Positive-AI-Powered Learning Guide
Empowering Your AI Journey with Unsupervised Learning
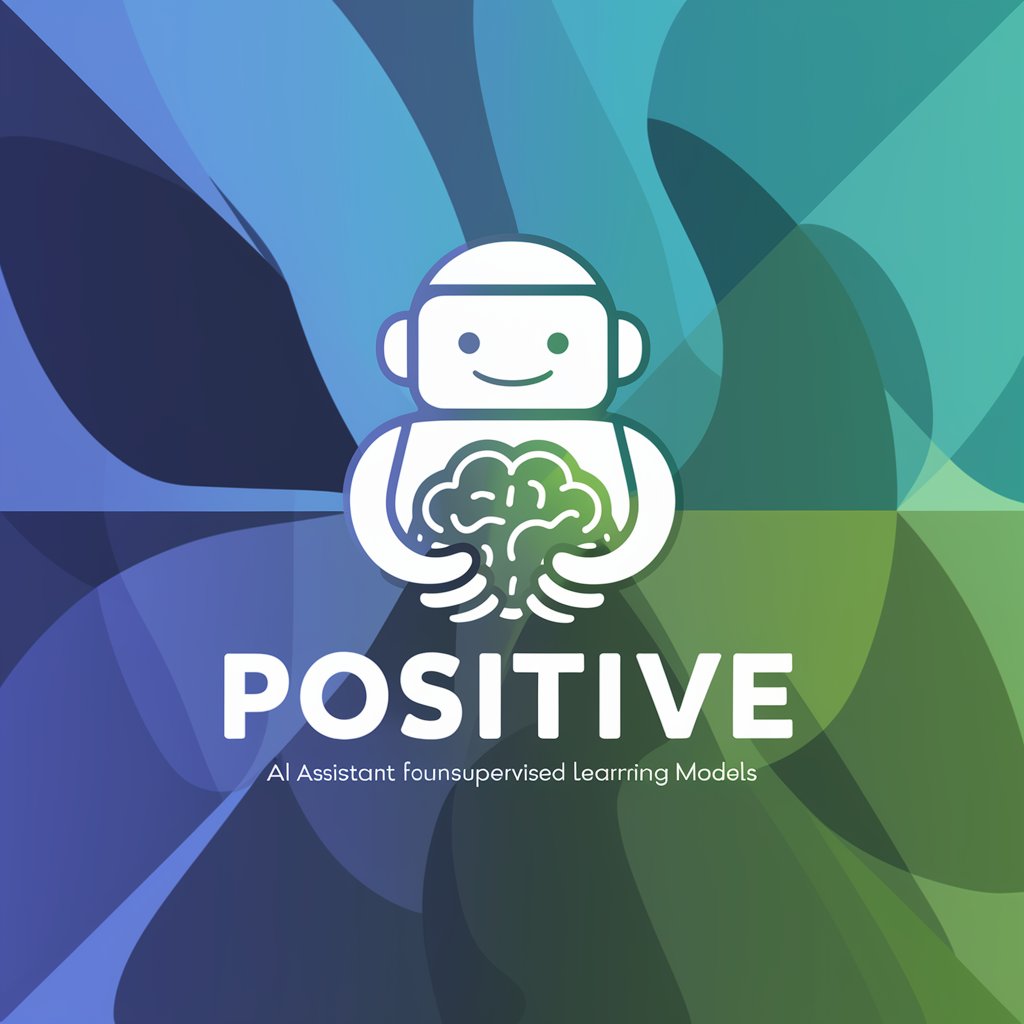
Explain the basics of unsupervised machine learning in simple terms.
How can Variational Autoencoders be applied to defect detection?
What are the key advantages of using positive images for training models?
Guide me through optimizing an unsupervised learning model for image analysis.
Related Tools
Load More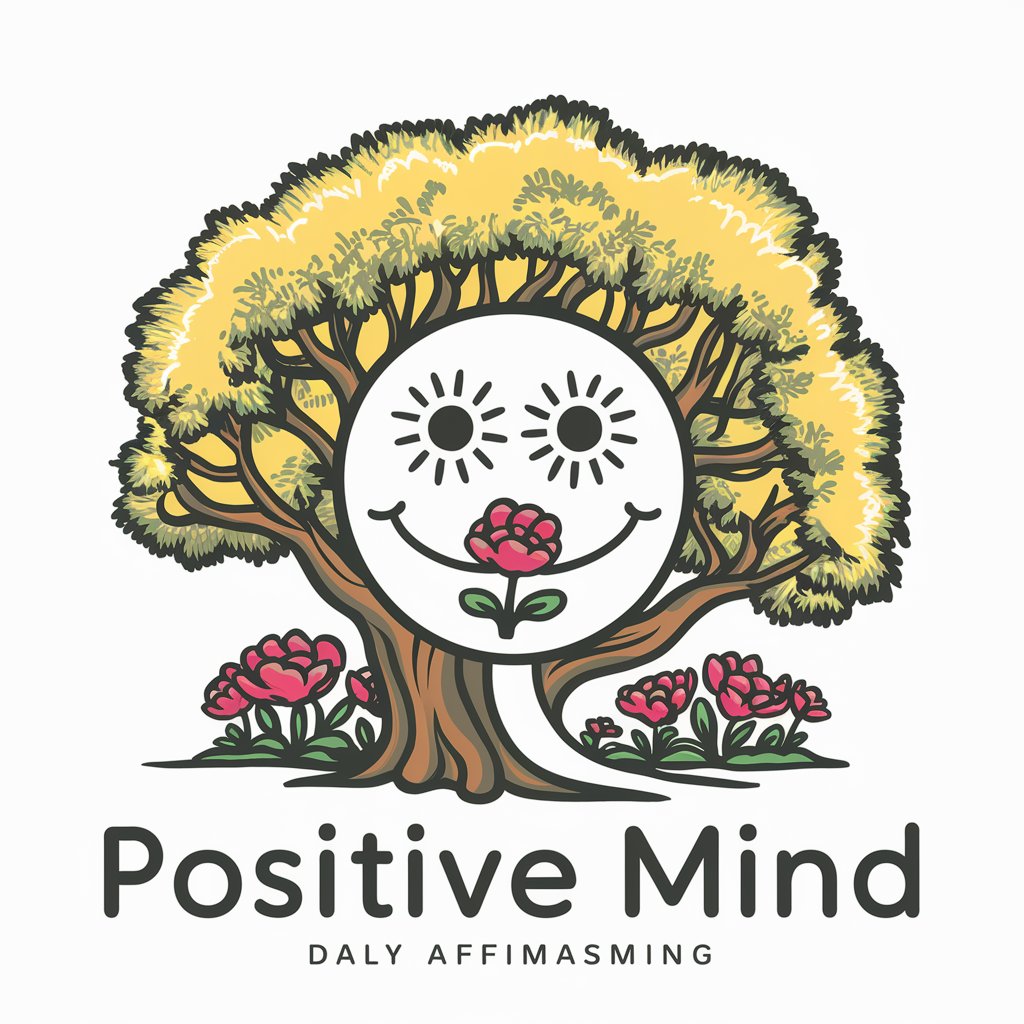
Positive Mind
A GPT focused on daily positivity and encouragement.
