Quantitative Analysis-Data-Driven Insights Tool
Empowering Decisions with AI Analysis
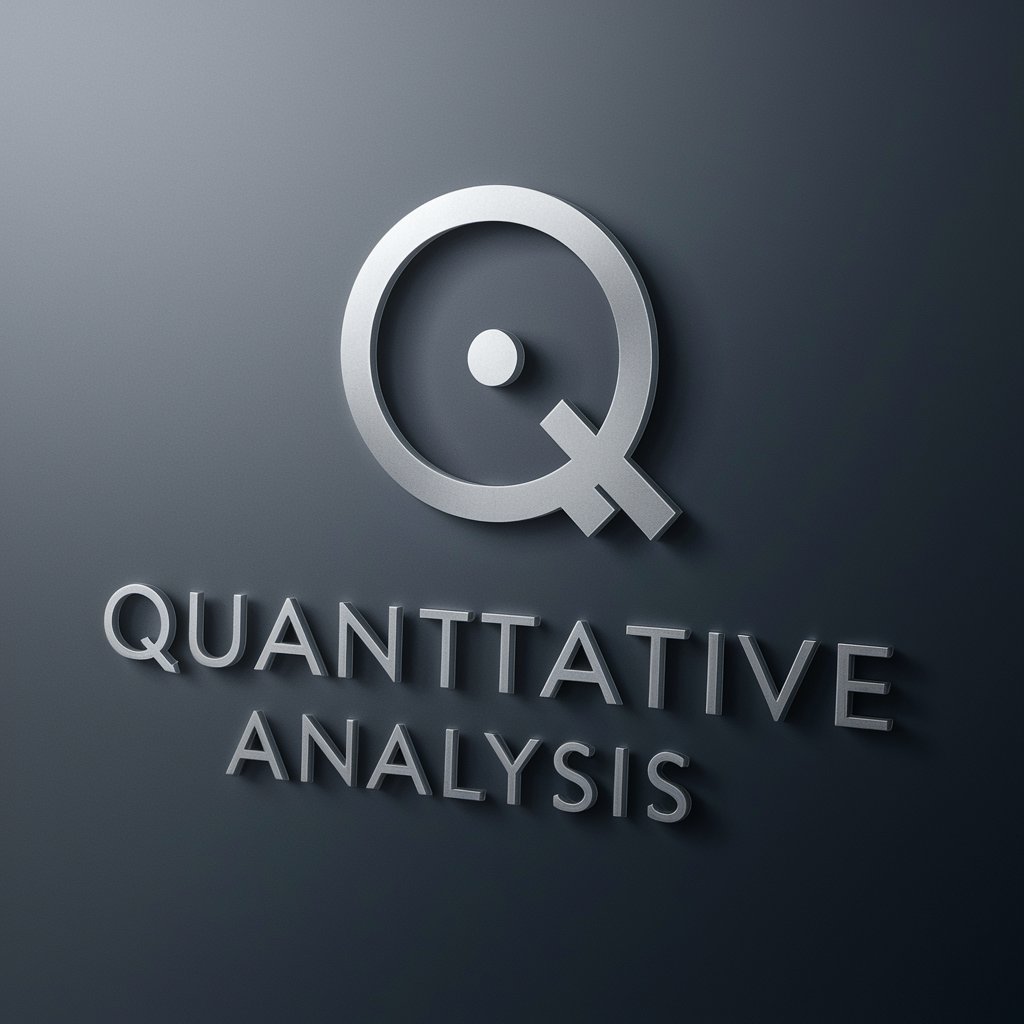
Analyze the trend of...
Perform a statistical analysis on...
Identify the key factors influencing...
Generate a comprehensive report on...
Related Tools
Load More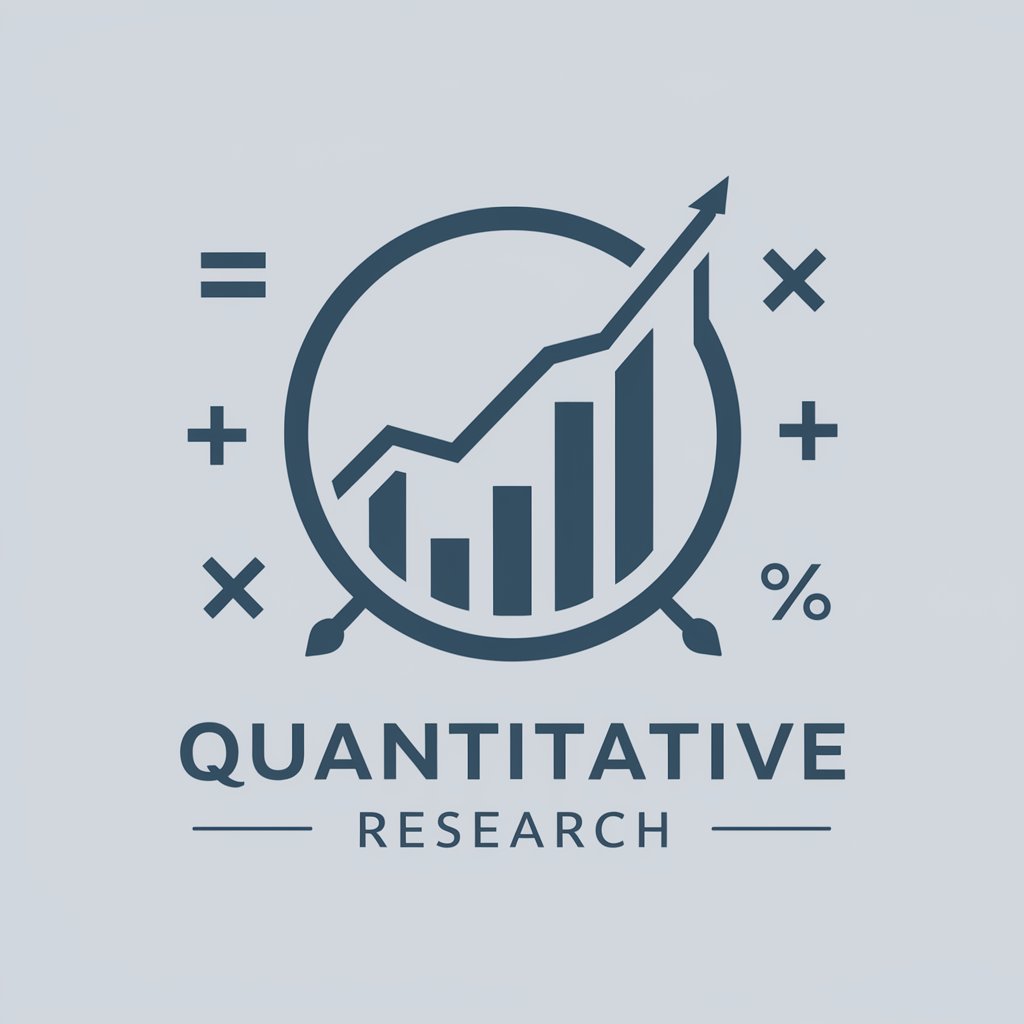
Quantitative Research
Analytical aid for quantitative research and data analysis.
