Federated Learning Guide-Federated Learning Insights
Unlocking Federated Learning with AI
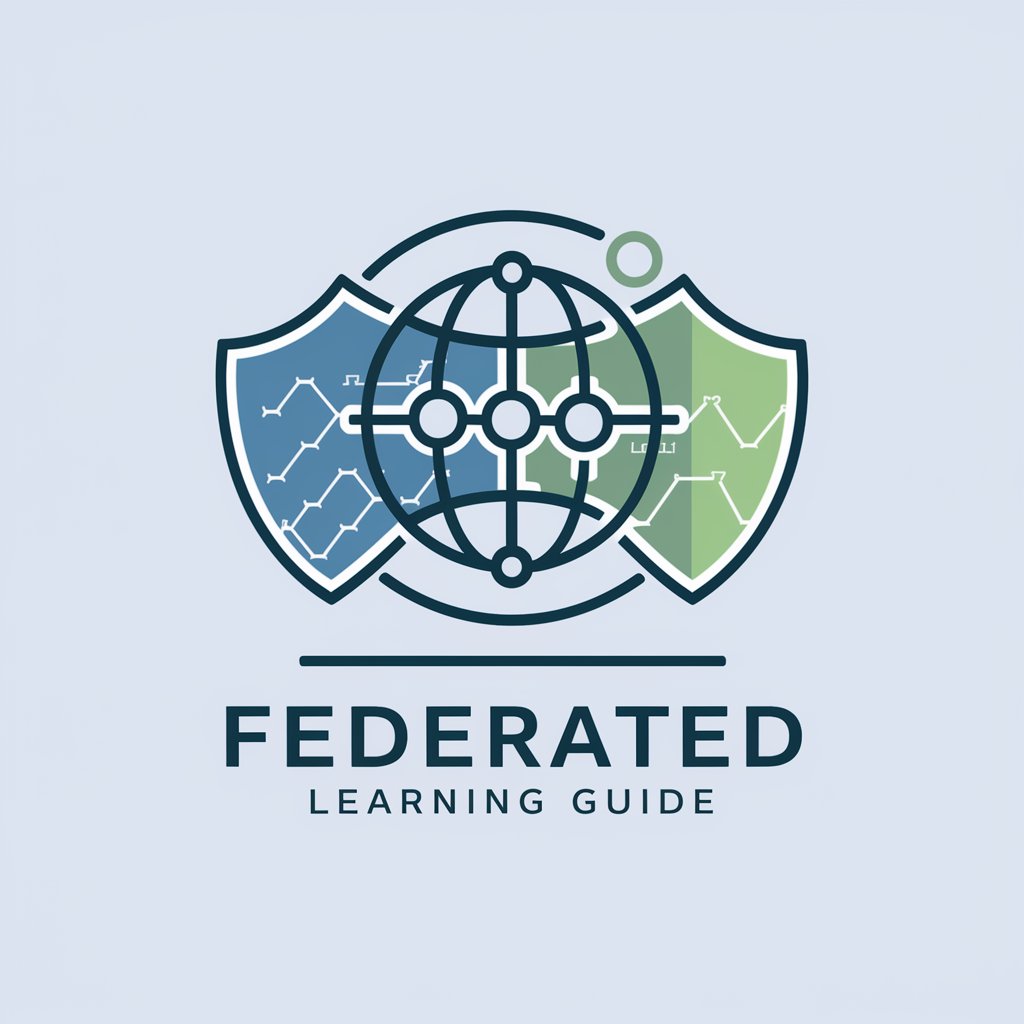
Explain the basic principles of federated learning and its key advantages.
How does federated learning ensure data privacy and security?
Describe the different algorithms used in federated learning and their applications.
What are the main challenges in implementing federated learning in real-world scenarios?
Related Tools
Load More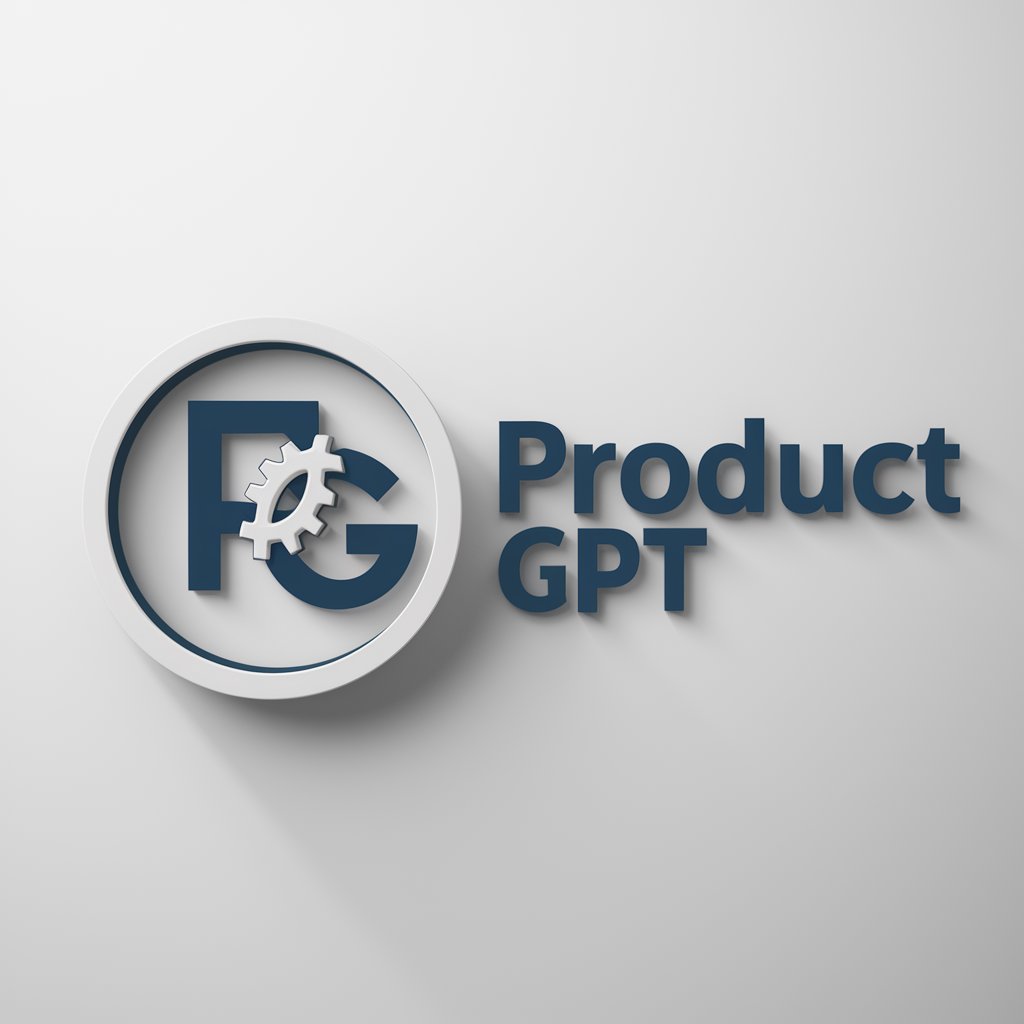