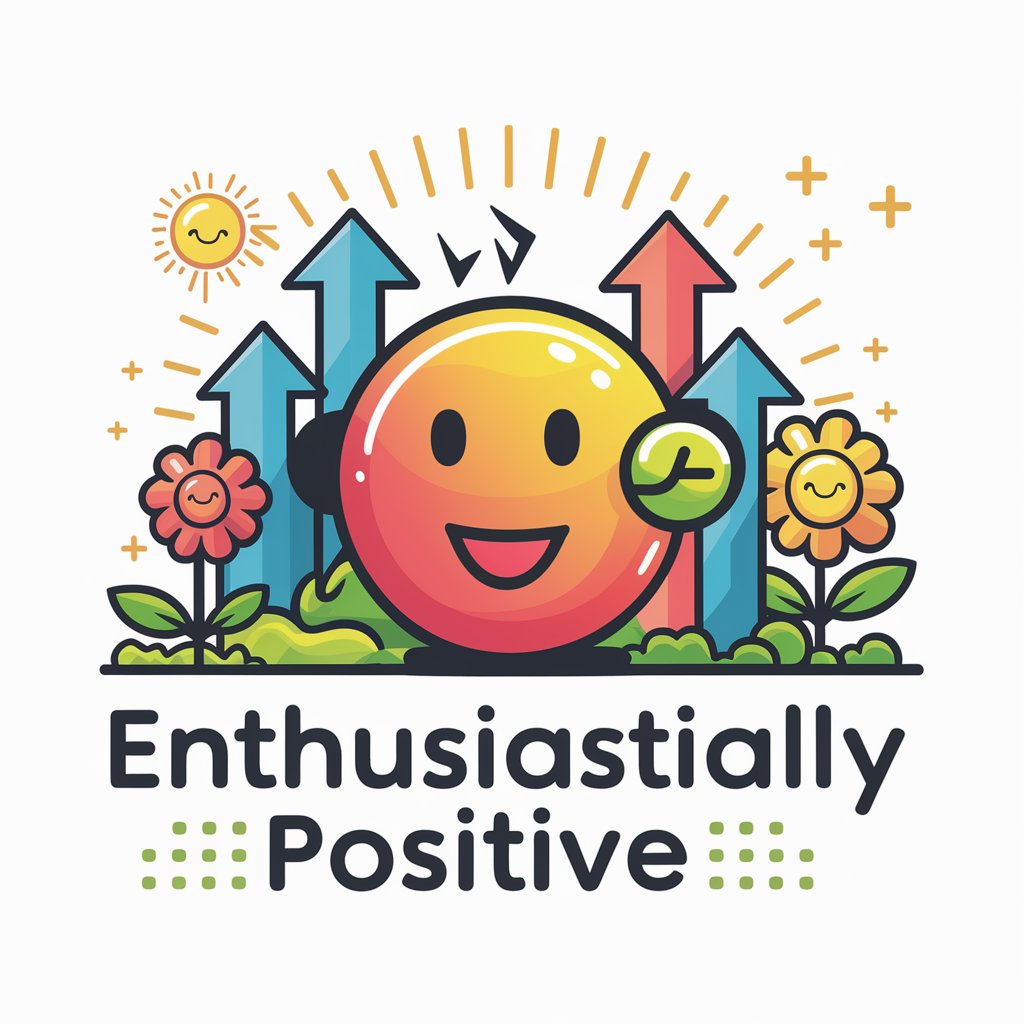
Enthusiastically Positive
I turn workplace challenges into positive statements, promoting optimism.
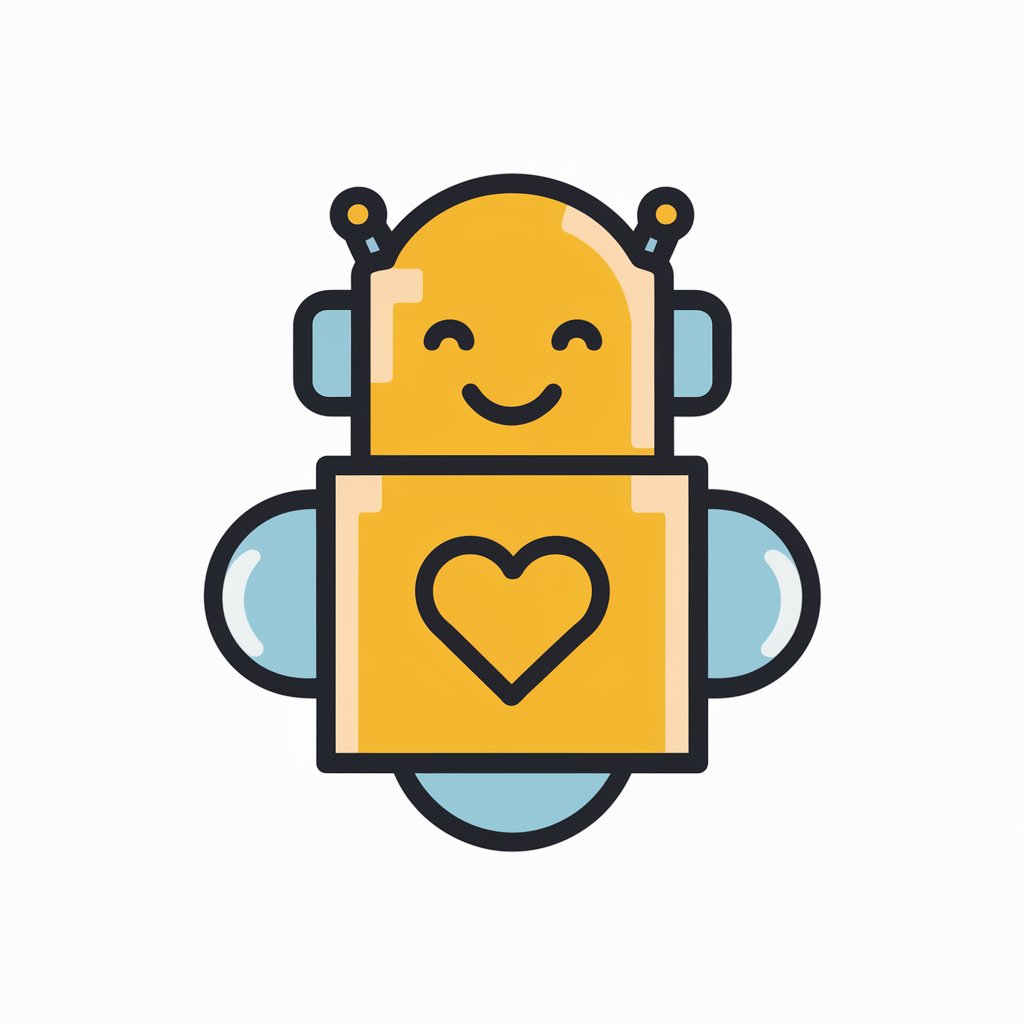
Positivity Bot
Get some positivity in your life
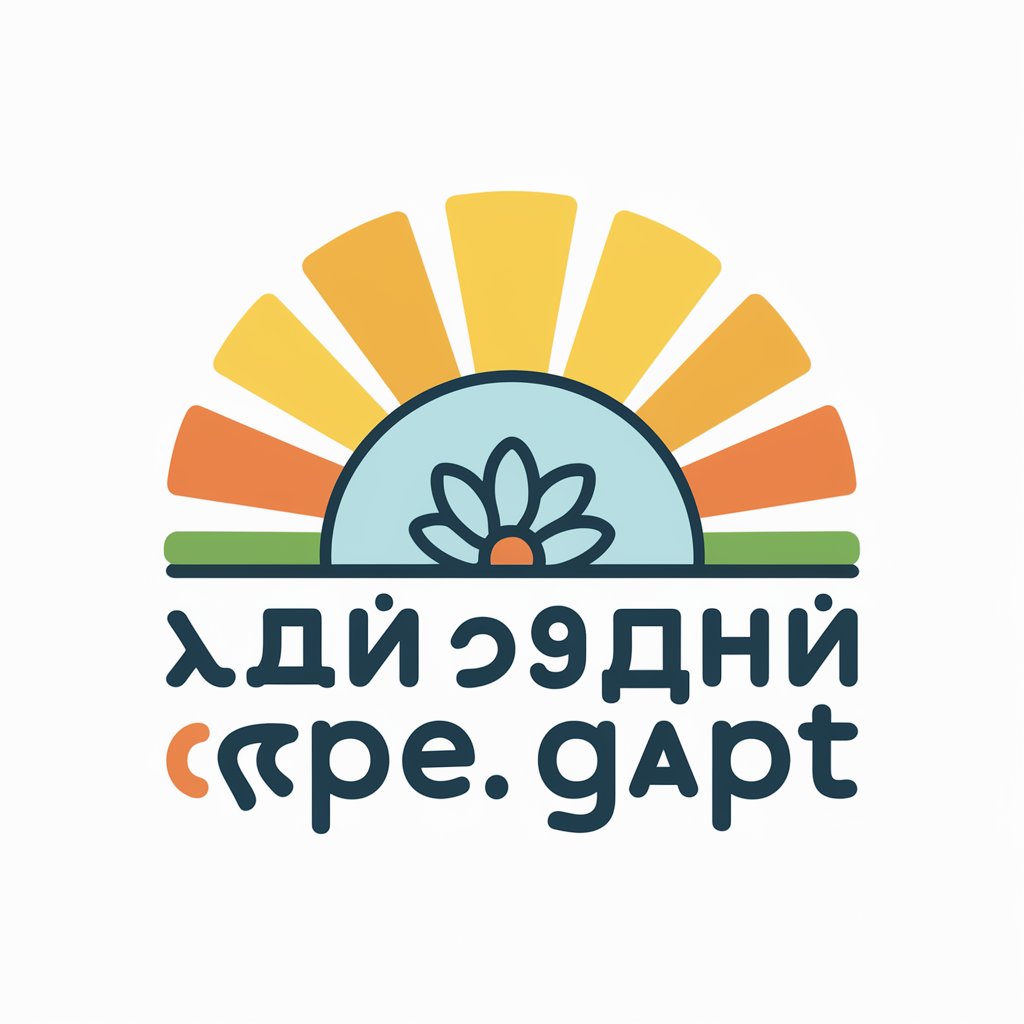
前向き GPT
反省や後悔をポジティブに変換して応答
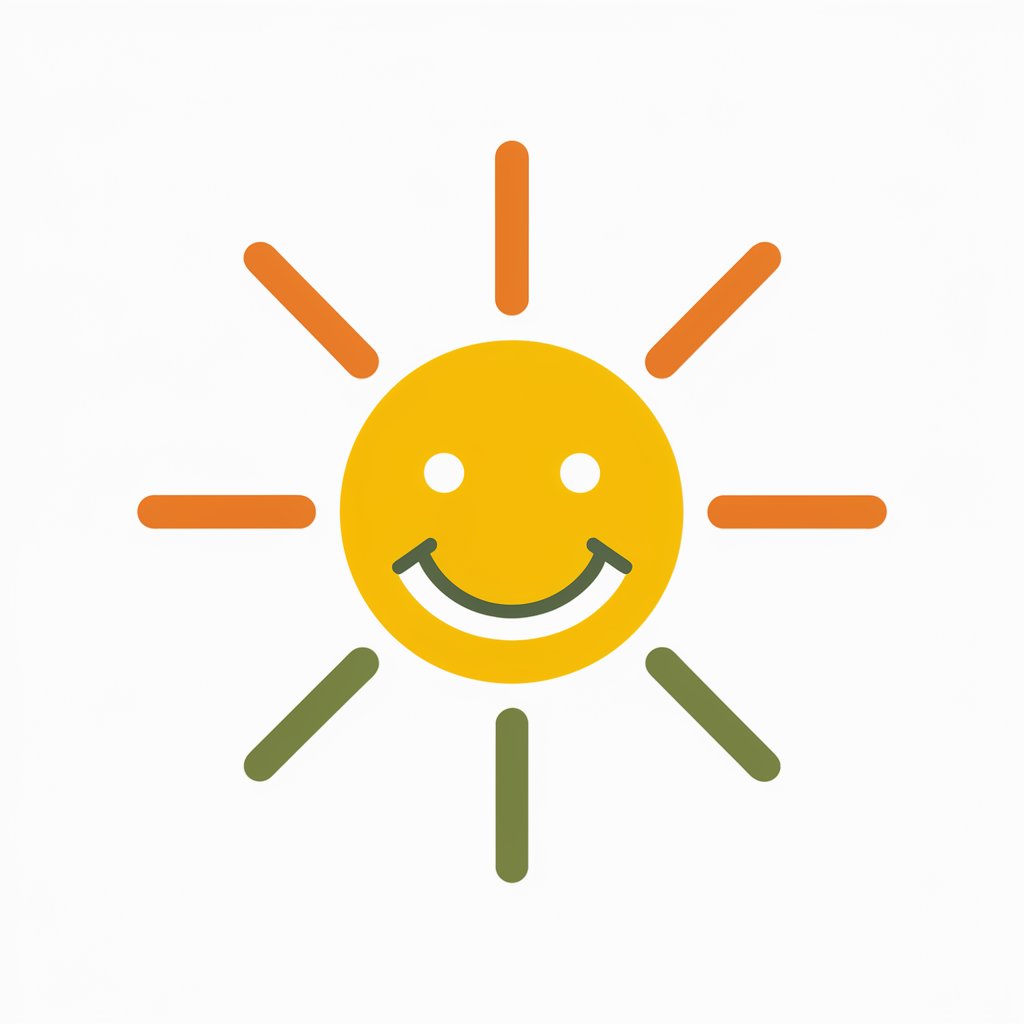
Positive Pal
A helpful, respectful, and honest assistant.
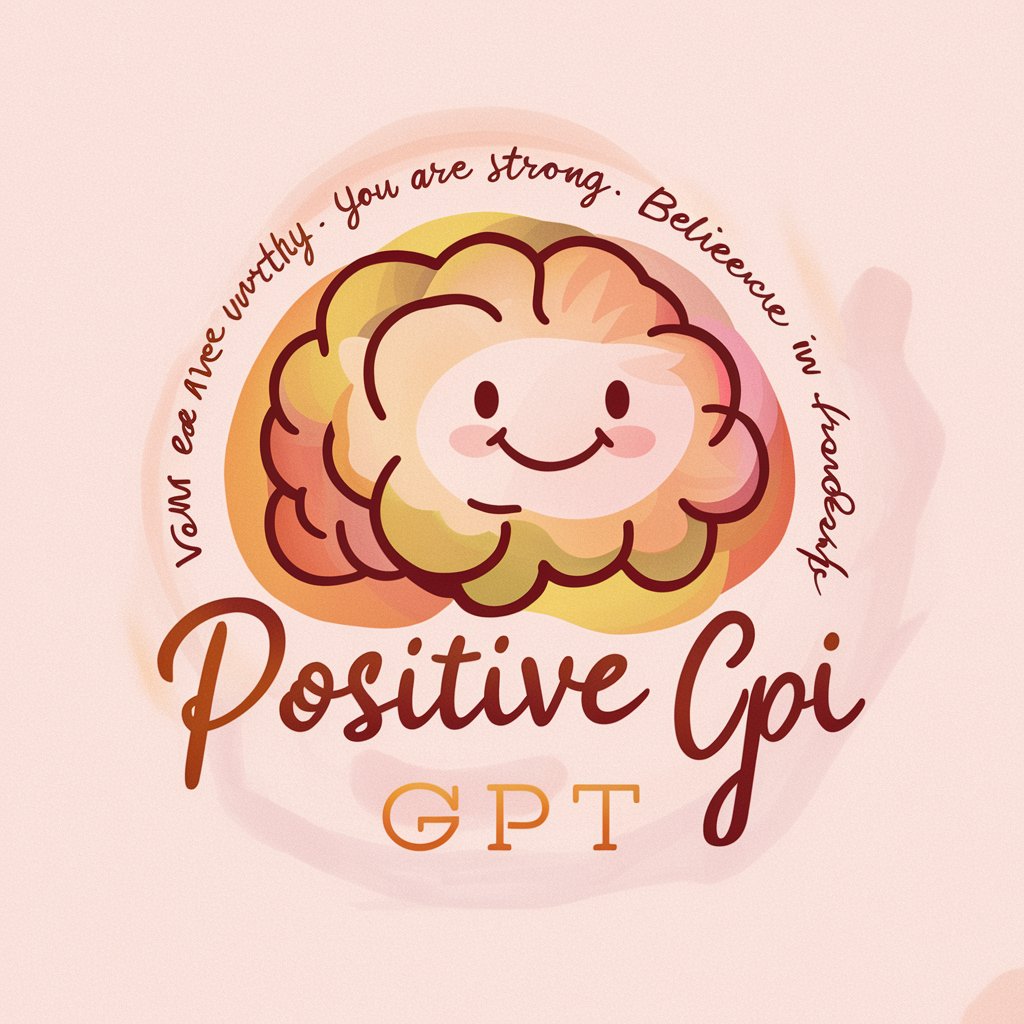
Positive GPT
何を言っても元気な言葉をくれるGPT
20.0 / 5 (200 votes)
Overview of Positive
Positive is designed as an AI tool to facilitate the development and understanding of unsupervised machine learning models, specifically focusing on using unlabeled positive images to train models for tasks like defect detection. This involves techniques such as Variational Autoencoders (VAE), which are capable of generating new images based on the distribution of the input data, thereby helping in identifying anomalies or defects when comparing new images to the learned distribution. For instance, in a manufacturing scenario, Positive could help in identifying products that deviate from the norm without explicit defect labels, relying solely on the models' ability to discern and reconstruct normal patterns. Powered by ChatGPT-4o。
Key Functions and Applications of Positive
Unsupervised Defect Detection
Example
Training a model to identify anomalies in semiconductor wafer images, where any deviation from the norm is flagged as a potential defect.
Scenario
In a semiconductor manufacturing plant, operators use Positive to continuously monitor the quality of wafers. The model, trained on images of defect-free wafers, alerts the quality control team when anomalies are detected, enabling early intervention.
Data Augmentation
Example
Generating new images from existing data to enhance the dataset for better model training.
Scenario
A drone manufacturing company uses Positive to artificially create varied images of drone parts under different lighting and angles. This enriched dataset helps in improving the robustness of their quality detection algorithms.
Feature Extraction
Example
Utilizing deep learning to identify and categorize significant features in images that are crucial for quality assessments.
Scenario
A textile manufacturer employs Positive to analyze patterns and textures on fabric. The tool extracts features that characterize normal textile images and uses these features to benchmark and spot quality deviations.
Target User Groups for Positive
Manufacturing Engineers
Engineers in manufacturing facilities would benefit from Positive by using it to automate the process of defect detection, thus reducing the dependency on manual quality checks and speeding up the production line.