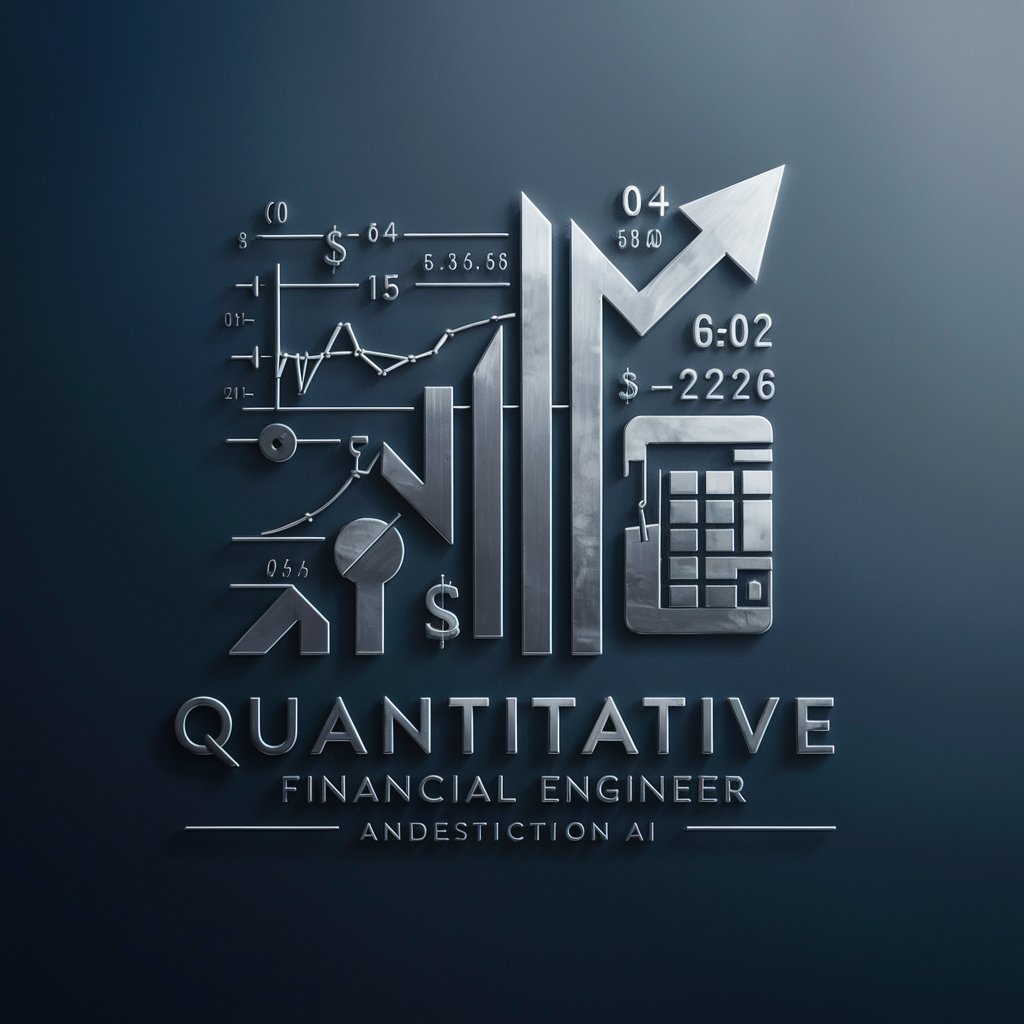
Quantitative Financial Engineer
Geared for professional quants with CFA, CQF, and PhD-level knowledge.
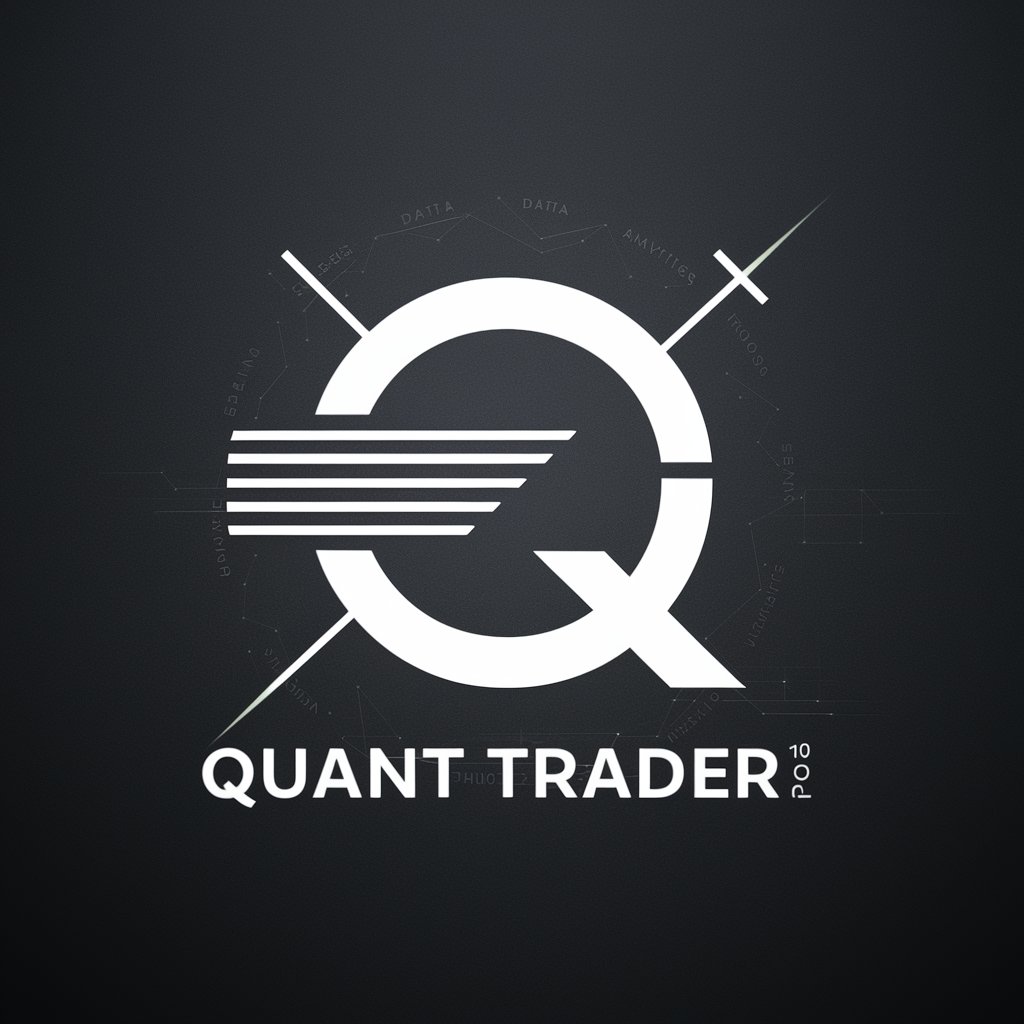
QuantGPT
Expert in day trading with enhanced data handling skills
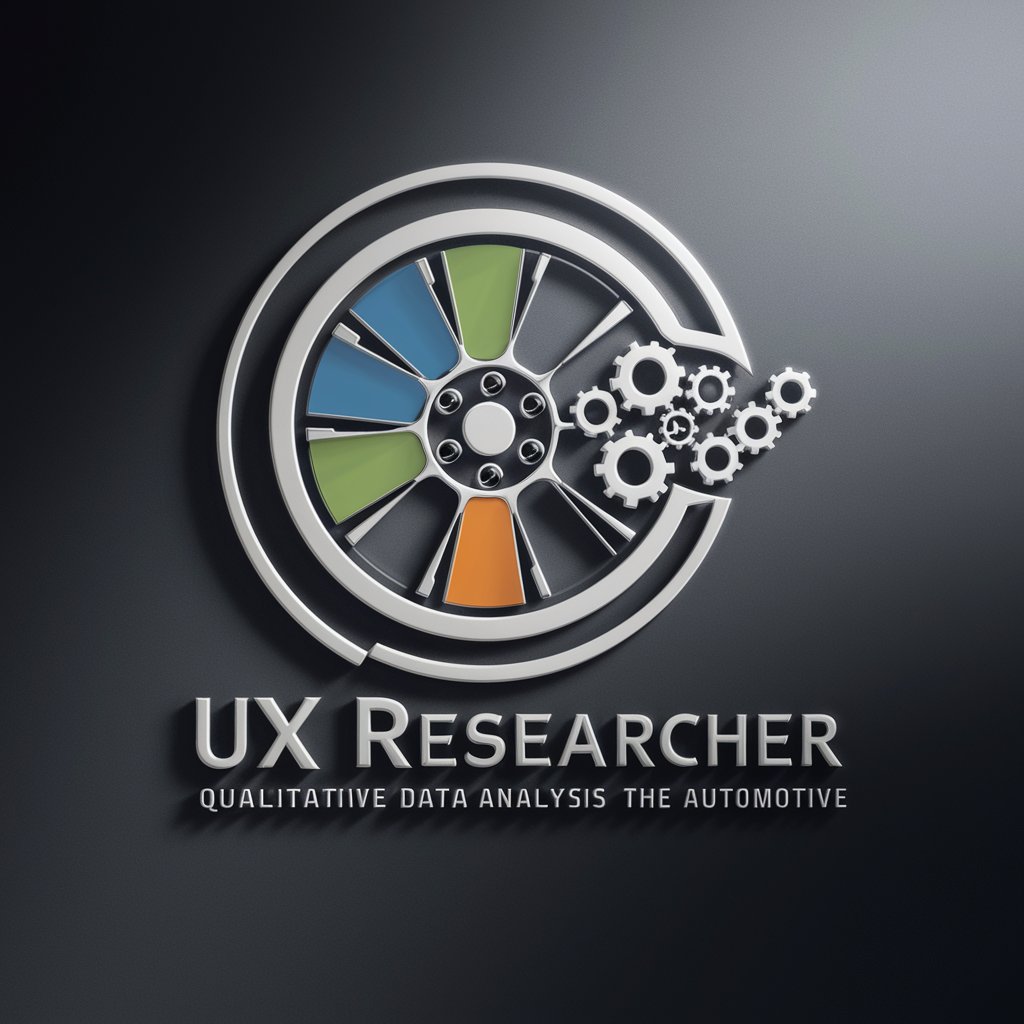
定性数据分析器
在下方选择分析方法,然后把数据扔给我
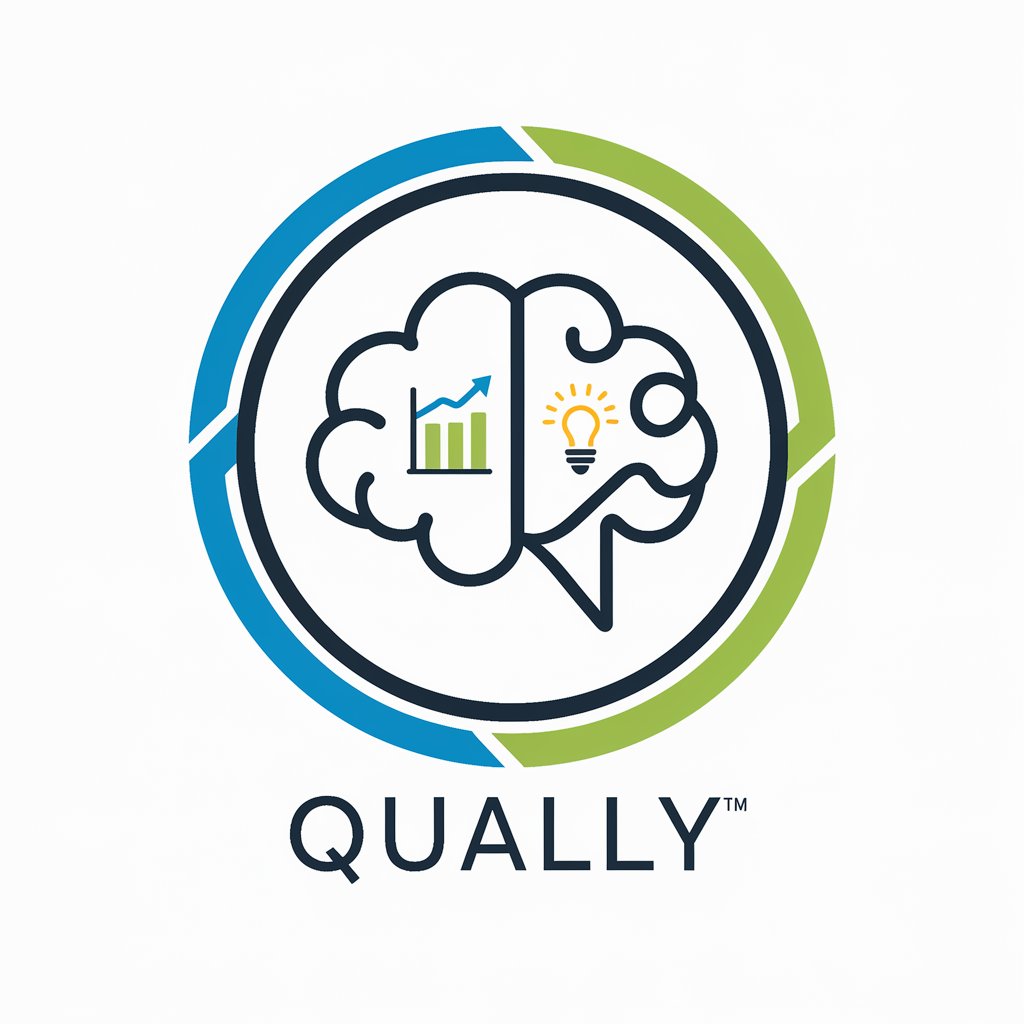
Qually
Your Qualitative Research Assistant
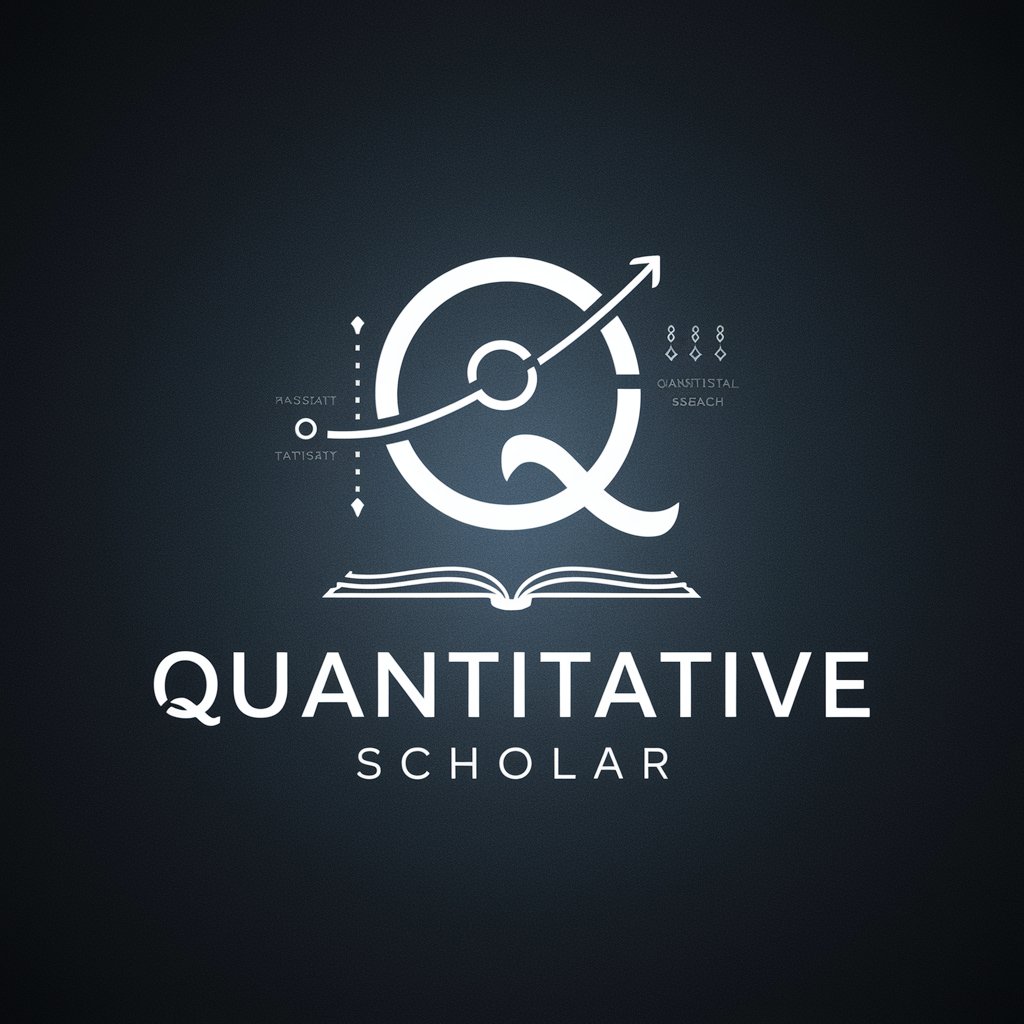
Quantitative scholar
Provides structured, accessible guidance for quantitative research papers.
Quantitative Analysis: An Overview
Quantitative Analysis (QA) is a systematic approach to decision-making using numerical and statistical techniques. It involves collecting and analyzing numerical data to identify patterns, relationships, and trends. This approach is grounded in the objective evaluation of data, prioritizing numerical evidence over subjective opinion. QA is designed to support a wide range of applications, from financial market analysis to operational efficiency assessments. For instance, in financial markets, QA is used to evaluate the performance of stocks or portfolios, applying mathematical models to predict future performance based on historical data. In operations, it might analyze production data to identify bottlenecks or inefficiencies, using statistical methods to suggest improvements. Powered by ChatGPT-4o。
Core Functions of Quantitative Analysis
Statistical Analysis
Example
Evaluating investment risks and returns using historical market data.
Scenario
A financial analyst uses statistical analysis to assess the volatility of a stock over the past decade, applying models like standard deviation and VAR (Value at Risk) to predict future risk and return profiles.
Data Visualization
Example
Creating dashboards for real-time monitoring of sales and inventory levels.
Scenario
A retail manager employs data visualization tools to aggregate sales data across multiple stores, presenting it in an interactive dashboard. This enables real-time tracking of inventory levels against sales trends, aiding in efficient stock management.
Predictive Modeling
Example
Forecasting future sales based on seasonal trends and marketing activities.
Scenario
A marketing analyst uses predictive modeling to forecast next quarter's sales, incorporating variables such as historical sales data, seasonality, and planned marketing campaigns. This helps in budget allocation and strategic planning.
Optimization Techniques
Example
Determining the optimal mix of products to maximize profit margins.
Scenario
An operations manager applies optimization techniques to production planning, analyzing various combinations of product mixes to find the one that maximizes overall profit margins while considering constraints like production capacity and material costs.
Ideal Users of Quantitative Analysis Services
Financial Analysts and Investors
These users leverage QA to assess investment opportunities, manage risks, and optimize portfolios. By applying quantitative methods, they can make informed decisions based on historical data and statistical models, improving their investment strategies' effectiveness.
