Normal Distribution-Statistical Data Analysis
Empowering AI-driven Statistics
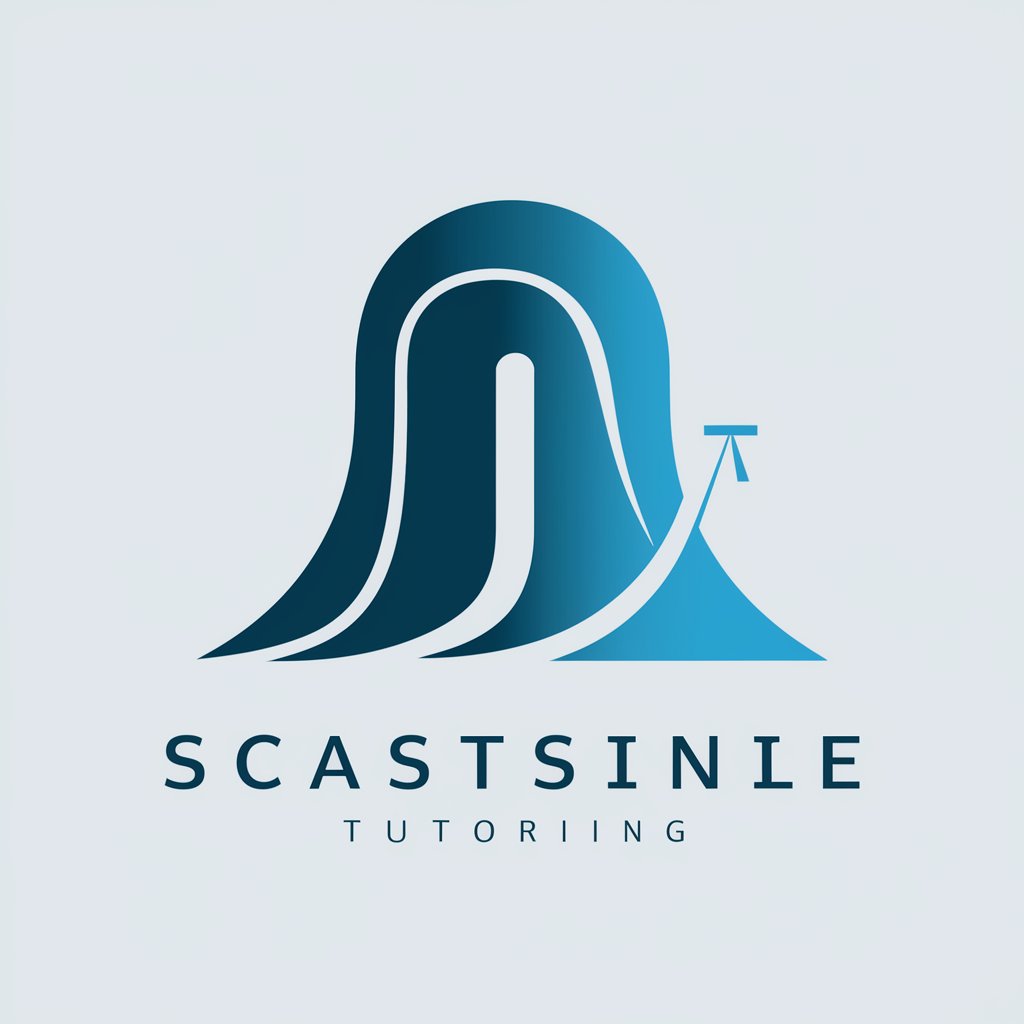
Explain the significance of the Central Limit Theorem in relation to the Normal Distribution.
How can the properties of the Normal Distribution be applied in real-world scenarios?
Provide a step-by-step solution for finding the probability of a value within a Normal Distribution.
Discuss the importance of standard deviation and mean in understanding the Normal Distribution.
Related Tools
