ml - Bayesian Inference Analysis

Hey! Ready for a quick dive into Bayesian Inference?
Unravel Data with AI-driven Insights
Explain non-conjugate models briefly.
Classifiers in Bayesian Inference?
Analyze this Bayesian inference image.
Real-world application of Bayesian Inference?
Get Embed Code
Introduction to the Bayes Explainer
The Bayes Explainer is an educational assistant designed to provide specialized support and detailed insights into Bayesian inference, particularly focusing on non-conjugate models and classifiers. It serves as a resource for understanding and applying Bayesian statistics, tailored to assist users in comprehending complex statistical models through a combination of concise summaries and in-depth explanations. By interpreting images related to Bayesian problems, it offers visual explanations that enhance learning. This GPT adjusts its communication to match the user's level of expertise, from beginners to advanced learners, facilitating a better grasp of Bayesian approaches in various contexts. Powered by ChatGPT-4o。
Key Functions of the Bayes Explainer
Educational Support on Bayesian Inference
Example
Explaining the use of Bayesian methods in machine learning algorithms like Naive Bayes classifiers, and the role of priors and likelihoods in updating beliefs about model parameters.
Scenario
A user, unfamiliar with Bayesian methods, requests an explanation of priors. Bayes Explainer provides a step-by-step guide, using real-world data to illustrate how prior beliefs are updated with new evidence.
Visual Explanation Capabilities
Example
Interpreting charts and graphs from statistical software outputs, explaining the significance of posterior distributions visualized in these outputs.