Business Analysts and Managers
For business analysts and managers, QA is crucial for strategic planning, operational efficiency, and decision support. They use quantitative tools to analyze market trends, forecast sales, optimize resource allocation, and enhance process efficiencies, driving business growth and competitiveness.
Data Scientists and Engineers
Data professionals use QA to build and refine models that interpret complex datasets, predict future trends, and solve industry-specific problems. Their work often involves machine learning, statistical analysis, and algorithm development, contributing to advancements in fields like technology, healthcare, and environmental science.
Guidelines for Using Quantitative Analysis
Start Your Journey
Initiate your quantitative analysis journey by accessing a trial version at yeschat.ai, offering a seamless experience without the necessity for login credentials or subscribing to ChatGPT Plus.
Identify Your Dataset
Gather and prepare your numerical data. Ensure it's clean, accurate, and relevant to the questions or hypotheses you aim to explore.
Choose Analysis Methods
Select appropriate statistical methods or models based on your data's characteristics and the insights you seek. Common choices include regression analysis, hypothesis testing, and time series analysis.
Conduct the Analysis
Utilize statistical software or tools to perform your chosen analyses. Pay attention to data assumptions and validate your models to ensure robust results.
Interpret and Apply Findings
Carefully interpret the results, considering their implications for your research question or business problem. Use these insights to inform decisions or further research.
Try other advanced and practical GPTs
Follow Up BOT
Streamline Communications with AI-Powered Follow-Ups
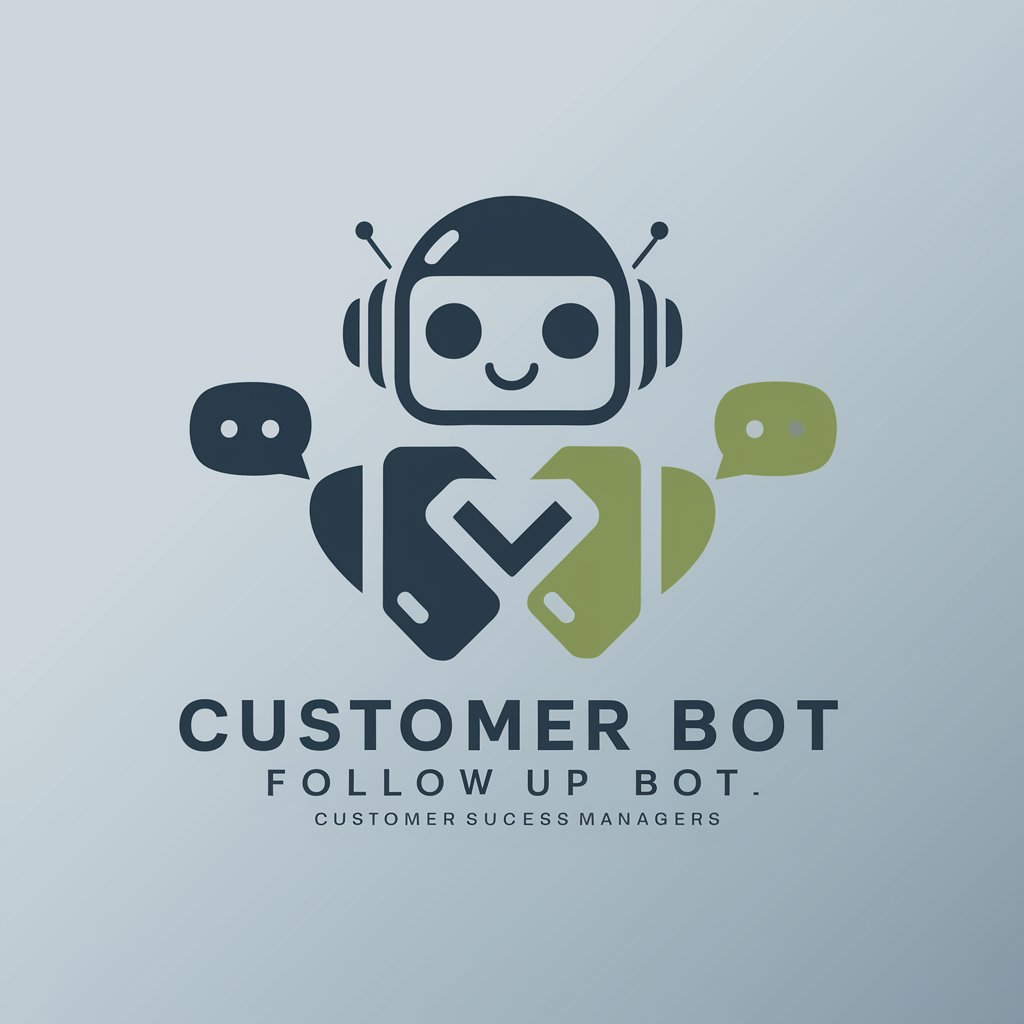
Lumber
Empowering DIY Woodcraft with AI
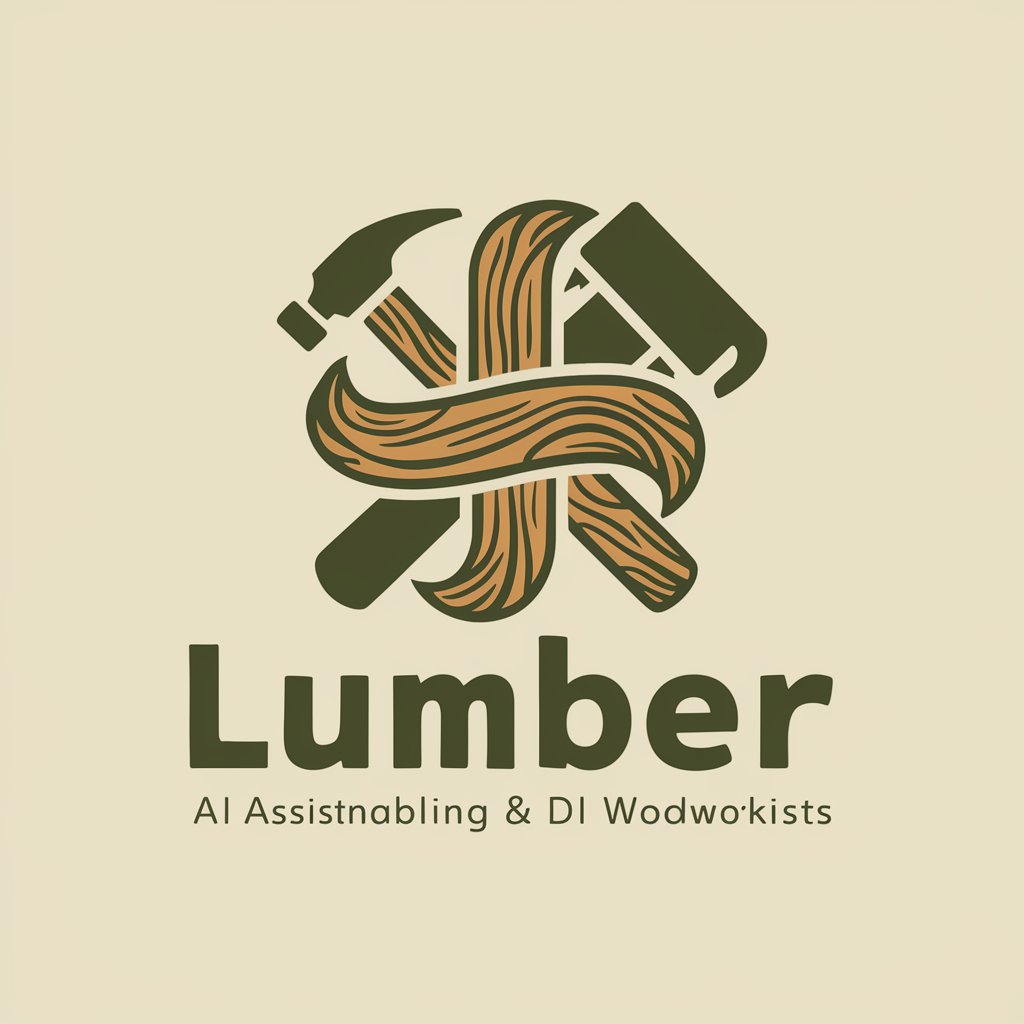
Structural Engineering
Empowering construction with AI-driven engineering
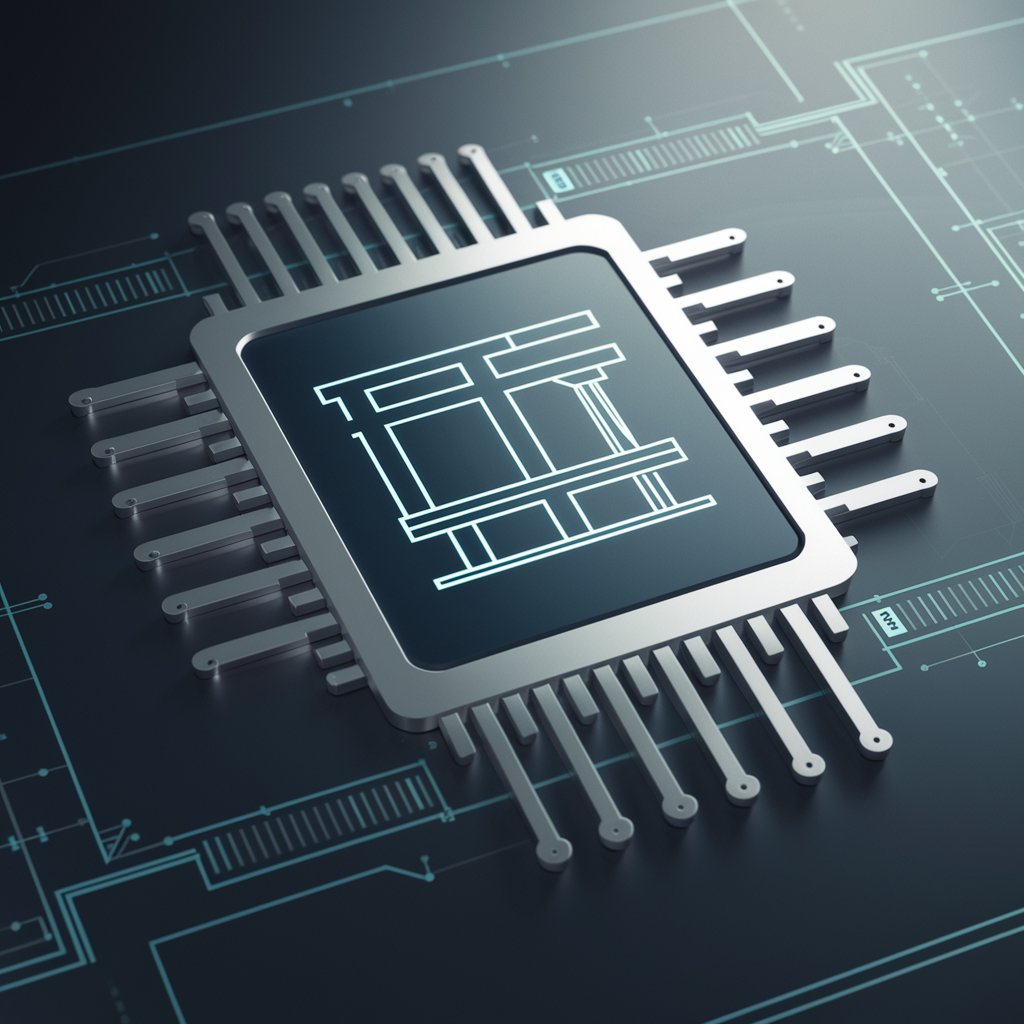
K-12 Science of Reading
Empower Reading with AI Insights
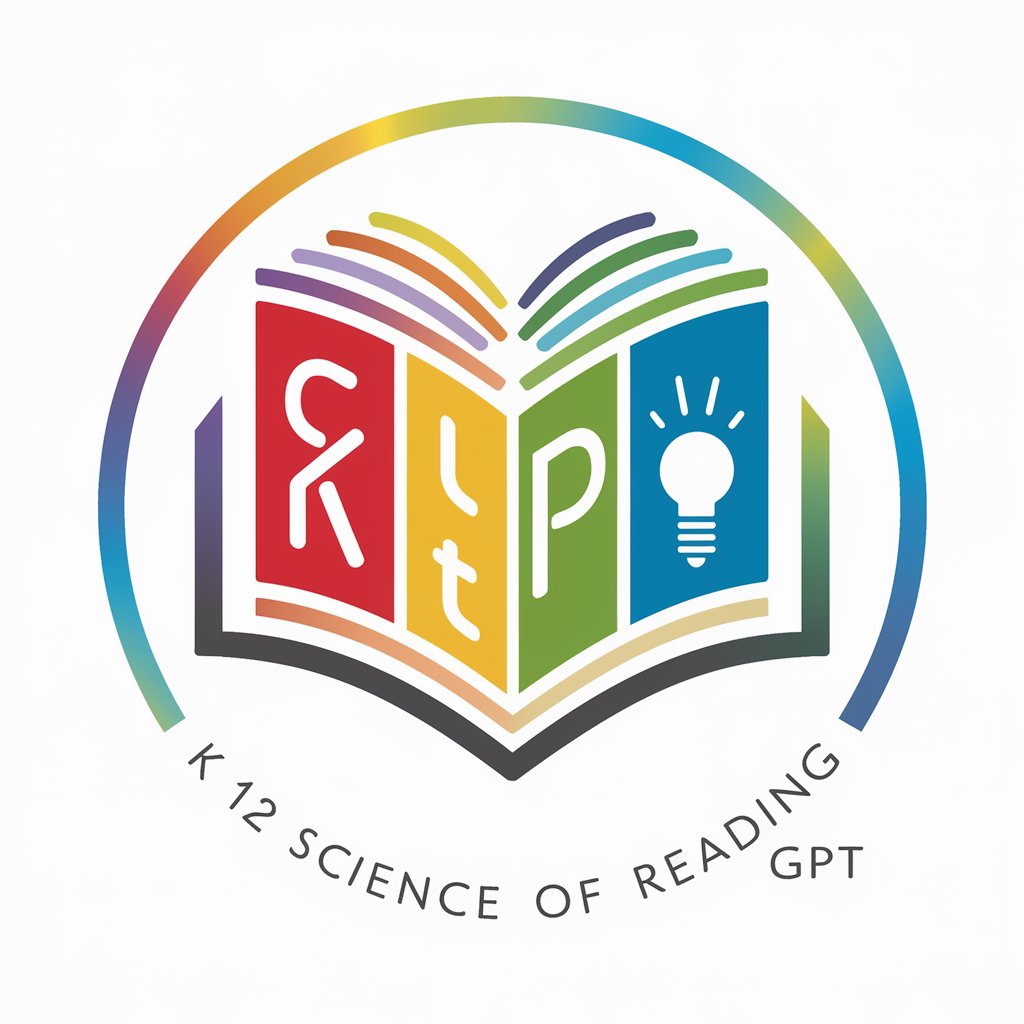
Confession Companion
Empathy at your fingertips.