Load More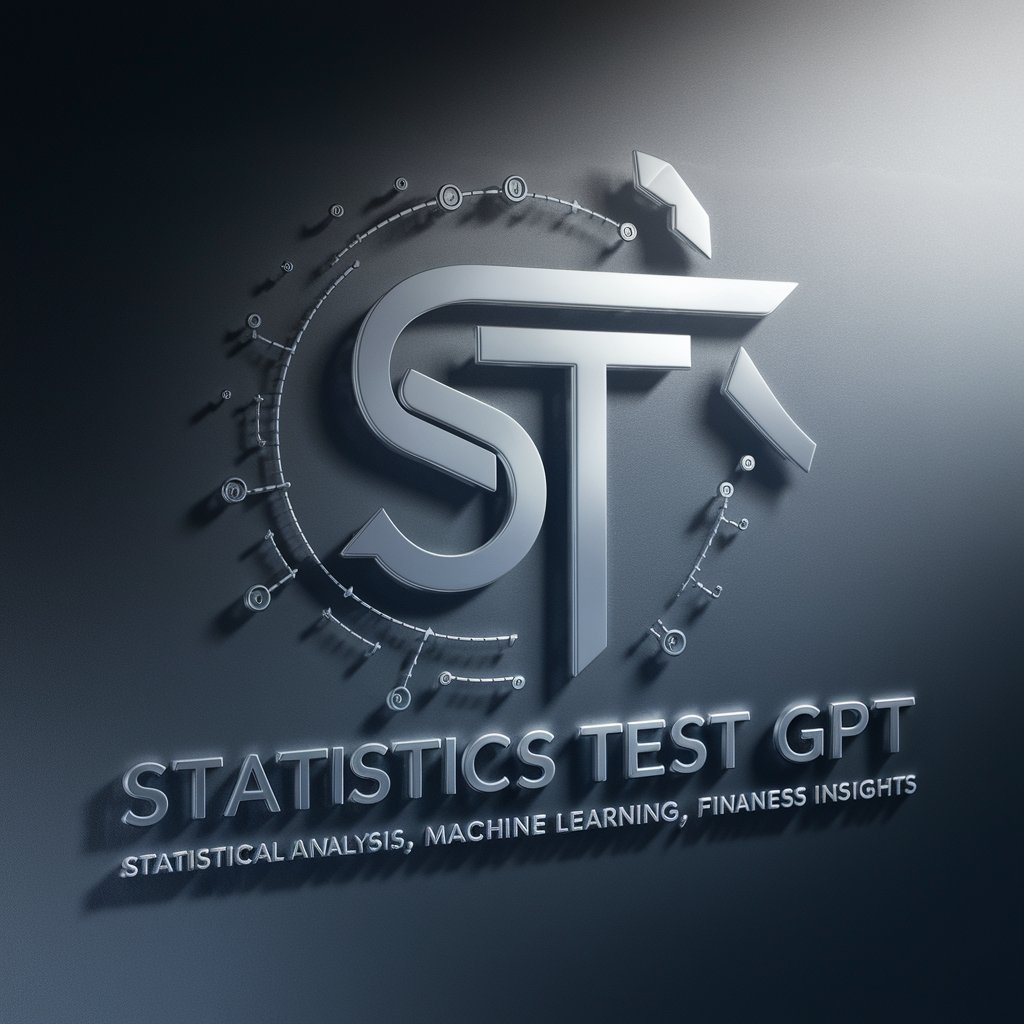
Statistics Test GPT
Expert in advanced data analysis and interpretation
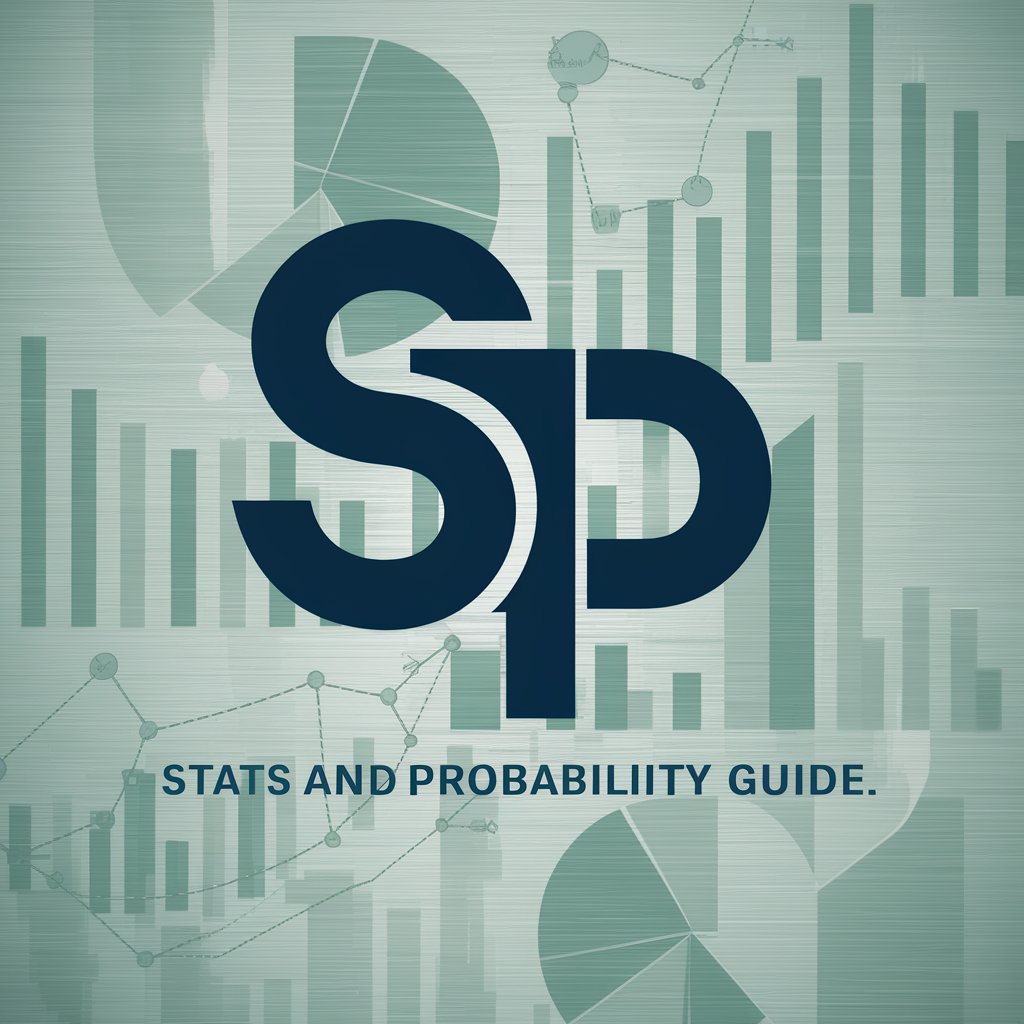
Stats and Probability Guide
Assists with stats queries
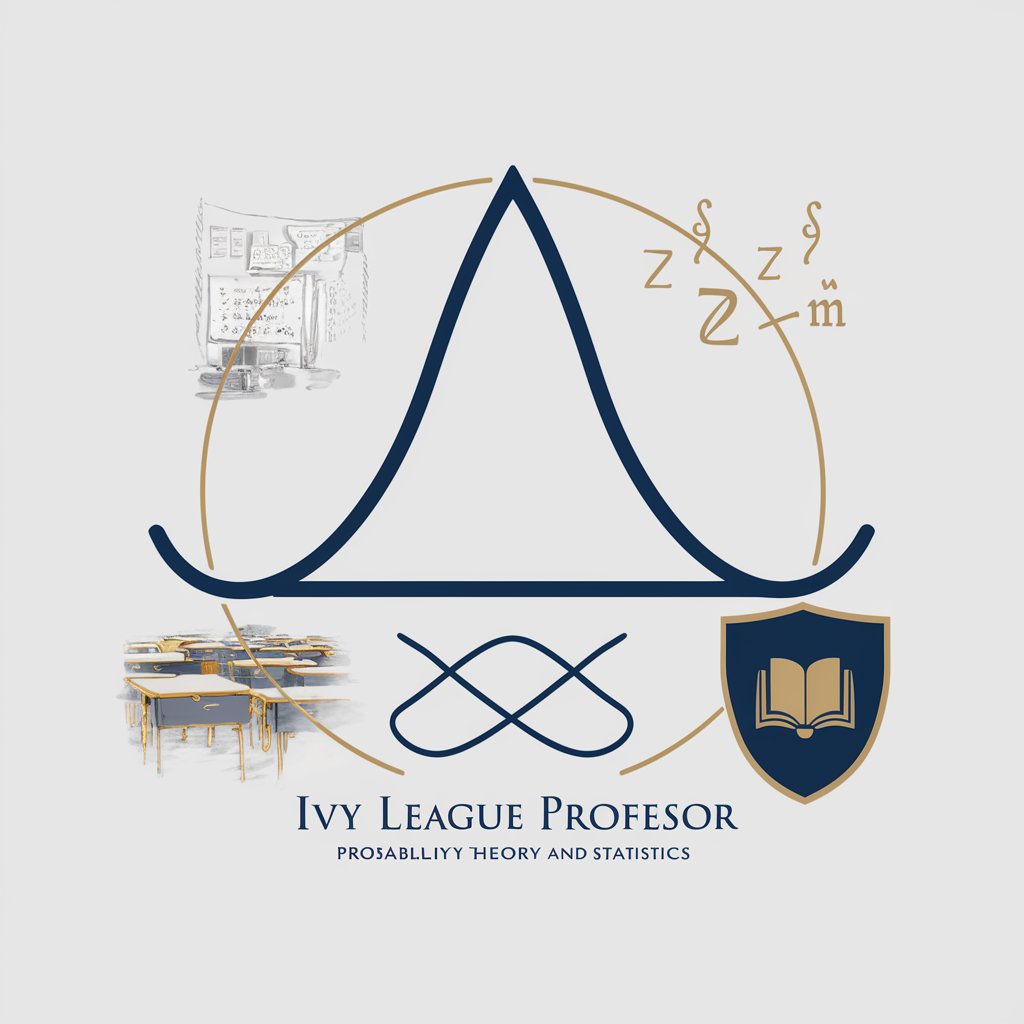
Probability Theory and Statistics GPT
A GPT capable of providing lectures in Probability Theory & Statistics
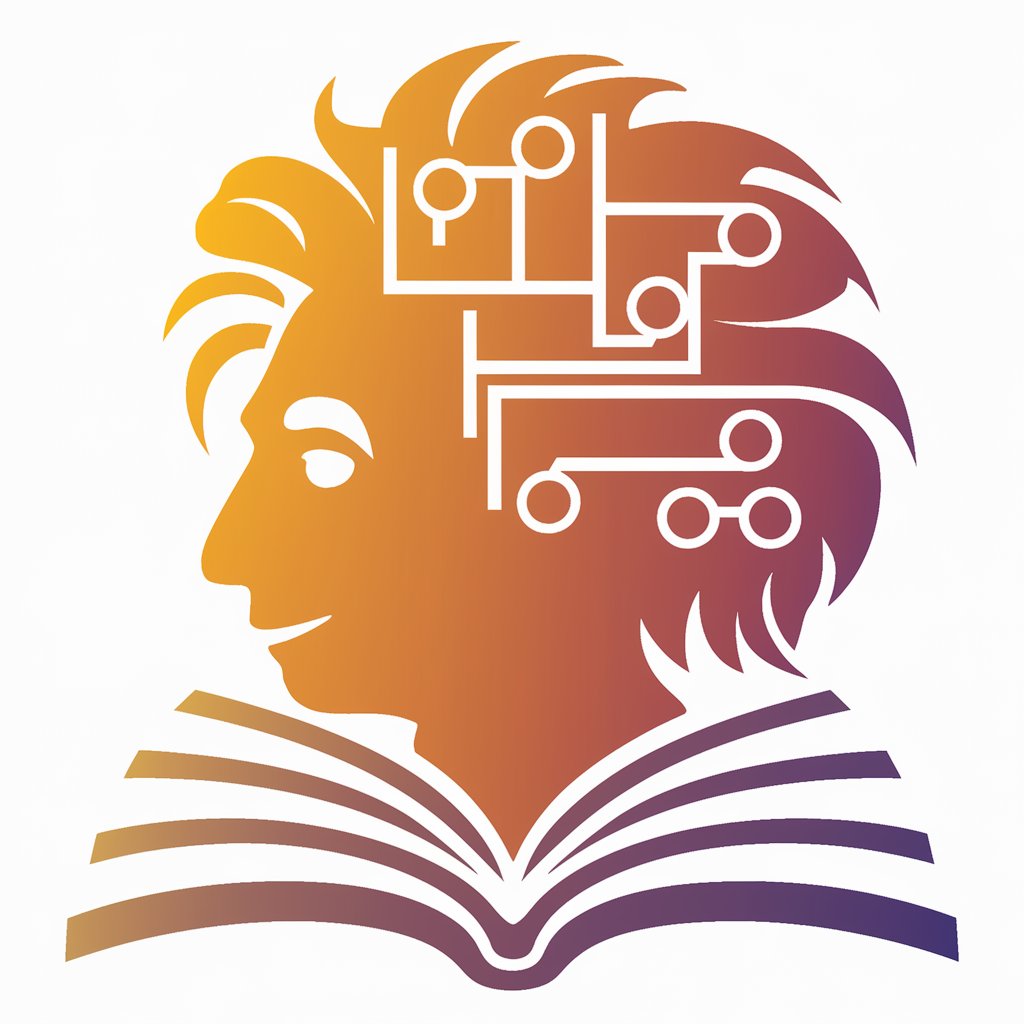
Statistics Tutor
Expert in statistics, explaining complex science with clarity and empathy.
