Named Entity Recognition (NER) Agent-Text Analysis Tool
AI-powered insight into your text
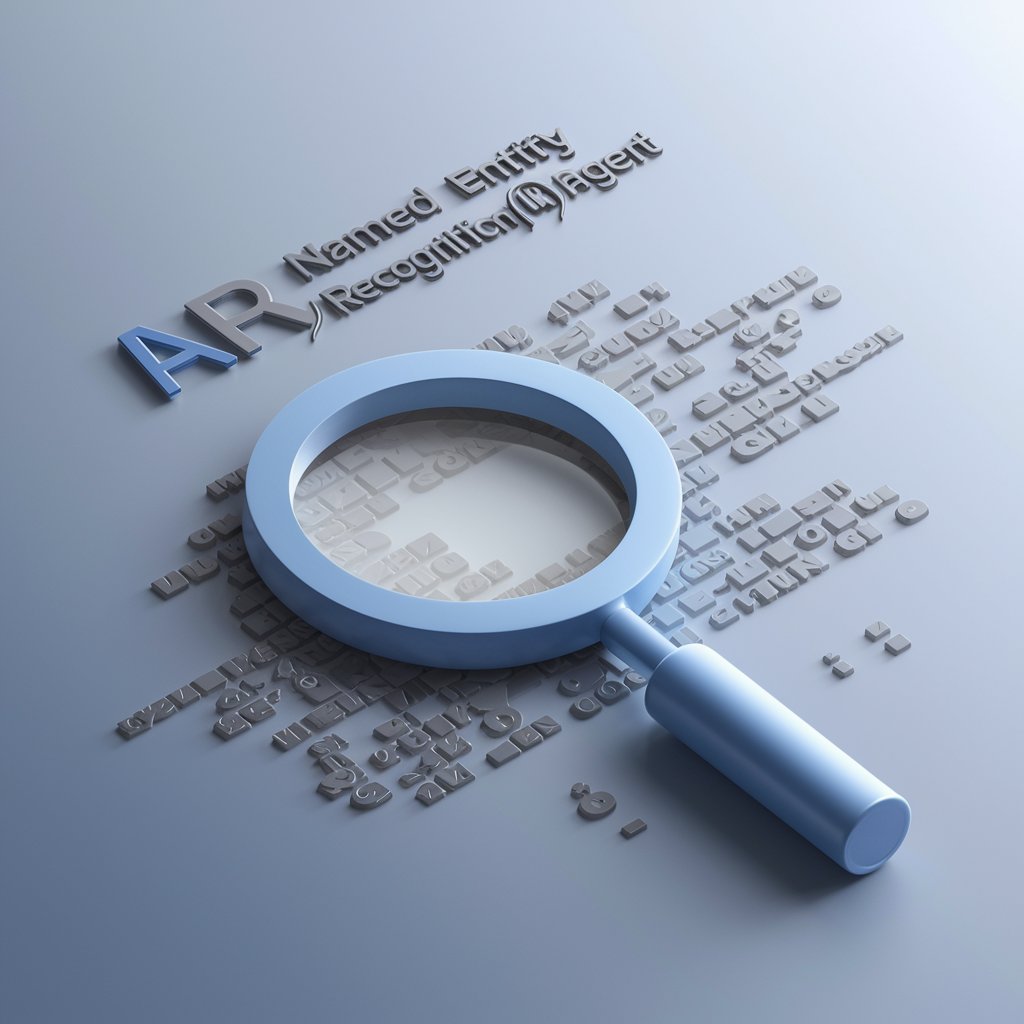
Highlight all named entities in the following text:
Identify and categorize the entities in this document:
List all persons, places, and organizations mentioned in the text:
Extract dates and times from the following passage:
Related Tools
Load More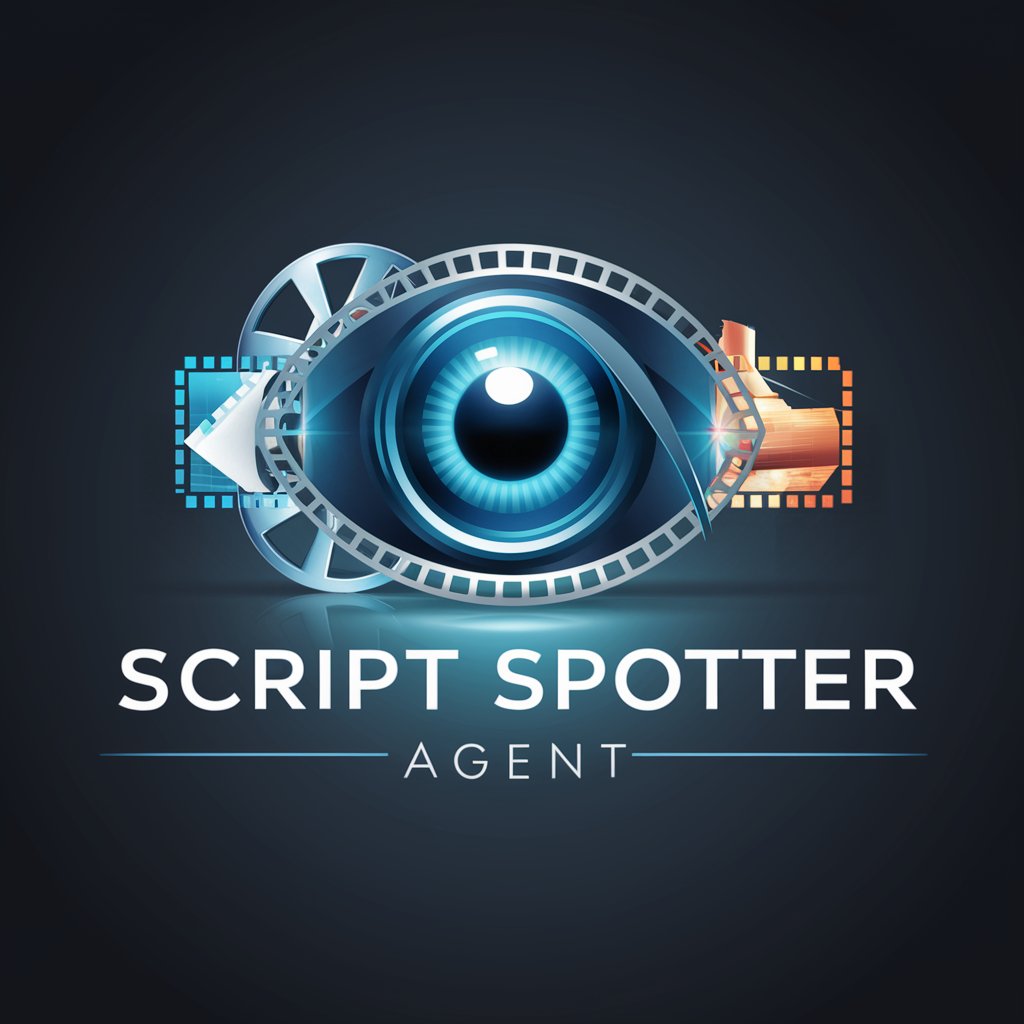
Script Spotter Agent
Your helpful assistant for identifying product placement opportunities in film scripts. It will suggest brand sponsorships seamlessly into your story, aiding in securing funding and enhancing your narrative with aligned sponsorships.