Product GPT
Convert your idea into a detailed project document: generate features, personas, time-estimates and UI wireframes
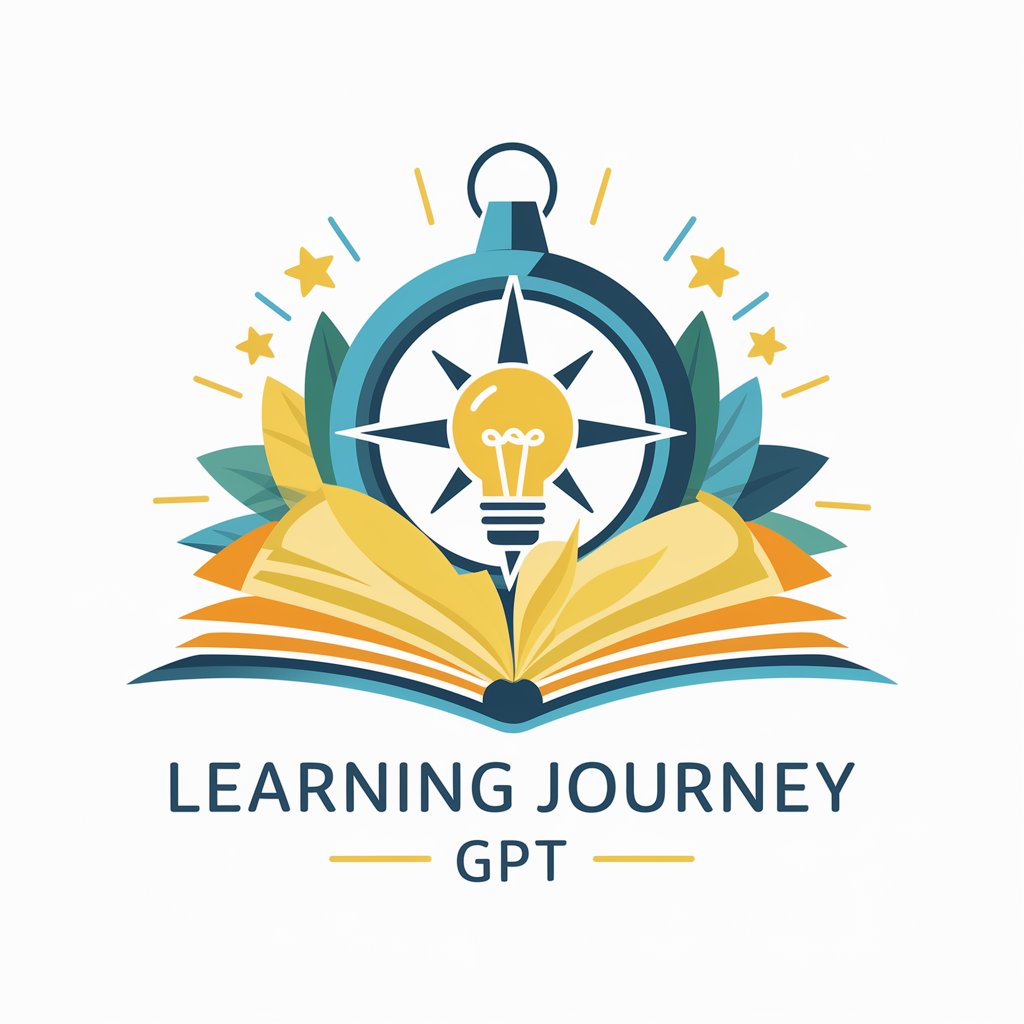
Learning Journey
A guide for personalized learning journeys in various subjects with interactive modes.

DeepLearning Assistant
Personal AI mentor for deep learning education
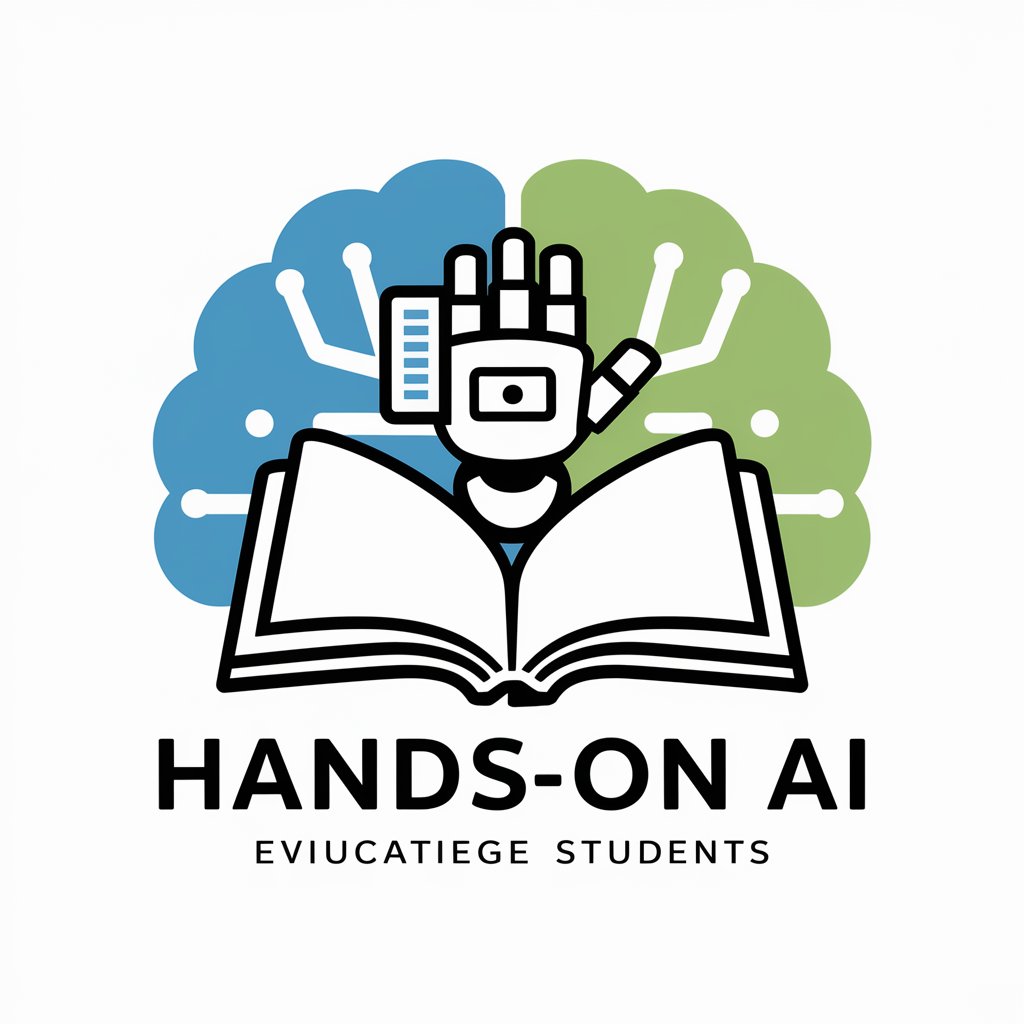
Hands-on AI
Help Beginners Get Started With AI

Thought Catalyst
A guide for deep, thought-provoking discussions.
