YOLOv5 Lite Helper - AI-powered Object Detection
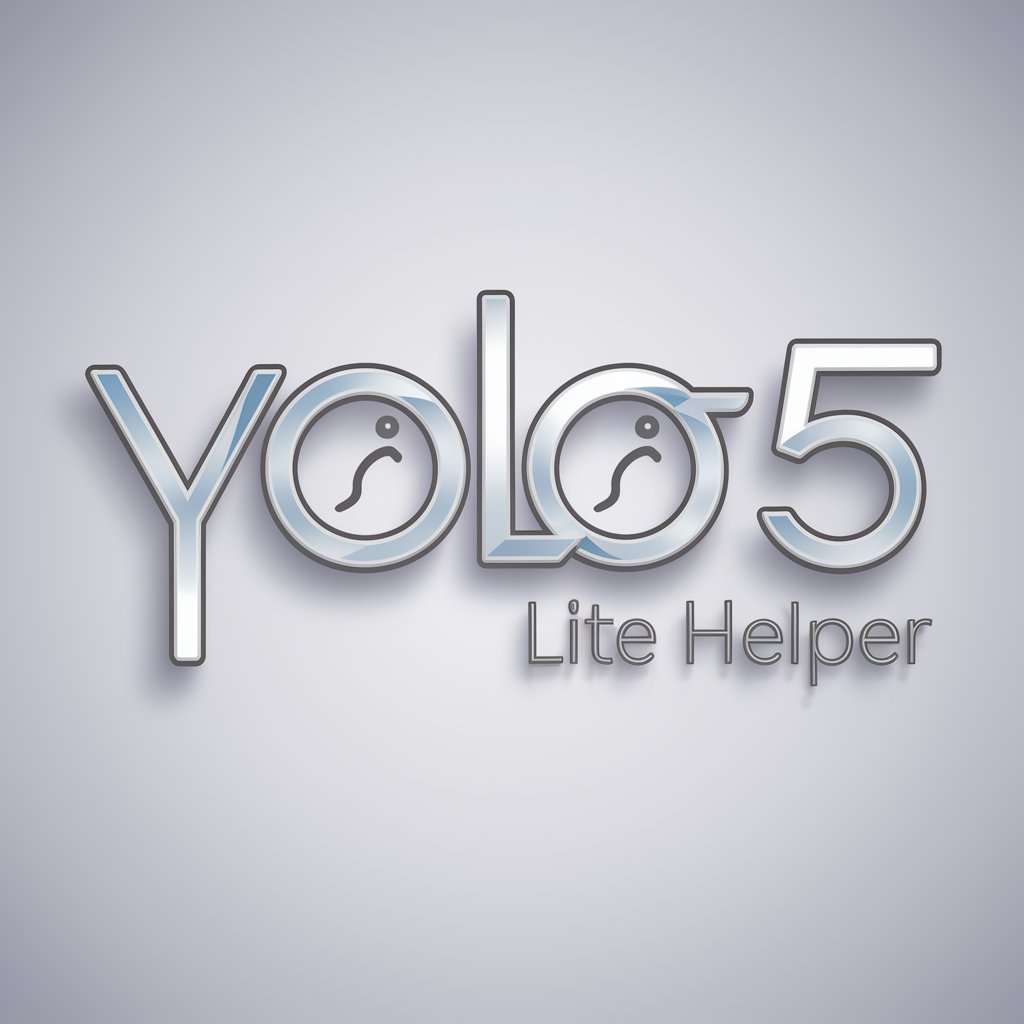
Hi there! Ready to master YOLOv5-lite together?
Revolutionize object detection with AI
Guide me through setting up YOLOv5-lite for the first time.
What are the best practices for training a YOLOv5-lite model?
How can I optimize the performance of my YOLOv5-lite model?
Can you help me troubleshoot an issue with YOLOv5-lite?
Get Embed Code
Overview of YOLOv5 Lite Helper
YOLOv5 Lite Helper is designed to assist users in navigating and utilizing the YOLOv5 Lite model, a streamlined version of the popular YOLOv5 object detection system. This assistant focuses on providing technical guidance, troubleshooting support, and optimization tips for YOLOv5 Lite. It simplifies complex technical information, making it accessible to users with varying levels of expertise. For example, it can guide a novice through the initial setup and configuration of YOLOv5 Lite, while also assisting a more advanced user in fine-tuning the model parameters to improve detection accuracy and speed. Powered by ChatGPT-4o。
Key Functions of YOLOv5 Lite Helper
Setup and Configuration Guidance
Example
Guiding users through the installation of necessary software dependencies, setting up the Python environment, and configuring the YOLOv5 Lite model for initial use.
Scenario
A beginner in computer vision attempts to implement YOLOv5 Lite on their local machine for a project to detect objects in video feeds. The assistant provides step-by-step instructions on how to install Python, PyTorch, and other necessary libraries, and explains how to configure the model for optimal performance.
Model Training and Customization
Example
Assisting users in training YOLOv5 Lite with custom datasets, including data preparation, annotation, and the training process itself.
Scenario
A university research team is developing an application to identify and count wildlife in aerial images. The assistant helps them prepare their dataset, annotate images with bounding boxes, and customize the training parameters to increase the accuracy of the model specifically for small and fast-moving objects.
Performance Optimization
Example
Providing strategies for model optimization to achieve better performance in terms of speed and accuracy, including hardware choices and inference tweaks.
Scenario
A tech startup wants to deploy YOLOv5 Lite in a real-time surveillance system. The assistant advises on optimizing the model to run efficiently on edge devices with limited computing power, such as adjusting the model size, simplifying the network, and selecting the right inference engine.
Target User Groups for YOLOv5 Lite Helper
Developers and Engineers
This group includes software developers and engineers who need to integrate object detection into applications. They benefit from the assistant’s ability to simplify the setup, explain model tuning, and optimize performance for specific applications.
Academics and Researchers
Students, professors, and research scientists who are exploring computer vision technologies for academic projects or innovative research can utilize YOLOv5 Lite Helper to better understand the nuances of model training and data handling, which are crucial for their experimental work.
Technology Enthusiasts
Hobbyists and technology enthusiasts interested in AI and machine learning projects, such as DIY home automation or personal tech projects involving object detection, find this assistant particularly useful for learning and applying YOLOv5 Lite in practical, real-world scenarios.