Scenario
A user submits a histogram of a posterior distribution obtained from a Markov Chain Monte Carlo simulation. Bayes Explainer analyzes the image, identifies key features of the distribution, and explains implications for the user’s research question.
Customization to User Expertise
Example
Adapting explanations of Bayesian statistics topics, from basic concepts like Bayes' Theorem to more advanced topics like hierarchical Bayesian models.
Scenario
An advanced user asks about hierarchical Bayesian models for data analysis in psychology. Bayes Explainer delves into the multi-level structure, use of hyperpriors, and advantages over traditional frequentist methods, with relevant examples and applications.
Ideal Users of Bayes Explainer Services
Statistics Students
Students taking courses in statistics, especially those with a focus on Bayesian statistics, can benefit from tailored explanations, step-by-step problem-solving guidance, and visual aids provided by Bayes Explainer to deepen their understanding of complex statistical theories and practices.
Research Scientists and Analysts
Researchers and data analysts in fields that utilize Bayesian inference, such as bioinformatics, psychology, and political science, can use the Bayes Explainer to interpret data outputs, refine models, and understand the statistical underpinnings of their analyses.
Machine Learning Engineers
Professionals and enthusiasts in machine learning who implement Bayesian methods in their models, like Bayesian neural networks, can leverage the detailed, context-sensitive explanations and visual interpretation skills of Bayes Explainer to optimize and debug their algorithms.