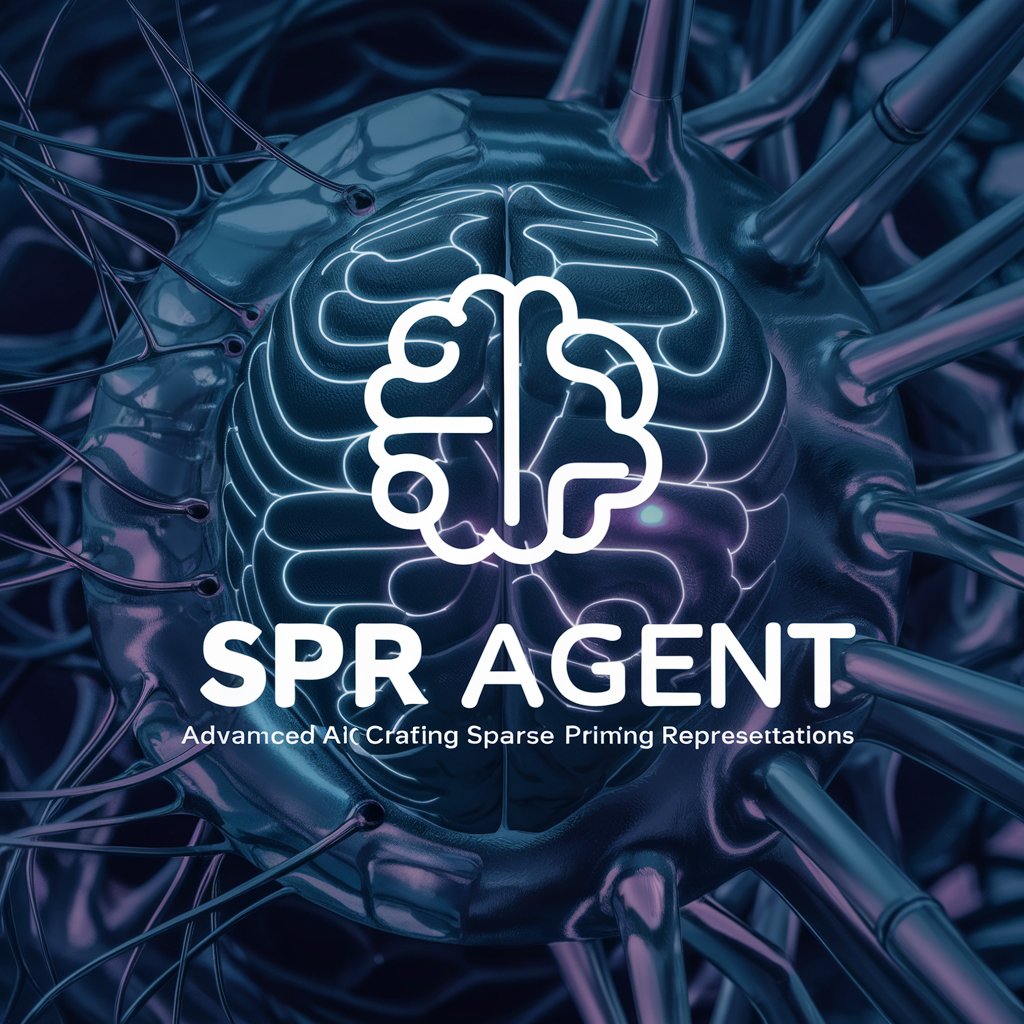
SPR Agent
This agent streamlines messy human input into Sparse Priming Representations (SPRs), effectively compressing the contained information into its core aspects for later usage by interactions with language models e.g. in Custom Instructions. It's inspired by
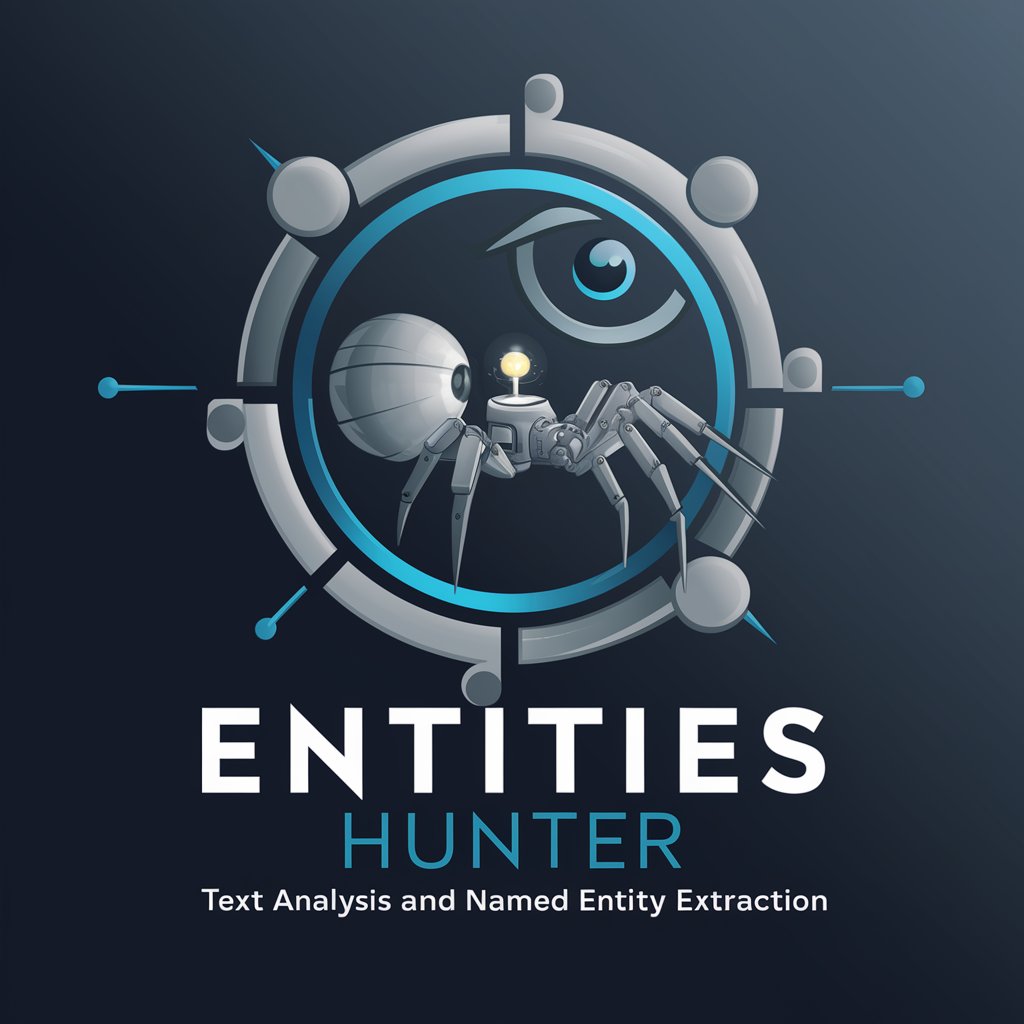
Entities Hunter
Spécialiste en extraction d'entités nommées avec liaison Wikipedia et formatage Json automatique à partir d'un texte. Créé par Pierre Gaudard pour inéolab. Version 0.0.5