How to Use YOLOv5 Lite Helper
Start a Free Trial
Visit yeschat.ai to start using YOLOv5 Lite Helper without needing to log in or subscribe to ChatGPT Plus.
Prepare Your Data
Collect and label a dataset appropriate for your specific object detection needs. Ensure images are clear and labels are accurate.
Configure the Model
Adjust the configuration files to match your dataset specifics. Set parameters like batch size, epochs, and learning rate for optimal training.
Train the Model
Use the custom training scripts provided by YOLOv5 Lite to train the model on your dataset. Monitor performance metrics like loss and accuracy.
Deploy and Evaluate
After training, deploy the model to your application or device. Perform real-world tests and refine the model as needed based on performance feedback.
Try other advanced and practical GPTs
GeoML Navigator
Transforming imagery into insights.
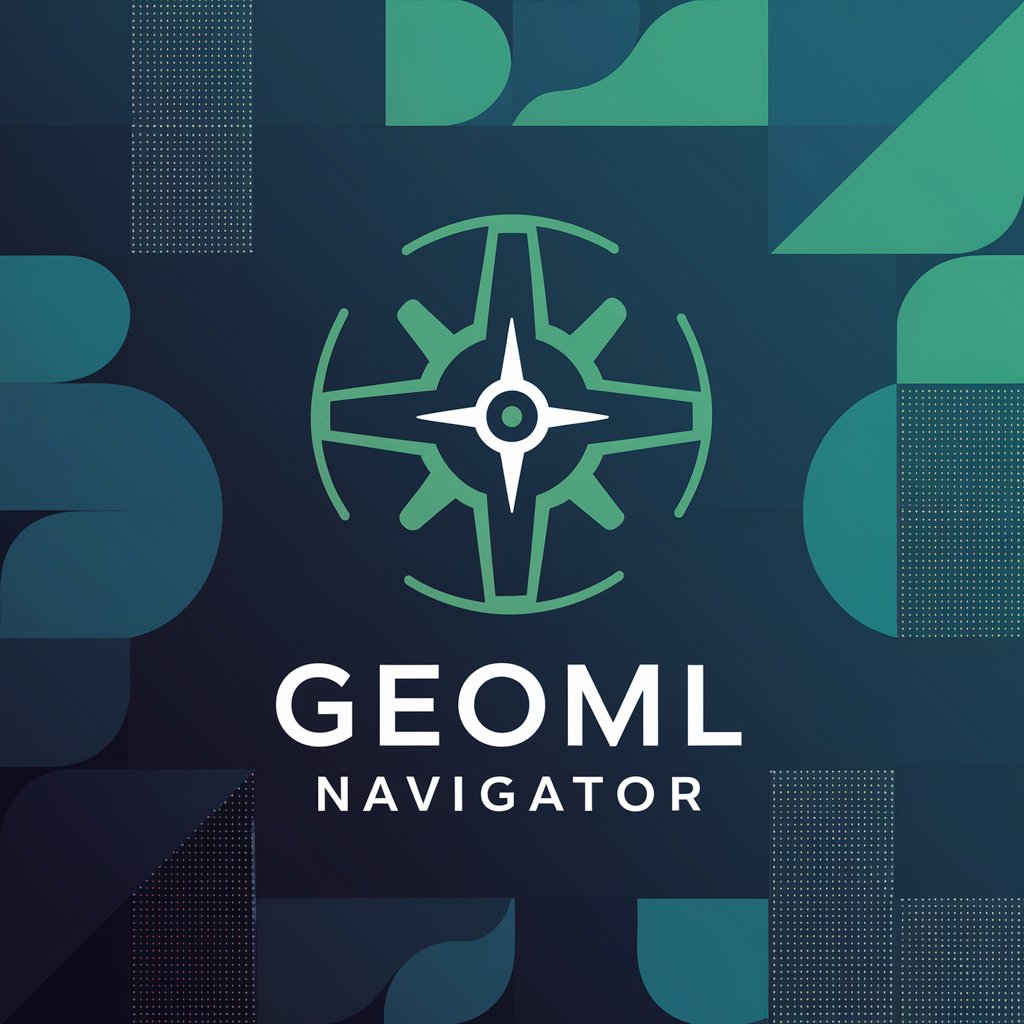
计算机视觉专家 - Dr. 艾米丽
Empowering Vision with AI Expertise
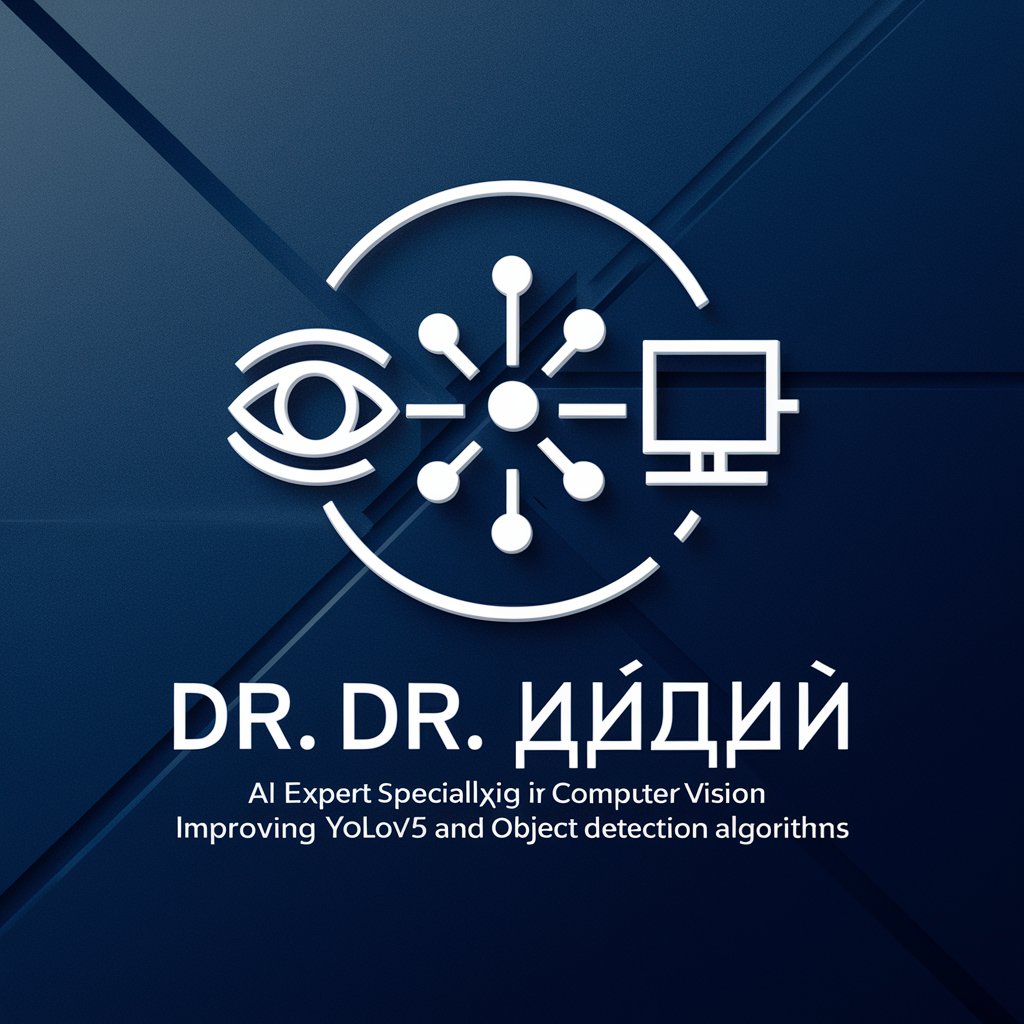
FRC Visionary Guide
Empowering vision with AI
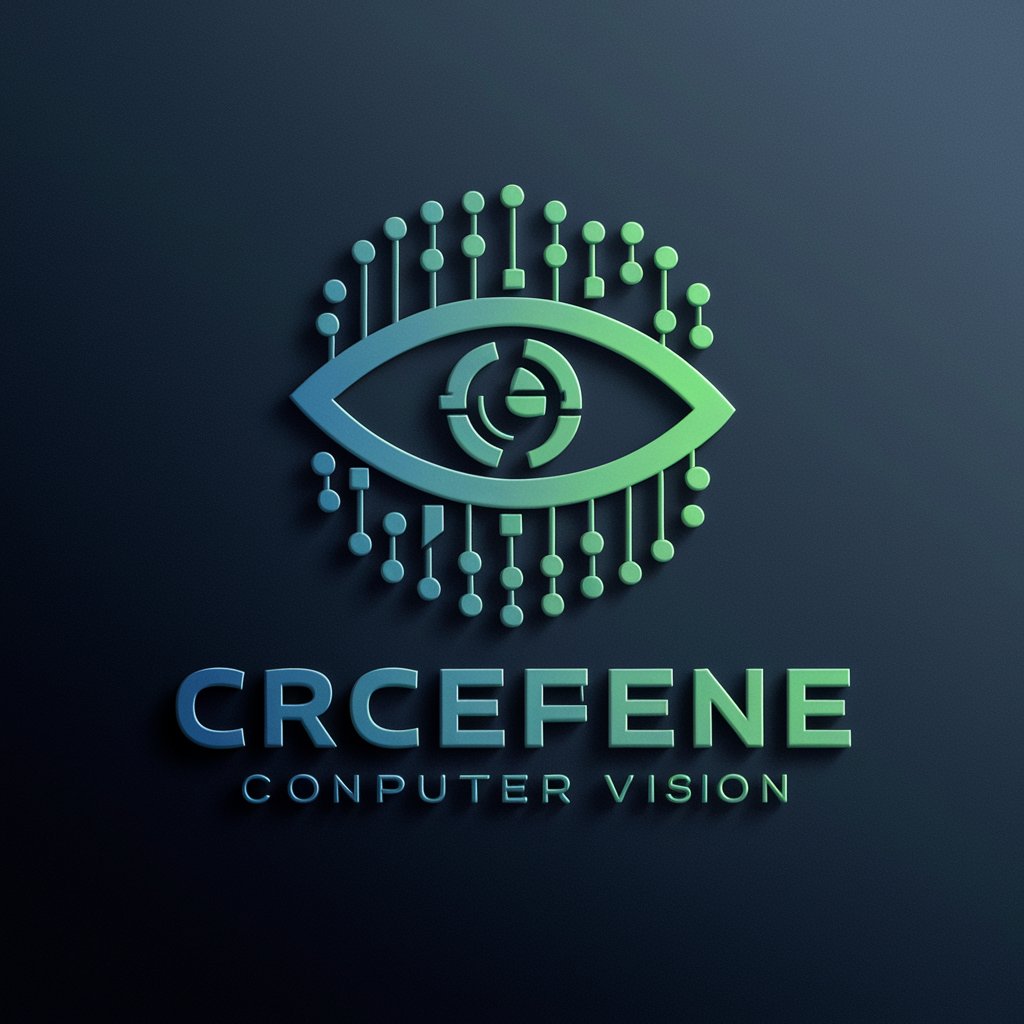
YOLOv5目标检测技术专家
Empowering Vision with AI
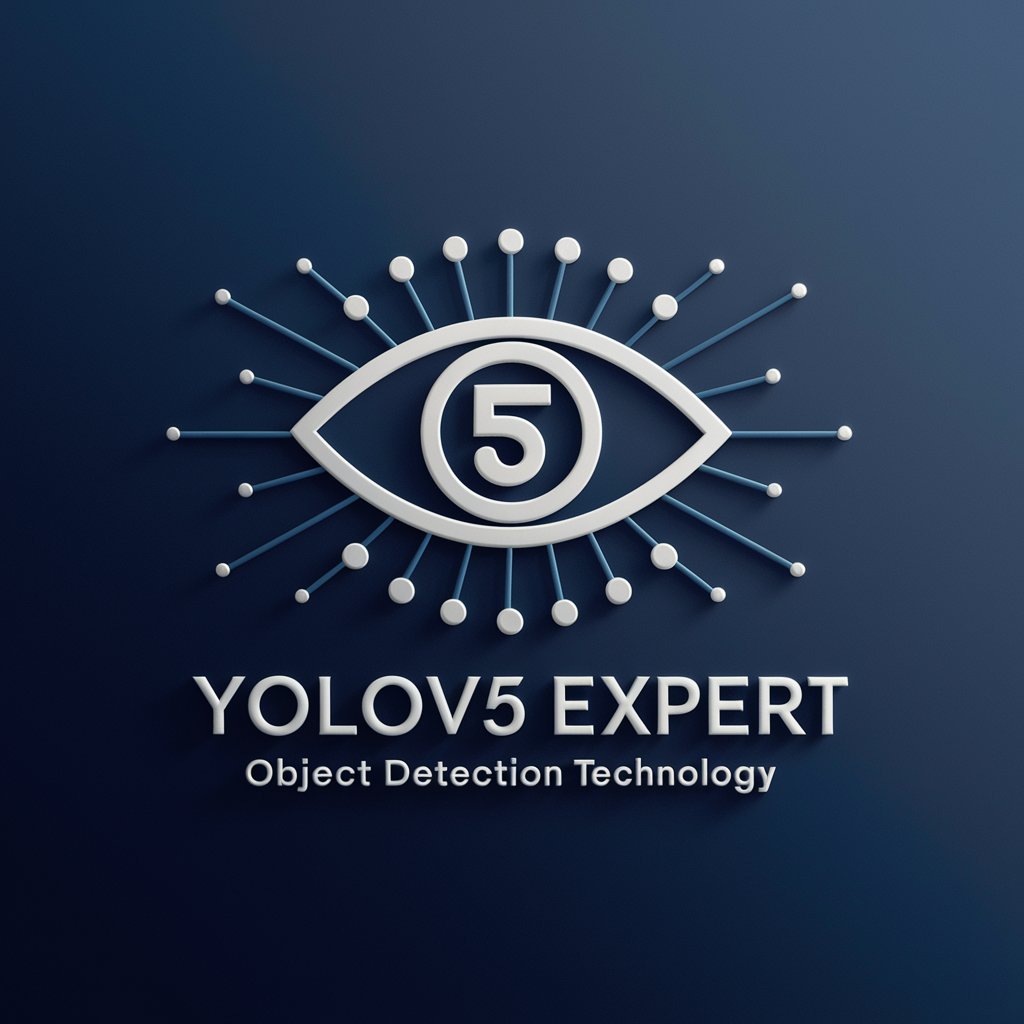
YOLOv8 Guide
Enhancing AI-powered object detection
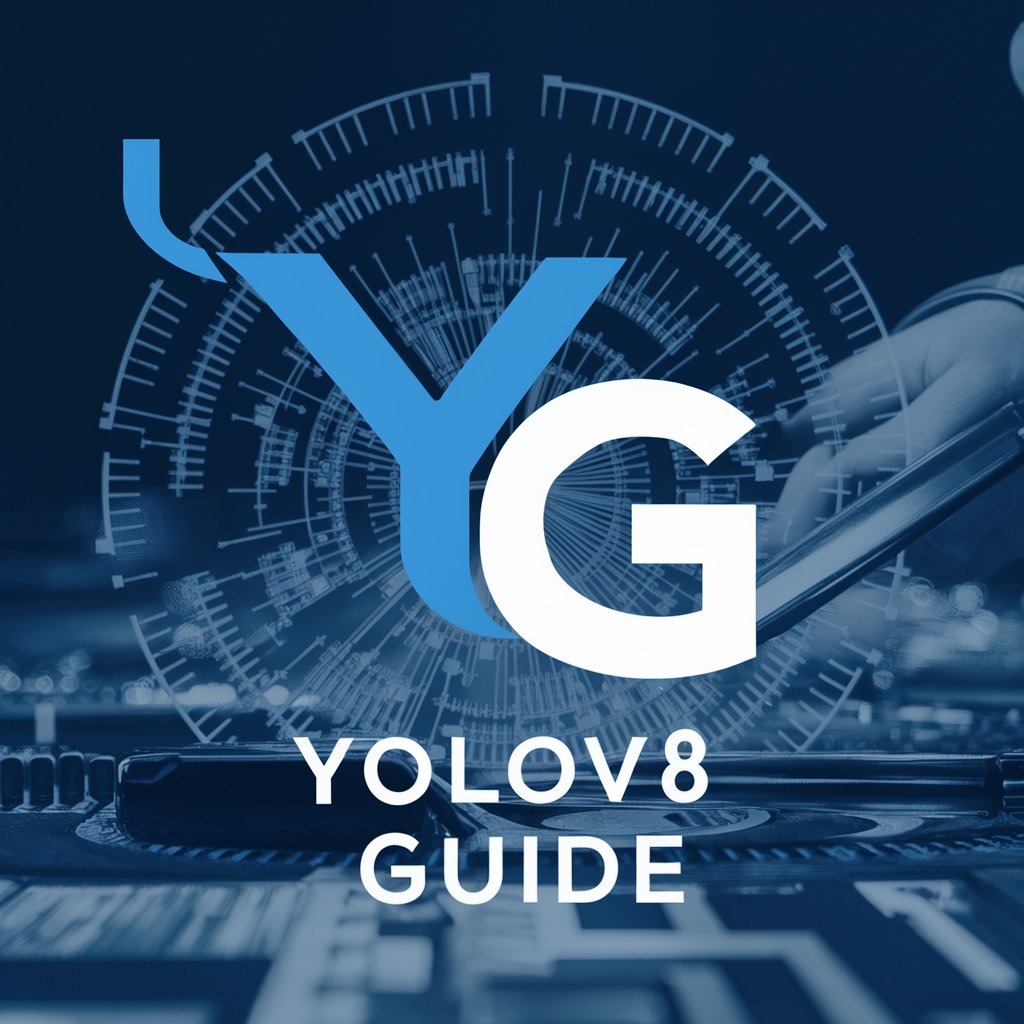
Liferay GPT - Best GPT for liferay
