FineTune Helper - AI Fine-Tuning Assistance
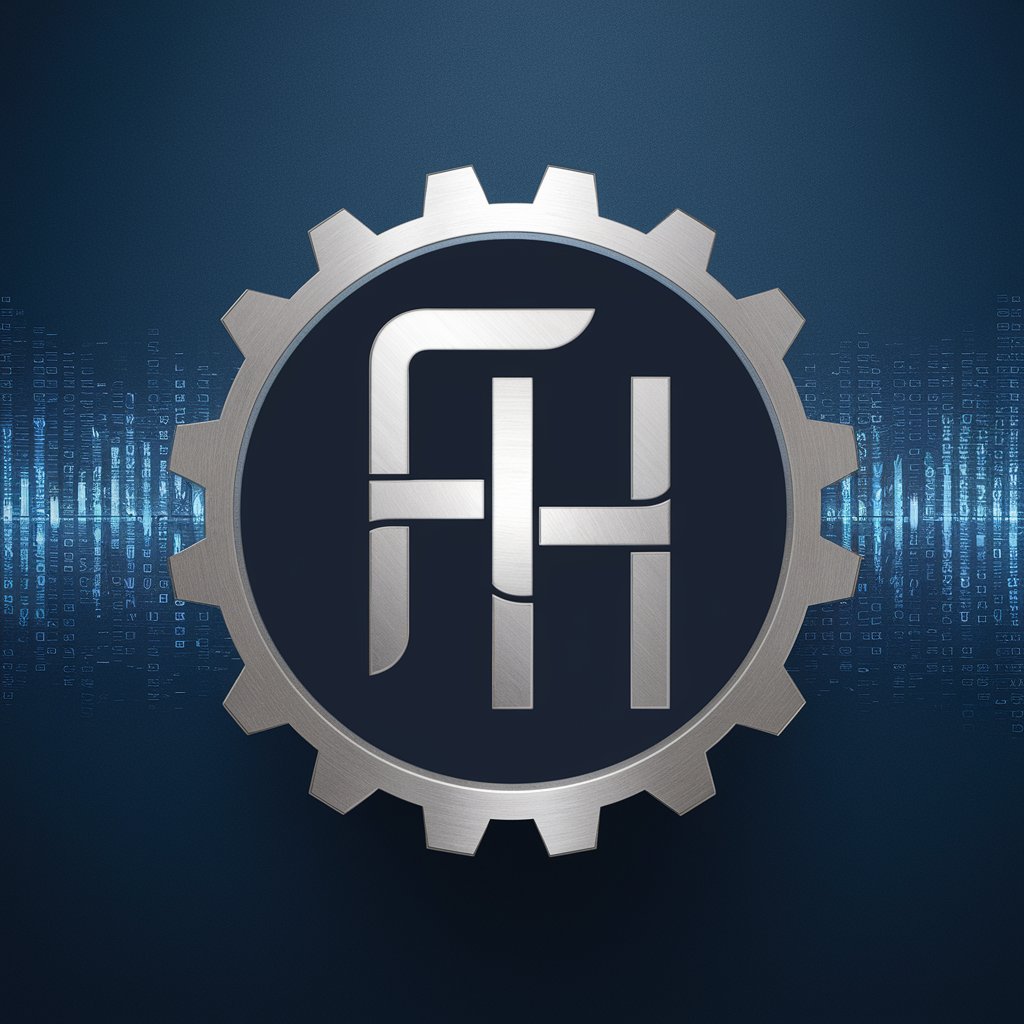
Welcome to FineTune Helper, your guide to mastering AI fine-tuning!
Customize AI with Precision
Explain how fine-tuning can improve the performance of AI models.
Describe the benefits of using OpenAI's fine-tuning API.
What are the steps involved in preparing data for fine-tuning?
How does fine-tuning reduce costs and latency for AI applications?
Get Embed Code
Overview of FineTune Helper
FineTune Helper is a specialized assistant designed to help users optimize the fine-tuning of OpenAI's language models. It is particularly focused on integrating and leveraging large datasets or specific web service documentation provided by users for fine-tuning OpenAI models. This specialized application allows users to better understand and execute the fine-tuning process, including preparing data, executing training jobs, and deploying fine-tuned models. For instance, a scenario where FineTune Helper can be utilized is in helping a user create and refine training datasets that adhere to the specific requirements of the OpenAI fine-tuning API, ensuring the data format and token counts are correct before submission. Powered by ChatGPT-4o。
Core Functions of FineTune Helper
Data Preparation Guidance
Example
Providing detailed steps on how to prepare and validate training datasets for fine-tuning.