Guide to Using ML
Start with a Free Trial
Visit yeschat.ai to access a free trial instantly, no signup or ChatGPT Plus subscription required.
Explore Features
Familiarize yourself with the various functionalities of ML, such as Bayesian inference analysis, image interpretation, and interactive Q&A sessions.
Define Your Objectives
Identify specific goals you want to achieve using ML, whether for academic purposes, research, or practical applications in data science.
Engage with the Tool
Use the tool to solve specific problems or to clarify concepts in Bayesian statistics, experimenting with different types of data inputs and queries.
Evaluate and Iterate
Review the outcomes and insights gained from using ML, and refine your queries or methods based on feedback and results to optimize learning or solution-finding.
Try other advanced and practical GPTs
100% Human Writing With Ai Content Detector
Detect AI, Embrace Authenticity
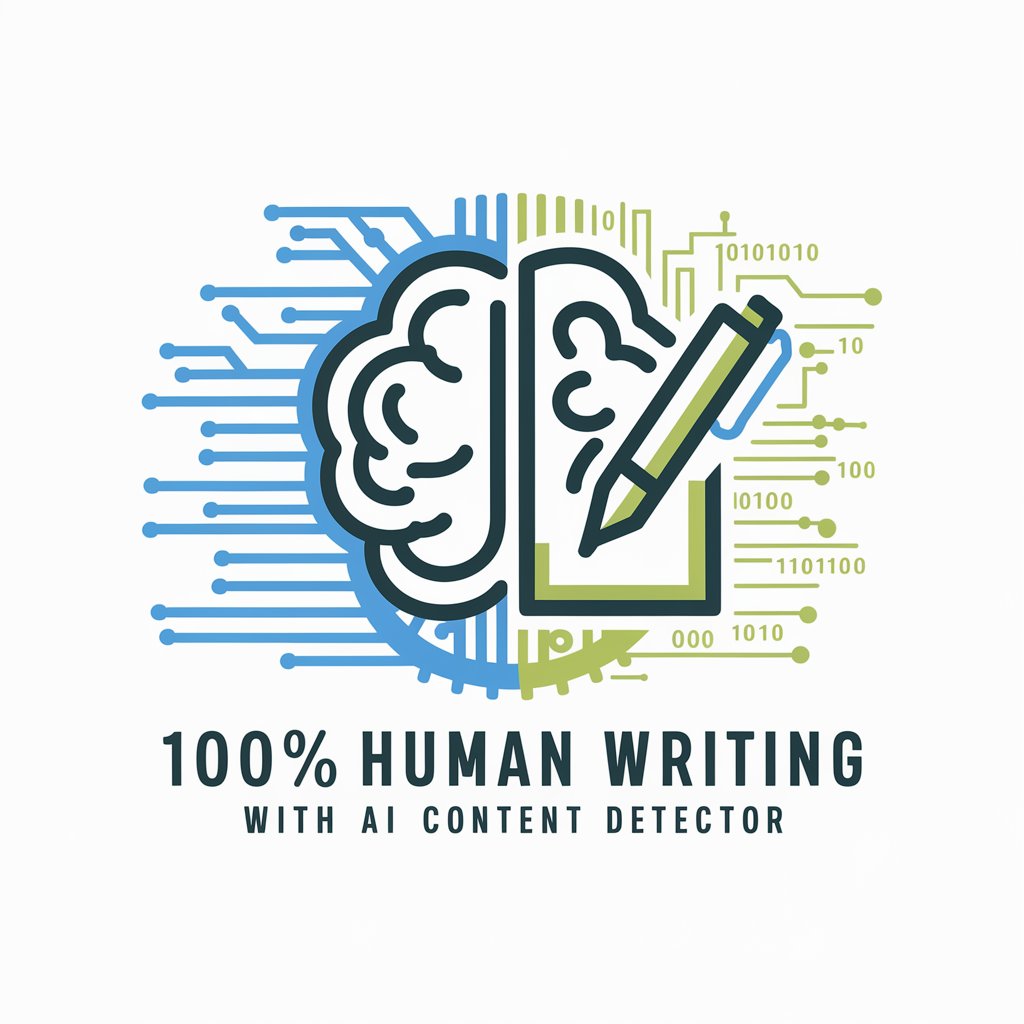
AAA AI Content Detector
Discover AI's Imprint in Your Text
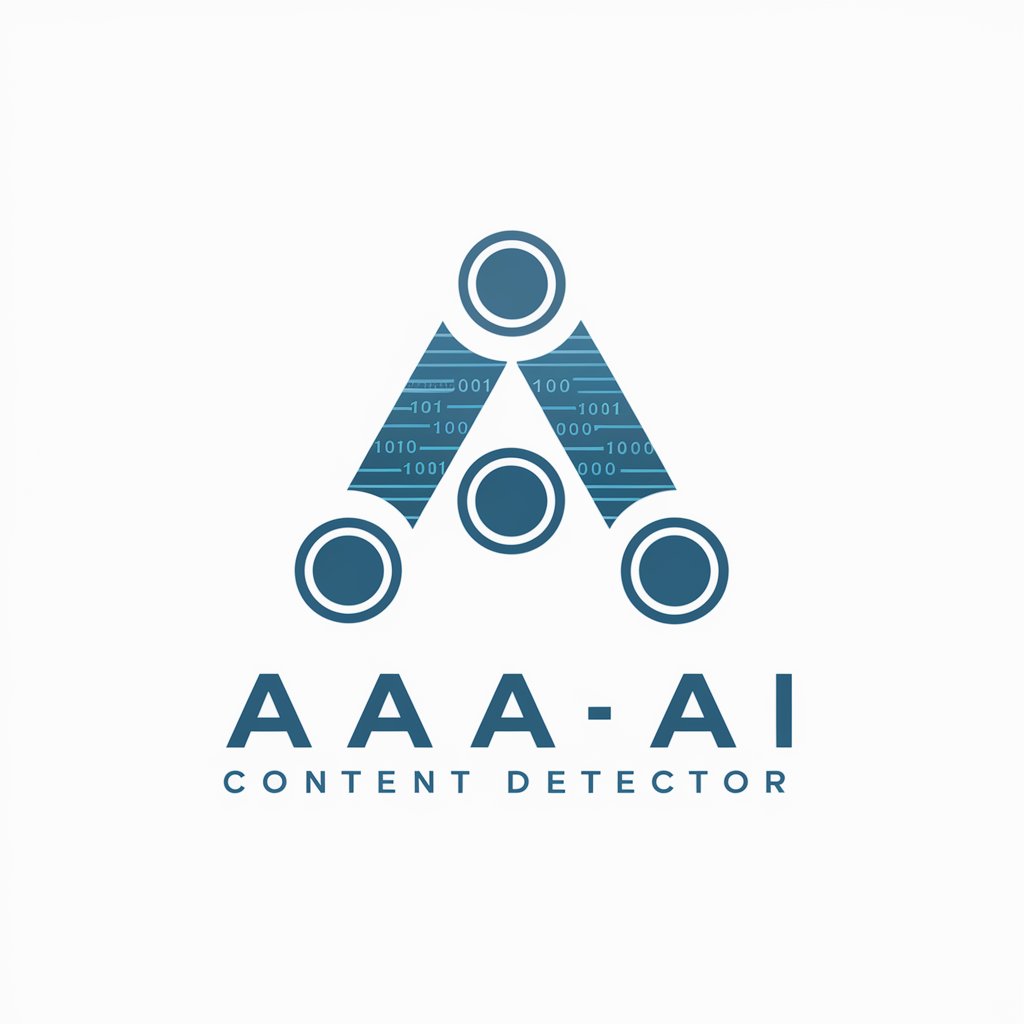
AI Detector -> Human Content
Unveil AI content with precision
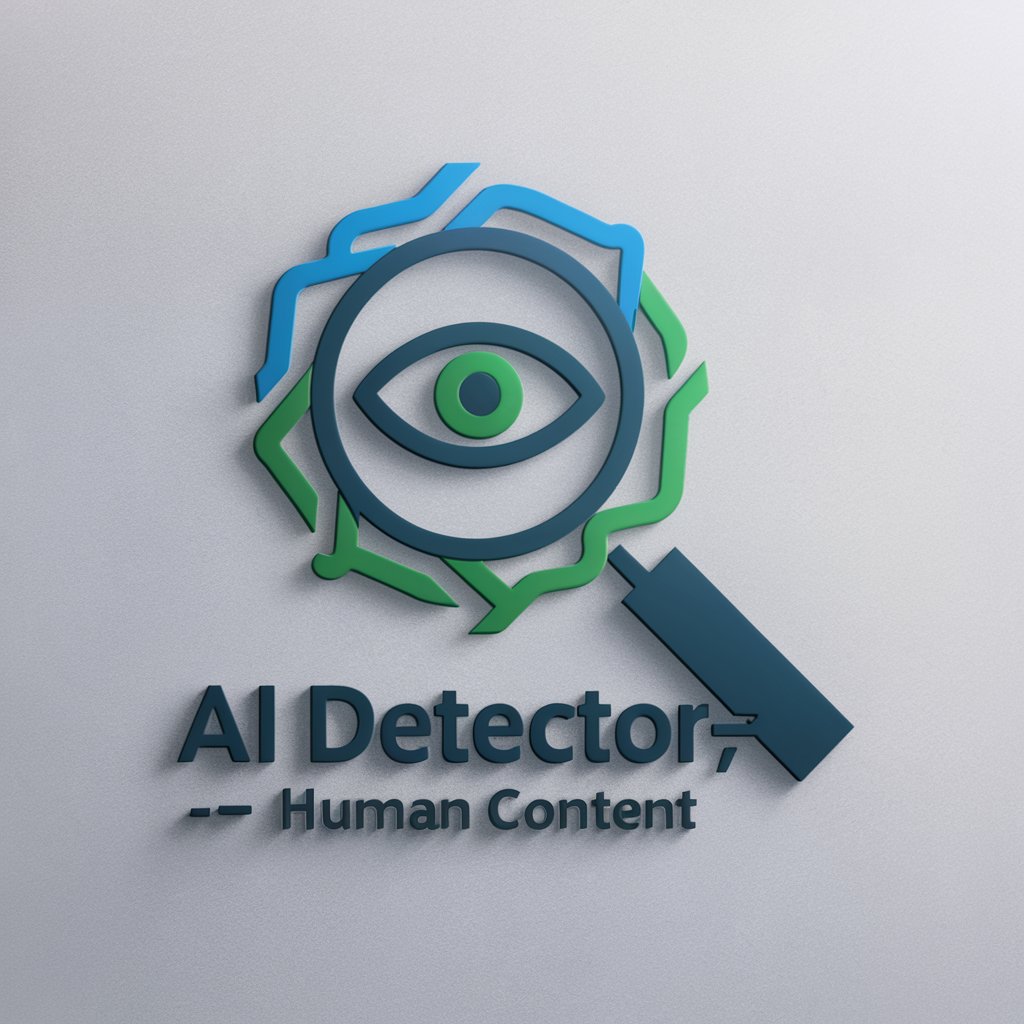
TechTrend AI
Stay Ahead with AI-Powered Insights
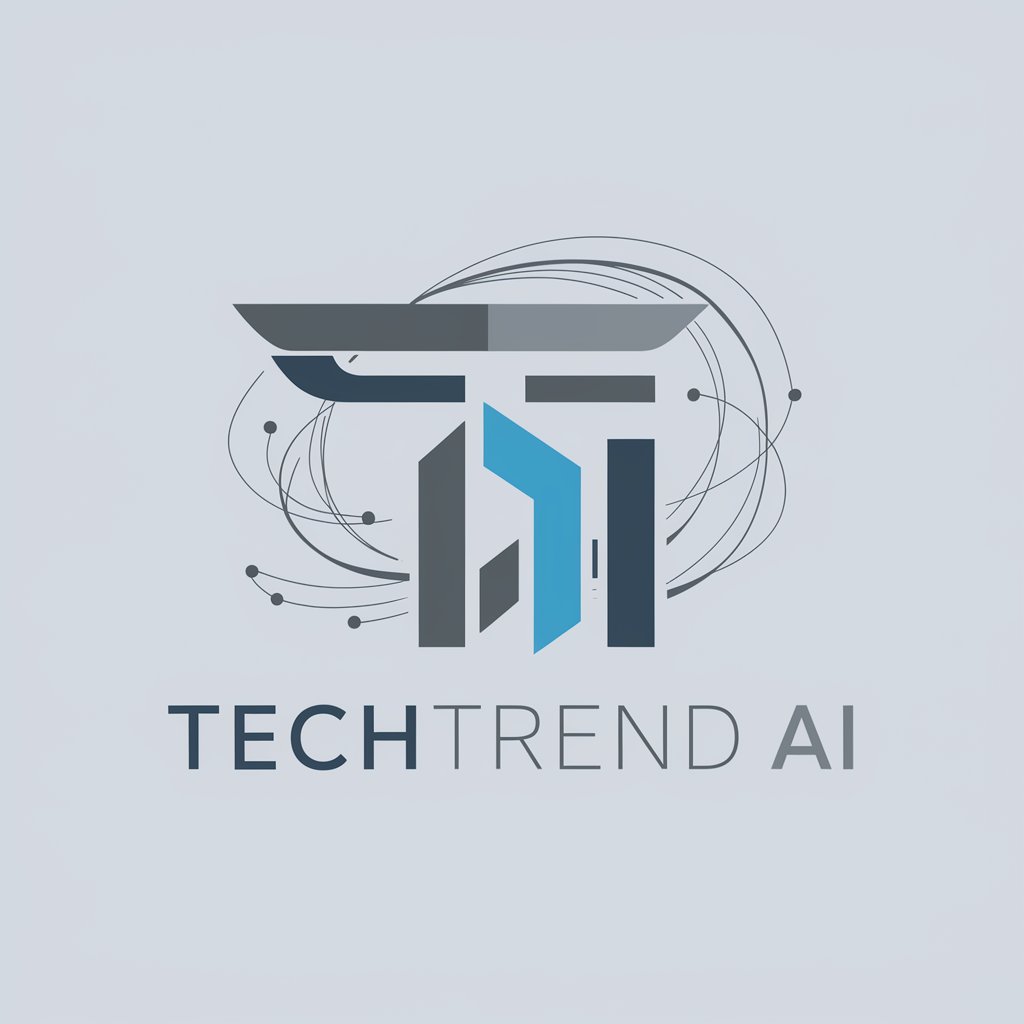
Mental Health DocuGen
Streamlining Mental Health Documentation with AI
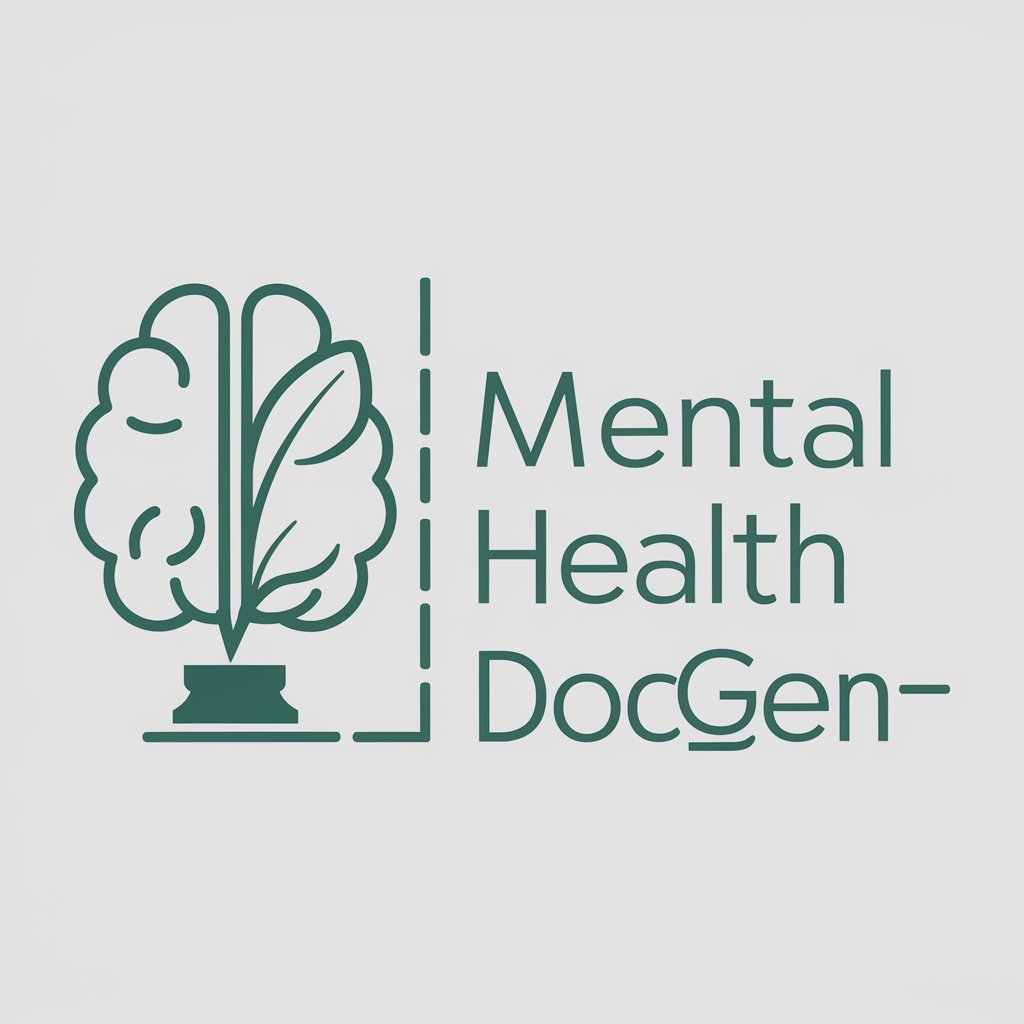
Free AI Note Taking
AI-powered Note-Taking Simplified
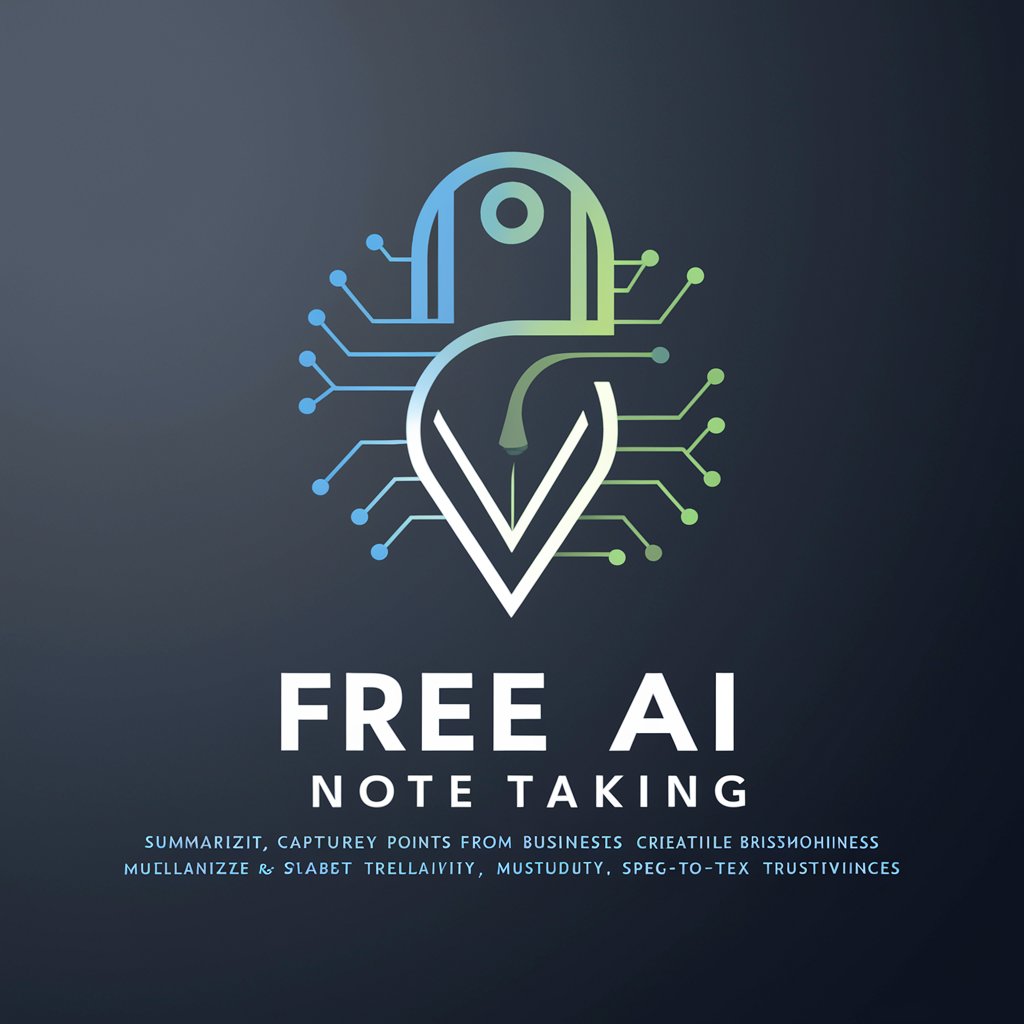
ML Mentor
Empowering your machine learning journey with AI.
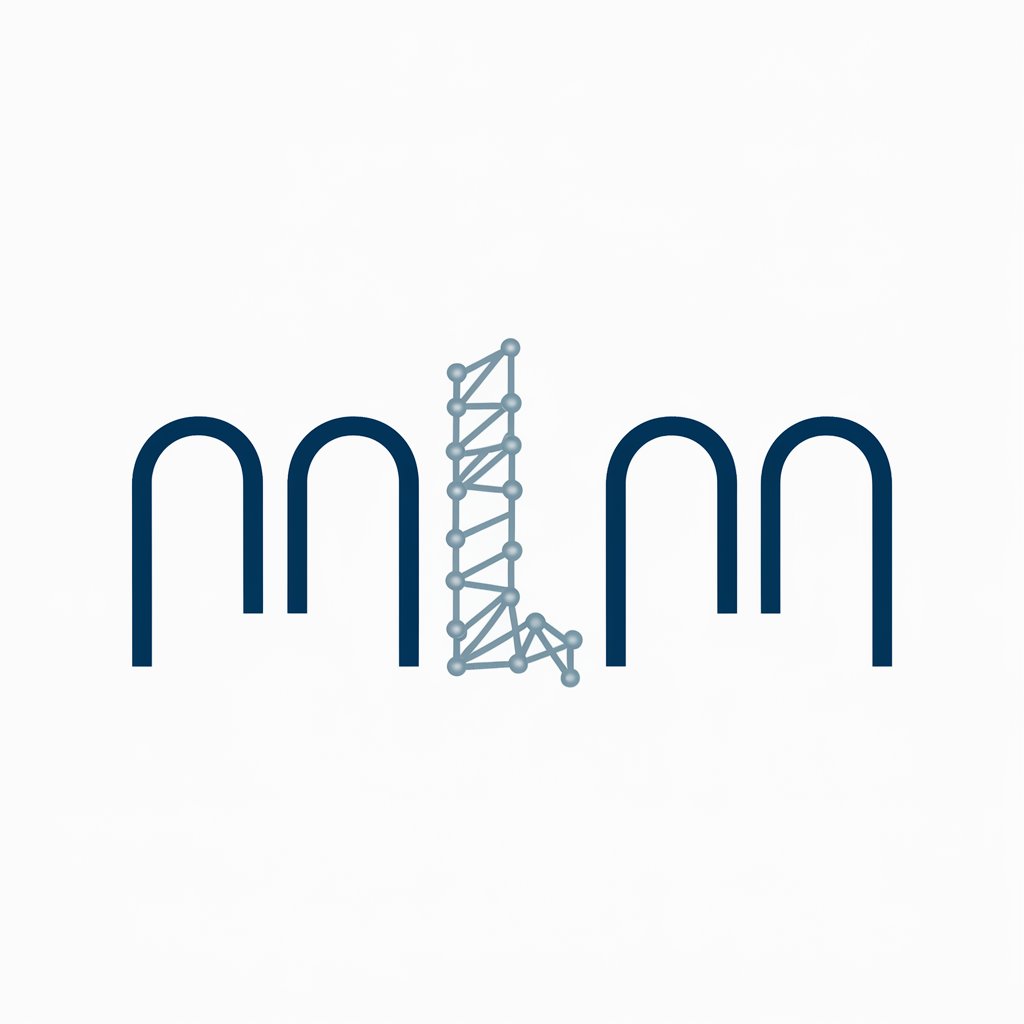