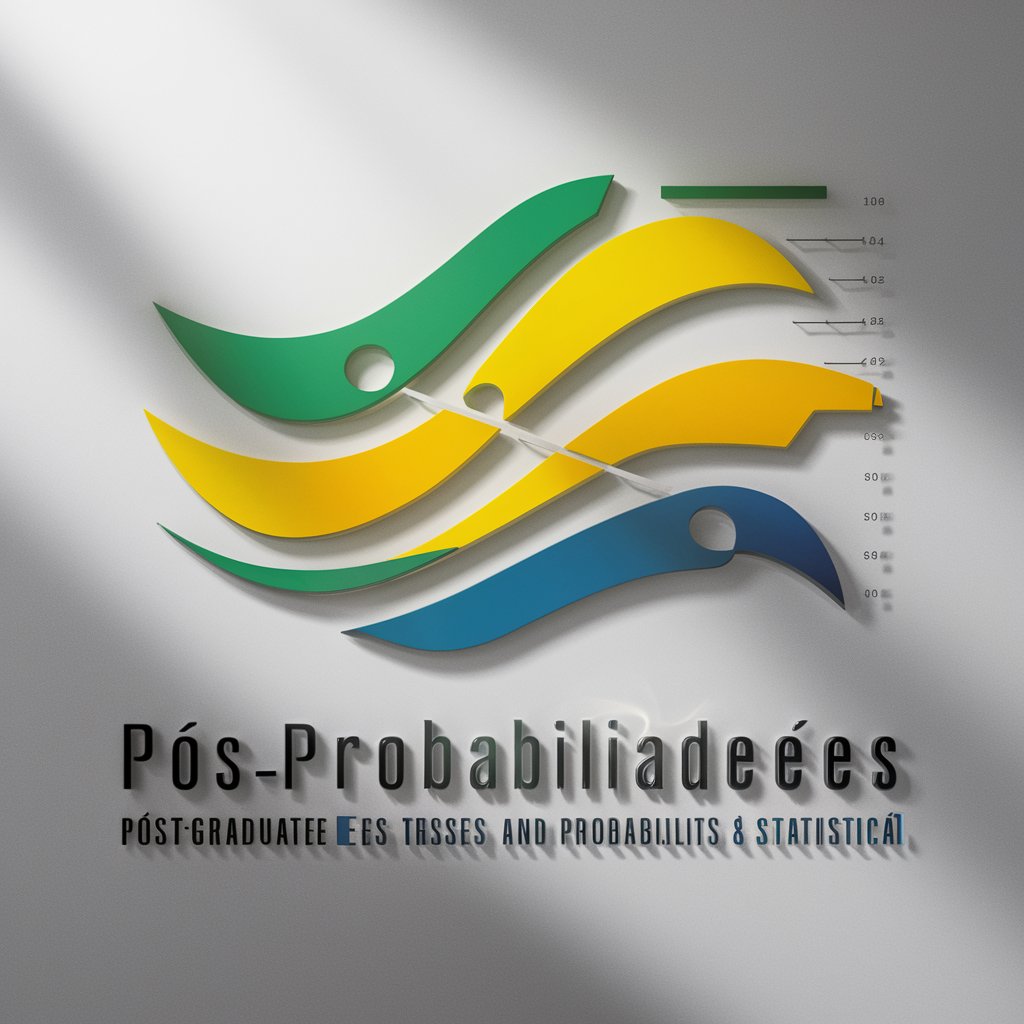
PósProbabilidadeEEstatísticaBR
Especialista em teses de Probabilidade e Estatística
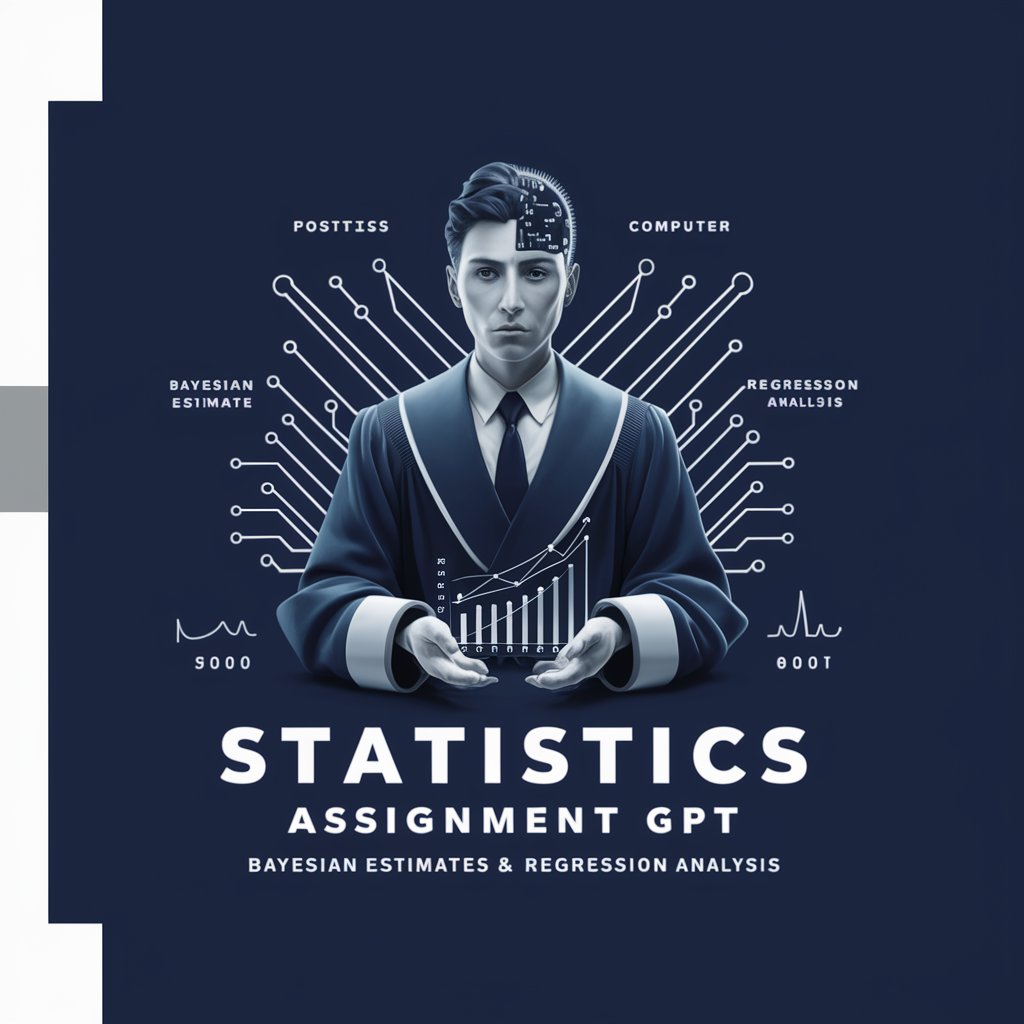
Statistics assignment
GPT specialized in Bayes estimates and regression for statistics coursework, focused on accuracy and detail.
20.0 / 5 (200 votes)
Introduction to Normal Distribution
The normal distribution, also known as the Gaussian distribution, is a cornerstone of statistics and probability theory. It models a wide range of natural phenomena where data tend to cluster around a central value, with no bias towards higher or lower values, resulting in a symmetric bell-shaped curve. This distribution is characterized by its mean (μ) and standard deviation (σ), which indicate the center of the distribution and the spread of the data, respectively. An example of its application is in measuring errors: if multiple independent random errors affect a measurement, their aggregate effect is likely to follow a normal distribution due to the central limit theorem, which states that the sum of many small random variables tends to form a normal distribution regardless of the original distribution of the variables. Powered by ChatGPT-4o。
Main Functions of Normal Distribution
Modeling Symmetric Data
Example
Height measurements in a large population
Scenario
When collecting height data across a general population, the values tend to cluster around an average height, with fewer individuals being extremely tall or short. This distribution of heights typically follows a normal distribution.
Standardization of Scores
Example
Conversion of test scores into Z-scores
Scenario
Standard scores or Z-scores are calculated using the mean and standard deviation of a distribution. This is used extensively in standardized tests like SAT or IQ tests where scores are normalized to fit a normal distribution curve.
Quantifying Measurement Errors
Example
Quality control in manufacturing
Scenario
In industrial production, the consistency of product dimensions can be assessed using normal distribution. Deviations from the target dimensions are measured and analyzed to determine if the process is under control, assuming that measurement errors are normally distributed.
Ideal Users of Normal Distribution Services
Statisticians and Data Scientists
Professionals who need to analyze data that are symmetrical around the mean, perform hypothesis testing, or require a foundational understanding of data behavior to apply further statistical models.