Elevating Liferay with AI Power
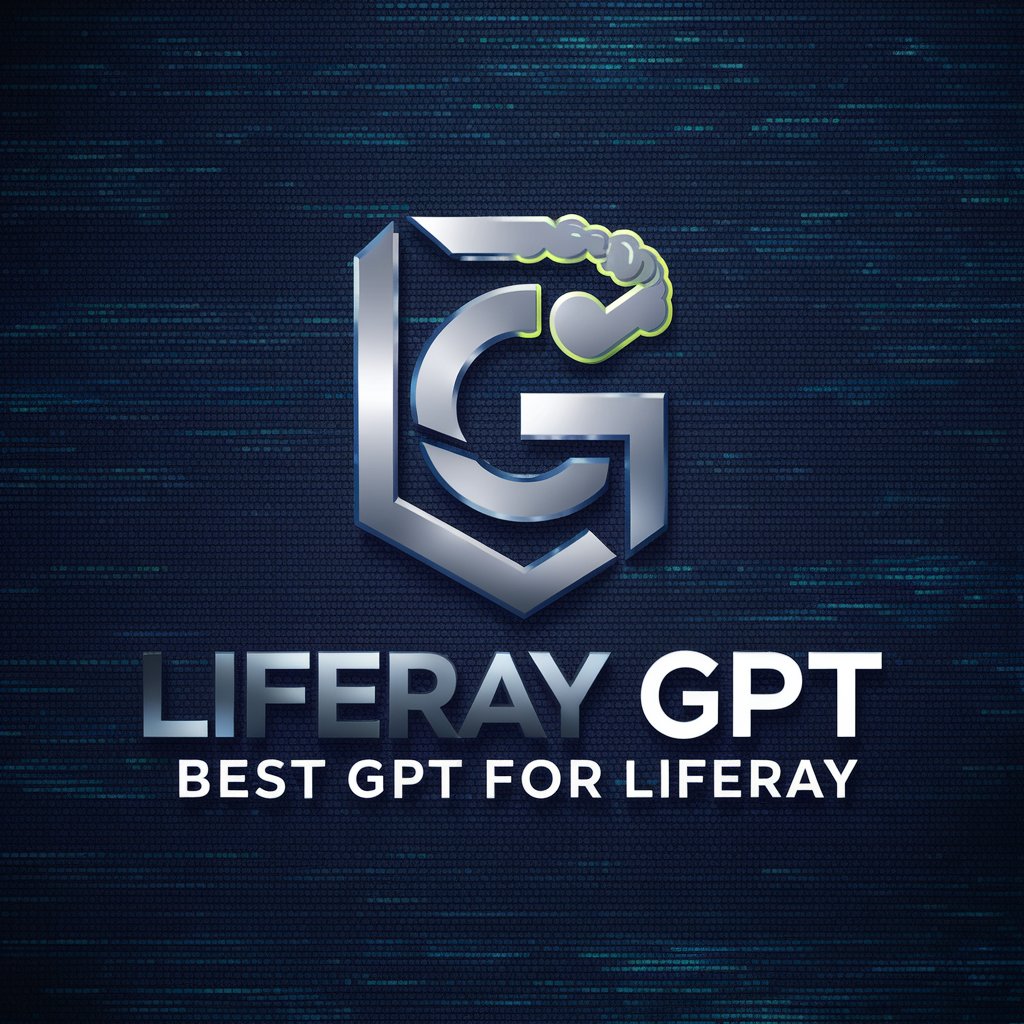
YOLOv8 Expert
Empowering AI-driven object detection
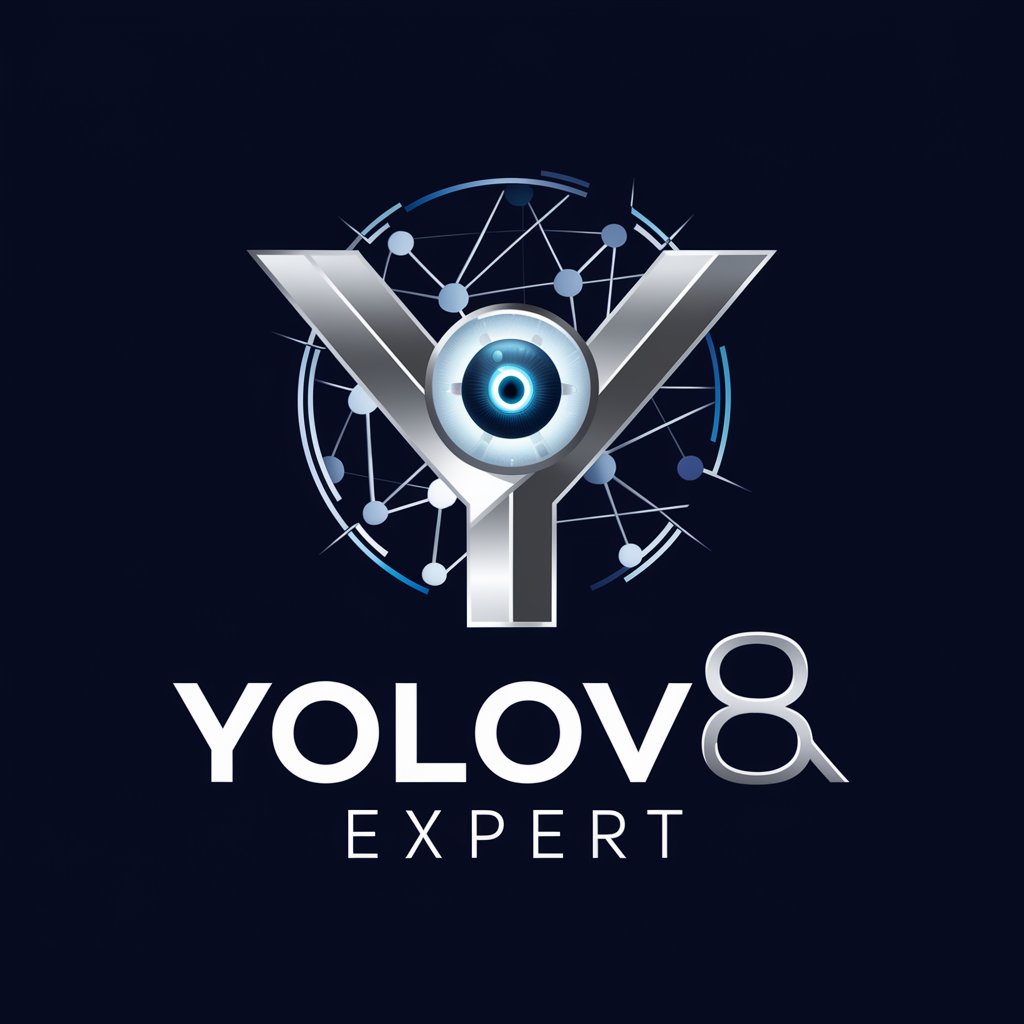
YOLOv5 Expert
Empowering object detection with AI
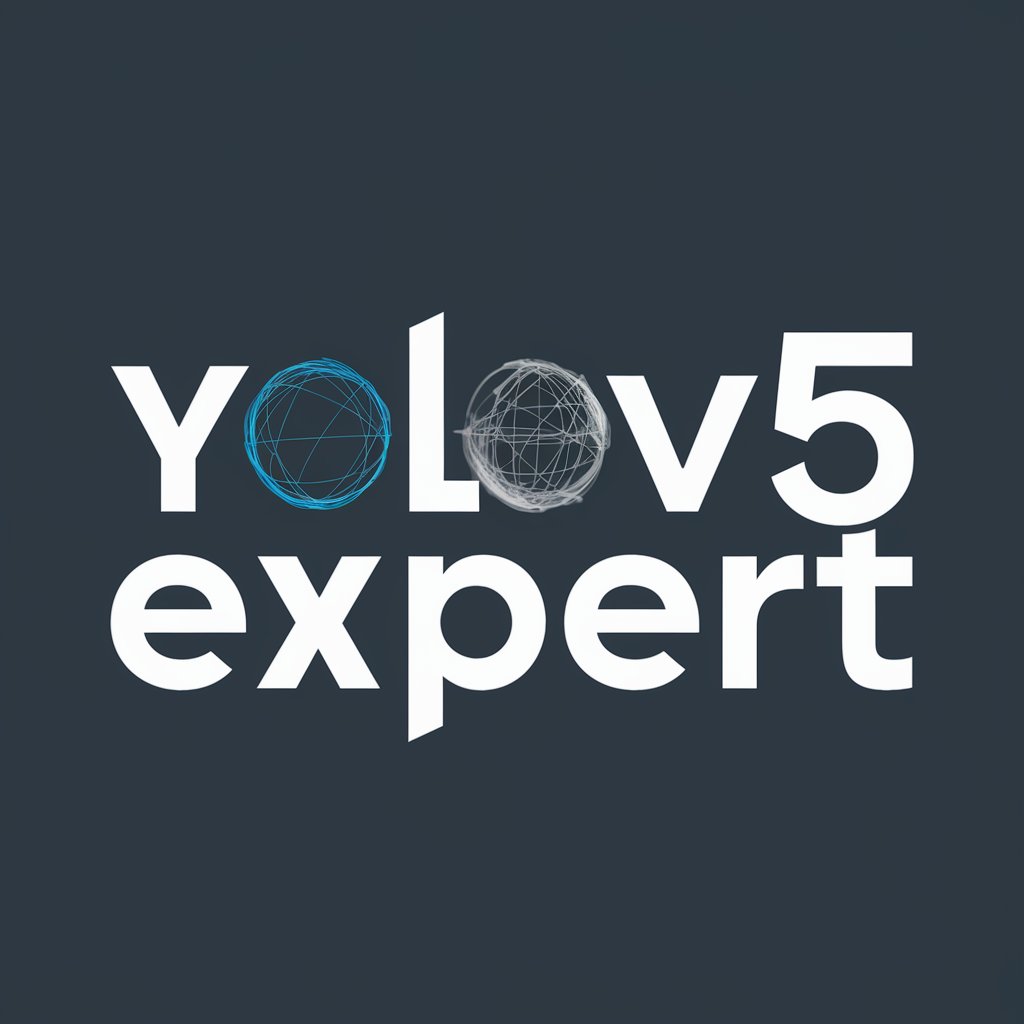
YOLOv8
Empower Vision with AI
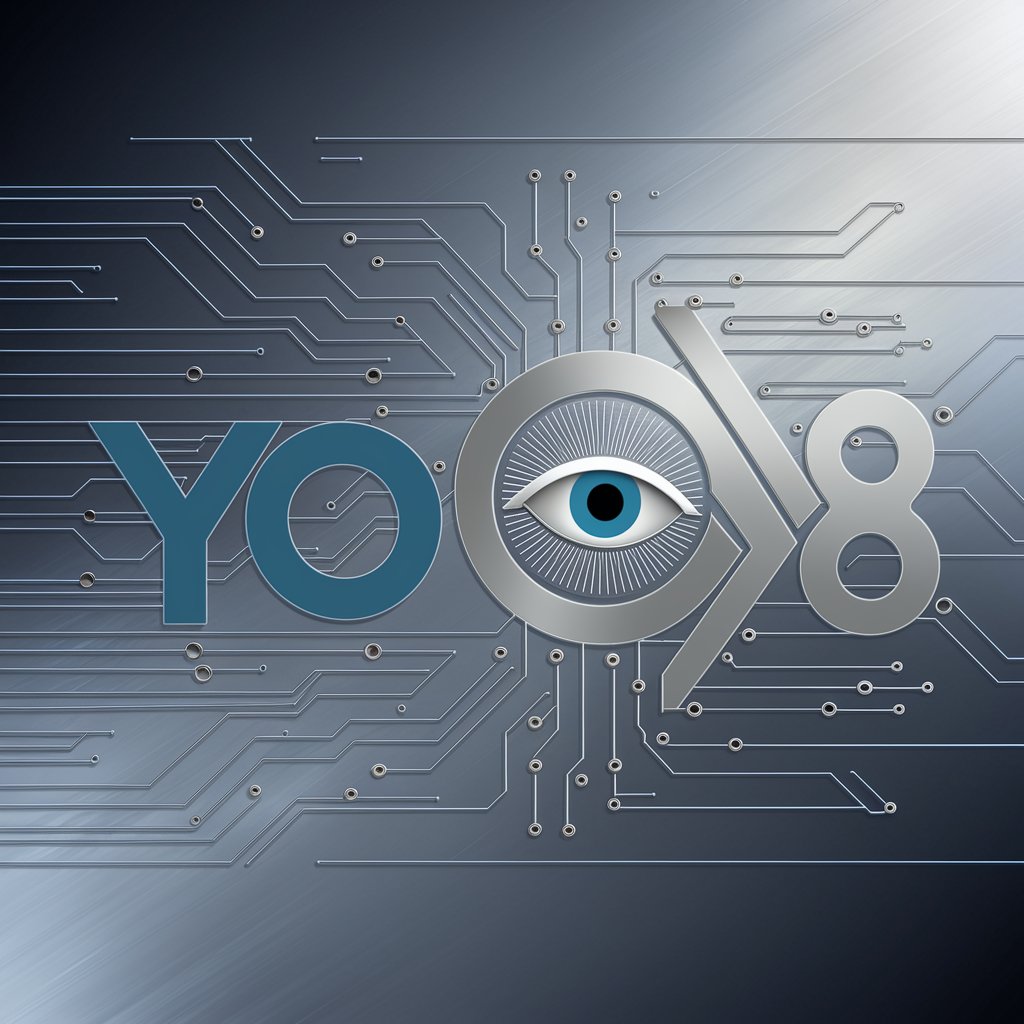
Matlab Maestro
Elevate Your Matlab Skills with AI
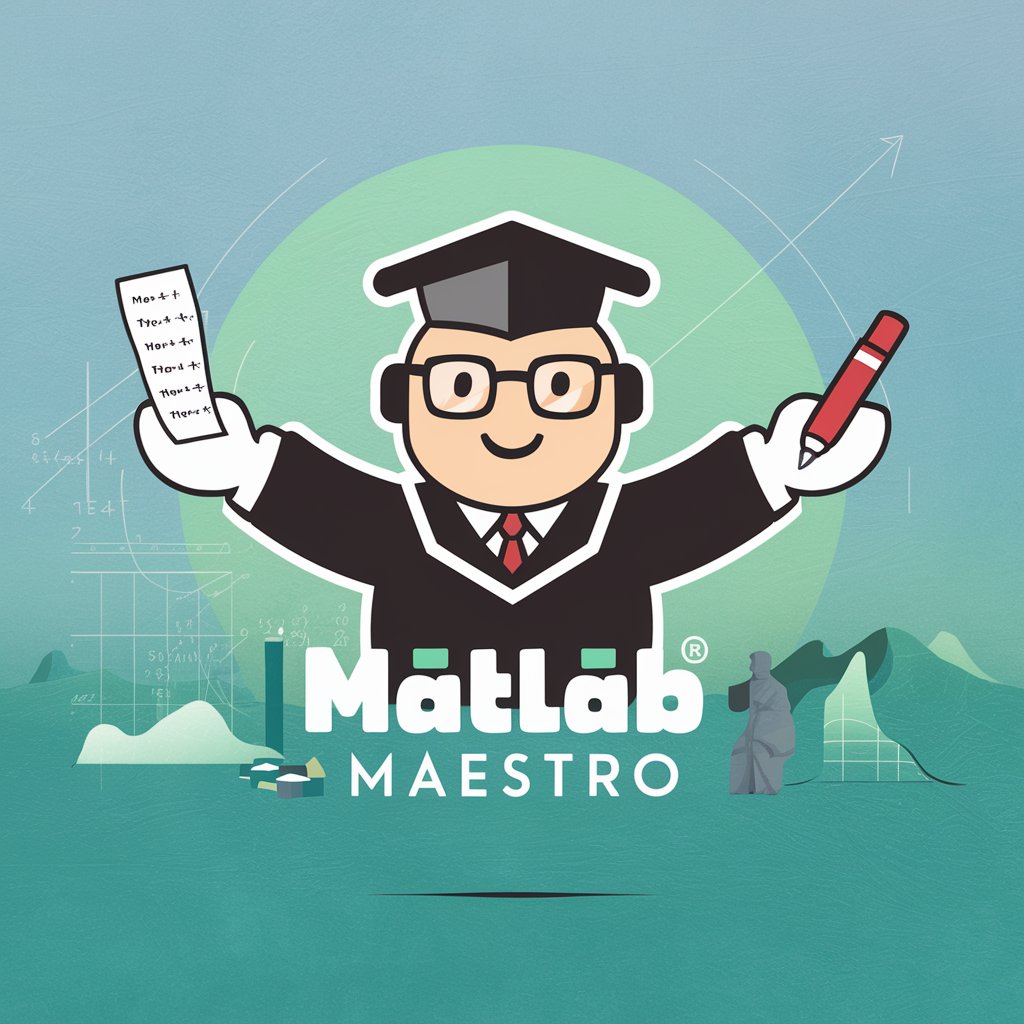
Matlab Mentor
Empowering your MATLAB Image Mastery