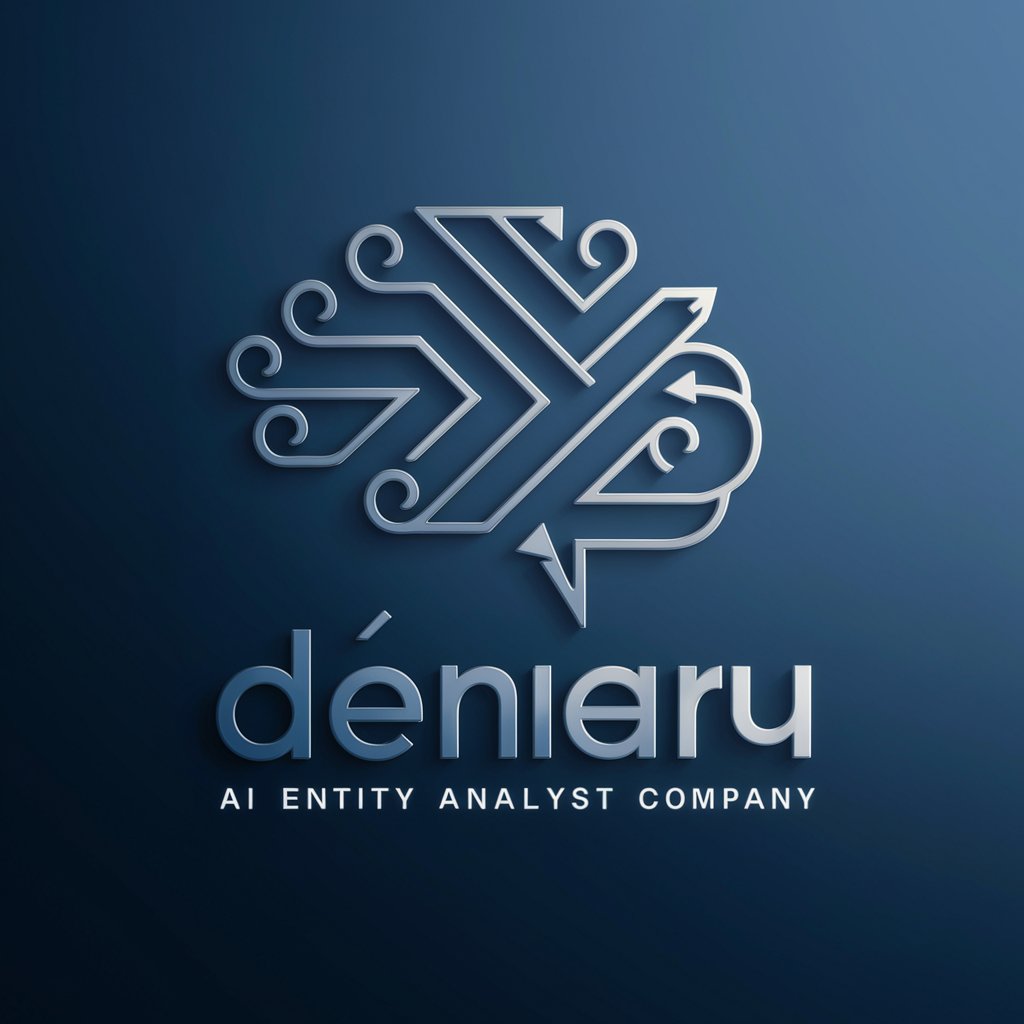
Entity Analyst GPT
Analyzes search queries using Google's patent 'Methods, systems, and media for interpreting entities in search queries'. https://patents.google.com/patent/US9116918B1/en
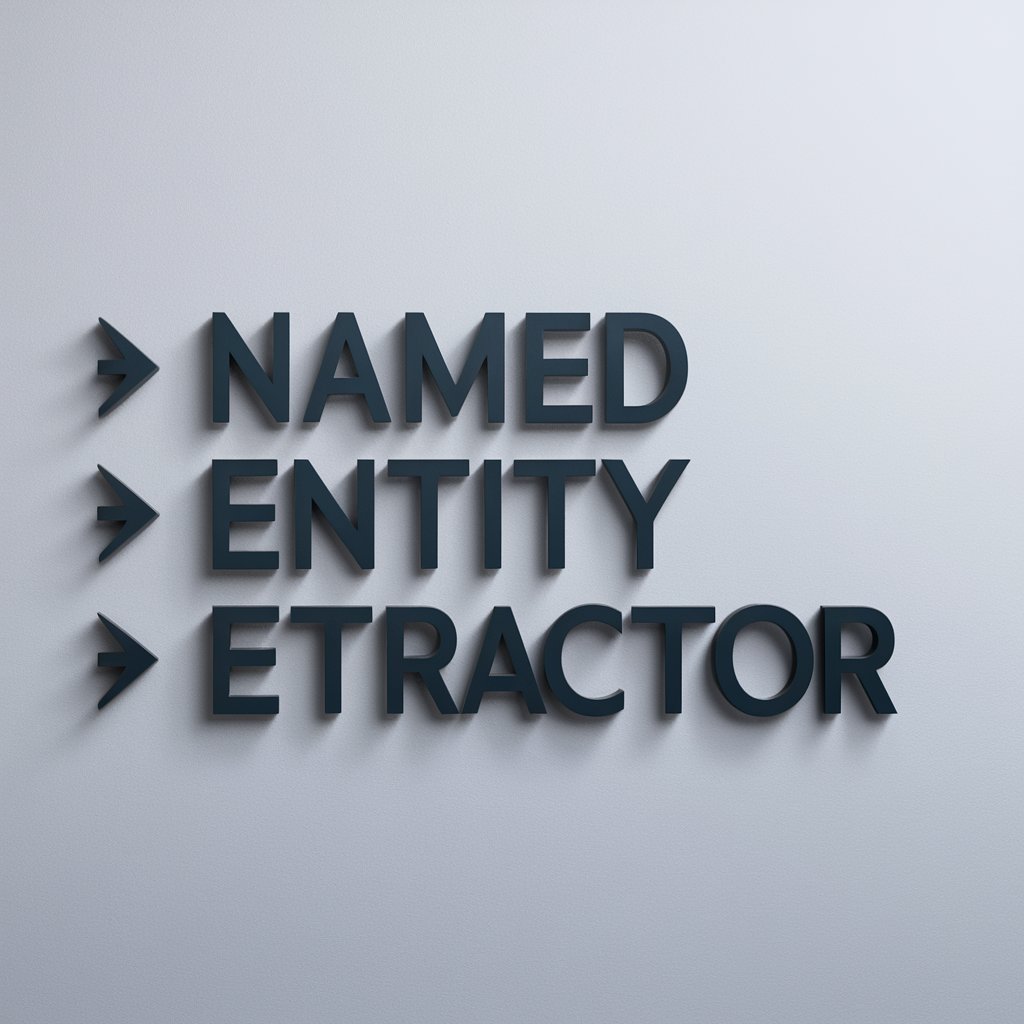
Named Entity Extractor
Entity Extractor GPT specializing in JSON formatted data.
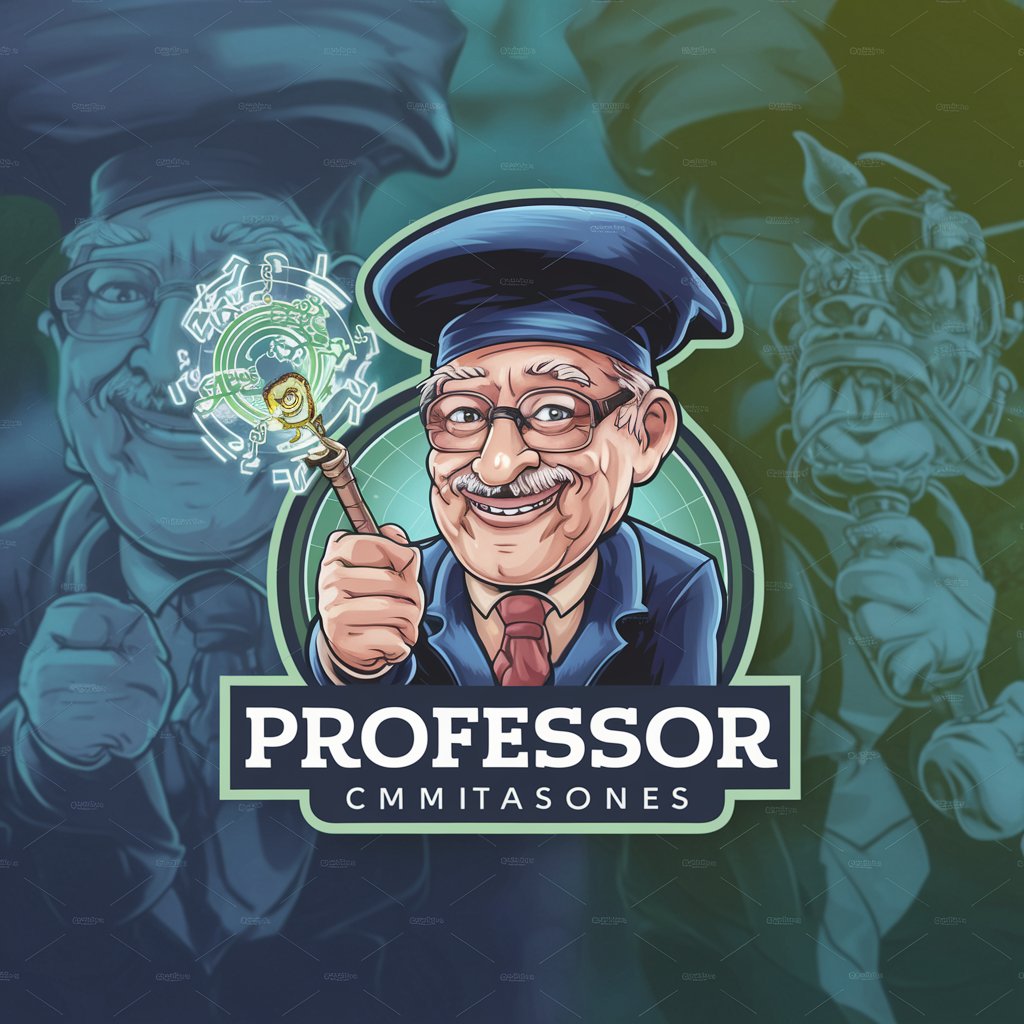
The Agents
Adept at aligning and achieving goals with expert agents.