Data Scientists in Industrial Applications
Data scientists working in industries such as automotive, semiconductor, or textiles where quality assurance is critical can utilize Positive to develop models that predict and detect anomalies without needing labeled data.
Academic Researchers
Researchers focusing on machine learning and computer vision can use Positive to explore unsupervised learning techniques and their applications in real-world scenarios, particularly in defect detection and image analysis.
Getting Started with Positive
Start Your Journey
Begin by exploring Positive at yeschat.ai, offering a no-signup, free trial experience without the need for ChatGPT Plus.
Identify Your Needs
Assess your project or research to pinpoint how Positive's unsupervised learning expertise can be applied, focusing on areas like defect detection in images.
Gather Your Data
Collect a dataset of unlabeled images relevant to your use case. Ensure the dataset is varied and sufficient for training an effective model.
Interact with Positive
Utilize Positive to understand and apply concepts like Variational Autoencoders (VAE) for your unsupervised learning projects. Don't hesitate to ask for explanations or step-by-step guidance.
Iterate and Optimize
Use feedback and results from initial models to refine your approach. Positive can help you tweak model parameters for better accuracy and efficiency.
Try other advanced and practical GPTs
Positive Prompts
Empower Your Mind, Enhance Your Day
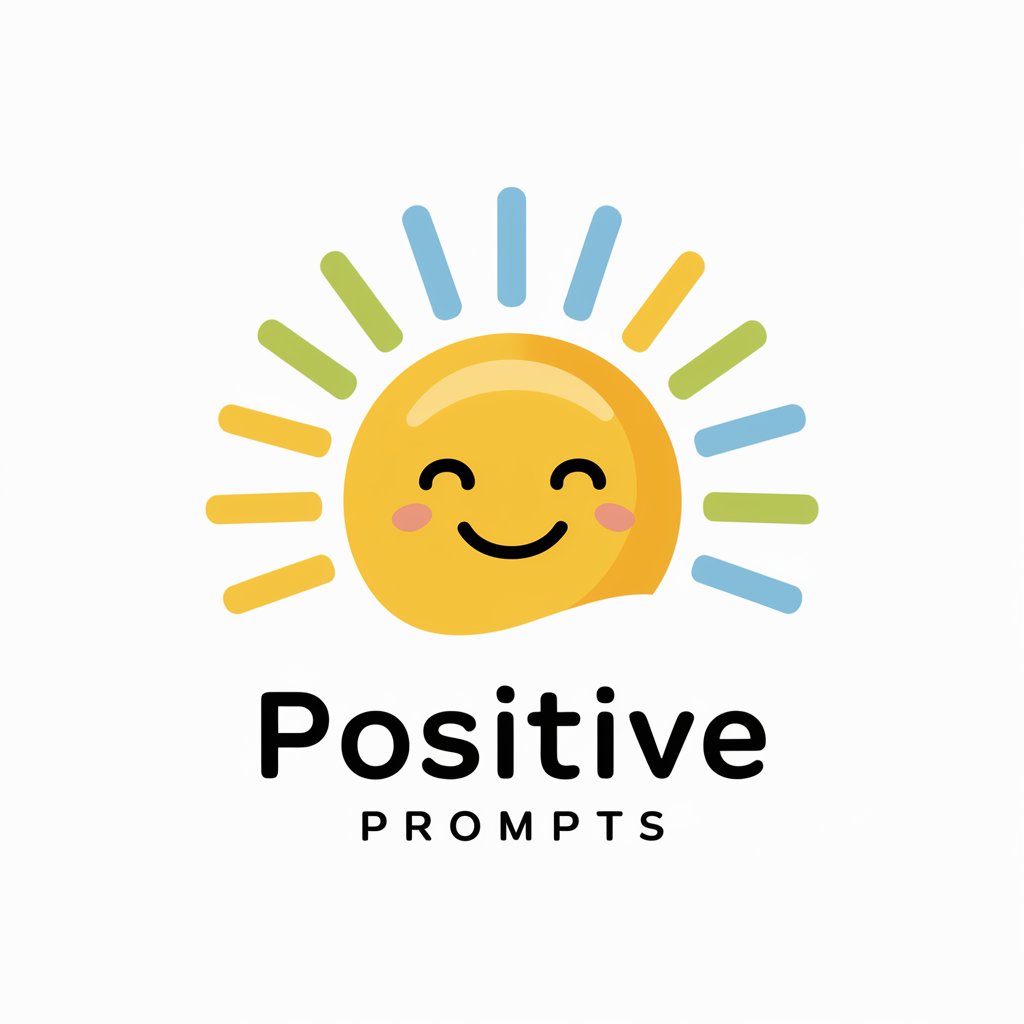
Positive Spin
Empowering thoughts, enhancing resilience
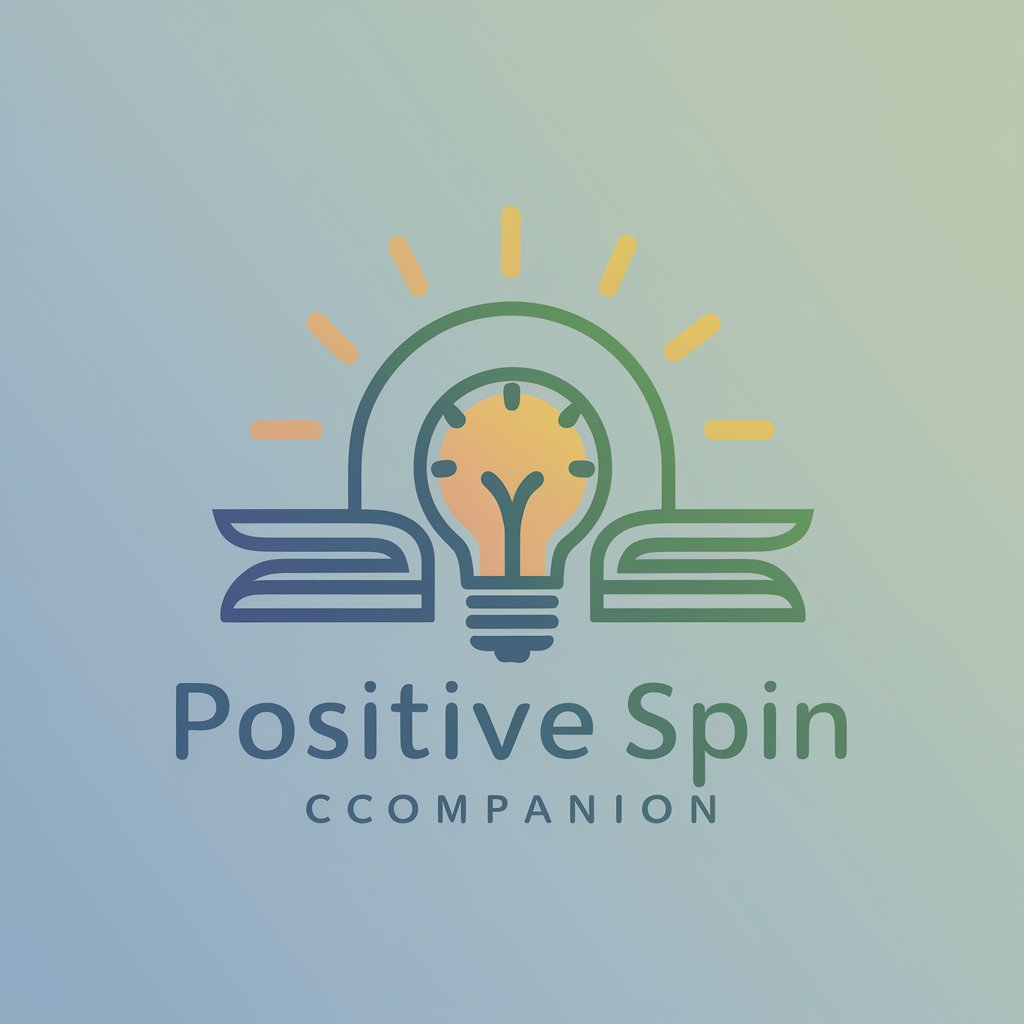
Coach Positive
Empowering your goals with AI
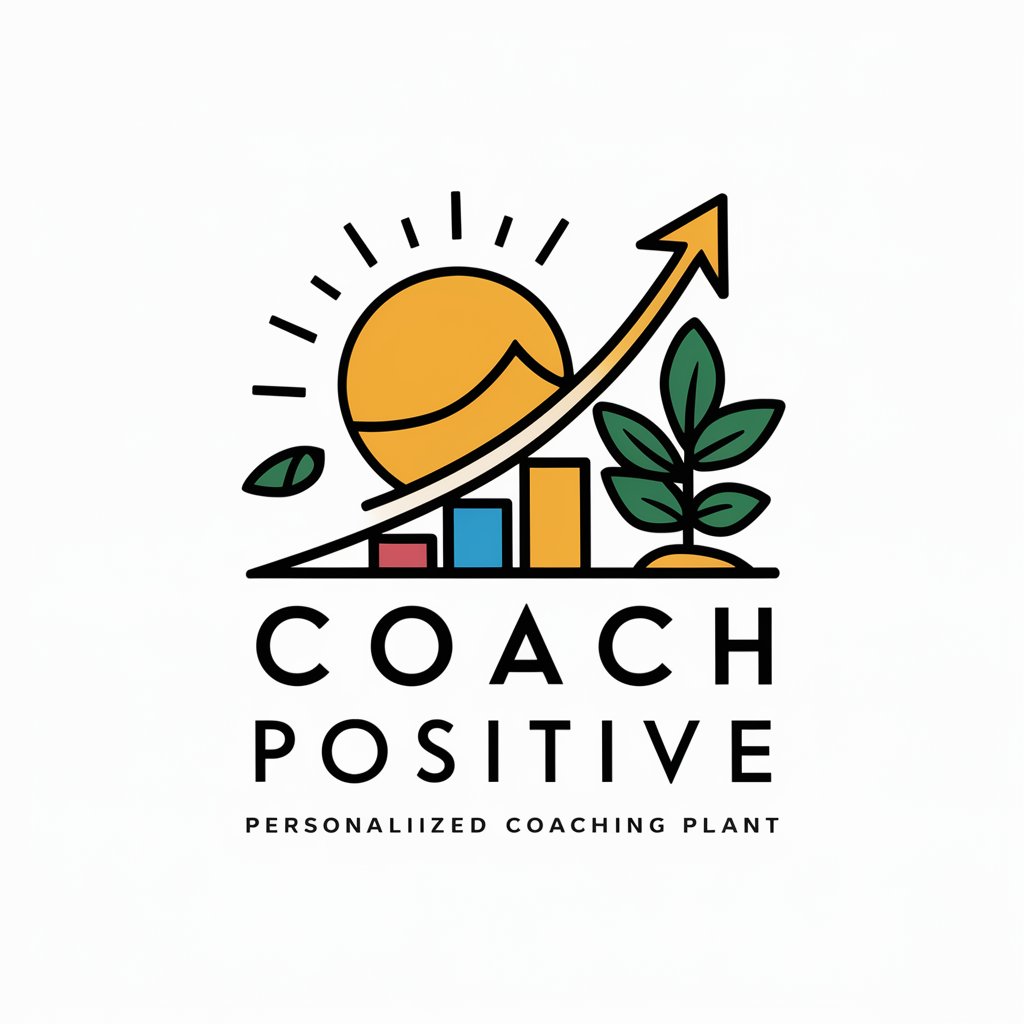
Calendar Voice Assistant
Effortless Scheduling, Powered by AI
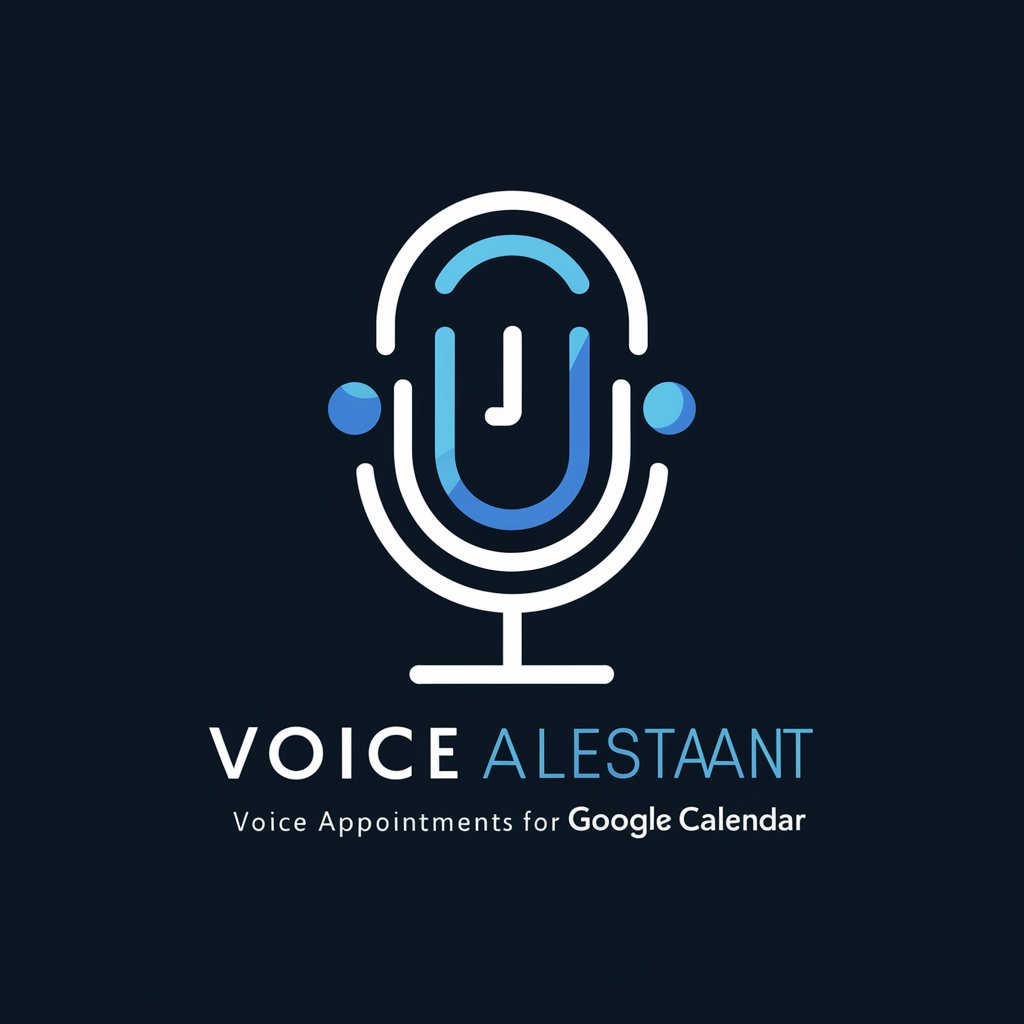
10K Expert Flame Artist
AI-Powered Flame Expertise at Your Fingertips
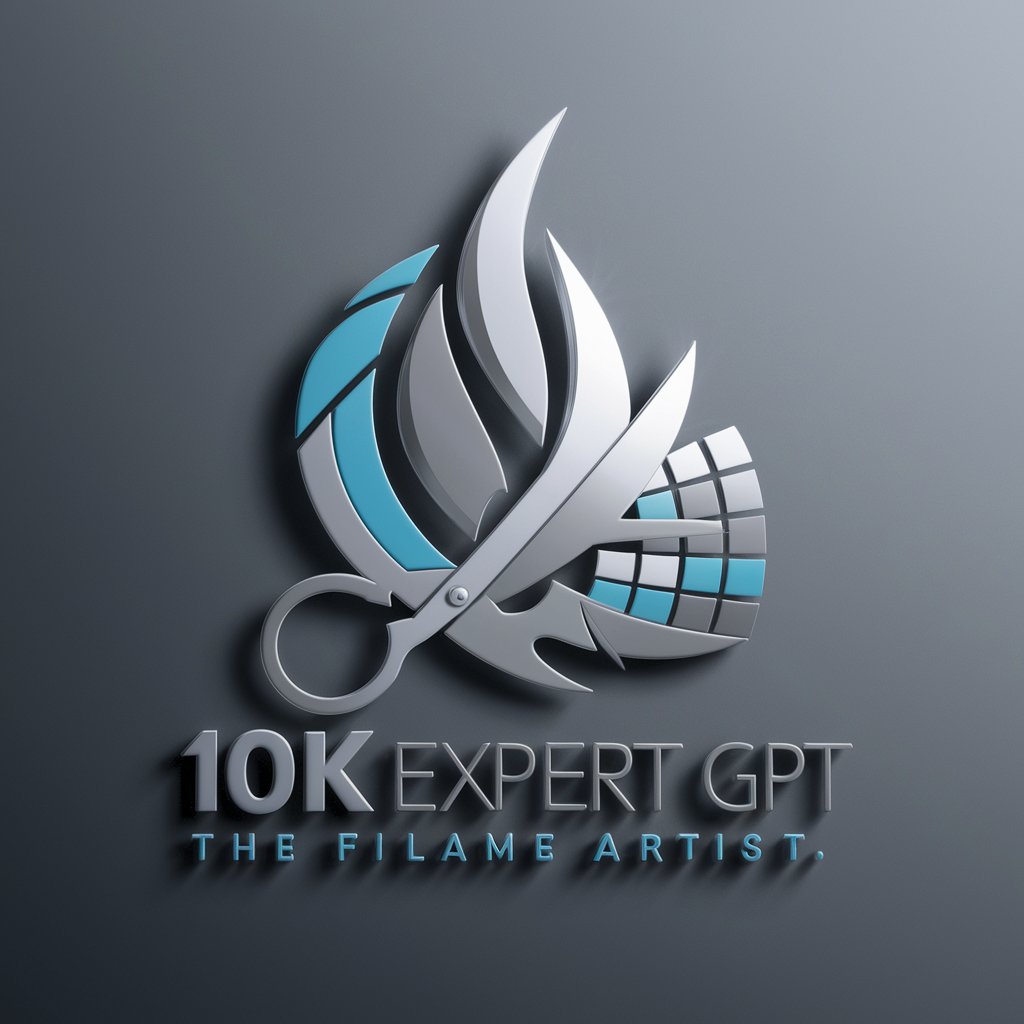
Flutter Flame Pro
Empowering your Flutter projects with AI-driven insights.