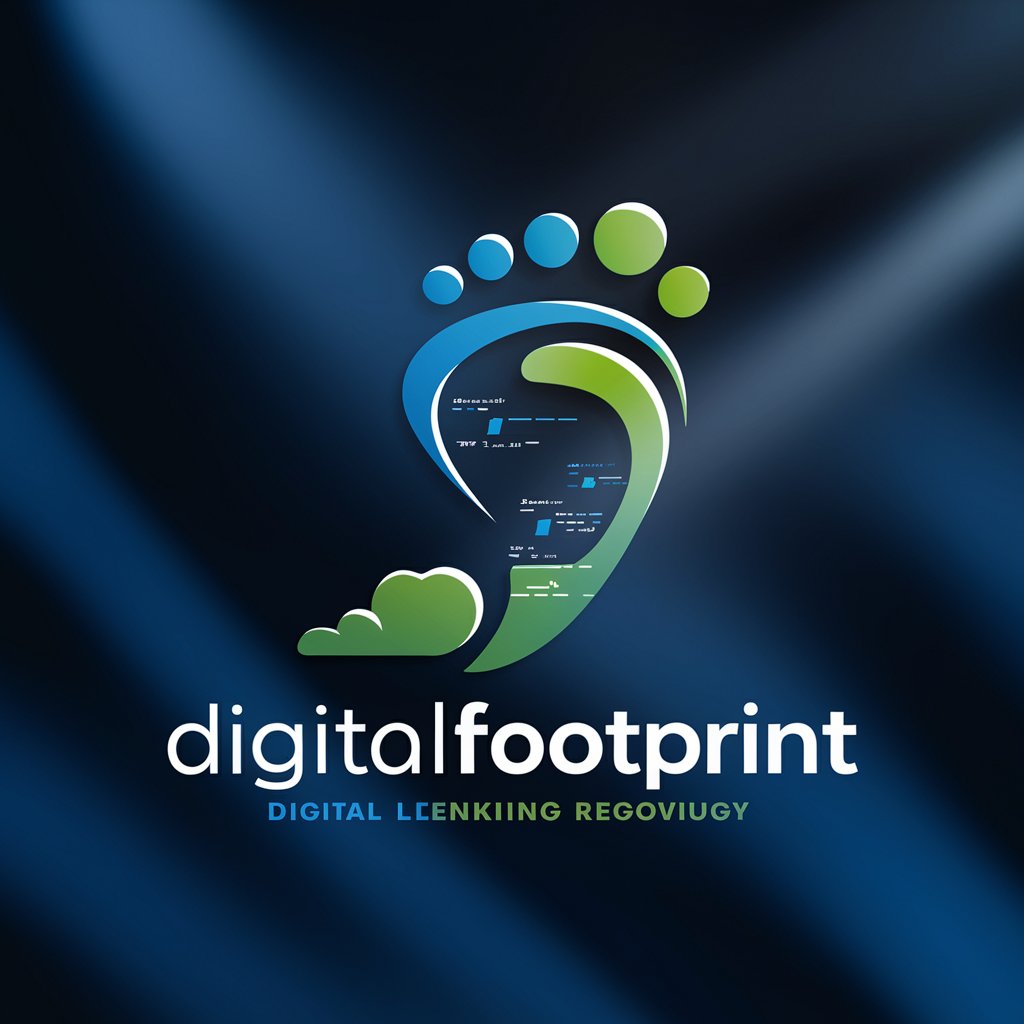
DigitalFootprint
Providing images, code, and business plans in every response. DigitalFootprint is your guide to establish a solid foundation in the cyberspace. Establish your place in the world and make your mark, whilst living your dream.
20.0 / 5 (200 votes)
Overview of Federated Learning Guide
The Federated Learning Guide is designed as a specialized assistant to offer in-depth insights and explanations on federated learning, a machine learning setup where the model is trained across multiple decentralized edge devices or servers without exchanging their data samples. This approach is pivotal for enhancing privacy, reducing data centralization risks, and minimizing data transmission costs. The design purpose of the Federated Learning Guide is to demystify the complexities of federated learning by providing detailed explanations of its algorithms, data privacy mechanisms, and its application in various domains. For instance, in a healthcare scenario, the Guide could explain how federated learning allows hospitals to collaboratively learn a predictive model for patient outcomes without sharing patient data, thereby preserving patient confidentiality. Powered by ChatGPT-4o。