20.0 / 5 (200 votes)
Overview of Named Entity Recognition (NER) Agent
The Named Entity Recognition (NER) Agent is a specialized artificial intelligence model designed to identify and categorize named entities in text into predefined categories such as persons, places, organizations, dates, times, products, and more. Its core function revolves around parsing unstructured text and highlighting significant elements, transforming them into structured data. This capability is crucial for extracting valuable information from vast amounts of text, enabling users to quickly understand and act upon the data within. For instance, analyzing news articles with the NER Agent could instantly reveal the key entities involved, such as the companies, locations, and individuals mentioned, along with any associated dates or events, providing a clear snapshot of the article's content without needing to read it in full. Powered by ChatGPT-4o。
Core Functions and Real-World Applications
Entity Identification and Categorization
Example
In a financial news article, the NER Agent can identify entities such as 'Apple Inc.' (Organization), 'Tim Cook' (Person), 'United States' (Location), and 'February 12, 2024' (Date).
Scenario
Journalists and analysts use this function to quickly gather pertinent information from publications, aiding in the creation of summaries or the analysis of industry trends.
Data Structuring from Unstructured Text
Example
Extracting structured information from customer reviews, such as product names, attributes, and sentiment, to populate a database for analysis.
Scenario
Businesses utilize this feature to understand customer sentiment towards specific products or services, facilitating better decision-making and strategy formulation.
Content Tagging and Organization
Example
Automatically tagging articles, research papers, or legal documents with relevant entities such as topics, names, and places for easier retrieval and organization.
Scenario
Librarians and researchers benefit from this capability by enhancing the searchability of large document databases, significantly reducing the time needed to find relevant documents.