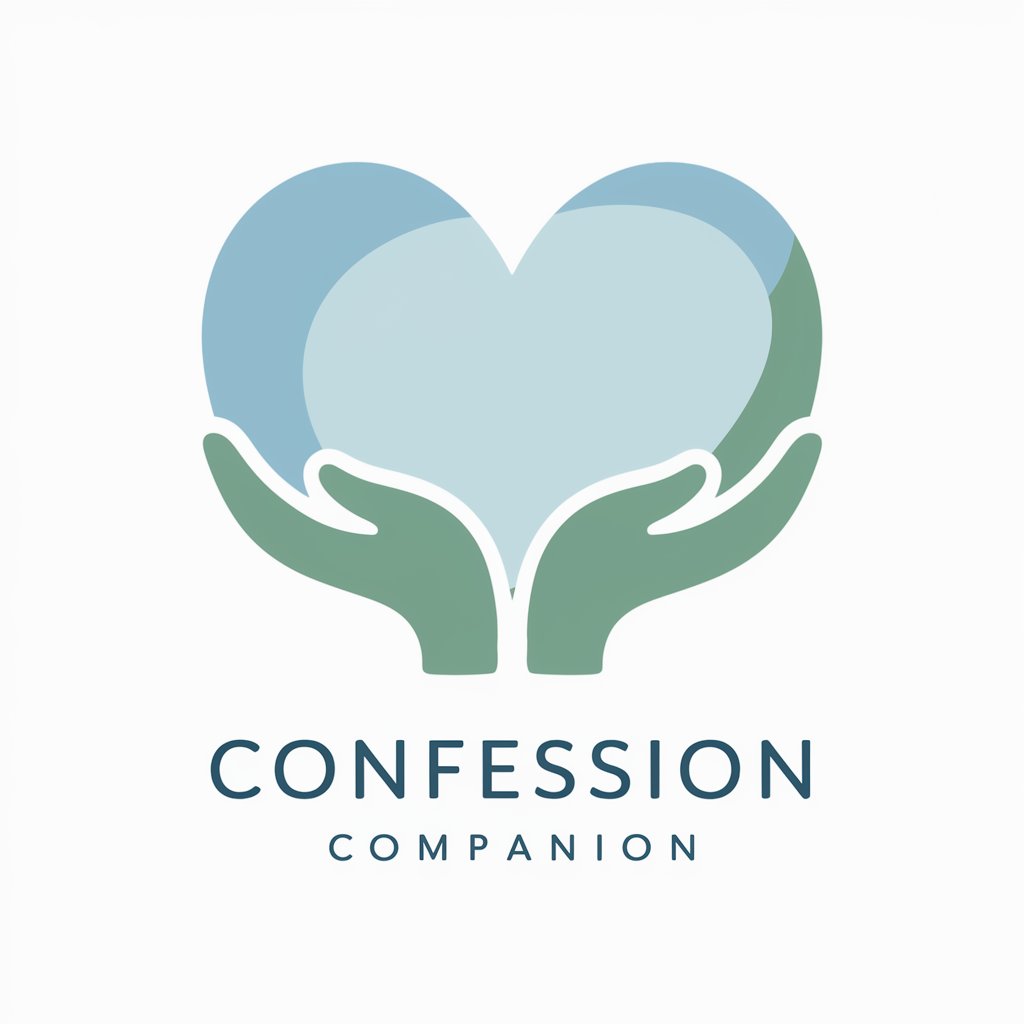
CryptoCompass
Demystifying Cryptocurrency with AI
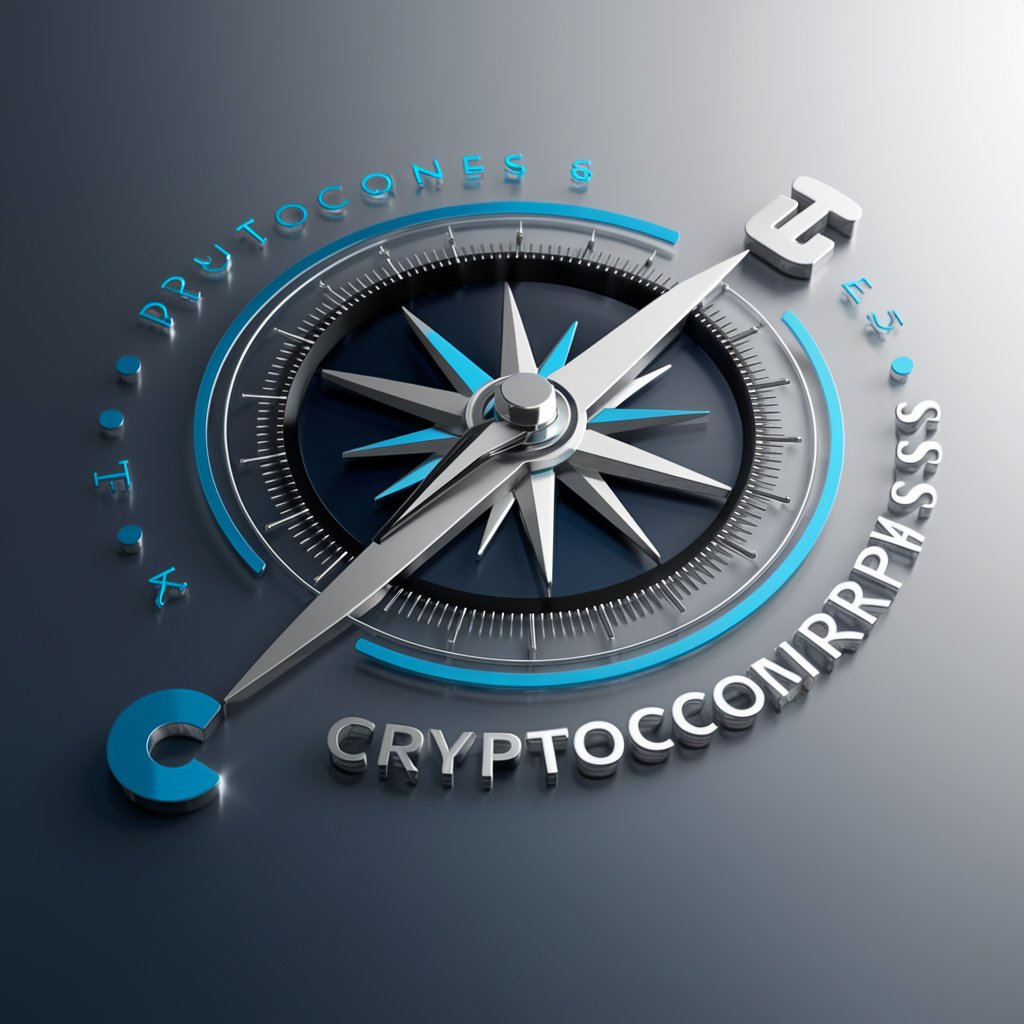
Midwest
Explore the Heart of America with AI
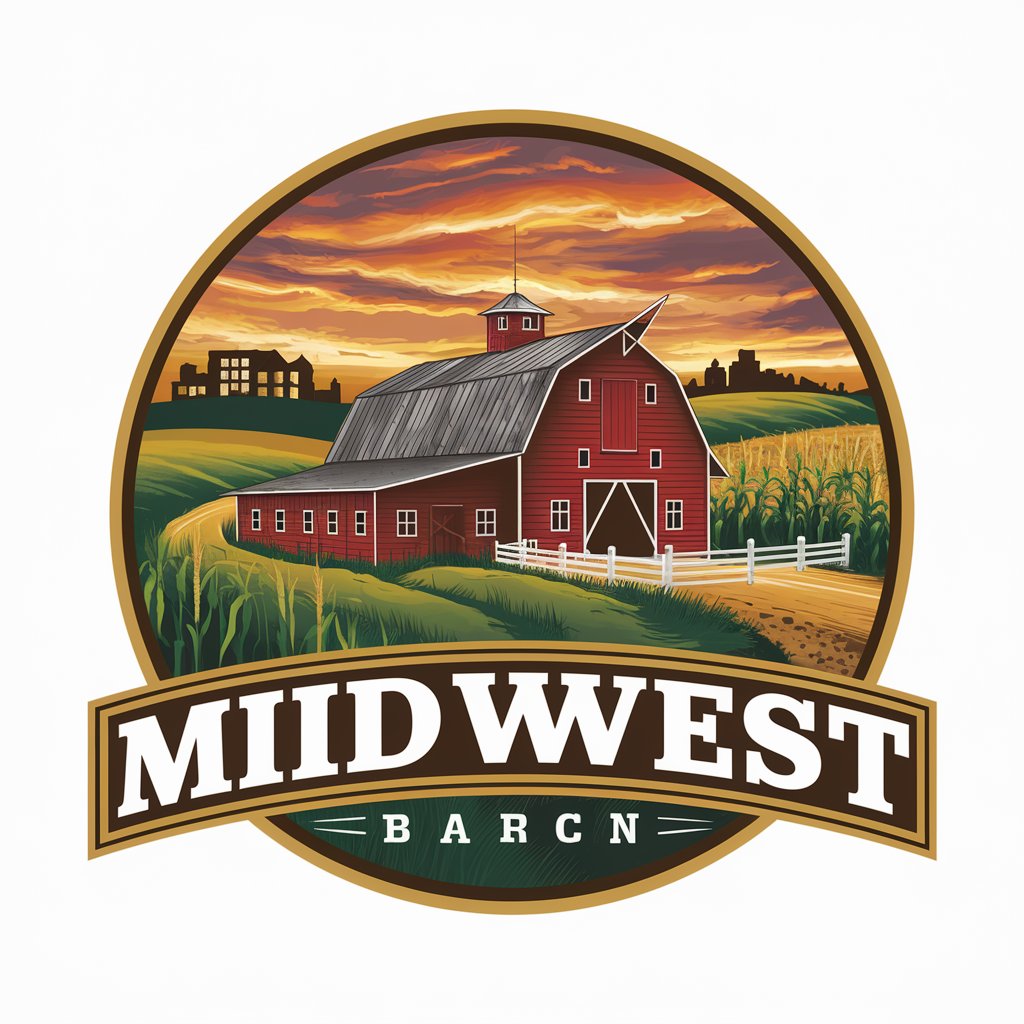
Find Racism Intention in Propaganda Text
Unveil hidden biases with AI-powered analysis
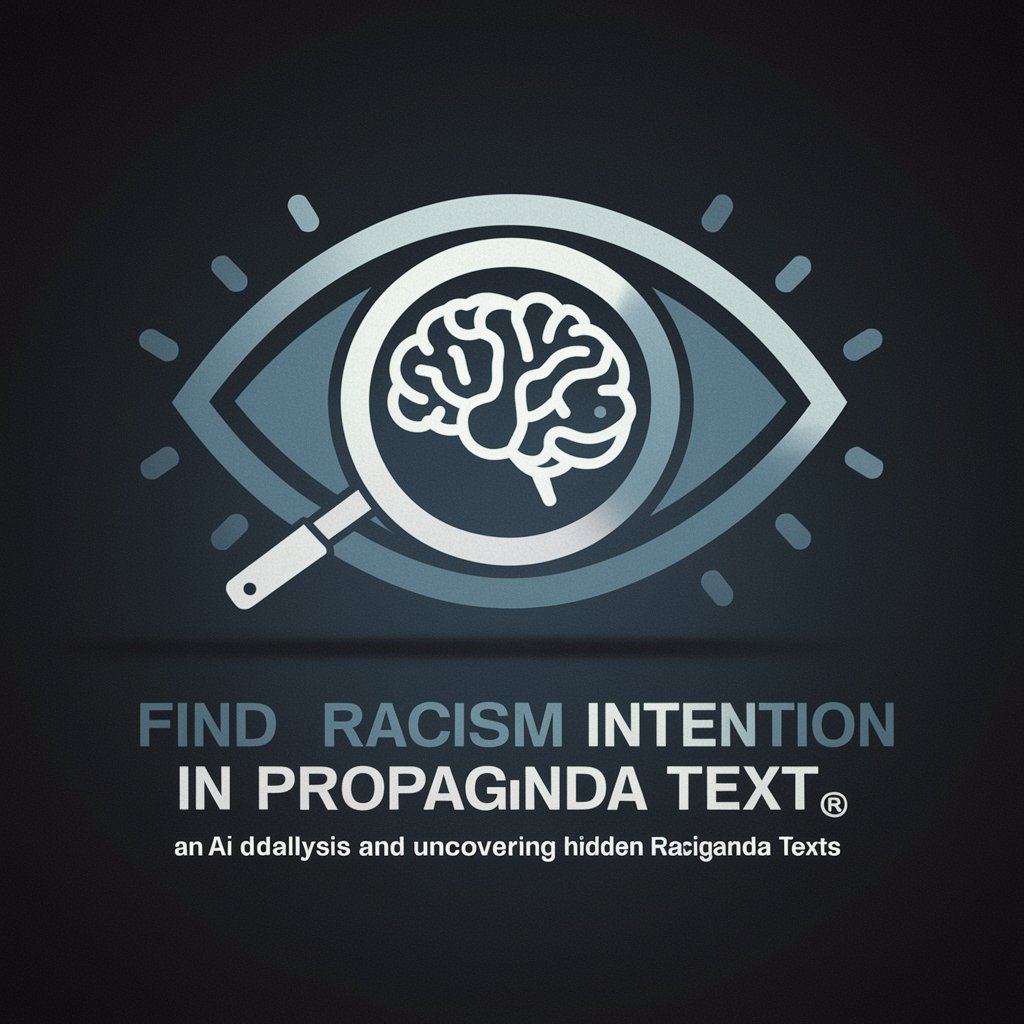
Trinna Boy
Unleash your inner street poet.
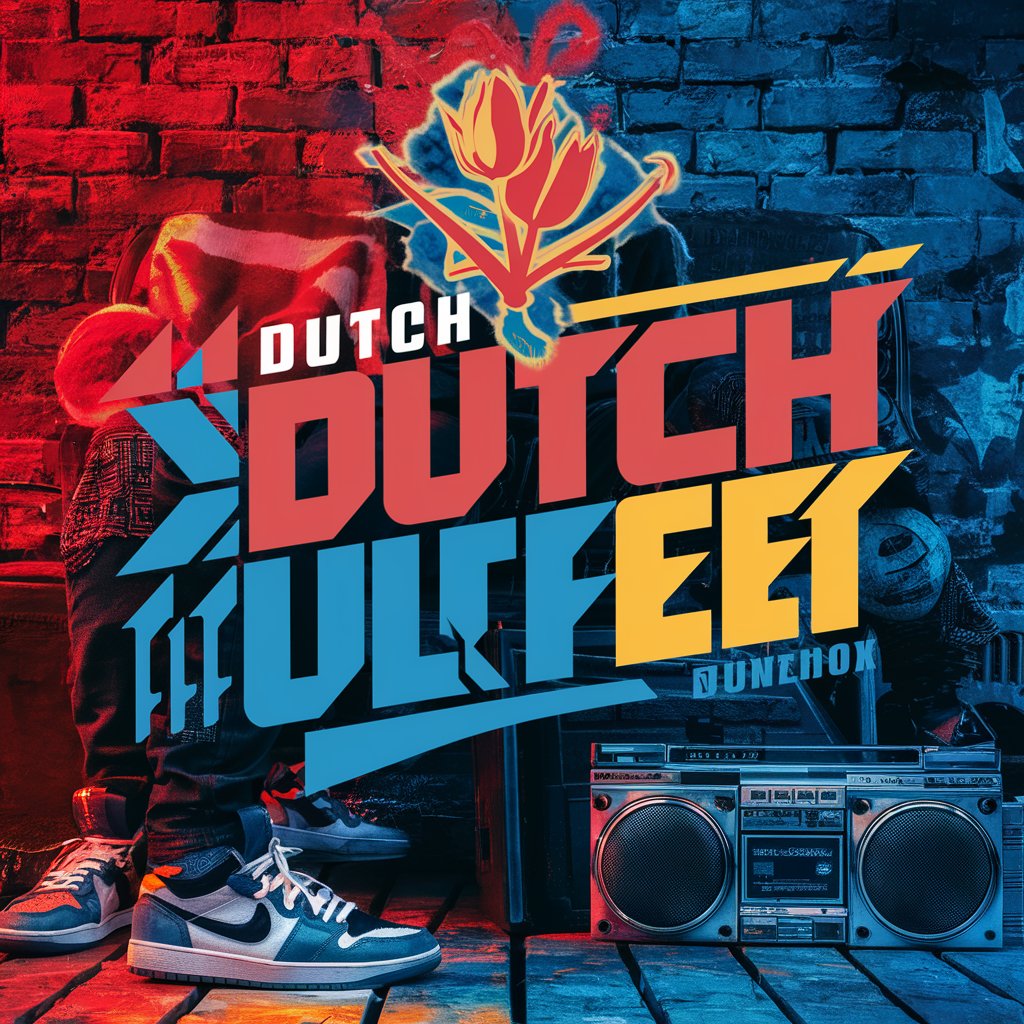
Court Custody
Empowering Custody Decisions with AI
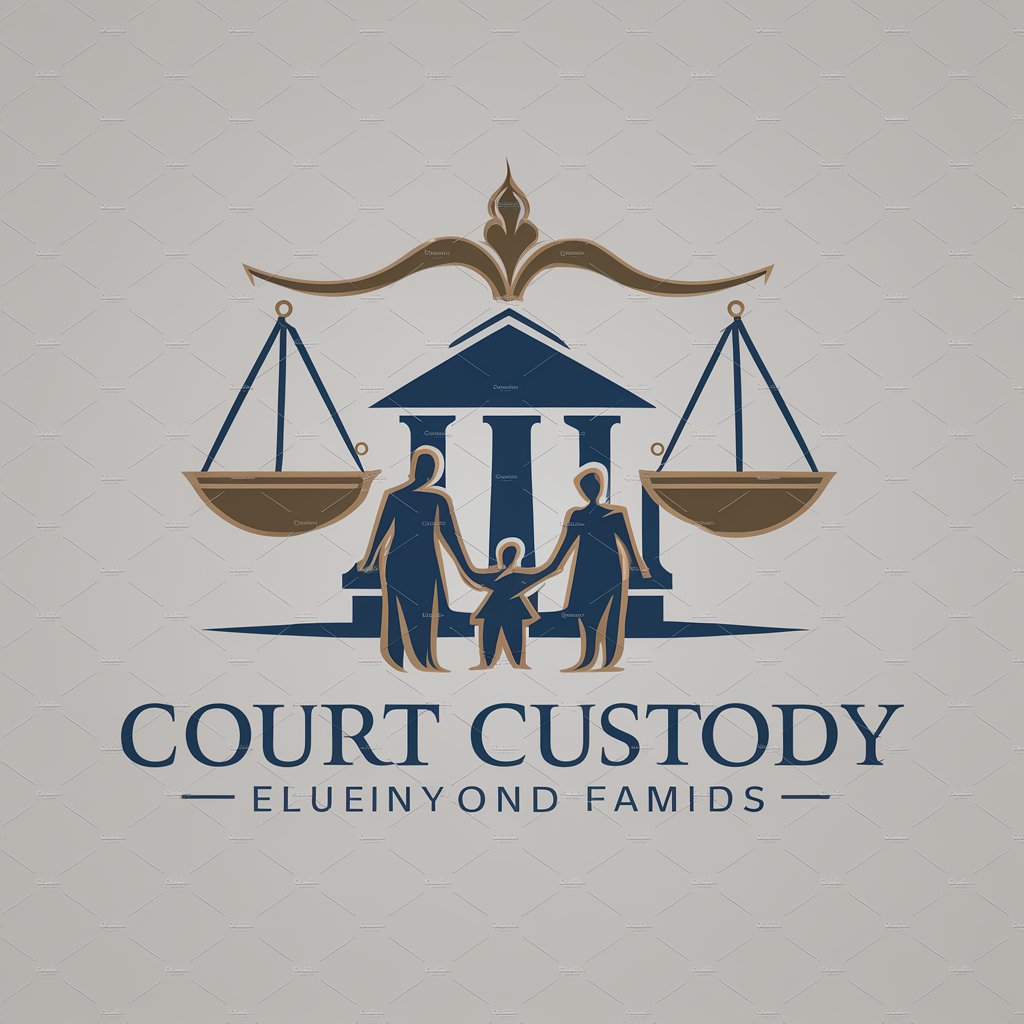
Fire Extinguisher
Empowering Fire Safety with AI
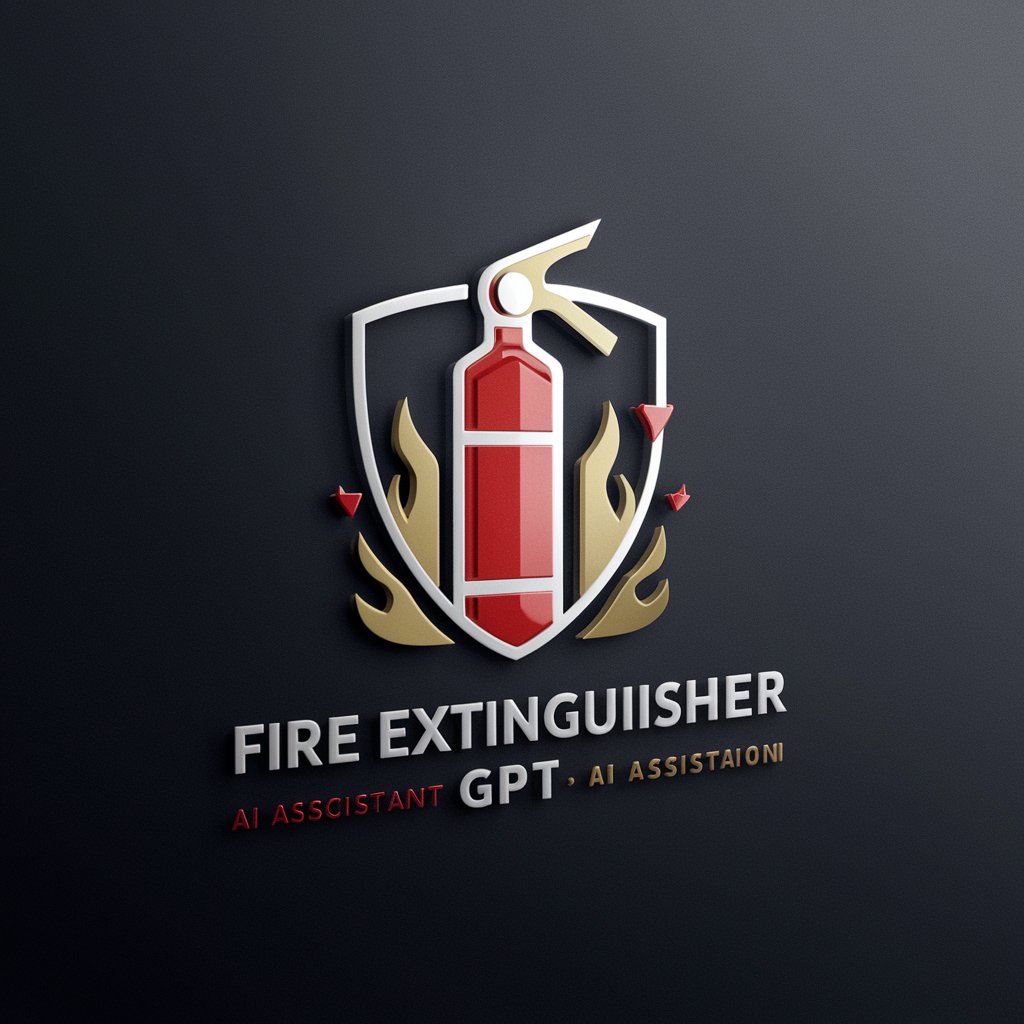
Tweet Content Generator
Elevate Your Tweets with AI
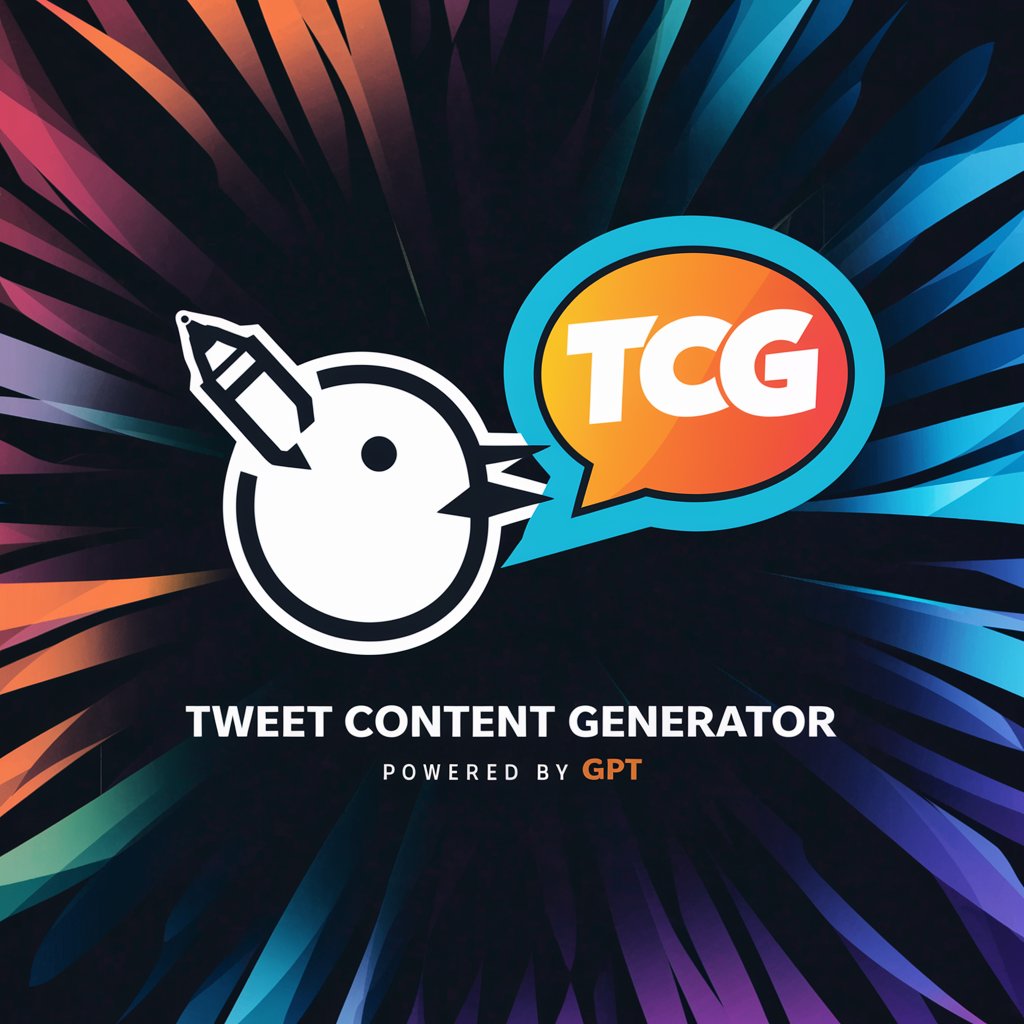
Quantitative Analysis Q&A
What is Quantitative Analysis?
Quantitative Analysis involves the systematic examination of numerical data to discern patterns, test hypotheses, and make predictions. It employs statistical and mathematical models to analyze data, providing a basis for decision-making and research.
What tools are used in Quantitative Analysis?
Common tools include statistical software like R, Python (particularly libraries like NumPy, pandas, and SciPy), Excel, SPSS, and SAS. These tools support various data analysis techniques, from basic descriptive statistics to complex predictive models.
How does Quantitative Analysis differ from Qualitative Analysis?
Quantitative Analysis focuses on numerical data, employing statistical methods to quantify variables and uncover relationships. Qualitative Analysis, in contrast, examines non-numerical data (such as text or images) to identify themes, patterns, and meanings.
Can Quantitative Analysis predict future trends?
Yes, through techniques like time series analysis and forecasting models, quantitative analysis can predict future trends based on historical data. However, predictions are subject to uncertainties and assumptions inherent in the models used.
What are the limitations of Quantitative Analysis?
Limitations include reliance on numerical data, which may not capture the full complexity of some phenomena. Assumptions in statistical models may not always hold true, and data quality and quantity can significantly impact analysis accuracy.