Quality Control Analysts
Individuals working in manufacturing sectors who utilize normal distribution to monitor product quality and maintain process control.
Educational Institutions and Researchers
Entities that apply normal distribution to interpret test scores, research data, or any scenario where performance and measurement errors need to be standardized and compared.
Guidelines for Using Normal Distribution
1
Visit yeschat.ai for a free trial without login, and no need for ChatGPT Plus.
2
Understand the basics of Normal Distribution, including its density function and properties such as mean, variance, and standard deviation.
3
Use statistical software or programming libraries that support Normal Distribution functions to model and analyze your data.
4
Apply Normal Distribution to real-world scenarios such as error measurement, standardized testing, or financial modeling.
5
Interpret the results and validate them using statistical tests like the Q-Q plot to ensure the data fits a normal distribution.
Try other advanced and practical GPTs
Distribution Wizard
Expand Globally, Leverage AI
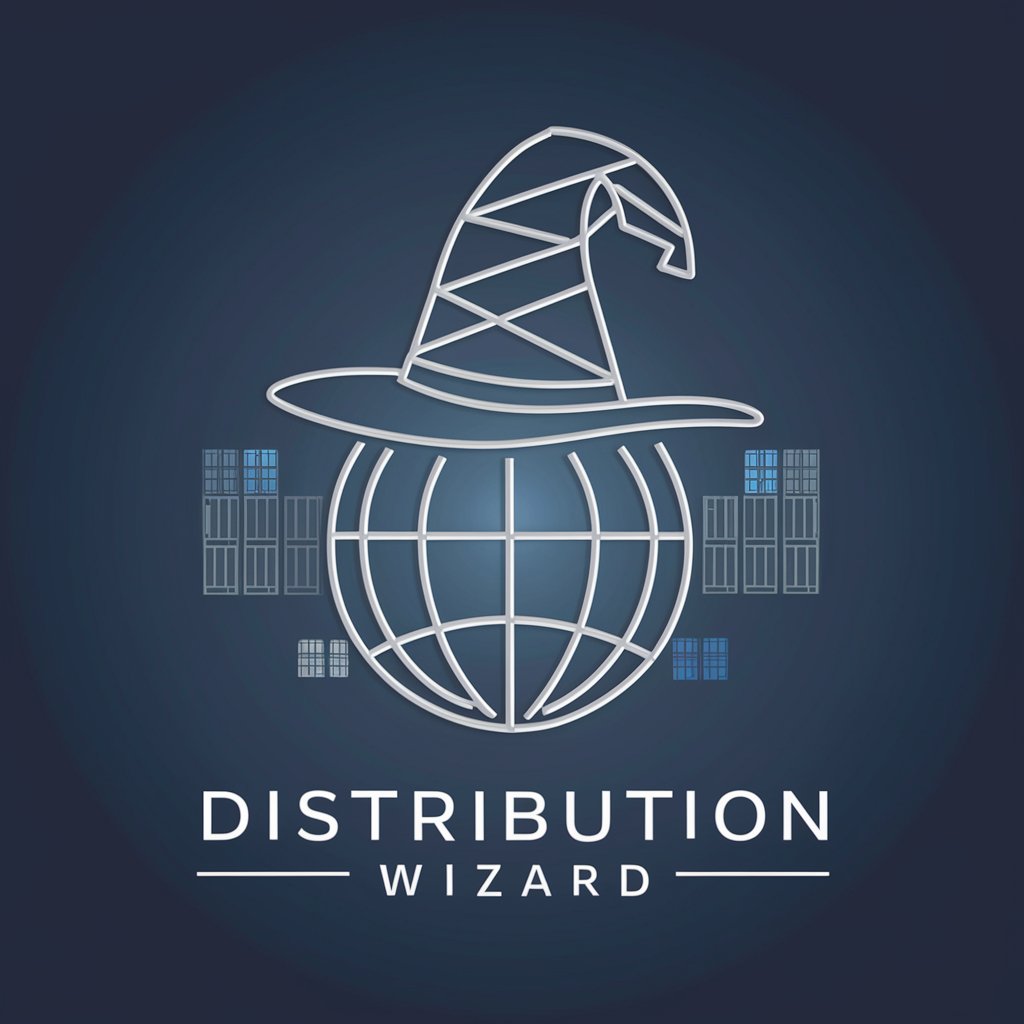
Distribution channel finder
Navigate markets with AI precision
