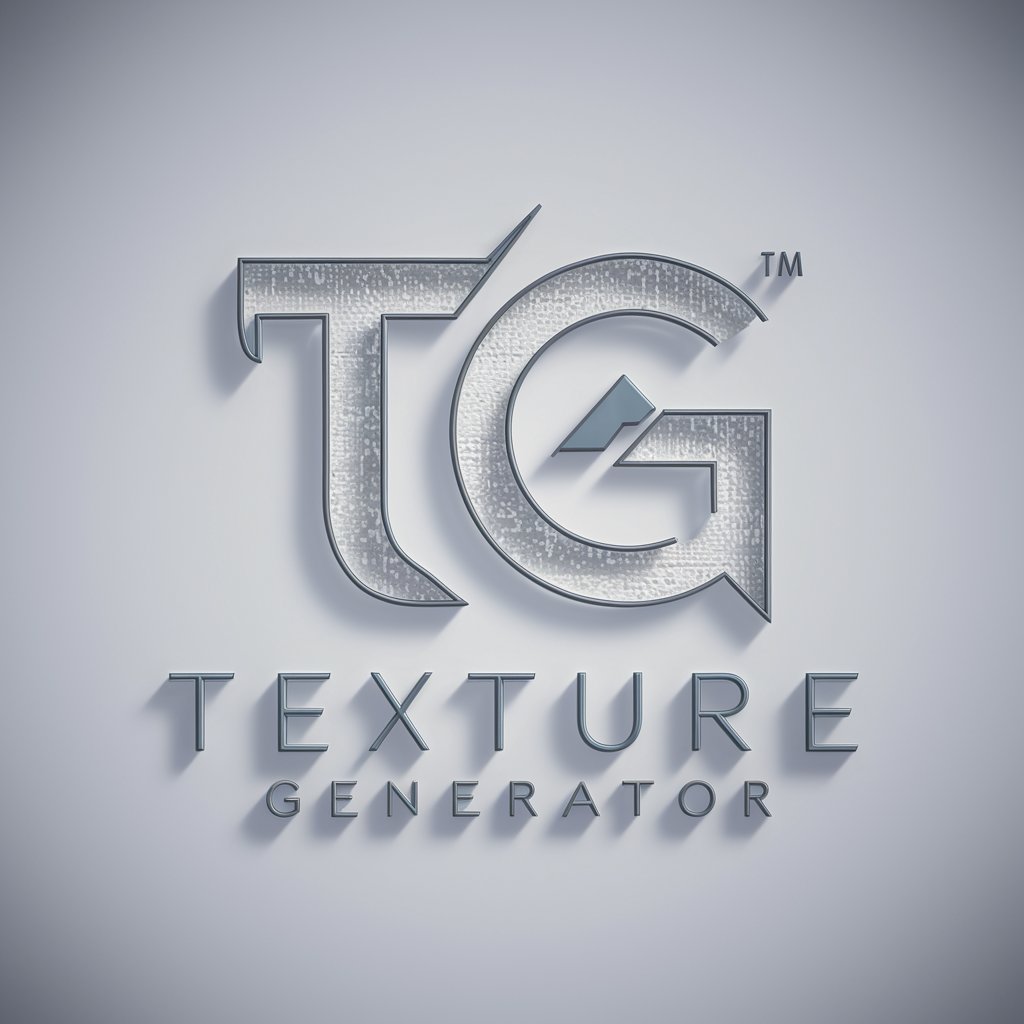
Material Intellect Nexus
Empowering material science with AI.

Quantum Computing Problem Solver
Solving complex problems with quantum AI.
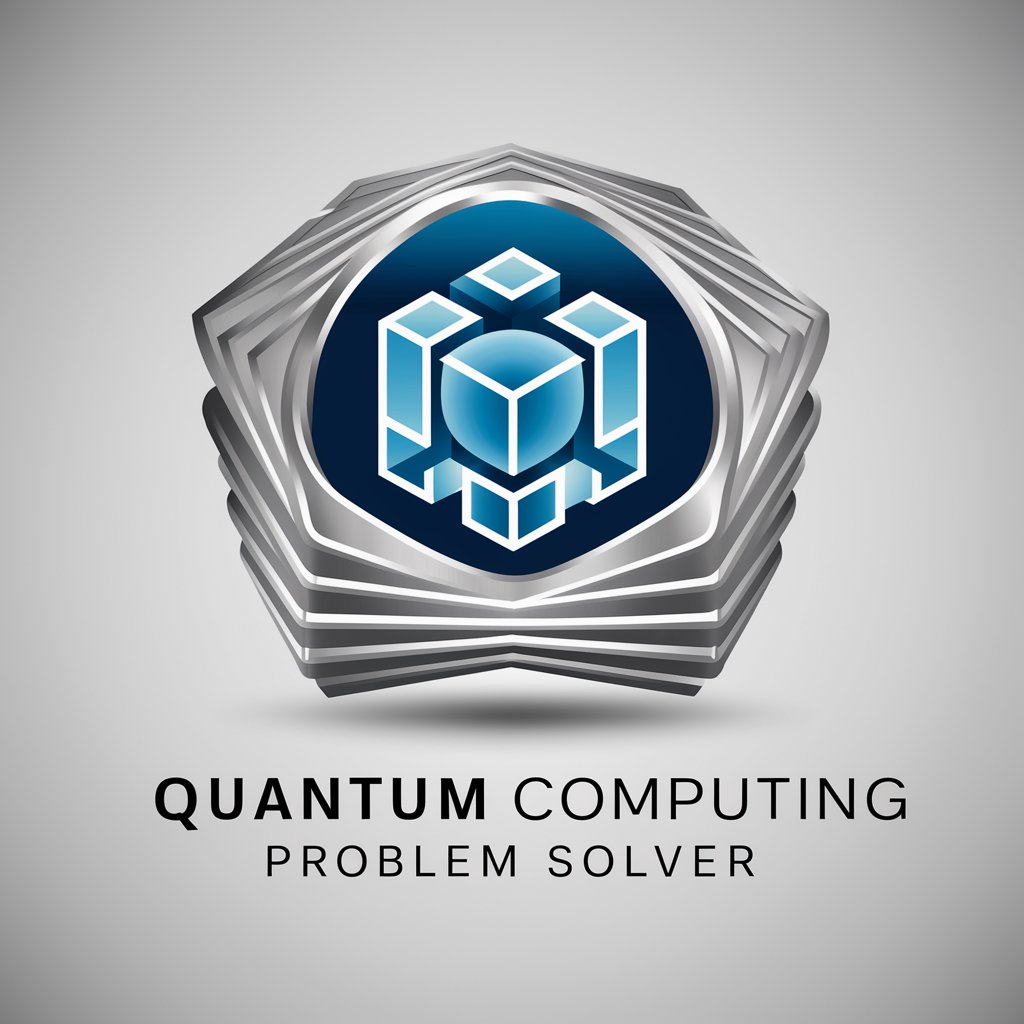
Key Attributes of Material Simulation GPTs
AI GPTs for Material Simulation distinguish themselves through their adaptability, precision, and breadth of application. Features include high-level data analysis, predictive modeling, and simulation capabilities that can be tailored from basic research to complex engineering solutions. They are equipped with language understanding for technical literature review, support advanced data analysis, and can create or interpret visual data representations. Unique to these tools are their abilities to learn from new data, simulate material responses under various conditions, and provide actionable insights for material development and optimization.
Who Benefits from Material Simulation AI?
The primary beneficiaries of AI GPTs for Material Simulation include researchers, material scientists, engineers, and educators in the field of material science and engineering. These tools are accessible to novices, offering user-friendly interfaces and guidance, while also providing extensive customization and programming capabilities for developers and professionals. This dual accessibility ensures that a wide range of users, from those without coding skills to experts requiring advanced functionalities, can leverage these tools for material innovation and problem-solving.
Try Our other AI GPTs tools for Free
Assistive Technology
Explore how AI GPTs revolutionize Assistive Technology, offering tailored, intuitive solutions that enhance digital accessibility for individuals with disabilities.
Global Inclusion
Discover how AI GPTs for Global Inclusion are making digital spaces more accessible and relevant across cultures and languages, promoting inclusivity and equality worldwide.
Universal Design
Explore AI GPTs for Universal Design: Cutting-edge tools designed to enhance accessibility and inclusivity across various domains, making the principles of Universal Design more accessible to all.
Dog Breeds
Discover the innovative AI GPT tools for Dog Breeds, designed to enhance understanding and care for various dog breeds through tailored information and features.
Lifestyle Fit
Discover how AI GPTs for Lifestyle Fit can transform your daily life with personalized insights and recommendations tailored to your unique goals and preferences.
Mystical Learning