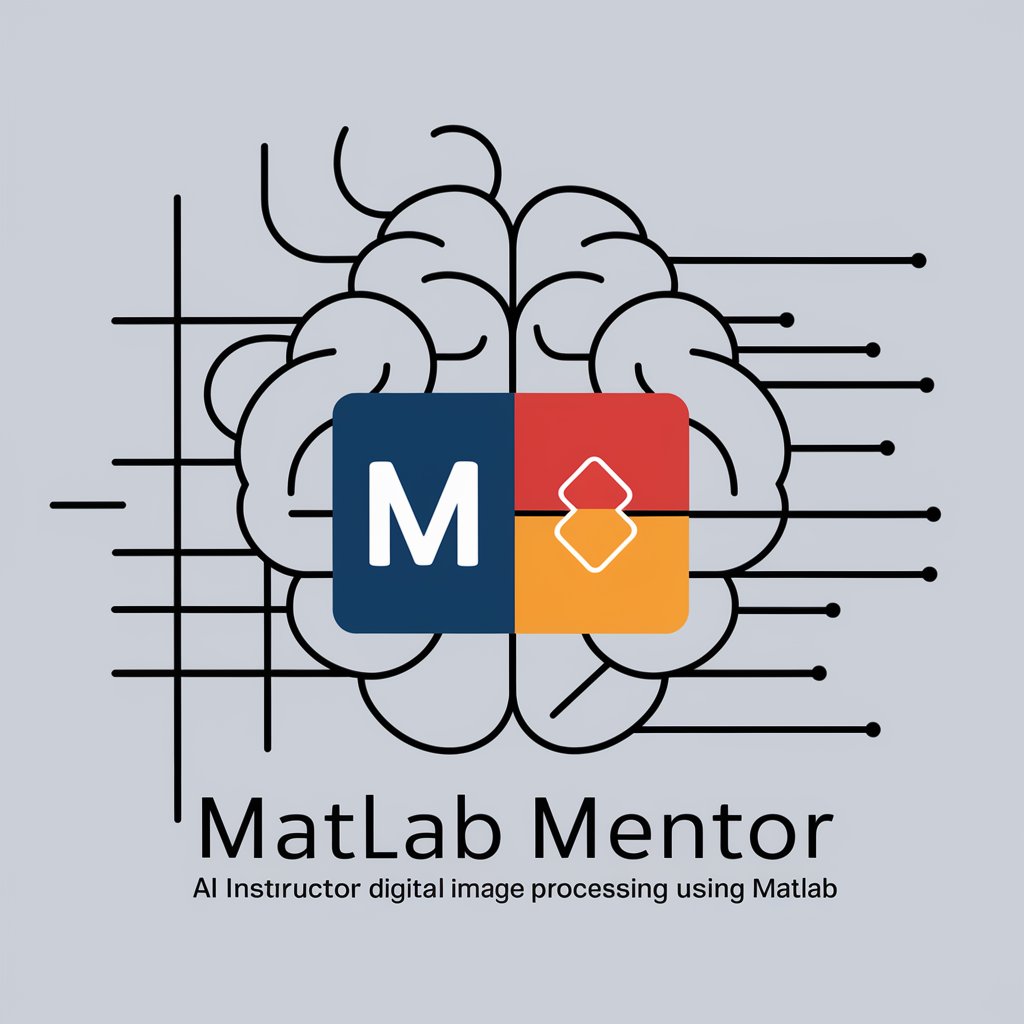
MATLAB Maven
Powering Electronics with AI
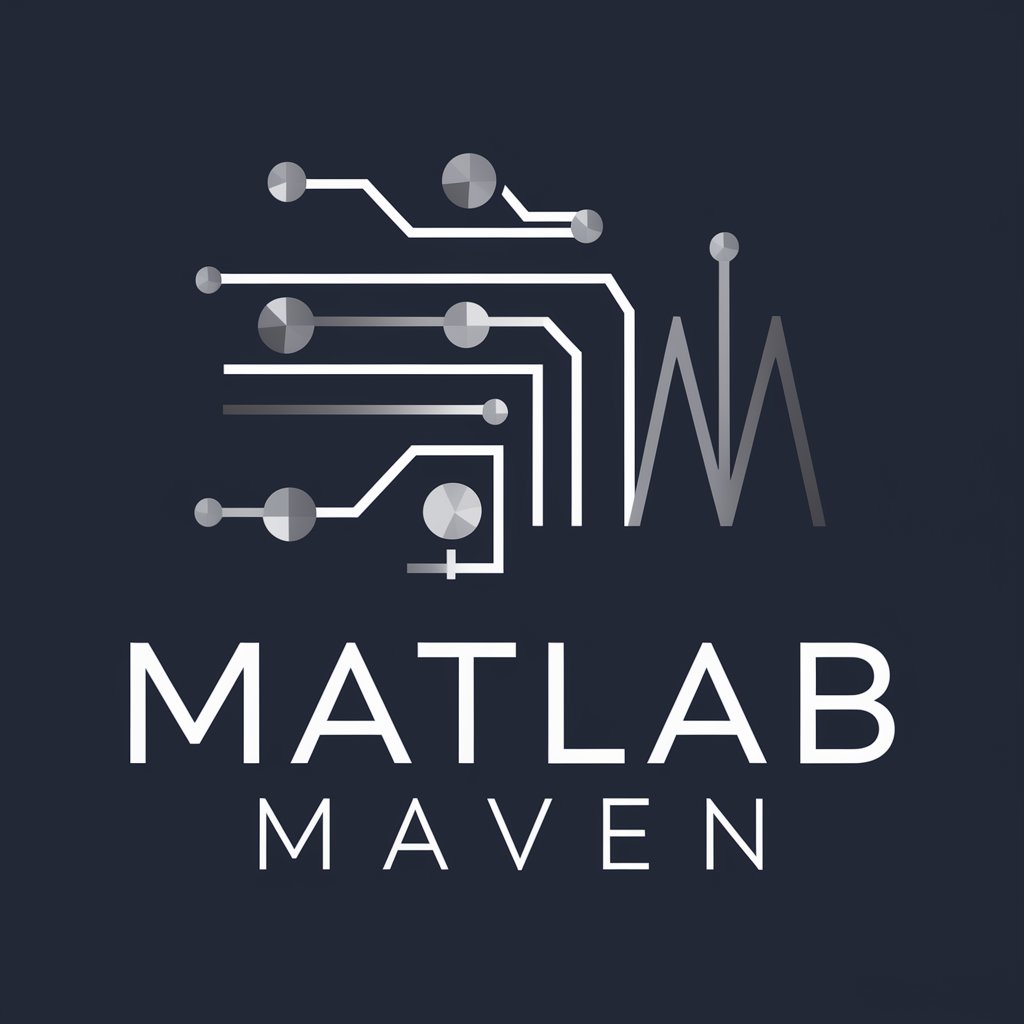
Frequently Asked Questions About YOLOv5 Lite Helper
What are the hardware requirements for training YOLOv5 Lite?
YOLOv5 Lite can be trained on most modern GPUs with at least 4GB of VRAM. For optimal performance, a CUDA-enabled GPU is recommended to accelerate training.
Can YOLOv5 Lite be used for real-time object detection?
Yes, YOLOv5 Lite is designed for efficiency and can be deployed on devices for real-time object detection, making it suitable for applications like surveillance and autonomous driving.
How do I improve the accuracy of YOLOv5 Lite?
To improve accuracy, focus on high-quality, well-labeled training data, augment the dataset, fine-tune hyperparameters, and possibly use transfer learning from a pre-trained model.
Is YOLOv5 Lite suitable for small-scale projects?
Absolutely, its lightweight architecture makes it ideal for small-scale projects and devices with limited computational resources.
What types of objects can YOLOv5 Lite detect?
YOLOv5 Lite can be trained to detect any type of object provided it has been trained with appropriate labeled images of those objects, from common items like vehicles and pedestrians to specific categories like industrial components.