Target User Groups
Journalists and Media Professionals
These users benefit from rapid identification of key entities in news articles or reports, facilitating quicker analysis and reporting on current events or stories.
Data Analysts and Researchers
For analysts sifting through large volumes of text data, the NER Agent helps in extracting and structuring relevant information, making data analysis more efficient and accurate.
Content Managers and Digital Archivists
Individuals responsible for managing large digital content libraries will find the NER Agent invaluable for tagging and organizing documents based on the entities they contain, improving searchability and accessibility.
How to Use the Named Entity Recognition (NER) Agent
1
Start with a visit to yeschat.ai for an immediate, no-login-required trial, bypassing the need for ChatGPT Plus.
2
Enter your text into the designated input field. This can range from paragraphs in an email to sections of a research paper.
3
Select 'Analyze' to submit your text. The NER Agent will process your text, identifying and classifying named entities within seconds.
4
Review the highlighted entities in your text. Each entity type, such as person, location, or organization, will be color-coded for easy identification.
5
Utilize the detailed list of entities provided post-analysis for research, content creation, or data organization purposes. Tips for an optimal experience include using clear, context-rich text and exploring the tool's settings to customize your analysis.
Try other advanced and practical GPTs
Prople
Empowering TOEIC Success with AI
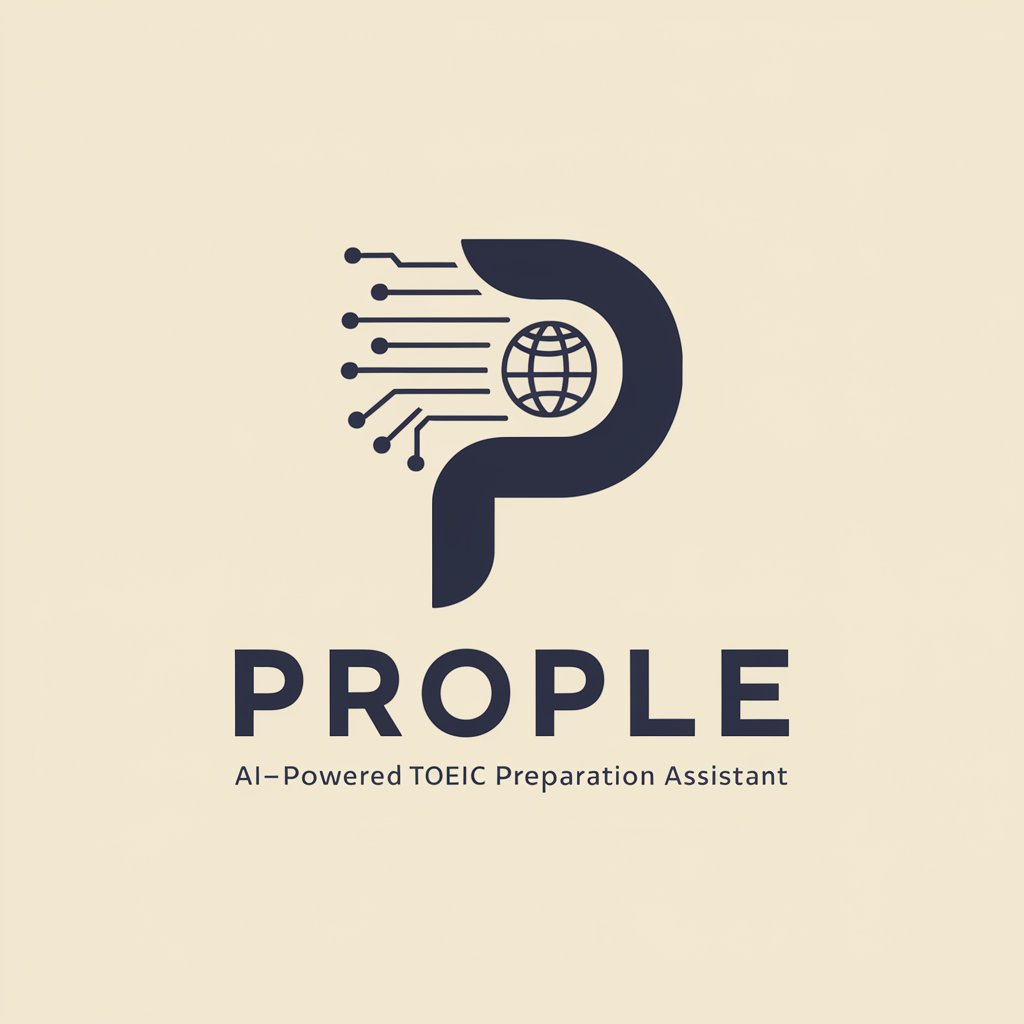
Time Management GPT
Optimize your time, enhance productivity with AI
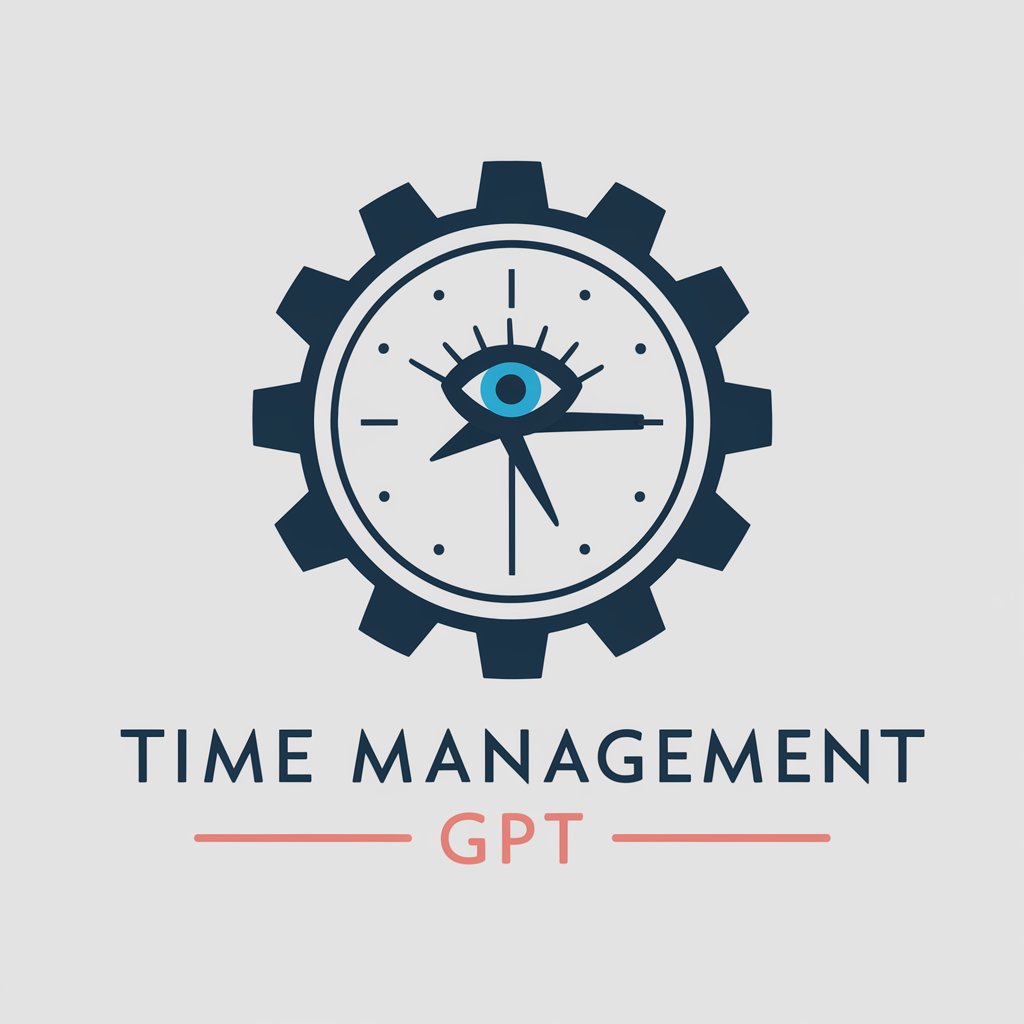
Cognitive Hacker
Unlock deeper understanding with AI
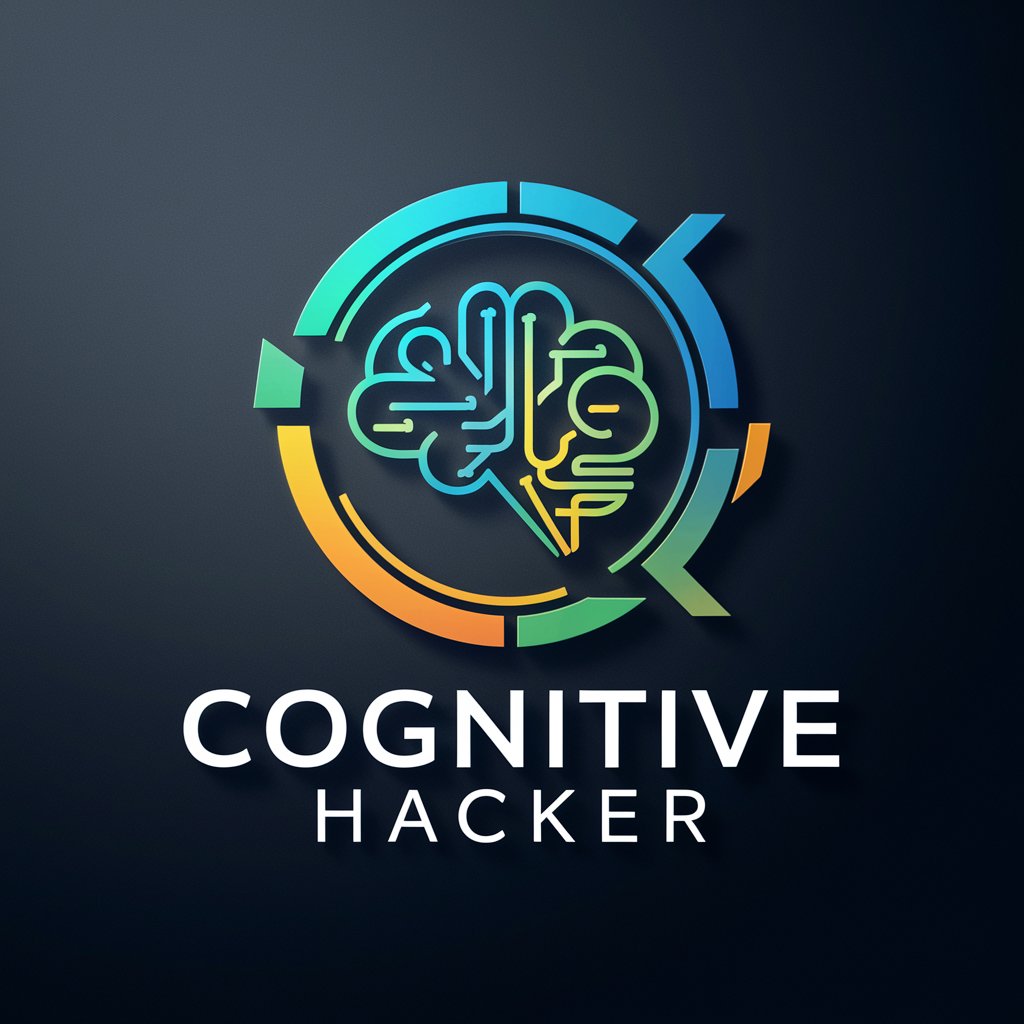
Sci-Fi Scenario Creator GPT
Craft immersive sci-fi worlds with AI.
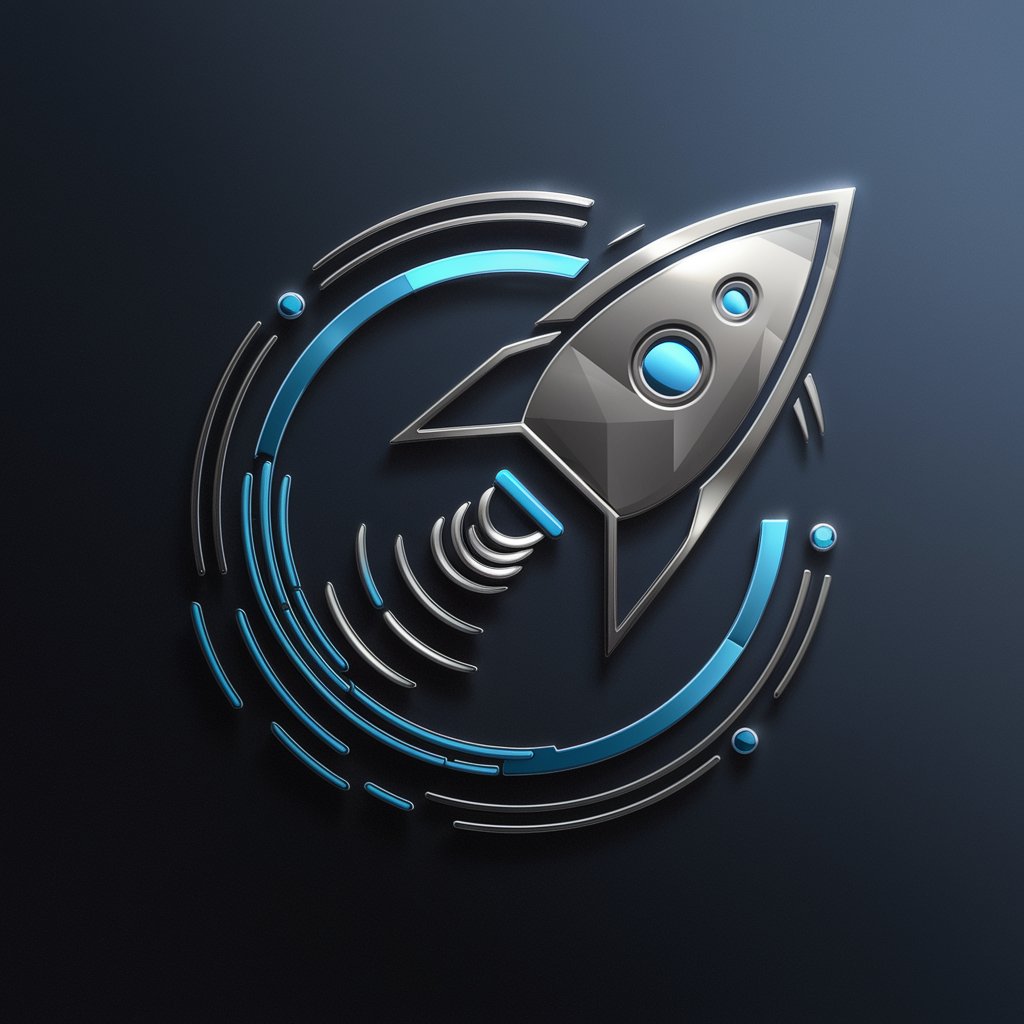
Regex Pro
Crafting regex made simple with AI.