Discover AI GPTs for Mystical Learning: your gateway to exploring the depths of spirituality and esoteric knowledge with advanced AI technology.
Expanding Horizons with AI in Material Science
AI GPTs for Material Simulation represent a significant advancement in the field, offering scalable solutions for complex challenges. They facilitate a deeper understanding of materials and their potential applications, accelerating innovation and reducing the time and cost associated with material development. The integration of these tools into research and development workflows promises to enhance decision-making and drive forward the discovery of novel materials and applications.
Frequently Asked Questions
What exactly can AI GPTs for Material Simulation do?
They can predict material properties, simulate behavior under different conditions, assist in material design, and provide insights for material optimization and innovation.
Do I need coding skills to use these tools?
No, many GPTs for Material Simulation are designed with user-friendly interfaces that do not require prior coding knowledge for basic functionalities.
Can these tools integrate with existing research or development workflows?
Yes, they are designed to be flexible and can often be integrated with existing software and databases to enhance research and development processes.
How do these tools learn and adapt to new material science data?
They use machine learning algorithms to continuously learn from new data, improving their predictions and simulations over time.
Are AI GPTs for Material Simulation applicable to all types of materials?
While they are highly versatile, the applicability may vary based on the specific tool and the data it has been trained on. However, most are designed to cover a wide range of materials.
Can these tools predict the environmental impact of materials?
Yes, many of these tools are capable of assessing environmental impacts by analyzing material lifecycles, sustainability factors, and potential degradation processes.
How can I customize a GPT tool for my specific material simulation needs?
Customization often involves programming and data training. Users with coding skills can adjust algorithms, add custom datasets, and modify parameters to tailor the tool's functions.
What are the limitations of using AI for material simulation?
Limitations include the need for large and high-quality datasets for training, potential biases in data, and the complexity of accurately simulating real-world material behaviors.