ML
Empowering Learning with AI

ML Explainer
Unraveling AI with AI-powered explanations
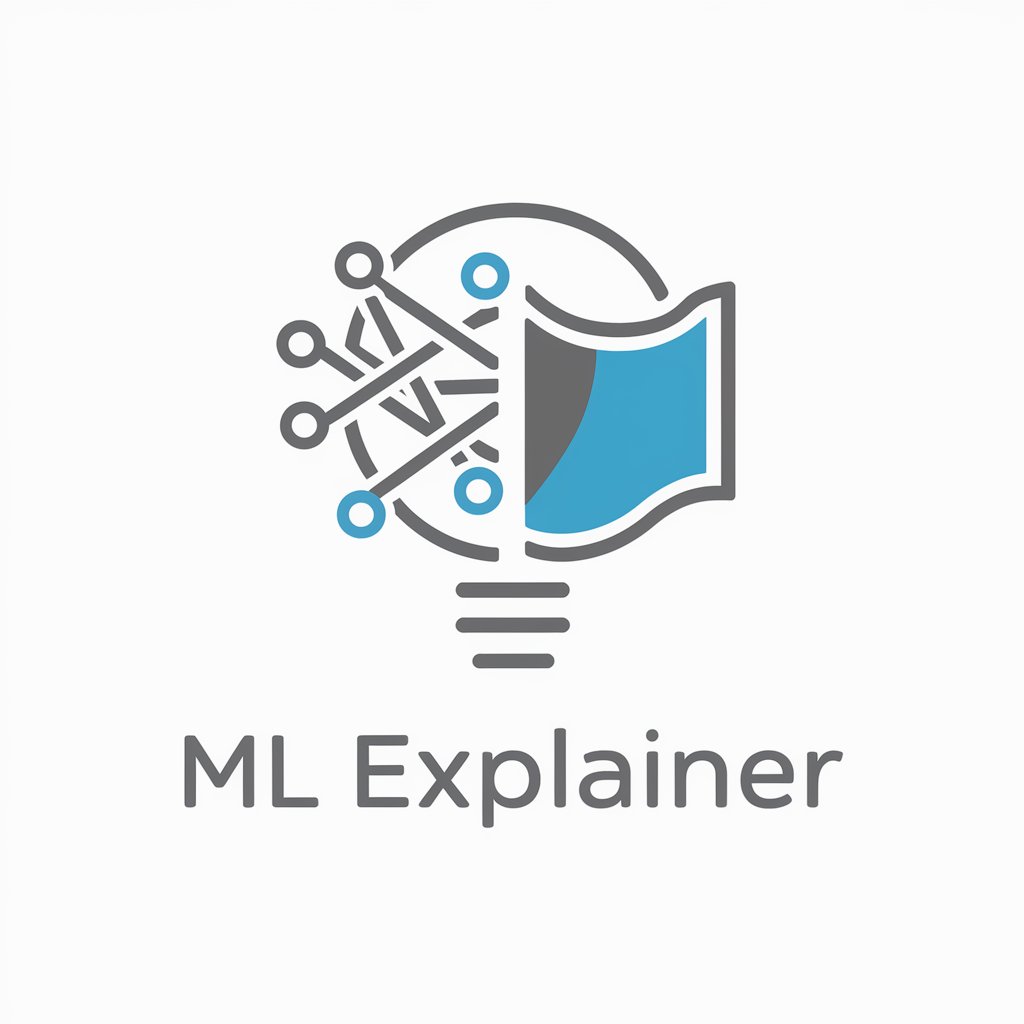
ML Wizard
Harnessing AI for Smarter Learning
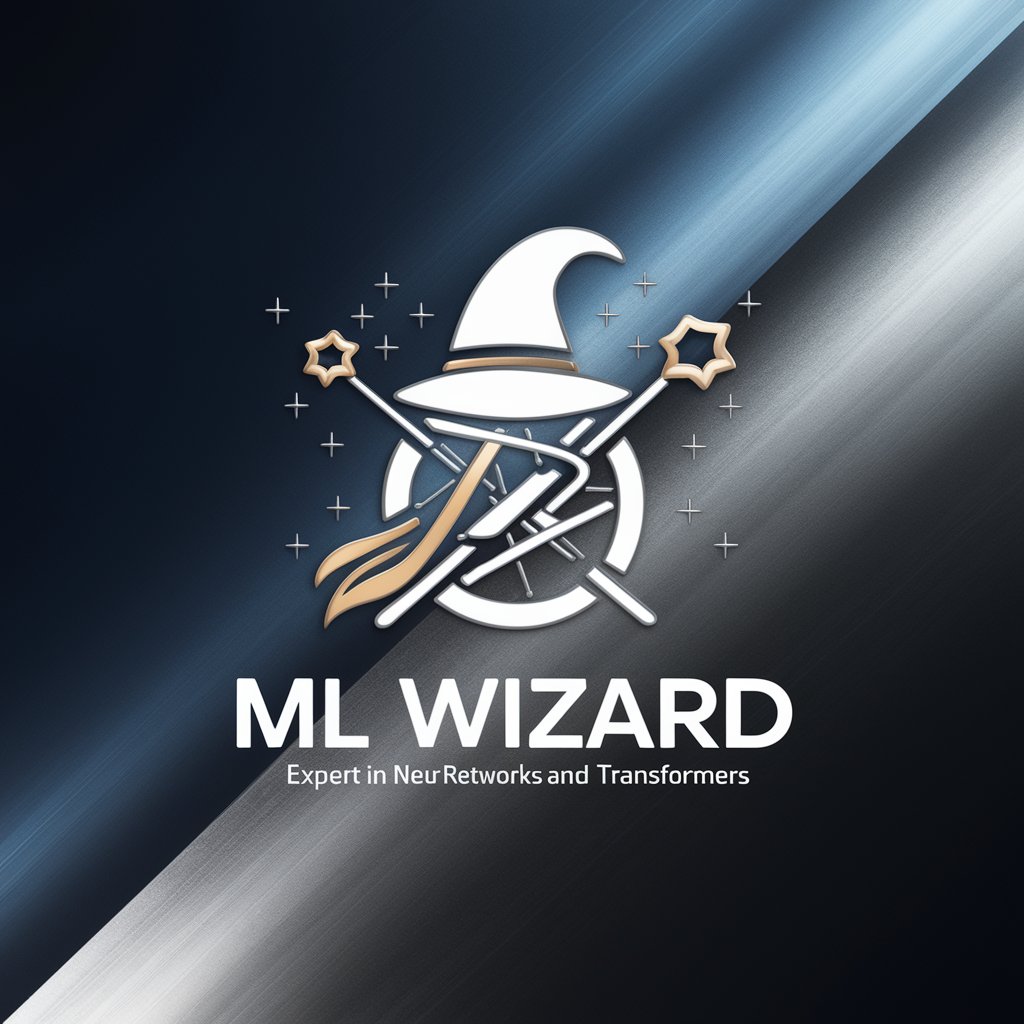
ML Tutor
Learn AI with your personal AI Tutor
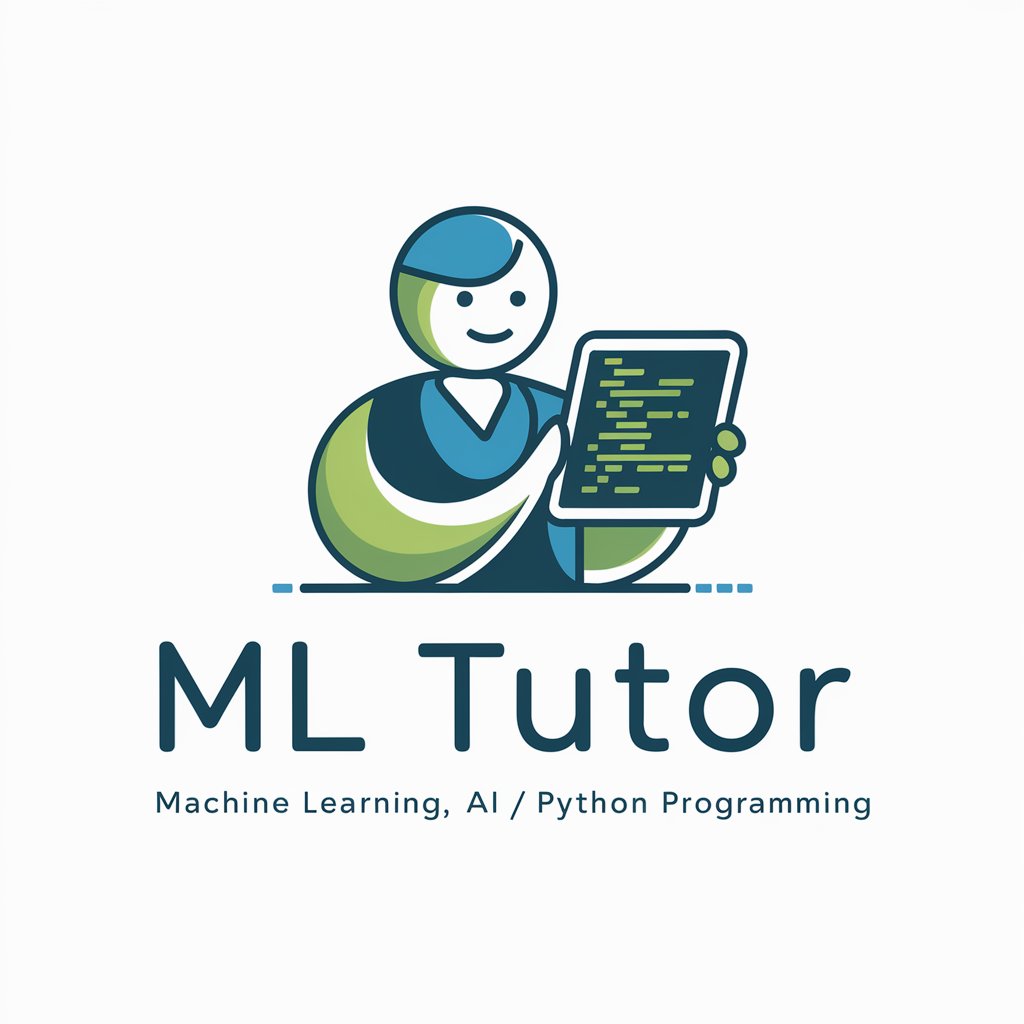
ML Coder
AI-driven Insights into Your ML Code
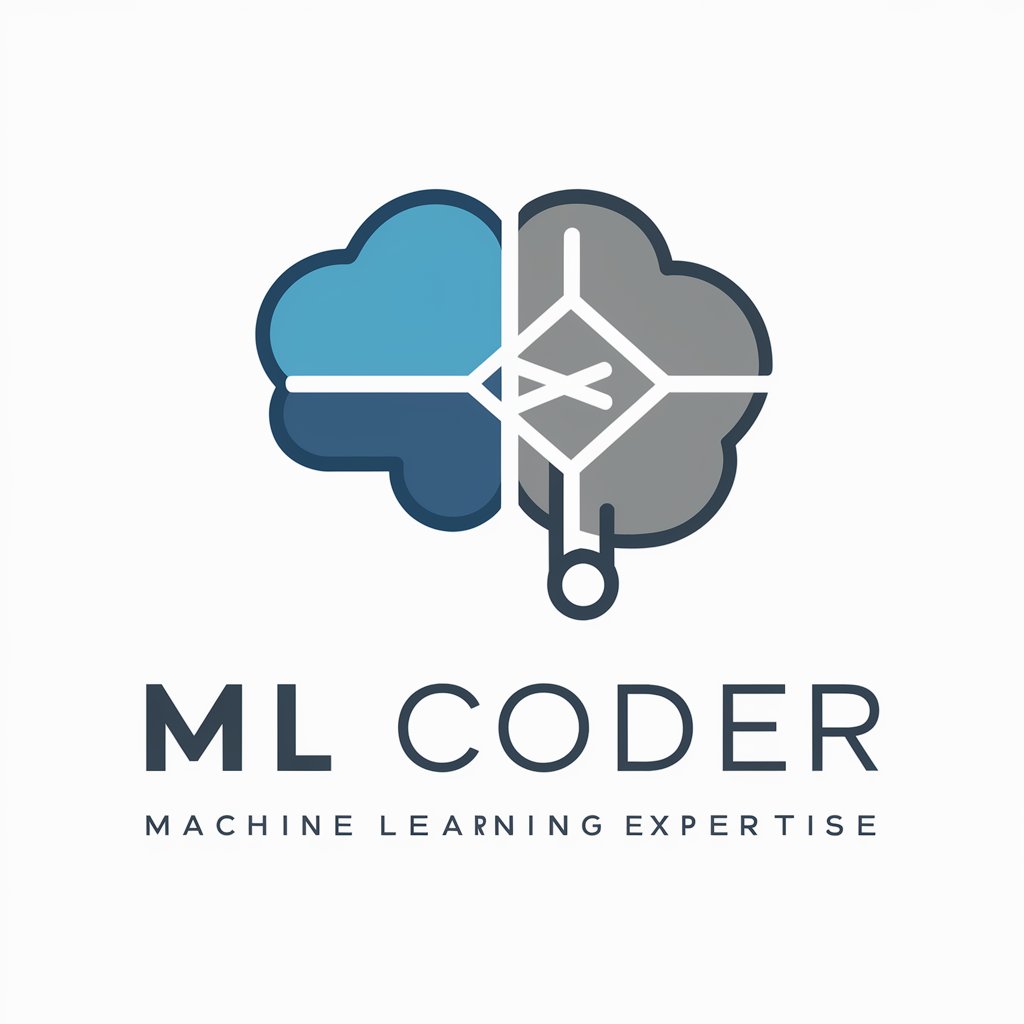
Frequently Asked Questions about ML
What is Bayesian Inference?
Bayesian inference is a method of statistical inference in which Bayes' theorem is used to update the probability for a hypothesis as more evidence or information becomes available.
How can ML assist in non-conjugate models?
ML can help analyze and interpret non-conjugate models by facilitating complex probability distributions and providing computational techniques to manage inference in these models, which lack simple analytical solutions.
What types of Bayesian classifiers can be explored with ML?
ML supports exploration of various Bayesian classifiers, including Naive Bayes, Bayesian Network, and others, allowing users to apply these classifiers to real-world data sets and scenarios.
Can I use ML for real-time data analysis?
Yes, ML is capable of handling real-time data analysis by applying Bayesian methods to continuously update beliefs and probabilities as new data flows in, making it ideal for dynamic and evolving datasets.
What makes ML different from other Bayesian tools?
ML distinguishes itself by offering specialized support for non-conjugate models and a user-friendly interface that simplifies complex statistical concepts, making Bayesian inference accessible to a wider audience.