영어 원서 인사이트 비서
Unveiling Linguistic Insights, Powered by AI
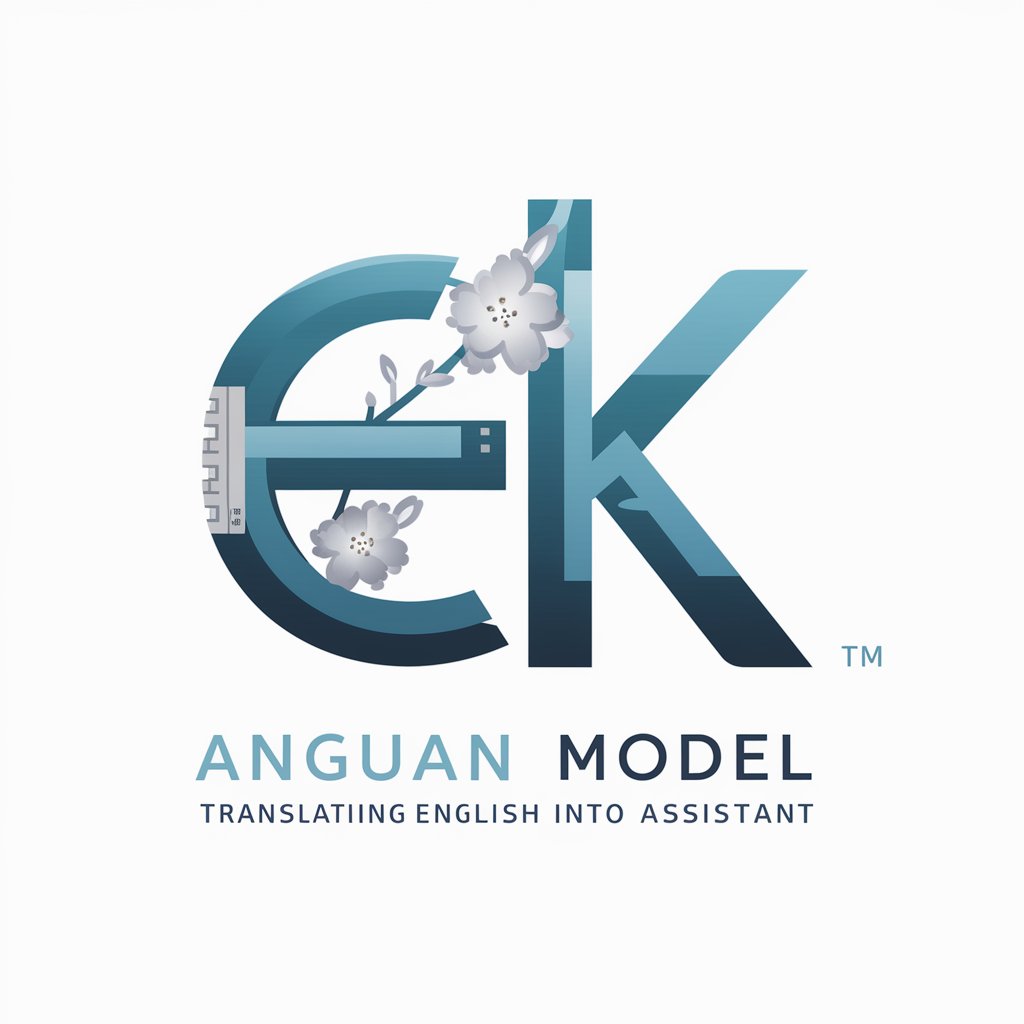
VASP Analyst
Empowering Compliance with AI
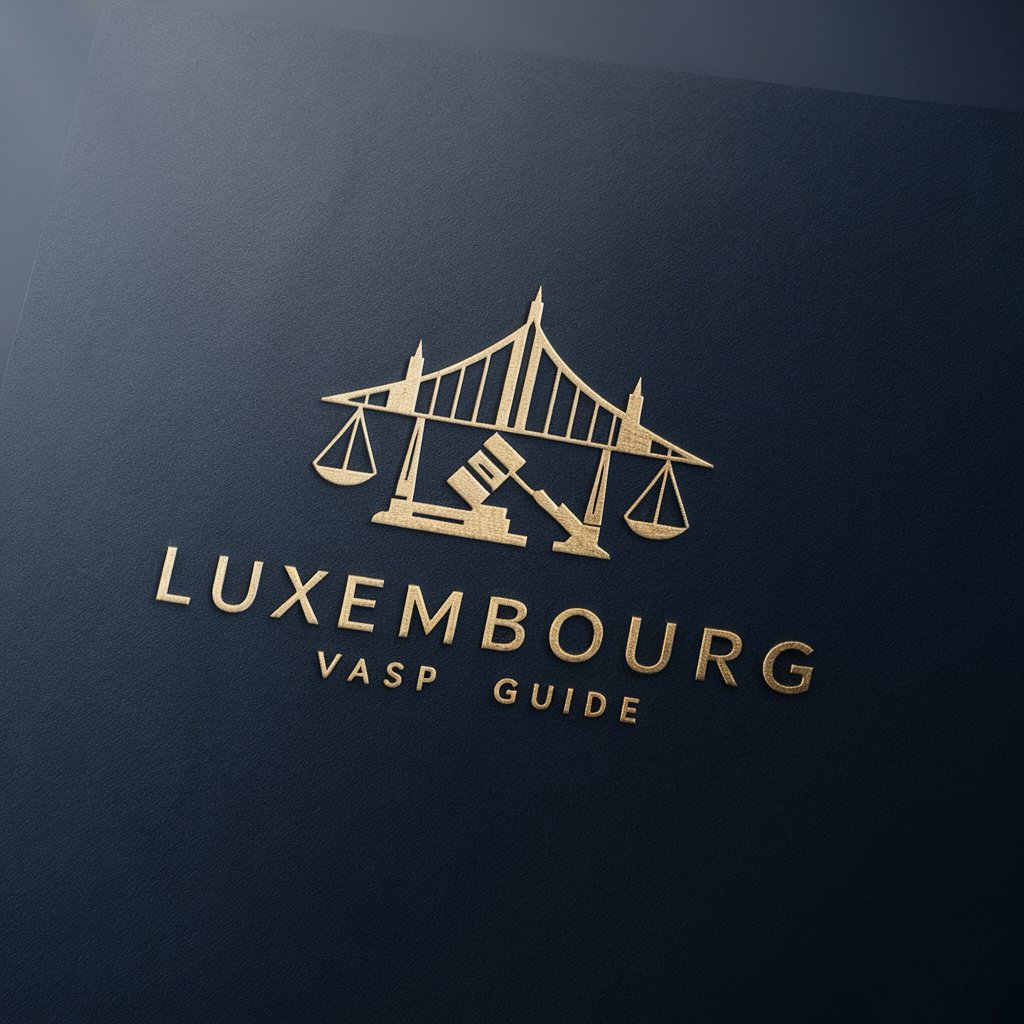
Perfect Prompt Generator
Crafting Precision AI Prompts
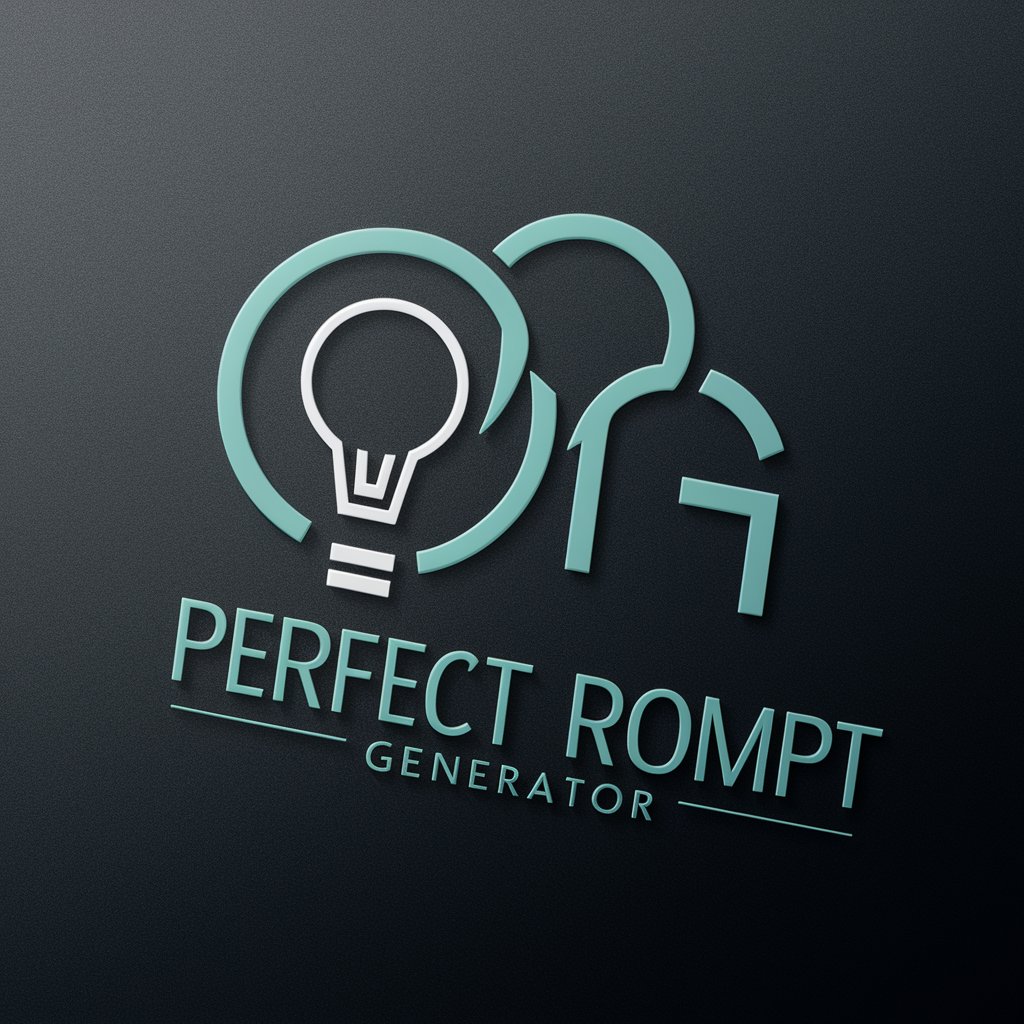
ကျေးလက်
Elevate Your Work with AI
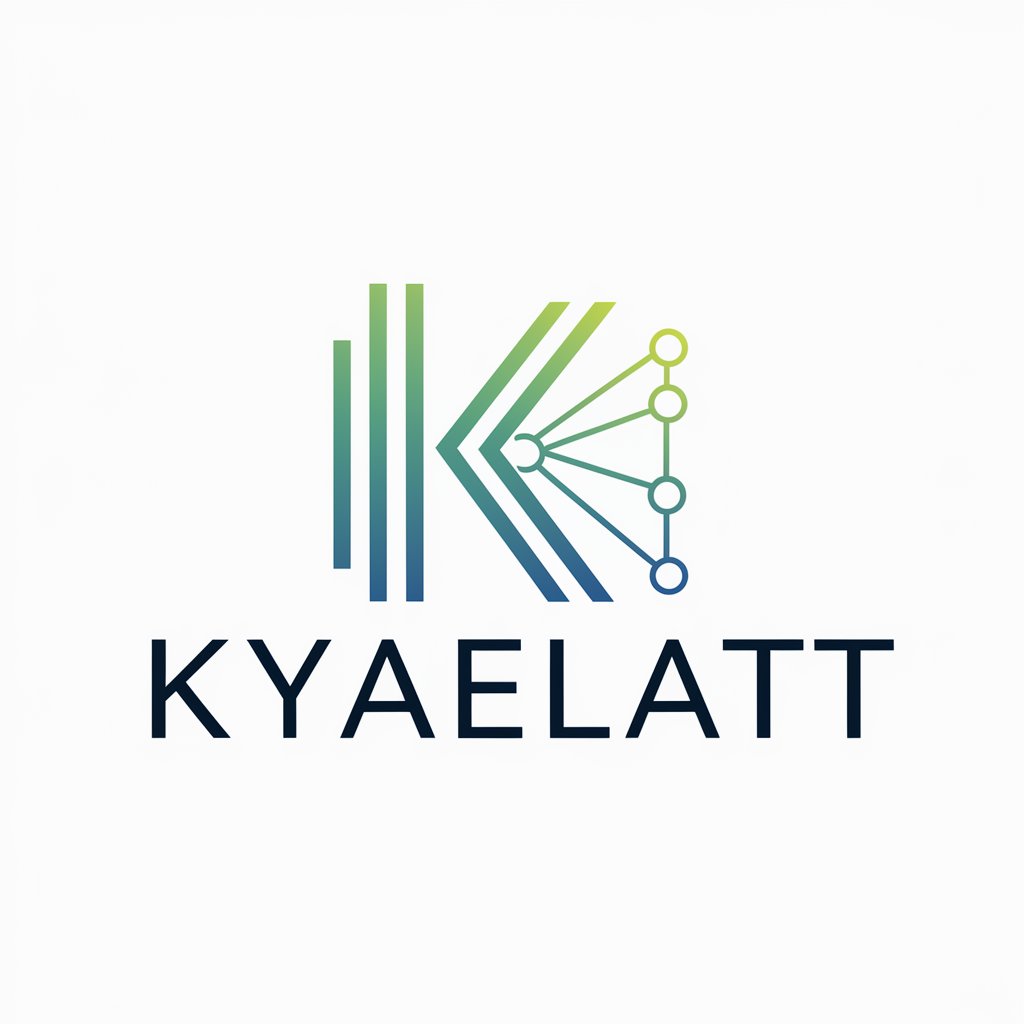
#AJIRU the DigiGénie
Empowering Creative Visions with AI
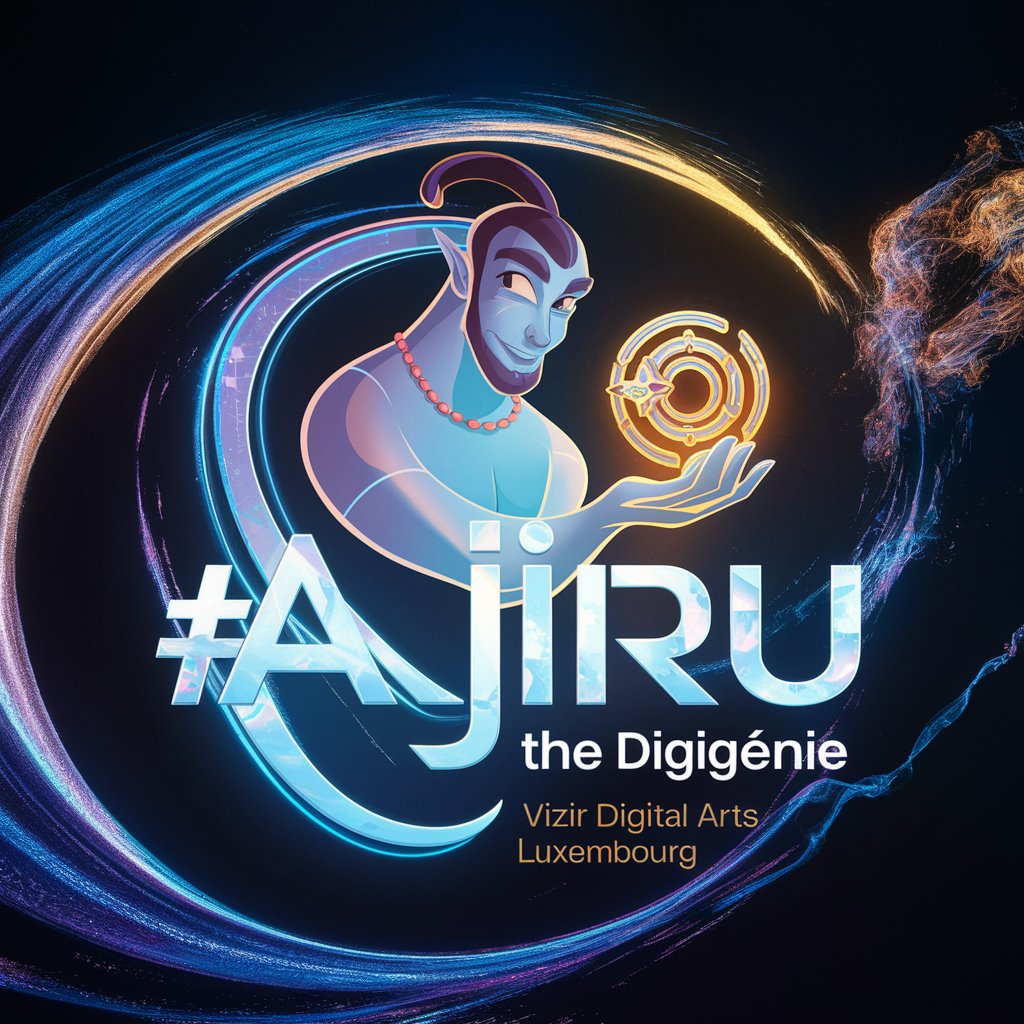
Service Savvy
Empowering Customer Service with AI
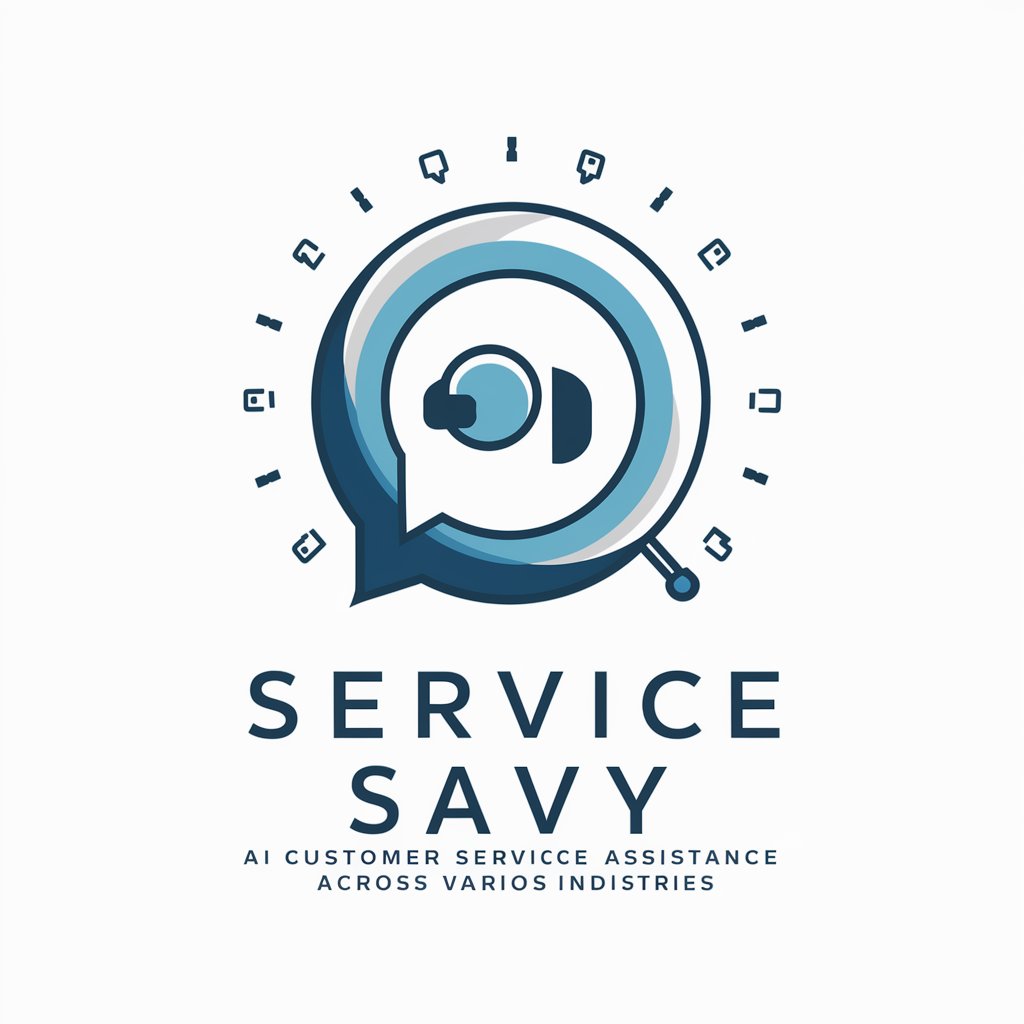
Practical Roadmap
AI-powered marketing insights at your fingertips
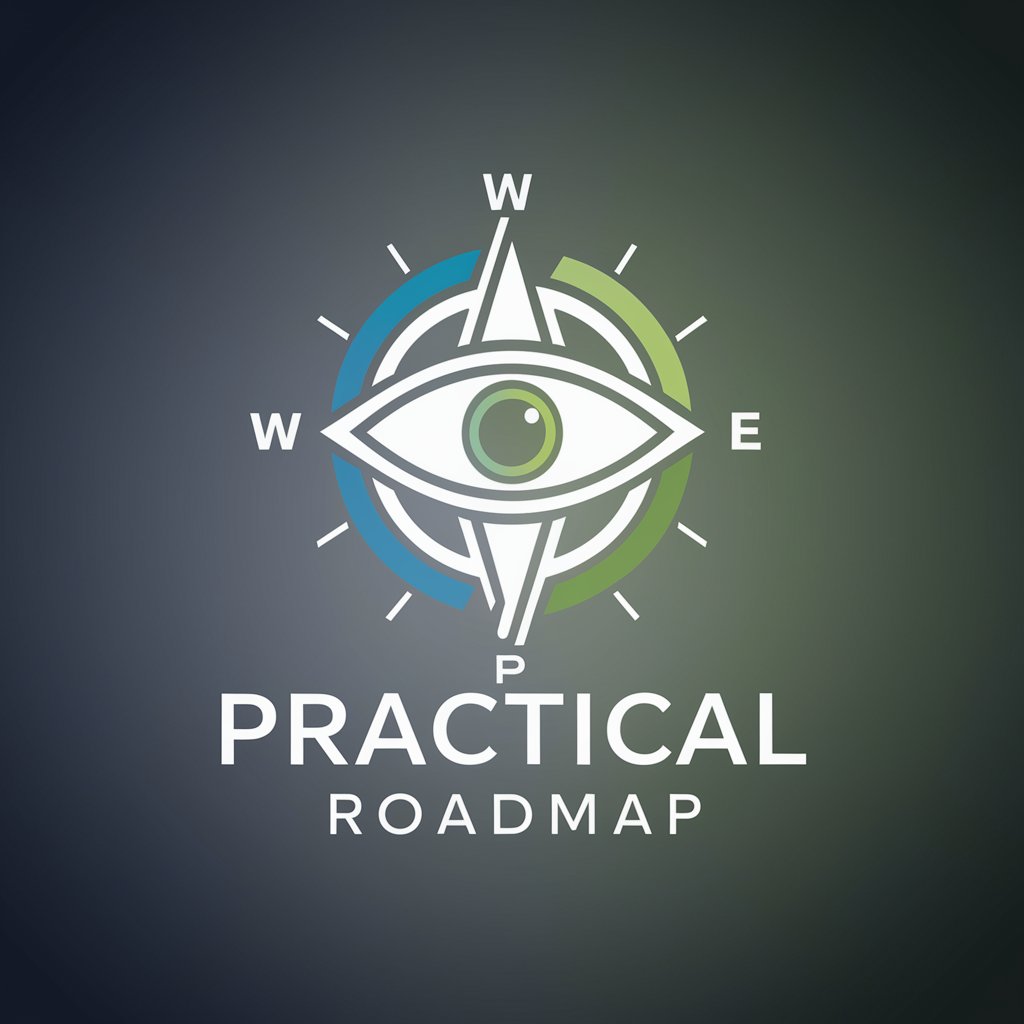
Frequently Asked Questions about the NER Agent
What is Named Entity Recognition (NER) and how does the NER Agent utilize it?
Named Entity Recognition (NER) is a form of natural language processing that identifies and classifies named entities in text into predefined categories such as names of persons, organizations, locations, expressions of times, quantities, monetary values, percentages, etc. The NER Agent applies advanced algorithms to analyze texts across multiple languages, offering precise categorization and making it easier to extract valuable information.
Can the NER Agent identify entities in languages other than English?
Yes, the NER Agent is designed to recognize and classify named entities in multiple languages, leveraging its sophisticated language models to ensure accurate identification across diverse linguistic contexts.
What are some common use cases for the NER Agent?
Common use cases include content analysis for media monitoring, data extraction for academic research, enhancing search engine optimization by identifying key entities in web content, organizing large datasets in business intelligence, and improving customer experience by analyzing feedback for specific mentions of products or services.
How accurate is the NER Agent in identifying and classifying entities?
The NER Agent boasts high accuracy rates thanks to its utilization of cutting-edge natural language processing technologies. Its performance continuously improves as it learns from new text inputs and adjusts to various contexts and nuances in language.
Can the NER Agent be integrated with other software or platforms?
While the current iteration is designed for direct use through a web interface, future updates may include API capabilities for integration with other software or platforms, enhancing its versatility and applicability in a wide range of technological ecosystems.