Key Functions of Federated Learning Guide
Explaining Federated Learning Concepts
Example
Detailed breakdown of federated learning algorithms like Federated Averaging (FedAvg), addressing how local models are trained on edge devices and then aggregated to update a global model.
Scenario
A developer looking to implement federated learning in a mobile app could use this function to understand how to optimize model training locally on users' devices.
Guidance on Data Privacy and Security
Example
Insights into techniques such as differential privacy and secure multi-party computation that enhance data privacy in federated learning systems.
Scenario
A data privacy officer at a financial institution may seek guidance on ensuring customer data remains protected while participating in a federated learning project to detect financial fraud.
Application in Various Domains
Example
Examples of federated learning applications in sectors like healthcare, finance, and smart cities, showcasing its versatility and benefits.
Scenario
Healthcare researchers might explore how federated learning can be used for collaborative research across hospitals worldwide without compromising patient privacy.
Target Users of Federated Learning Guide
Developers and Technologists
Individuals in tech roles looking to incorporate federated learning into their applications or services. They benefit from understanding both the theoretical underpinnings and practical implementation details provided by the Guide.
Researchers and Academics
This group includes those in academia or research institutions focusing on advancing federated learning algorithms or exploring its implications for privacy and security. The Guide offers deep dives into state-of-the-art research and methodologies.
Industry Professionals
Professionals across various industries, such as healthcare, finance, and telecommunications, interested in leveraging federated learning to improve services while ensuring data privacy. They benefit from use case examples and sector-specific guidance.