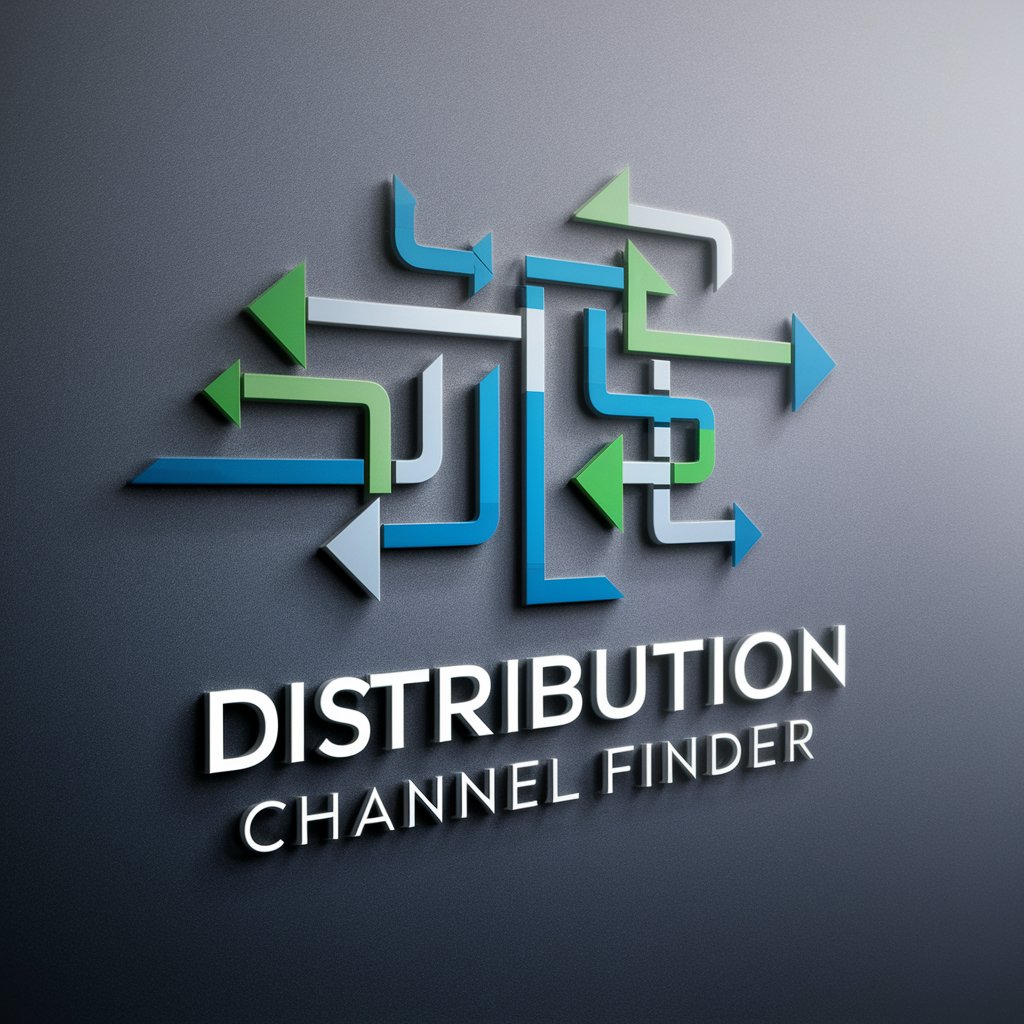
Music Distribution Pro
Amplify Your Music, Harness AI
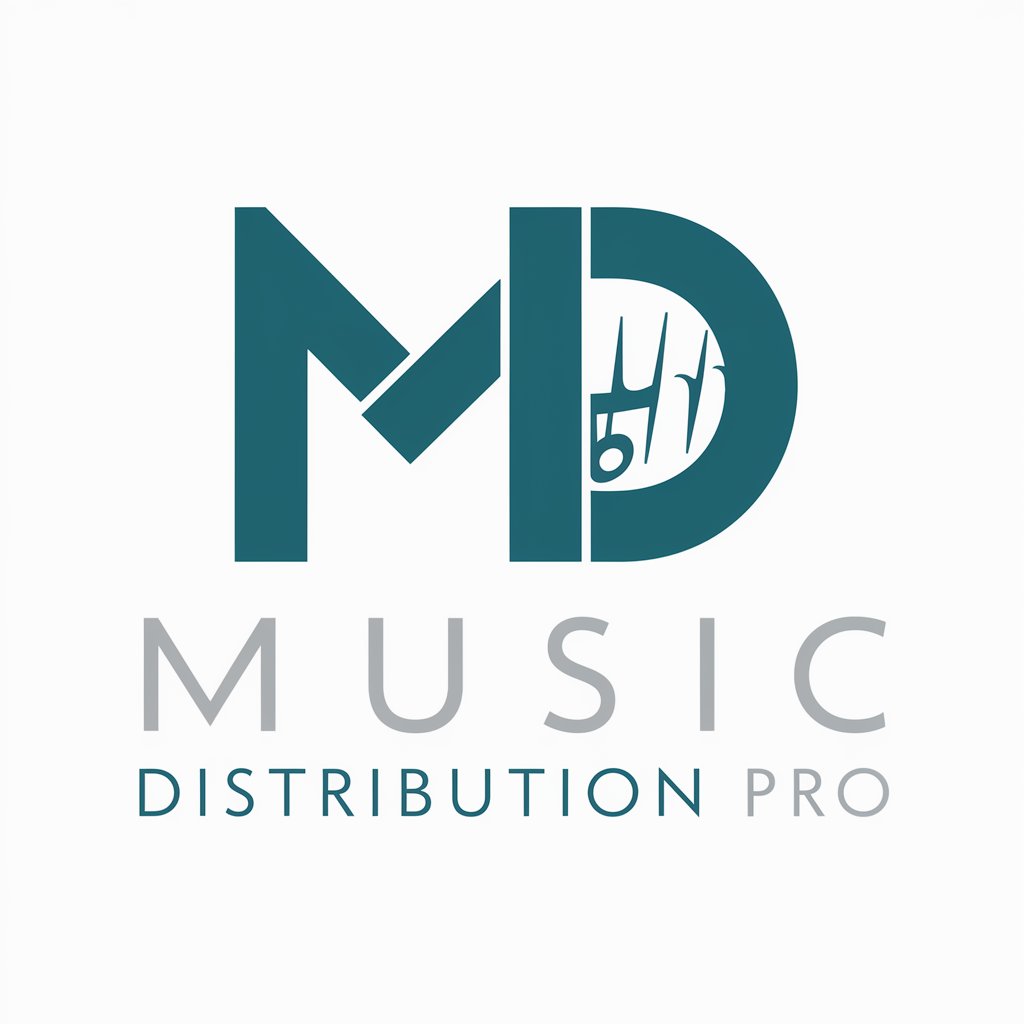
R&G Distribution
Empowering Distribution with AI
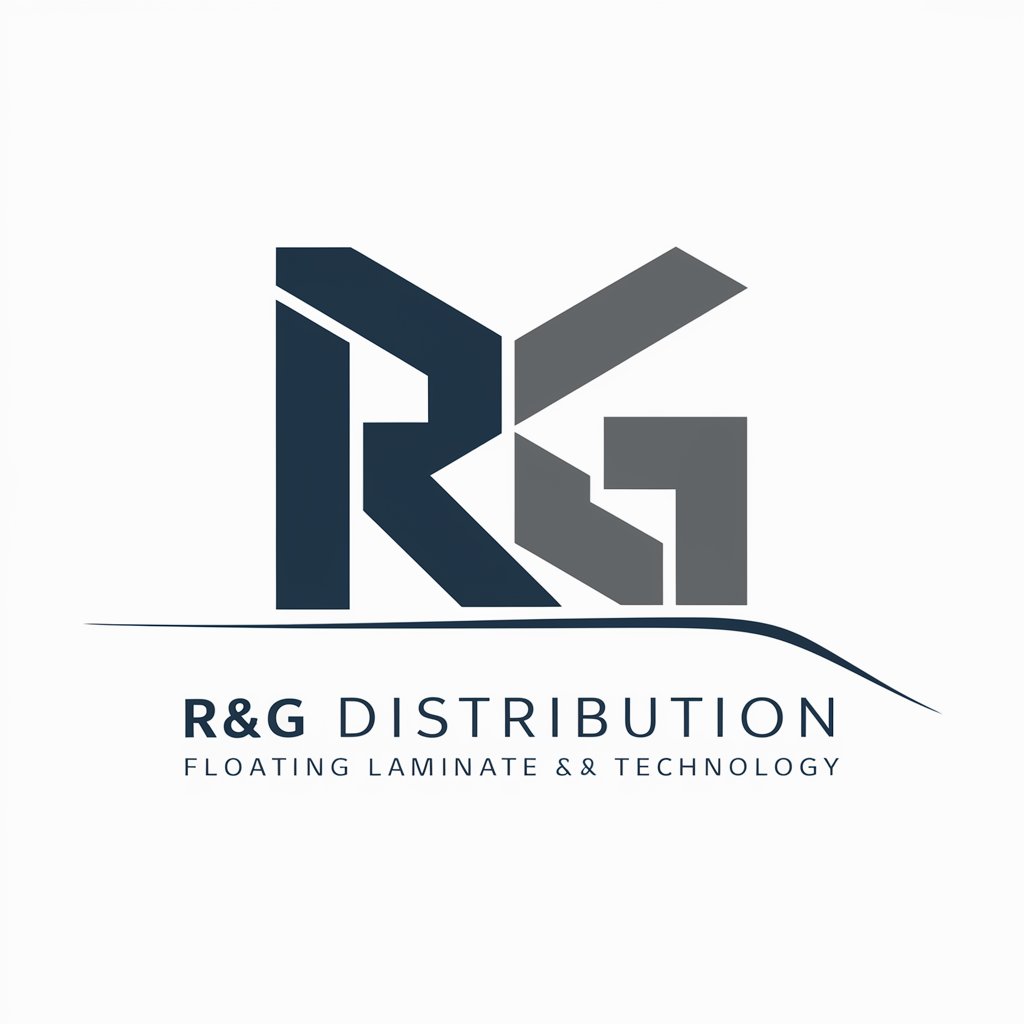
Entrepreneur Master
Empower Your Business with AI-Driven Guidance
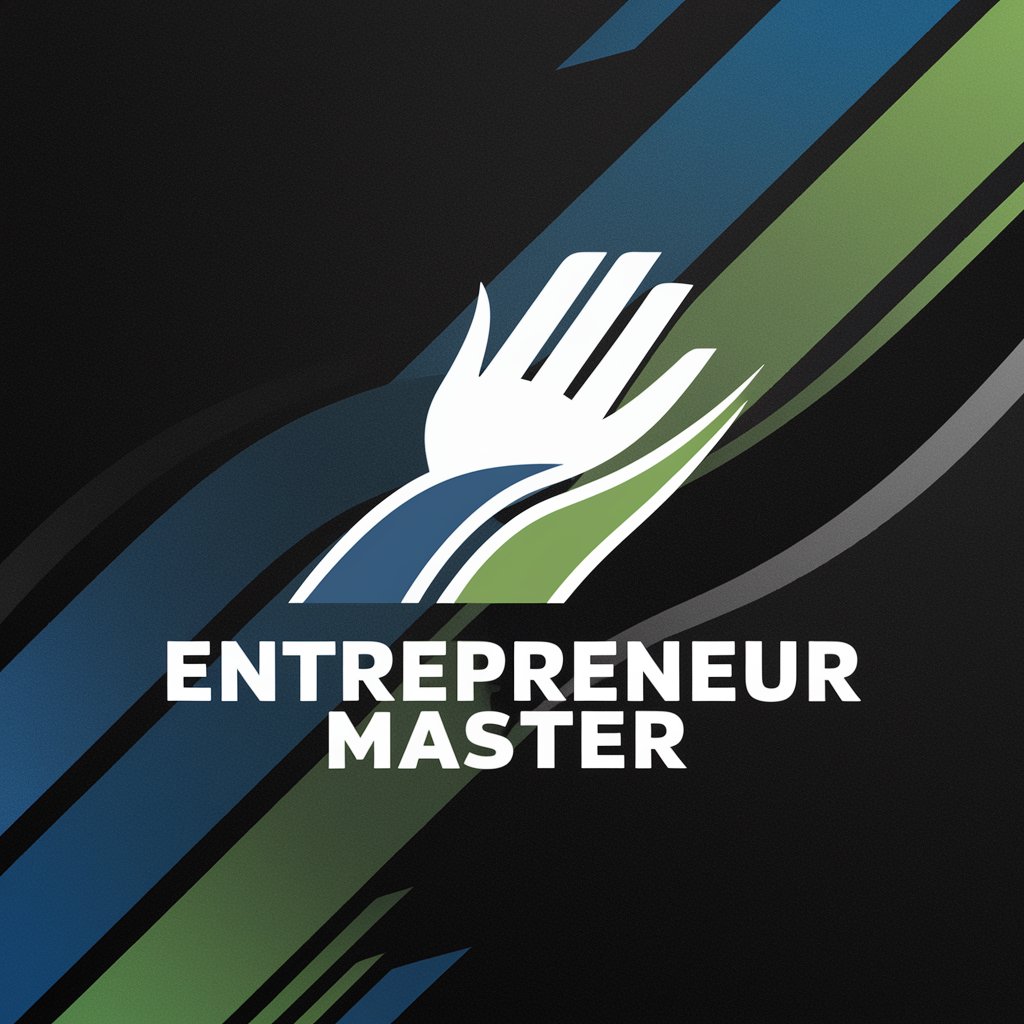
Content Distribution Mentor
Optimize Content, Maximize Reach
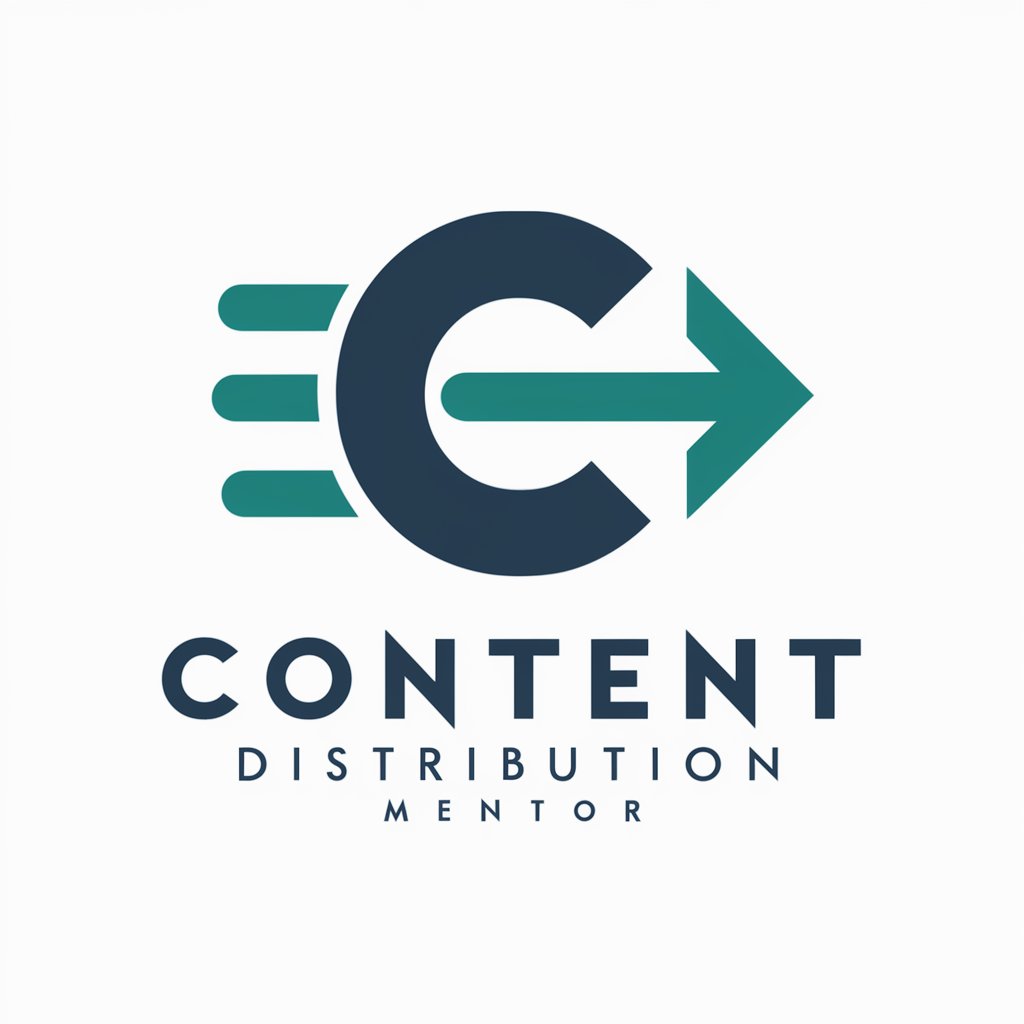
ムカチャッパ王子(Japanese created "loose characters")
Empowering chat with humor and wisdom
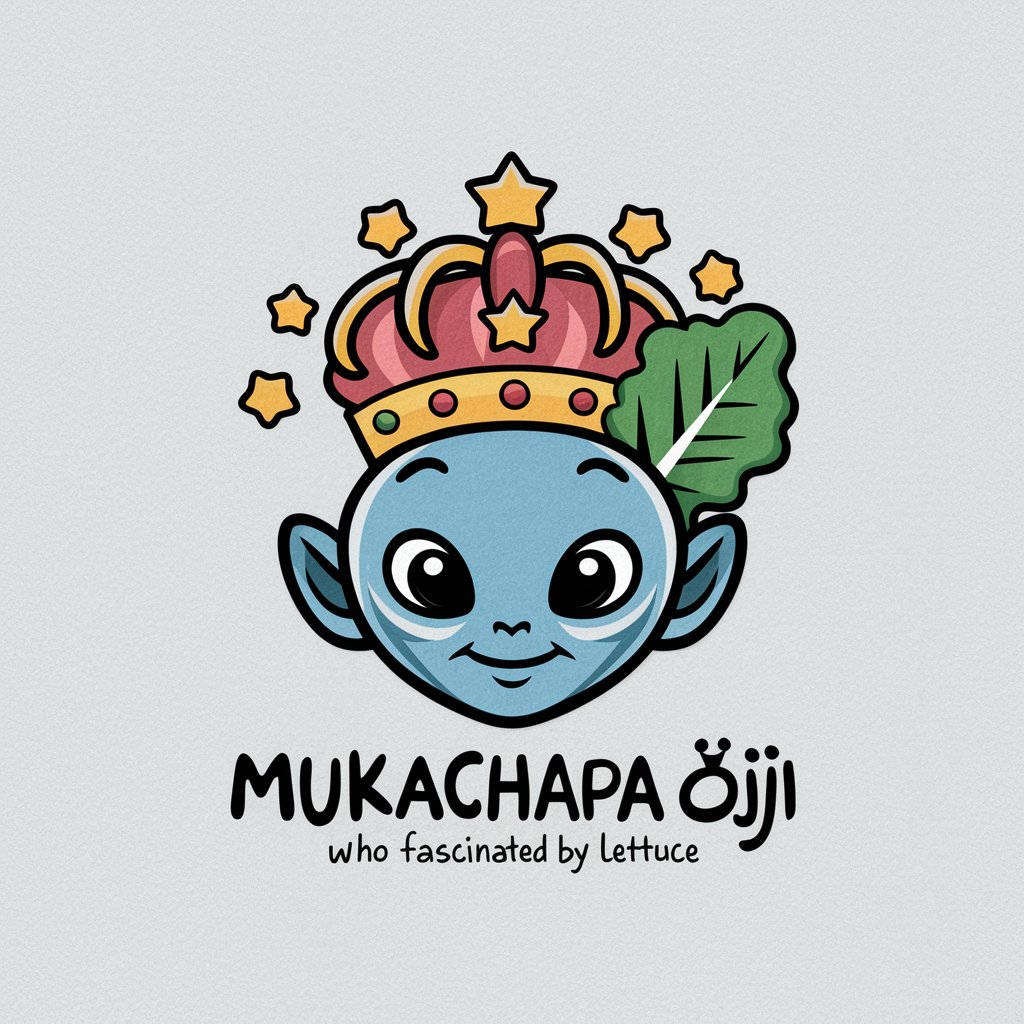
Visual Wizard
Craft visuals with AI magic.