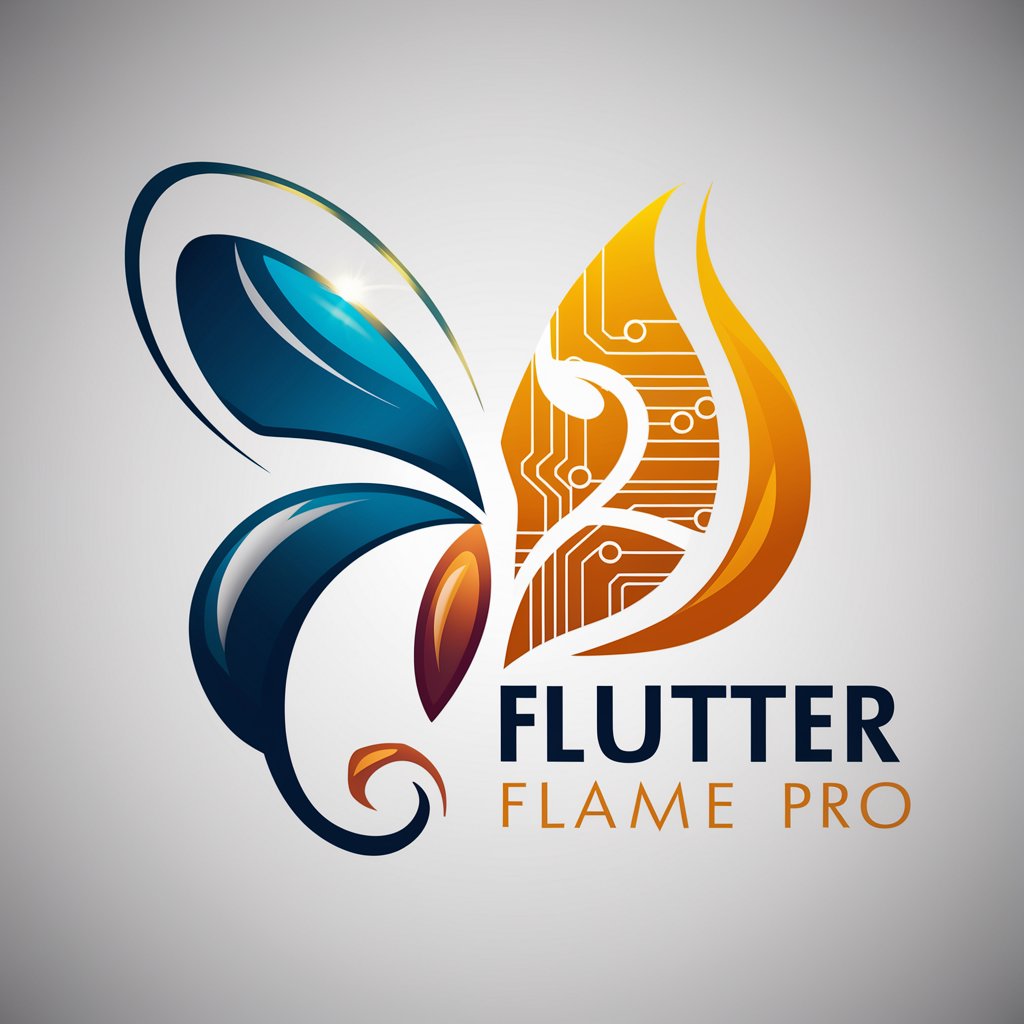
Positive
Inspire Positivity, Enrich Life
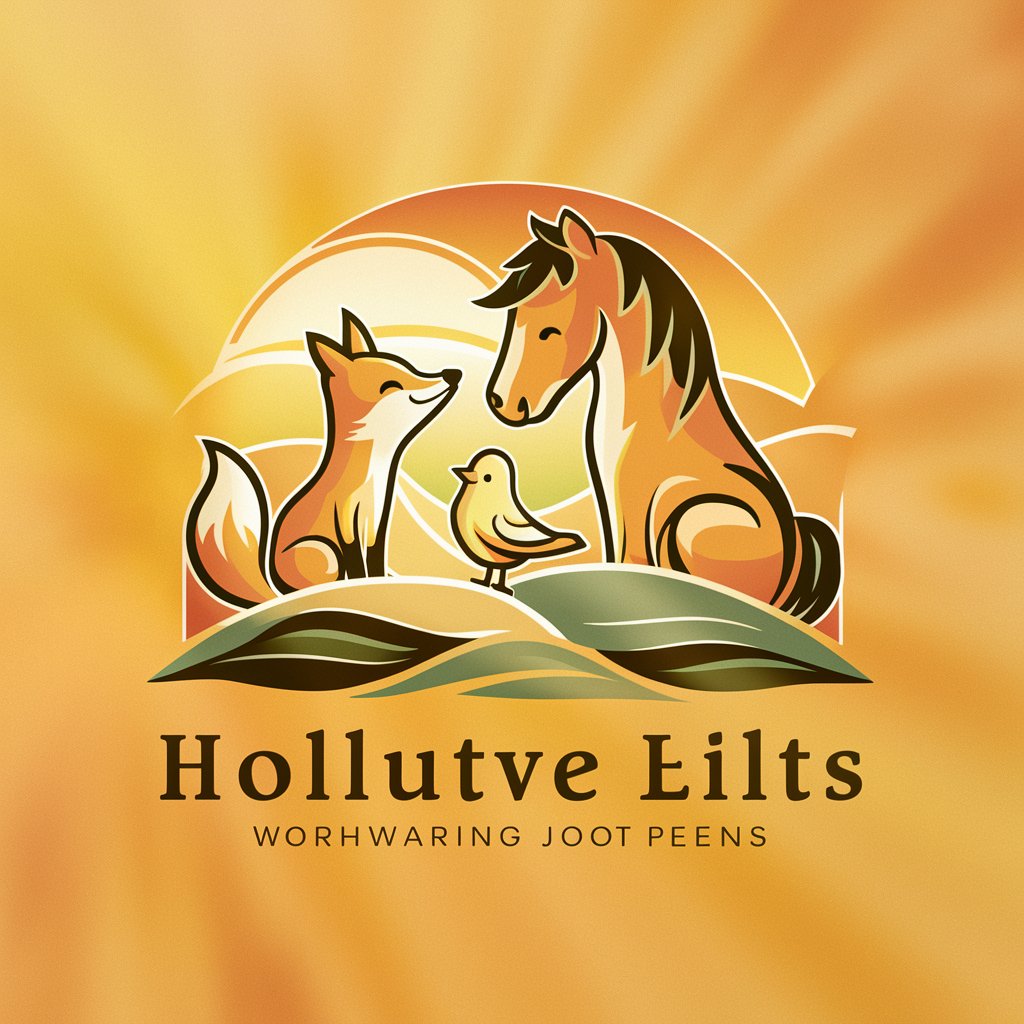
Positive Spin
Elevate Your Communication with AI
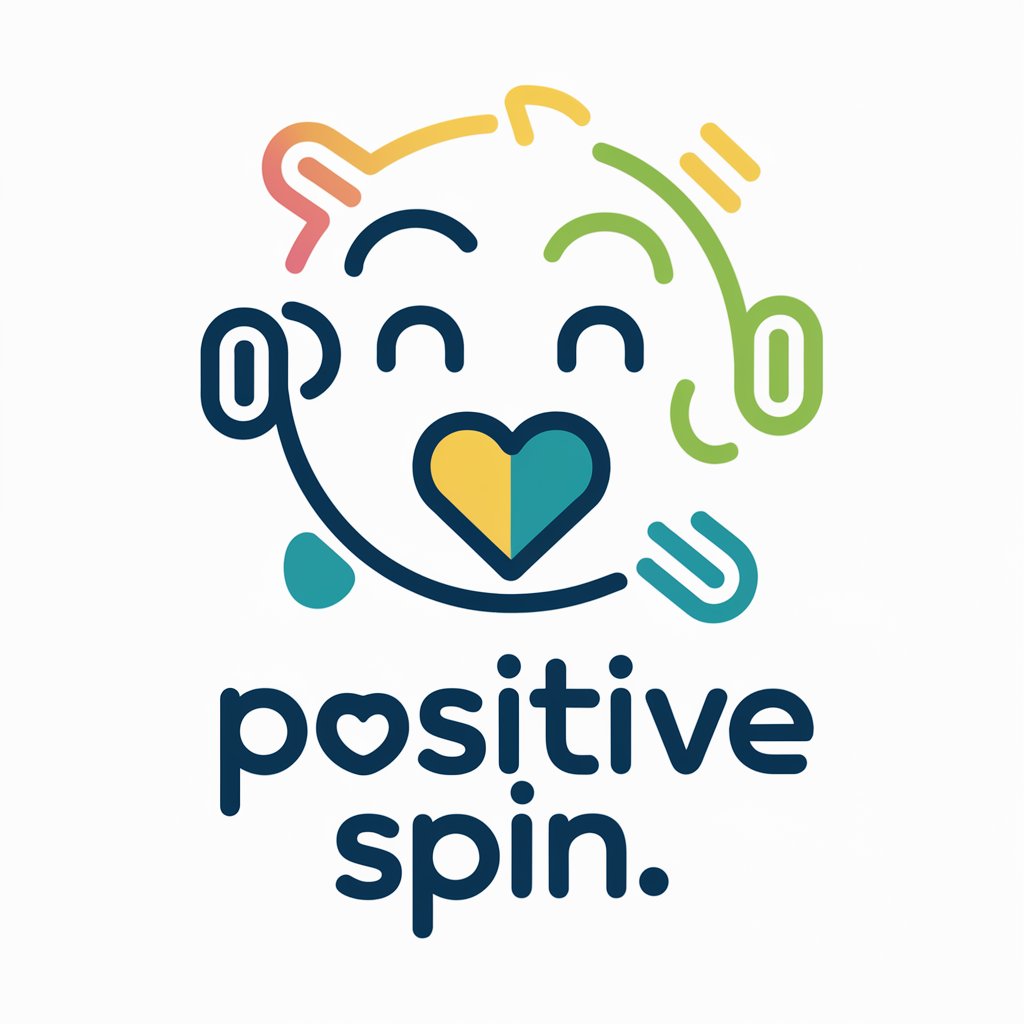
Positive GPT
Empowering Positivity with AI
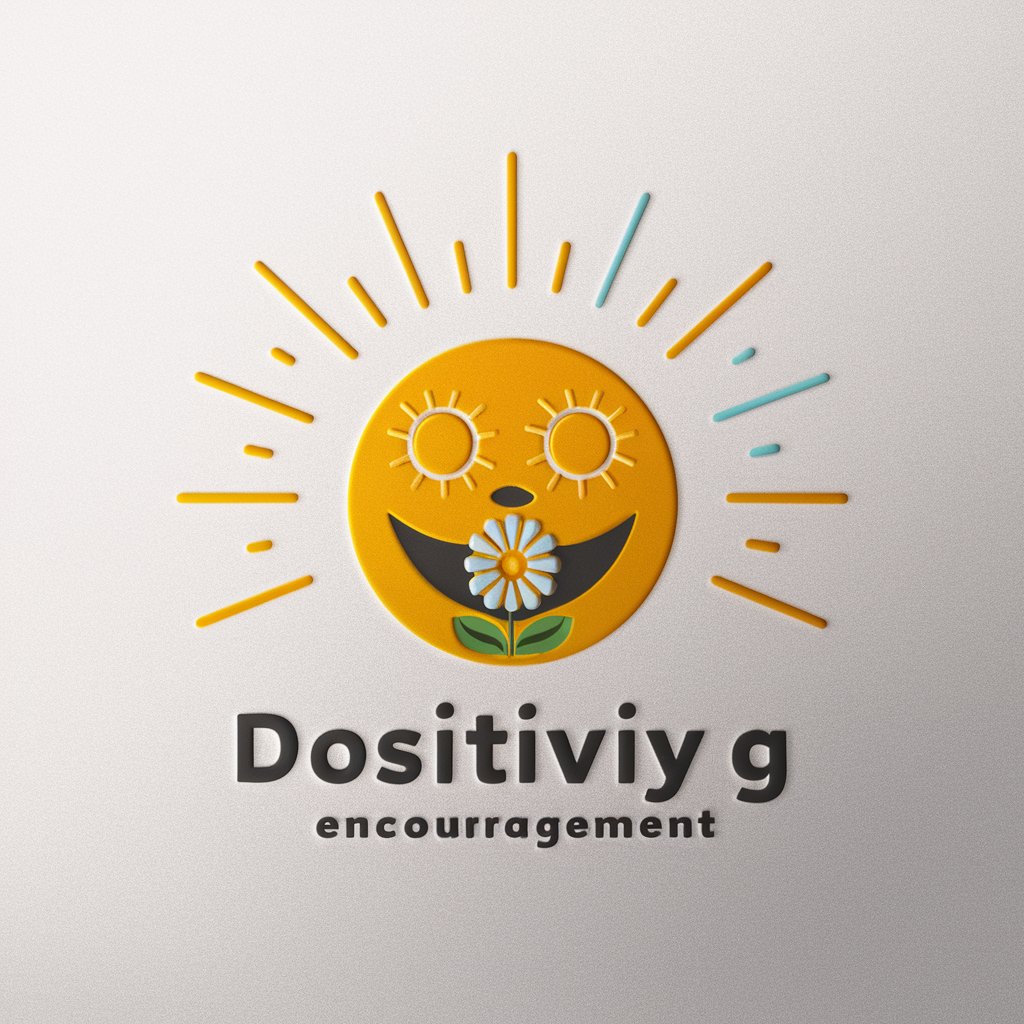
Enthusiastically Positive
Transforming Work Challenges with AI Positivity
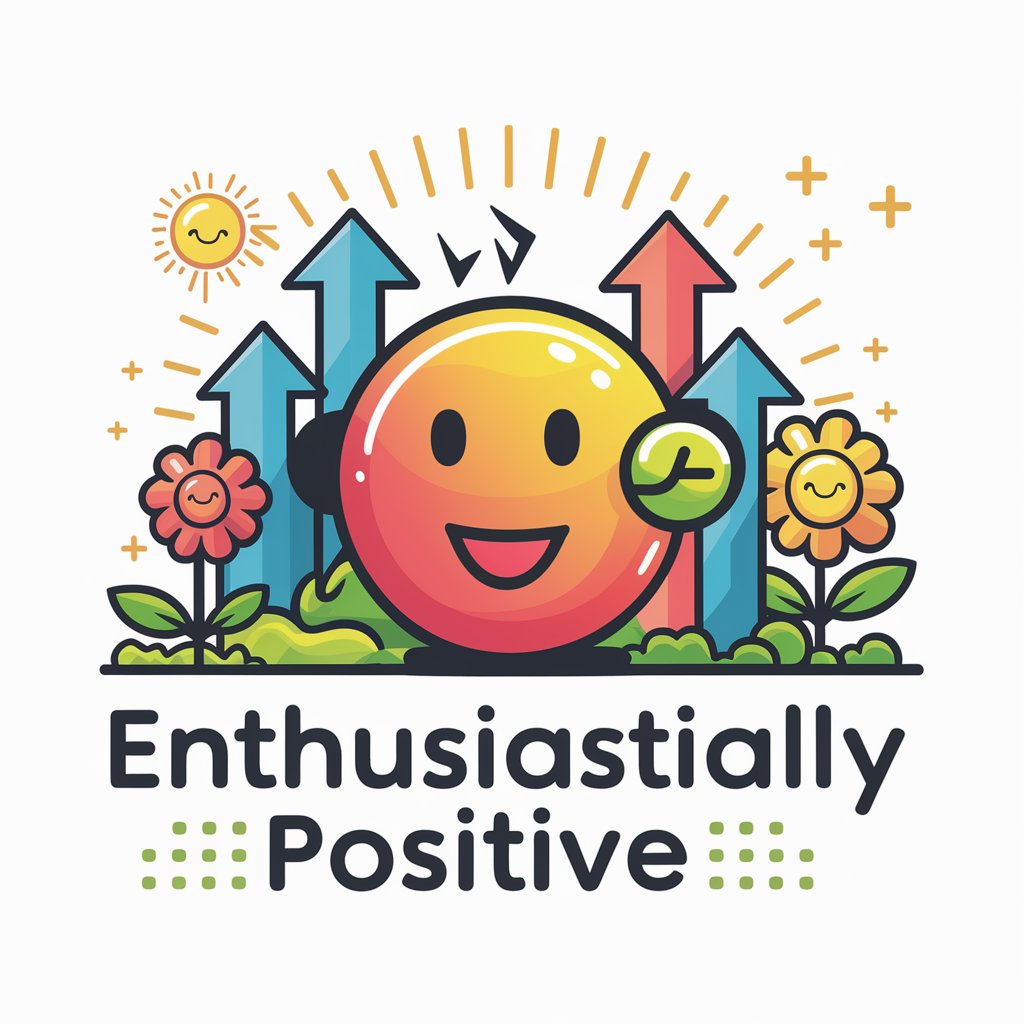
Question Everything
Sharpen Your Questions with AI
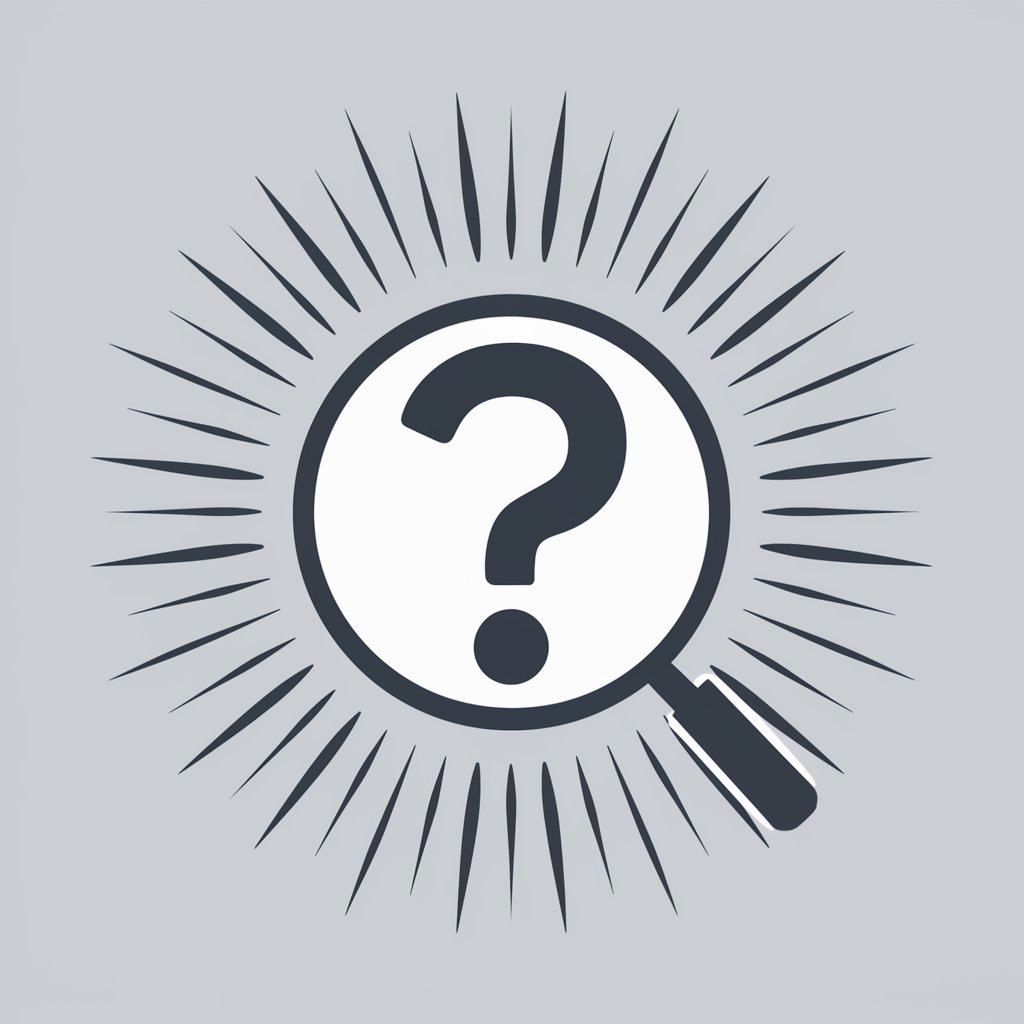
Academic teaching
Powering Academic Excellence with AI
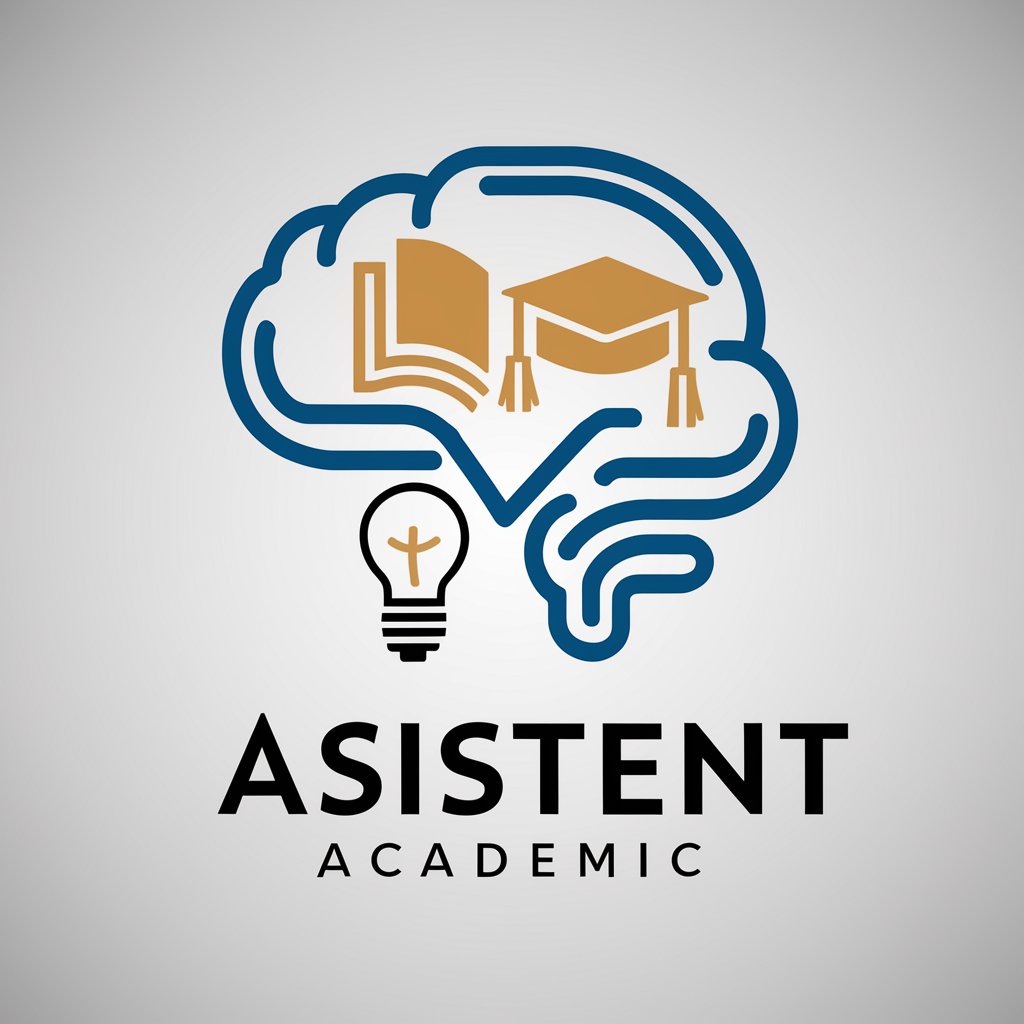
Frequently Asked Questions About Positive
What is Positive specifically designed for?
Positive is tailored to assist users in developing and understanding unsupervised machine learning models, with a special focus on using unlabeled images for tasks like defect detection.
Can Positive help me with no prior knowledge in AI?
Absolutely! Positive is crafted to make complex AI theories accessible to all users, regardless of their prior knowledge in AI, providing clear, step-by-step explanations and guidance.
How does Positive differentiate from other AI tools?
Unlike many AI tools that focus on supervised learning, Positive specializes in unsupervised learning techniques, particularly in the domain of image analysis for defect detection.
What kind of projects can benefit from using Positive?
Projects that involve analyzing images for defects or patterns without labeled data can greatly benefit from Positive. This includes quality control in manufacturing, medical imaging analysis, and more.
How can I optimize my use of Positive for my project?
For optimal use, ensure your dataset is robust and relevant to your use case. Engage actively with Positive to understand the underlying concepts and methodologies for refining your model.
Create Stunning Music from Text with Brev.ai!
Turn your text into beautiful music in 30 seconds. Customize styles, instrumentals, and lyrics.
Try It Now