How to Use the Federated Learning Guide
1. Start without Hassles
Visit yeschat.ai for a complimentary trial, no signup or ChatGPT Plus required.
2. Explore the Features
Familiarize yourself with the interface and available features to understand how Federated Learning Guide can assist you.
3. Identify Your Needs
Determine your specific questions or areas of interest in federated learning to make the most of the guide.
4. Engage with the Guide
Use the interactive Q&A feature to ask detailed questions. Utilize the custom queries option for more specific inquiries.
5. Apply the Insights
Incorporate the knowledge and insights gained from the Federated Learning Guide into your projects or research.
Try other advanced and practical GPTs
Personalized Language Mnemonics
Unlock Language Mastery with AI-Powered Mnemonics
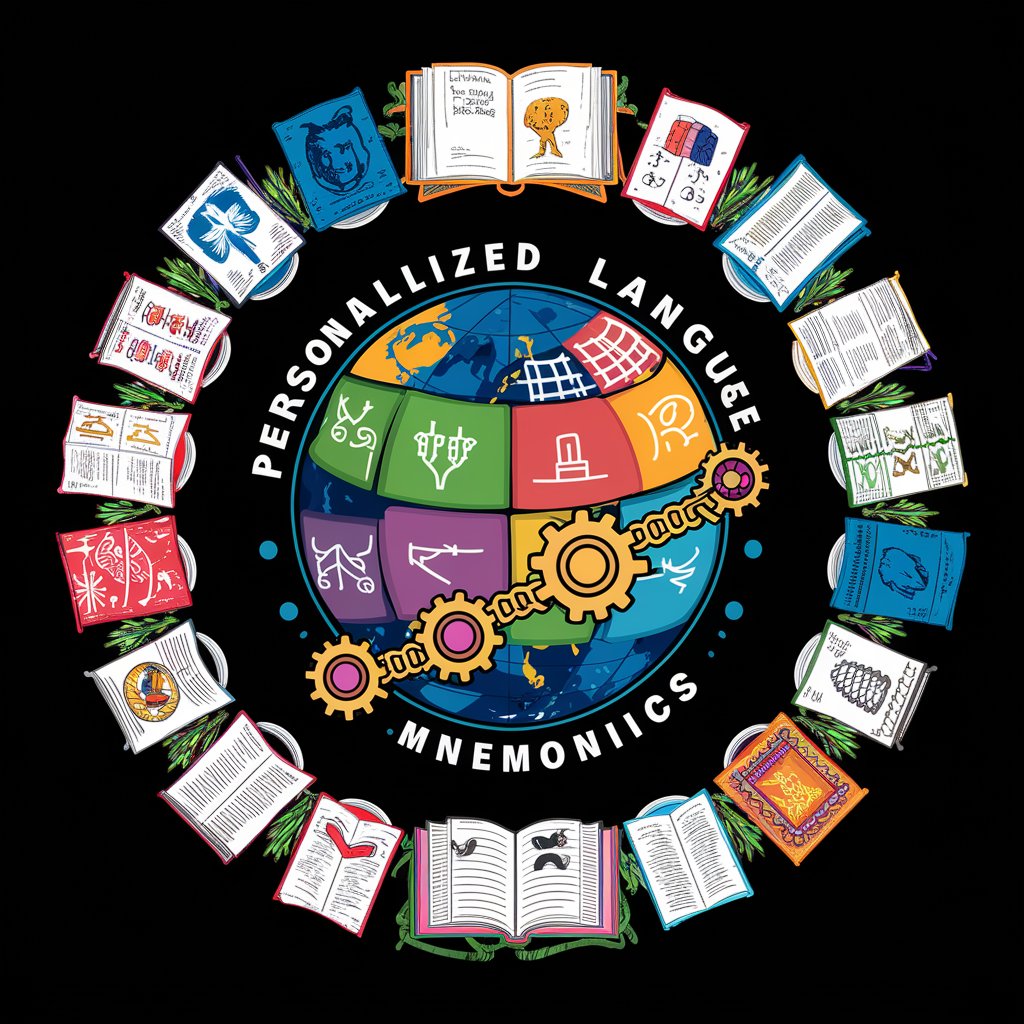
The Daily Dump
Humor Powered by AI
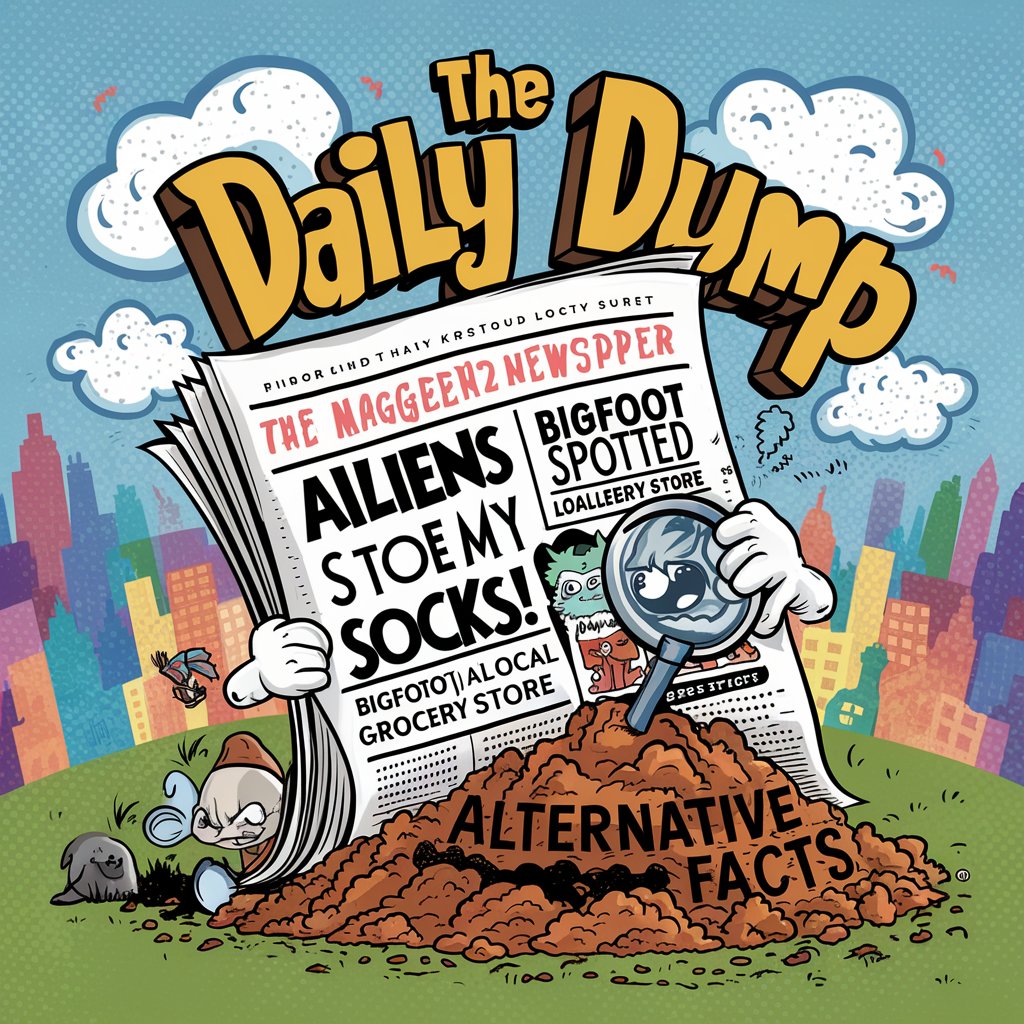
Jokester Genius
Elevate Your Humor with AI-Powered Wit
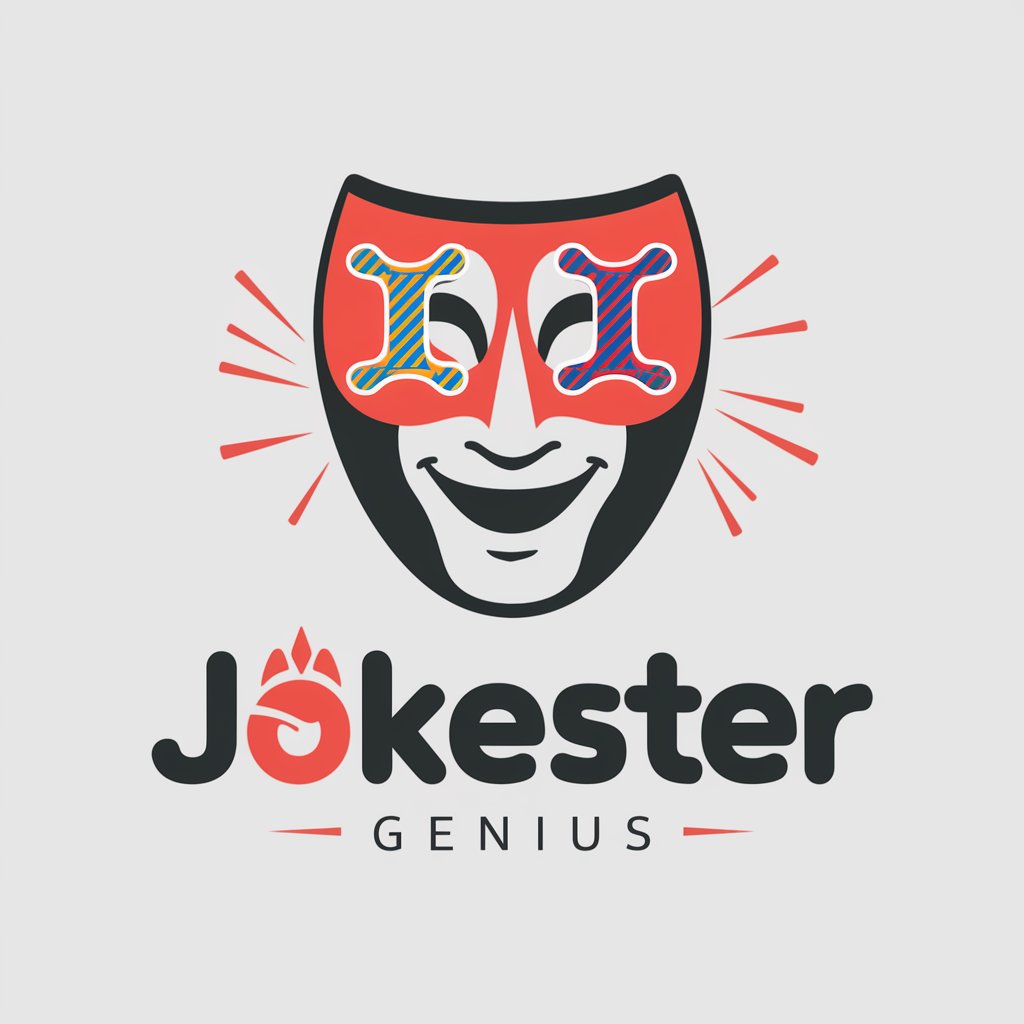
Allergy Chef
Culinary Freedom for Every Allergy