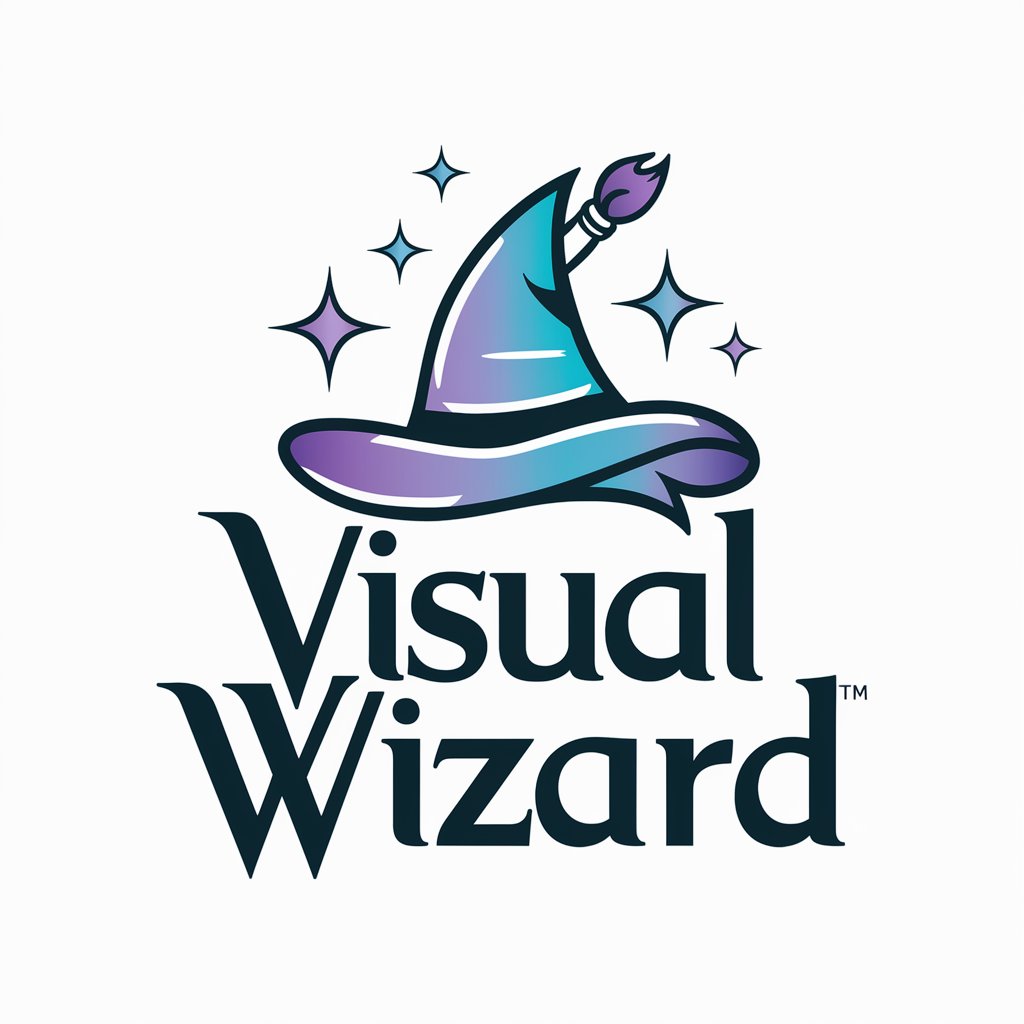
Relevant Tech Jobs
AI-powered Job Matchmaking
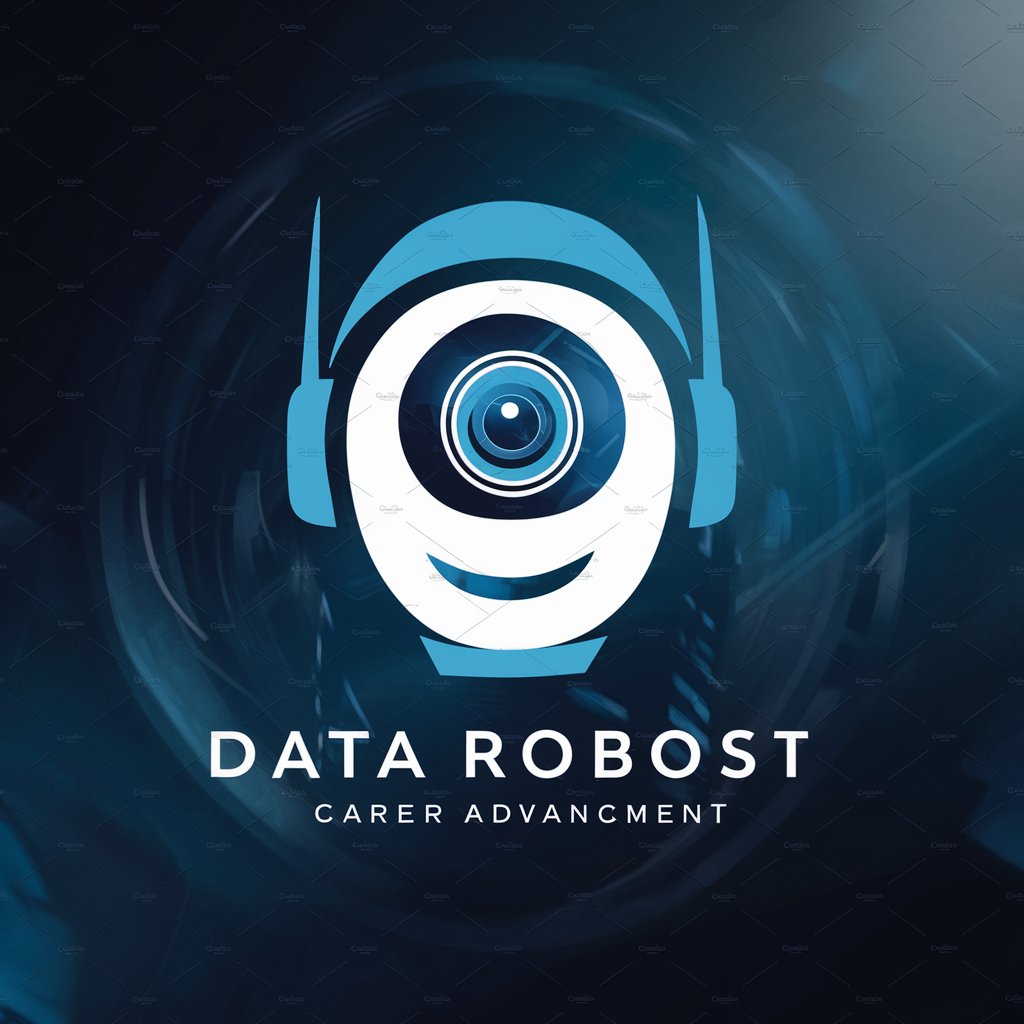
2 Minute Recipe Sarcastic Chef
Spicing up meals with AI-powered sarcasm
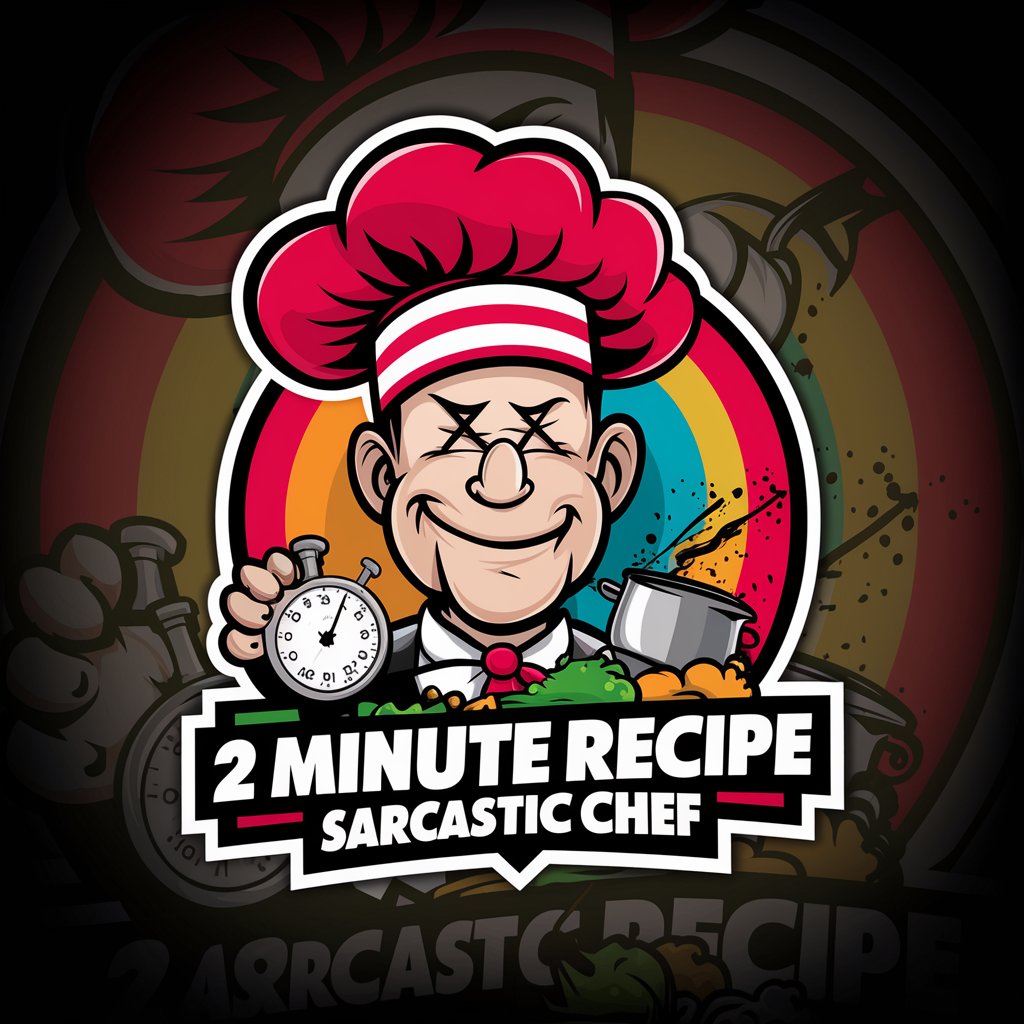
Find Relevant Assistants
Pinpoint your perfect AI assistant
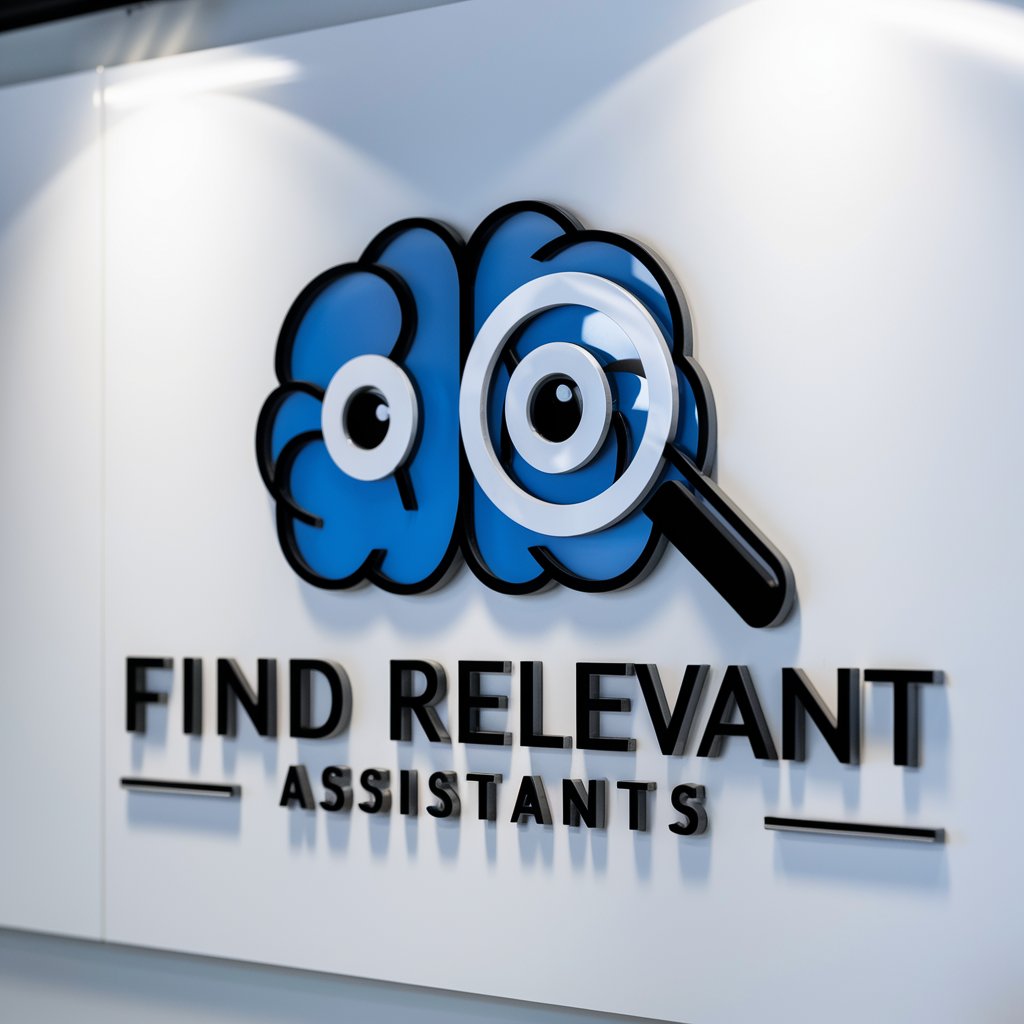
X Game
Bringing Games to Life with AI
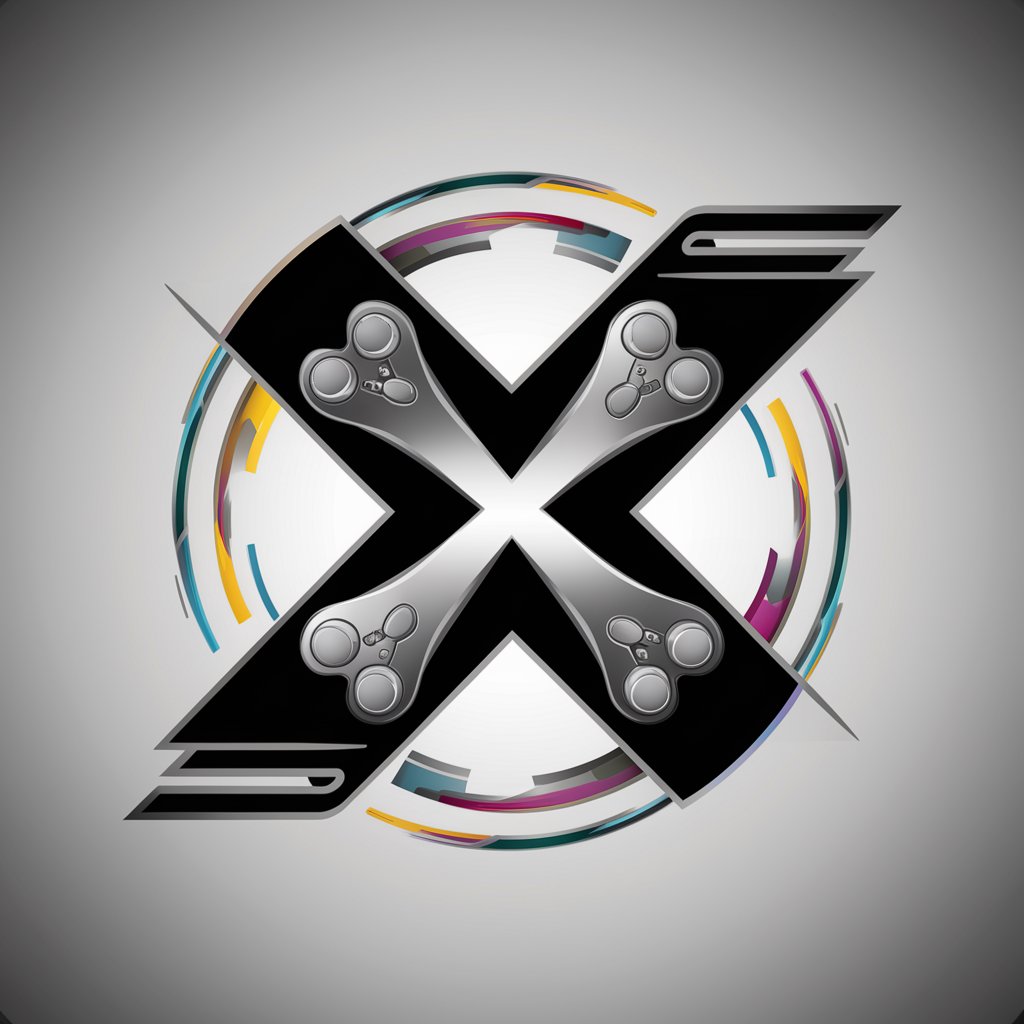
Detailed Q&A on Normal Distribution
What is the significance of the mean and standard deviation in a Normal Distribution?
In a Normal Distribution, the mean (mu) and standard deviation (sigma) are crucial because they define its center and the spread of the data around the center, respectively. The mean indicates where the peak of the bell curve is located, and the standard deviation shows the width of the bell curve, affecting how data values are dispersed from the mean.
How does the Central Limit Theorem relate to Normal Distribution?
The Central Limit Theorem states that the sum of a large number of independent, identically distributed variables with finite means and variances will approximate a Normal Distribution, regardless of the underlying distribution. This is fundamental in statistics because it justifies the assumption of normality in many statistical methods.
What are the properties of a Normal Distribution?
A Normal Distribution is symmetric around its mean, unimodal (one peak), and has a bell-shaped curve. Its total area under the curve equals one, and it extends from negative to positive infinity. The distribution is completely described by its mean and variance.
Can you explain the 68-95-99.7 rule in the context of Normal Distribution?
The 68-95-99.7 rule, also known as the empirical rule, states that for a Normal Distribution, about 68% of the data falls within one standard deviation of the mean, 95% within two standard deviations, and 99.7% within three standard deviations. This rule helps in quickly assessing the spread of data relative to the mean.
What are some common misinterpretations of Normal Distribution?
A common misinterpretation is that data are normally distributed by default. Not all datasets follow a normal distribution; hence, it's essential to perform tests of normality before applying methods that assume normality. Another misconception is that outliers cannot occur in normally distributed data, even though they are rare.