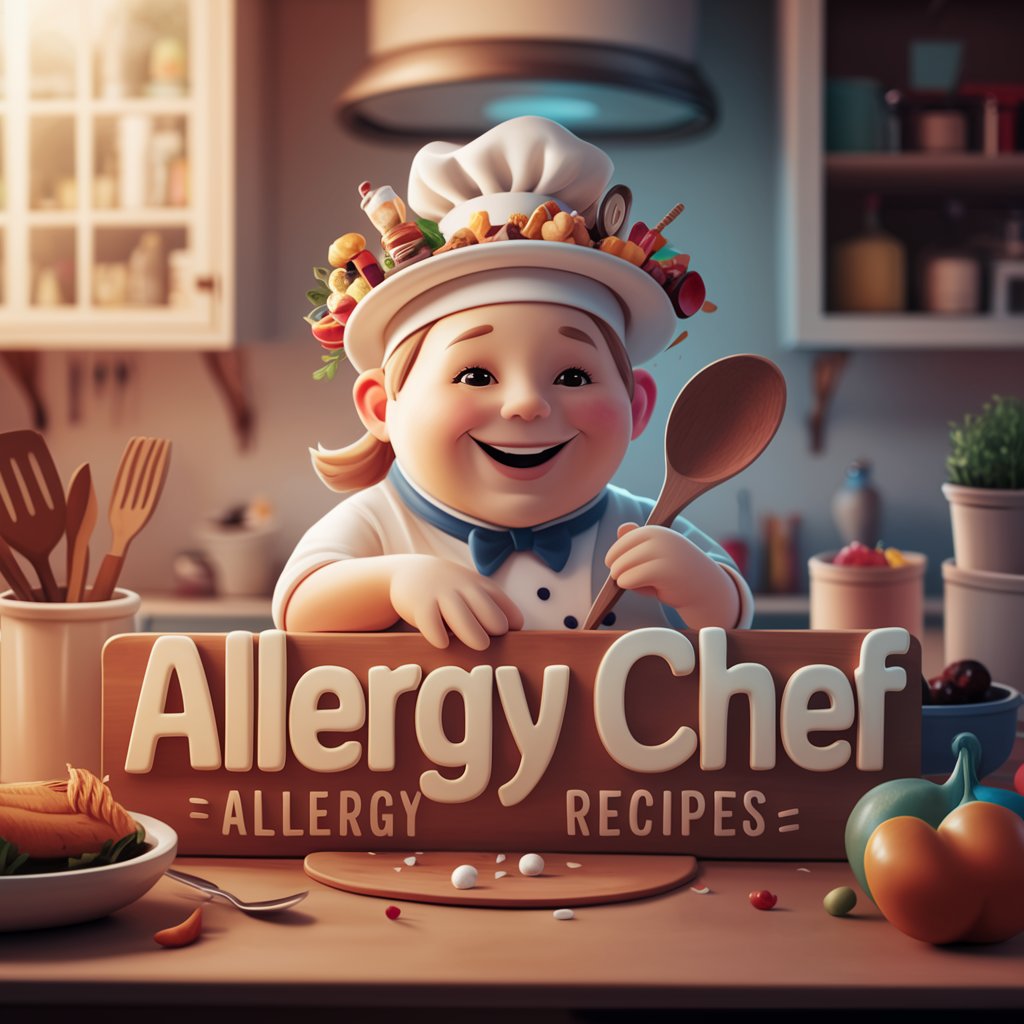
创业导师
Empowering Your Entrepreneurial Journey with AI
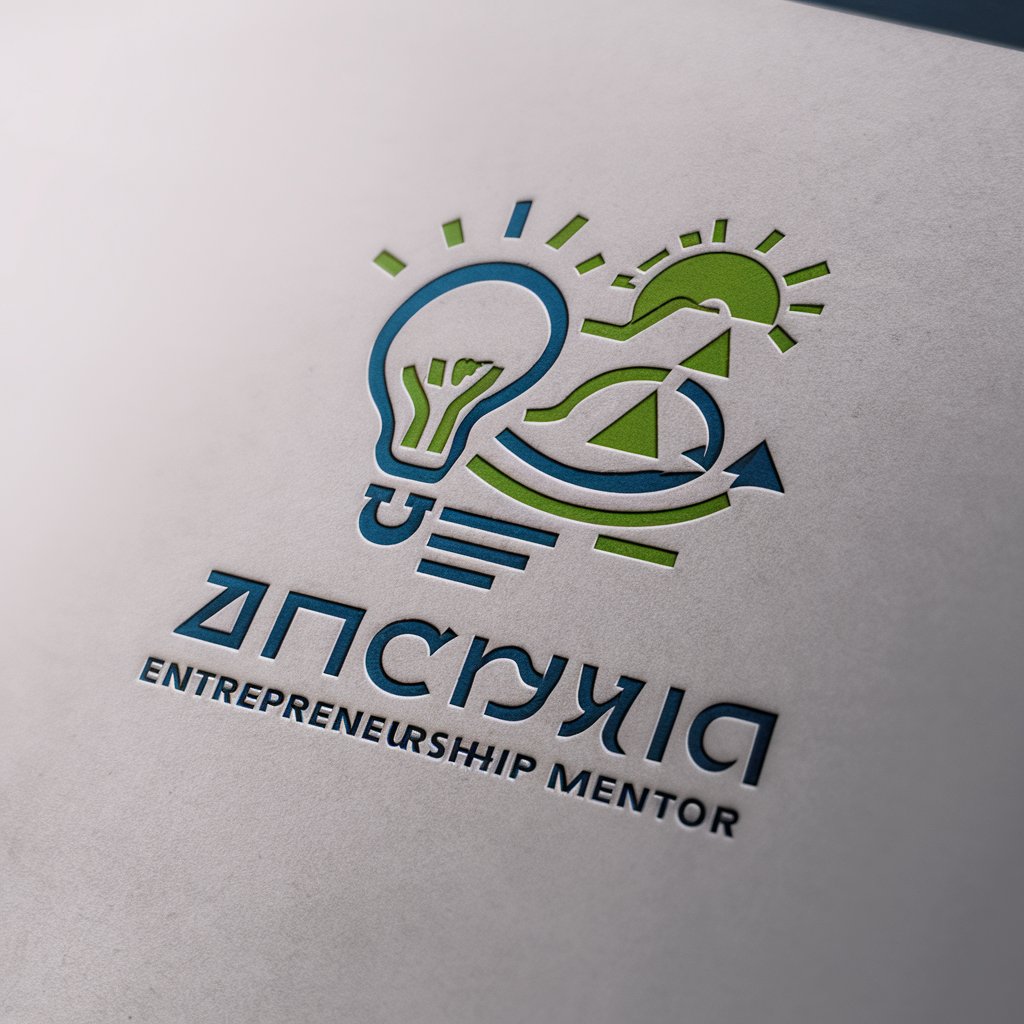
Visual Guide
AI-powered Logo Critique
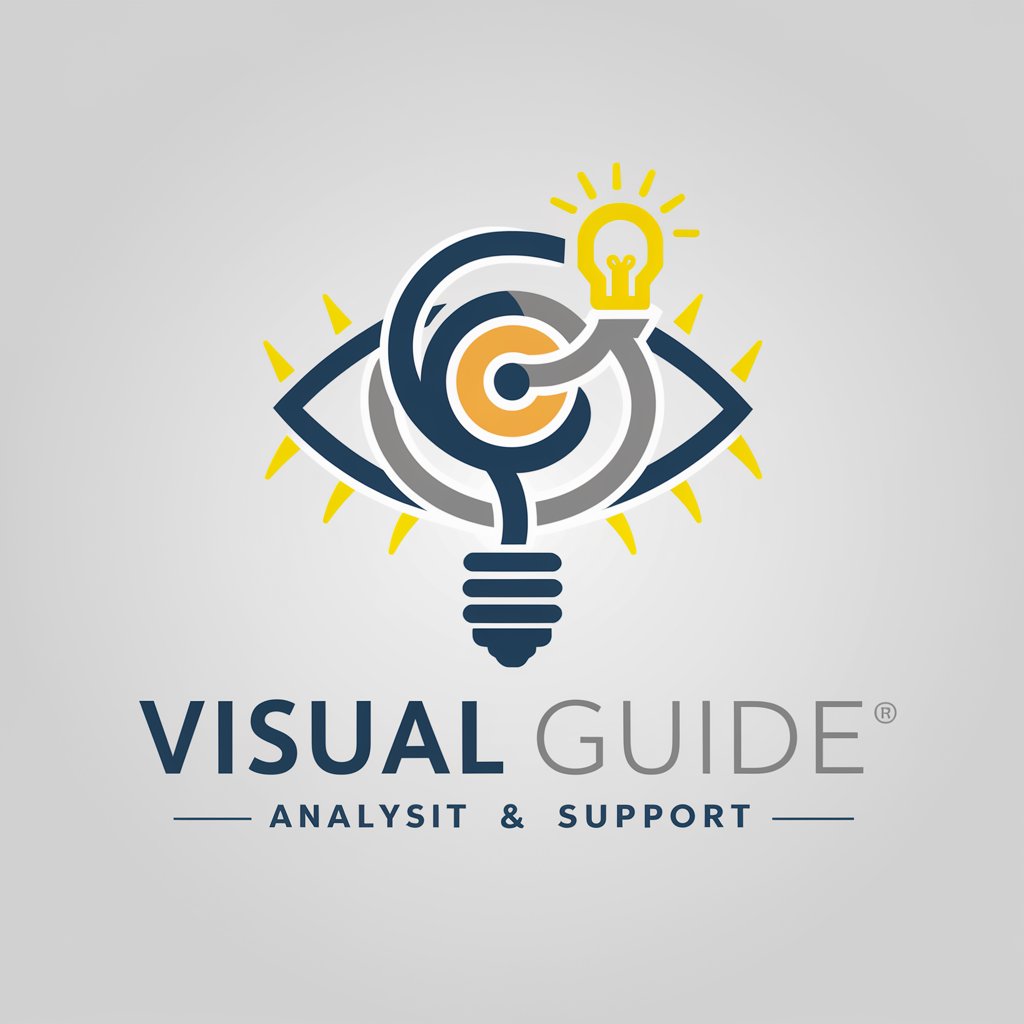
Dog Names
Crafting Unique Names for Every Dog
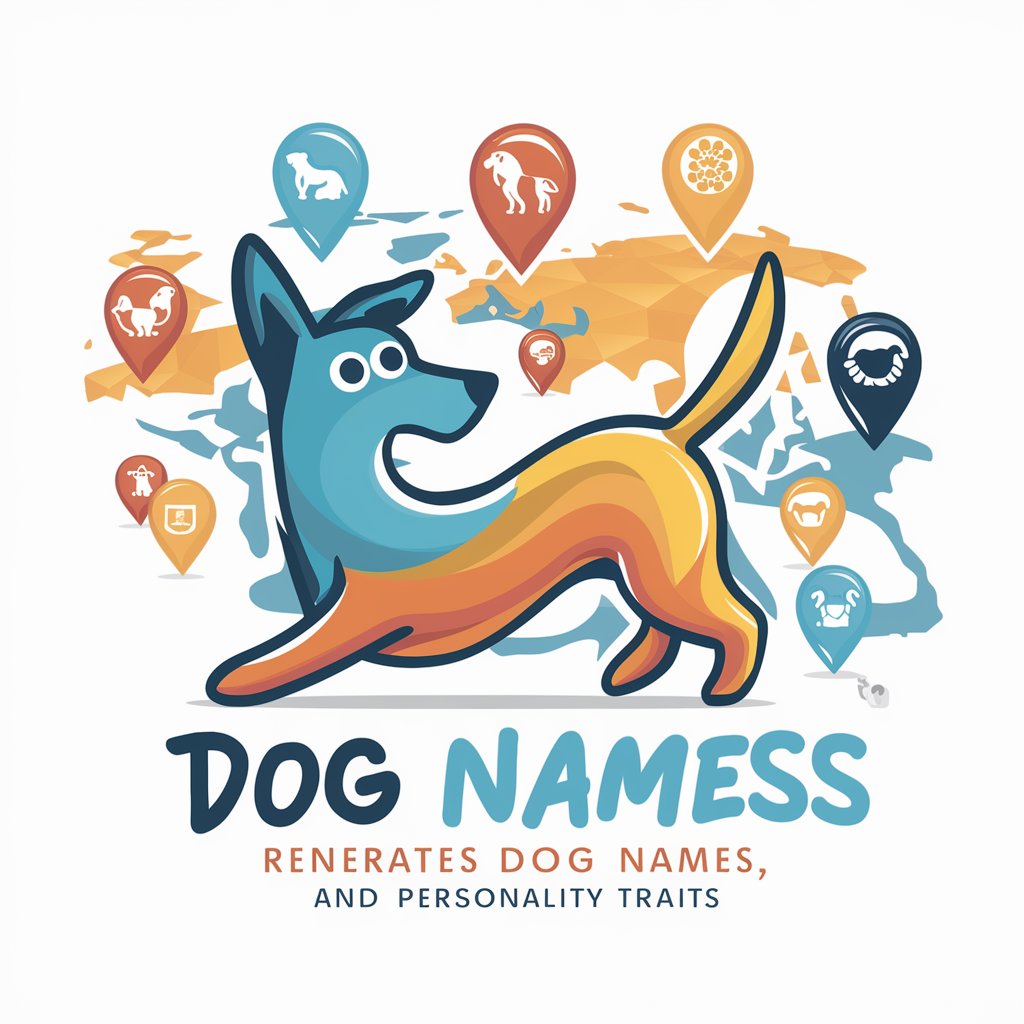
Support Team Assistant by Mojju
Empowering support with AI precision
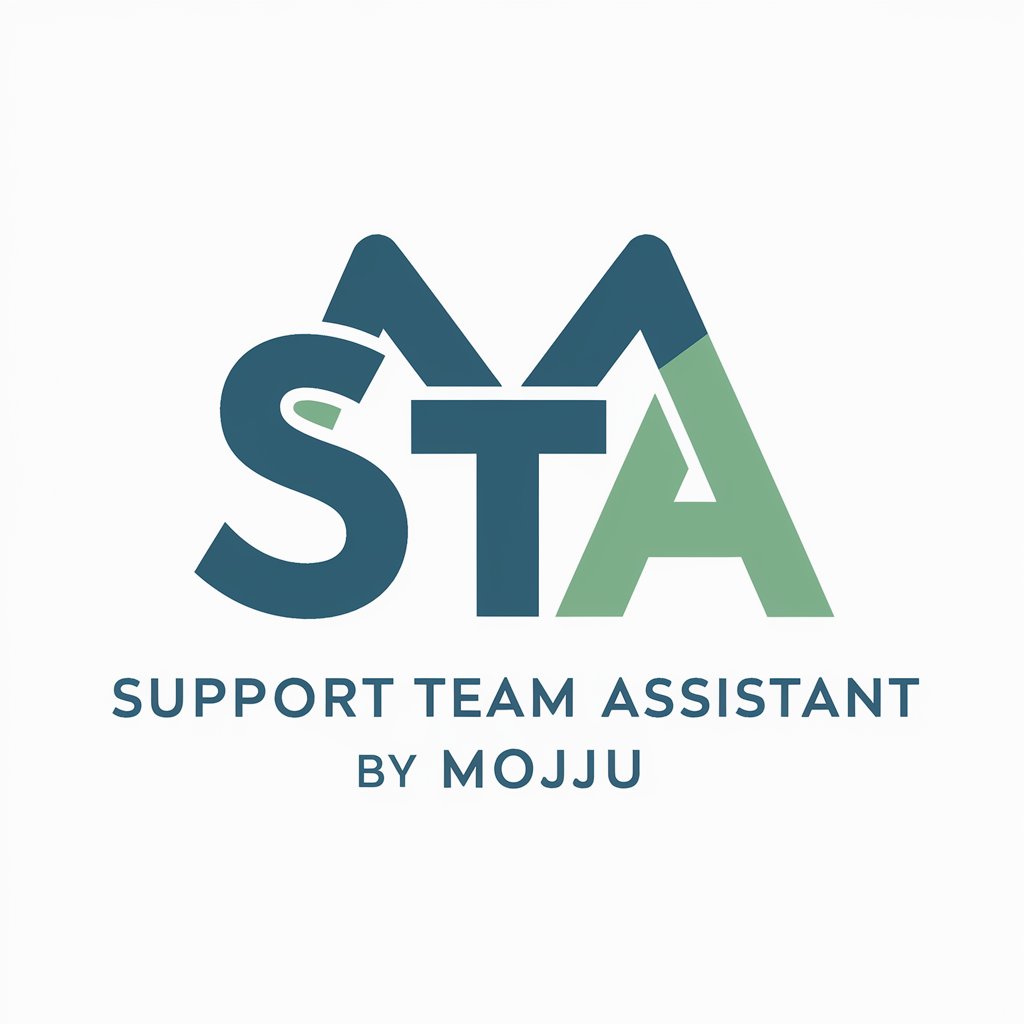
Case Study Navigator
Unlock Strategic Insights with AI
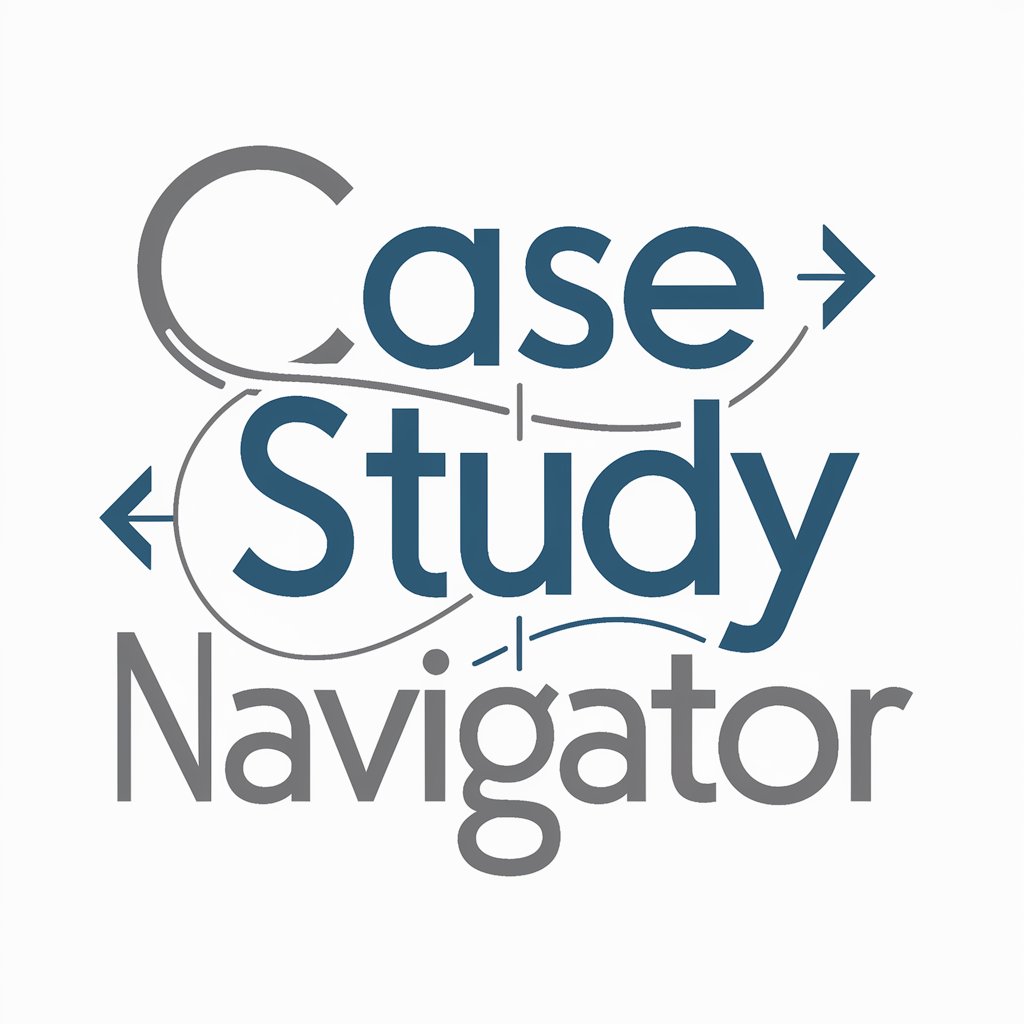
英字に慣れるトレーニング(SE向け)
Enhance your tech English with AI.
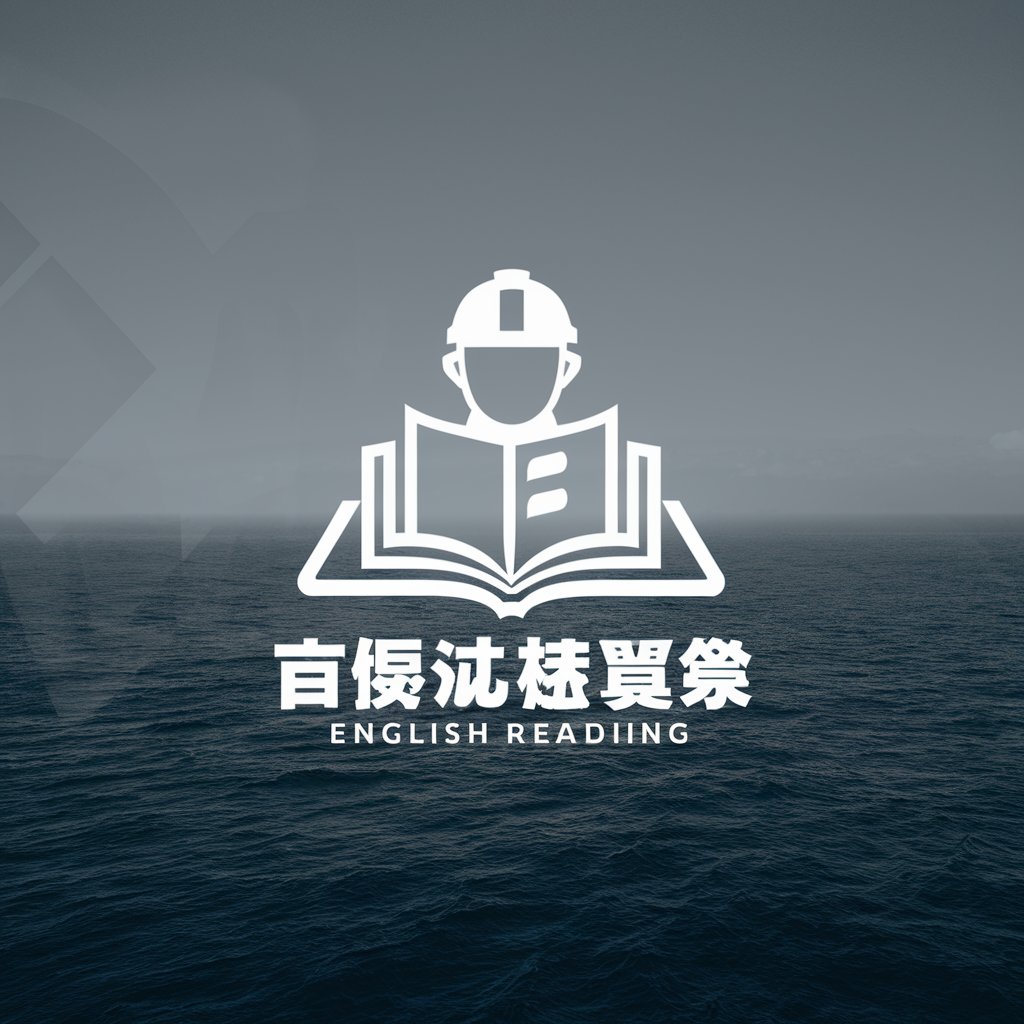
GuruGPT
Empowering wisdom through AI
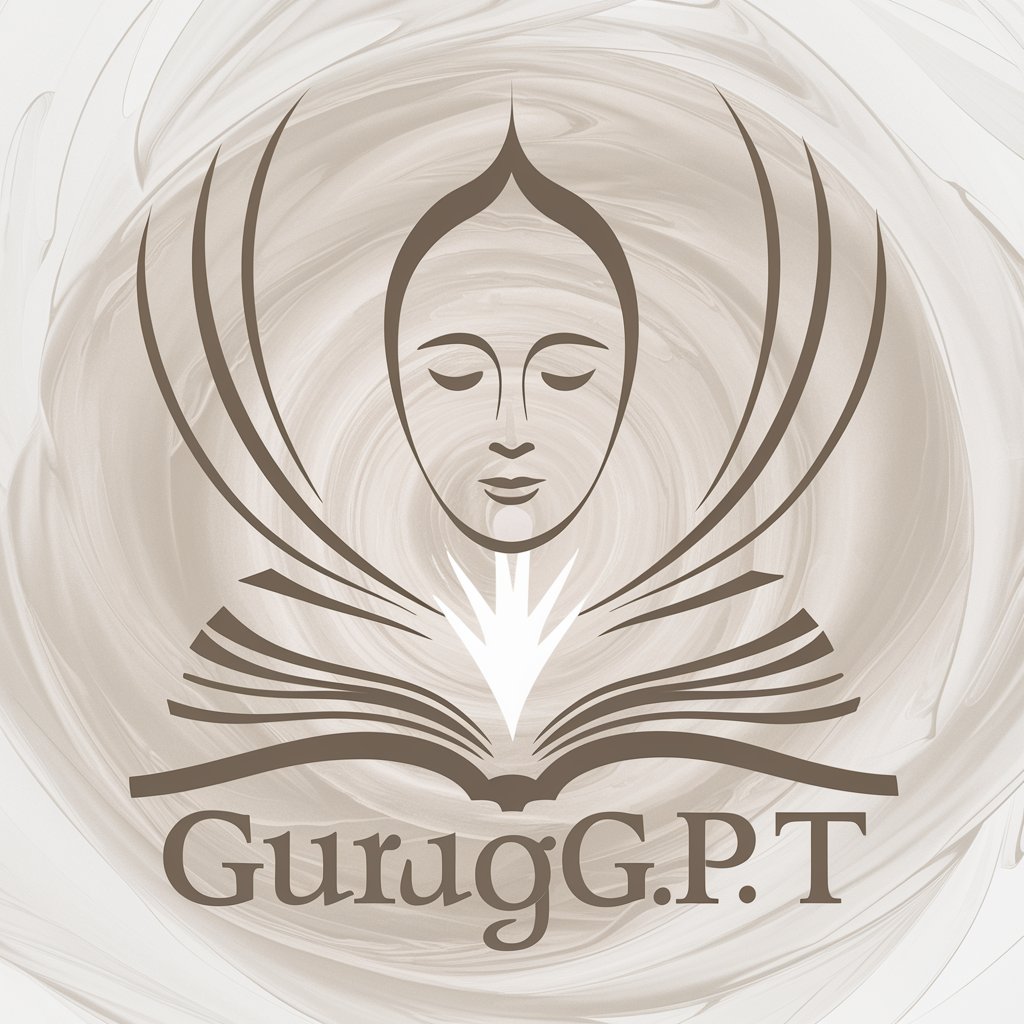
Academia de las Aves
Empowering bird enthusiasts with AI-driven insights.
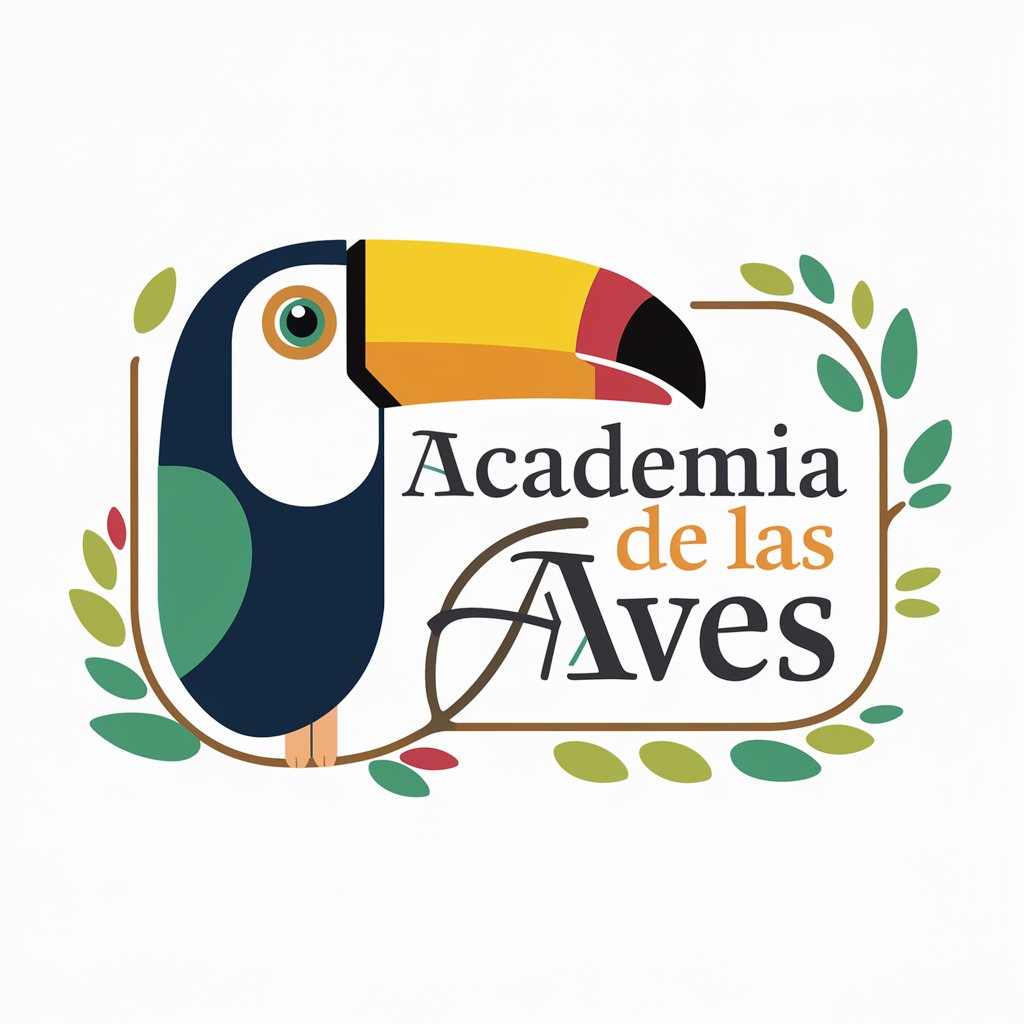
Frequently Asked Questions about Federated Learning Guide
What is Federated Learning Guide?
The Federated Learning Guide is an AI-powered tool designed to provide in-depth, comprehensive explanations and guidance on federated learning, including algorithms, data privacy, and practical applications.
How can I optimize my use of Federated Learning Guide?
Optimize your experience by clearly defining your questions or areas of interest, engaging with the tool's interactive features, and applying the insights to your federated learning projects or research.
What are some common use cases for Federated Learning Guide?
Common use cases include academic research, development of federated learning models, privacy-preserving data analysis, and enhancing understanding of federated learning principles and applications.
Can Federated Learning Guide help with specific technical issues in federated learning projects?
Yes, it can provide detailed advice on addressing specific technical challenges, such as model optimization, data distribution strategies, and privacy-enhancing techniques.
How does Federated Learning Guide handle queries about new or emerging trends in federated learning?
The tool is designed to stay updated with the latest federated learning research and developments, offering insights into emerging trends, technologies, and methodologies in the field.