Scenario
A user preparing to fine-tune a model for handling customer service requests can use FineTune Helper to ensure their conversation datasets are formatted correctly and the token count is within the allowed limit.
Fine-Tuning Process Management
Example
Assisting in the setup, monitoring, and adjustment of fine-tuning jobs using the OpenAI API.
Scenario
FineTune Helper guides a software developer through the process of starting a fine-tuning job in the OpenAI API, monitoring the job’s progress, and making adjustments based on preliminary results to optimize performance.
Deployment and Integration Advice
Example
Providing recommendations on how to deploy and integrate fine-tuned models into existing applications.
Scenario
After fine-tuning a model to generate technical documentation, FineTune Helper can advise on best practices for integrating this model into a software company’s documentation pipeline.
Target User Groups for FineTune Helper
AI Researchers and Developers
This group includes individuals and teams in academic or corporate settings who are actively involved in developing, fine-tuning, and deploying AI models tailored for specific tasks. They benefit from FineTune Helper by gaining insights into optimizing model performance and ensuring their models accurately reflect the nuances of their training data.
Technical Product Managers
Product managers overseeing AI-driven products require accurate, high-performing models. FineTune Helper can assist them in understanding the technical requirements and best practices of fine-tuning, helping to bridge the gap between developers and business requirements.
How to Use FineTune Helper
Step 1
Visit yeschat.ai for a free trial without login, also no need for ChatGPT Plus.
Step 2
Upload your specific documentation or training data using the provided interface to tailor the model to your needs.
Step 3
Define the parameters for fine-tuning, such as the number of training examples and the desired model behavior.
Step 4
Initiate the fine-tuning process and monitor the progress through the provided analytics dashboard.
Step 5
Once fine-tuning is complete, test the model's performance with your specific tasks to ensure it meets your requirements.
Try other advanced and practical GPTs
Proofread Queen
AI-Powered Feedback and Refinement
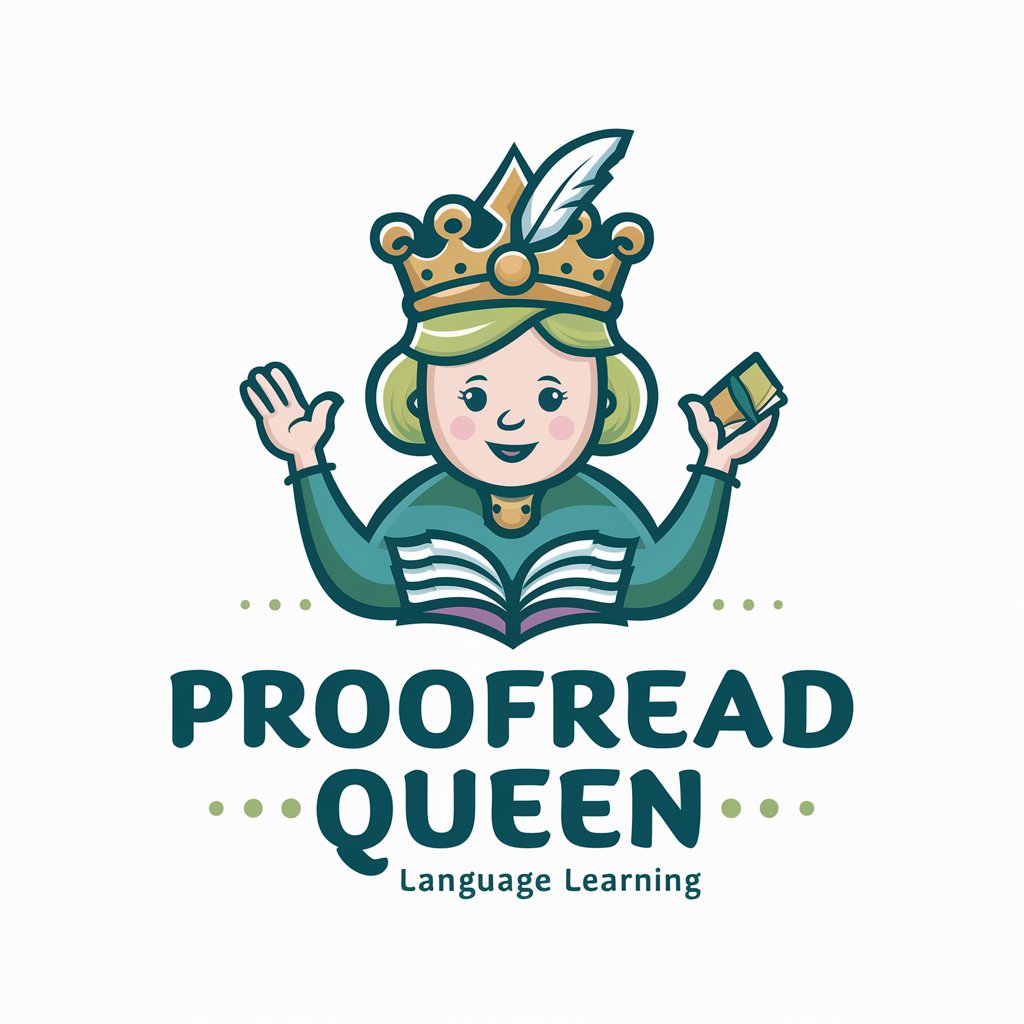
English Test Maestro
Ace Your English Tests with AI
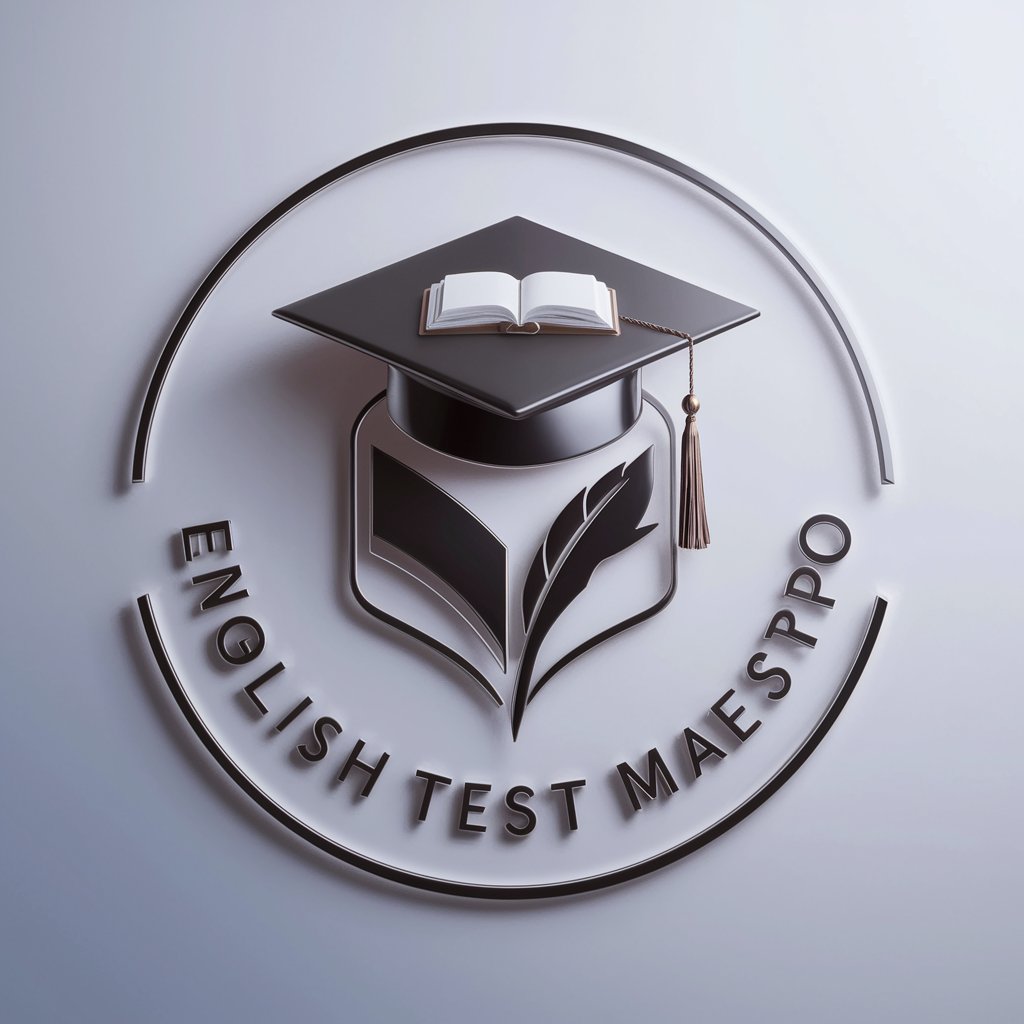
Godot 4 Copilot
AI-powered assistant for Godot 4 developers
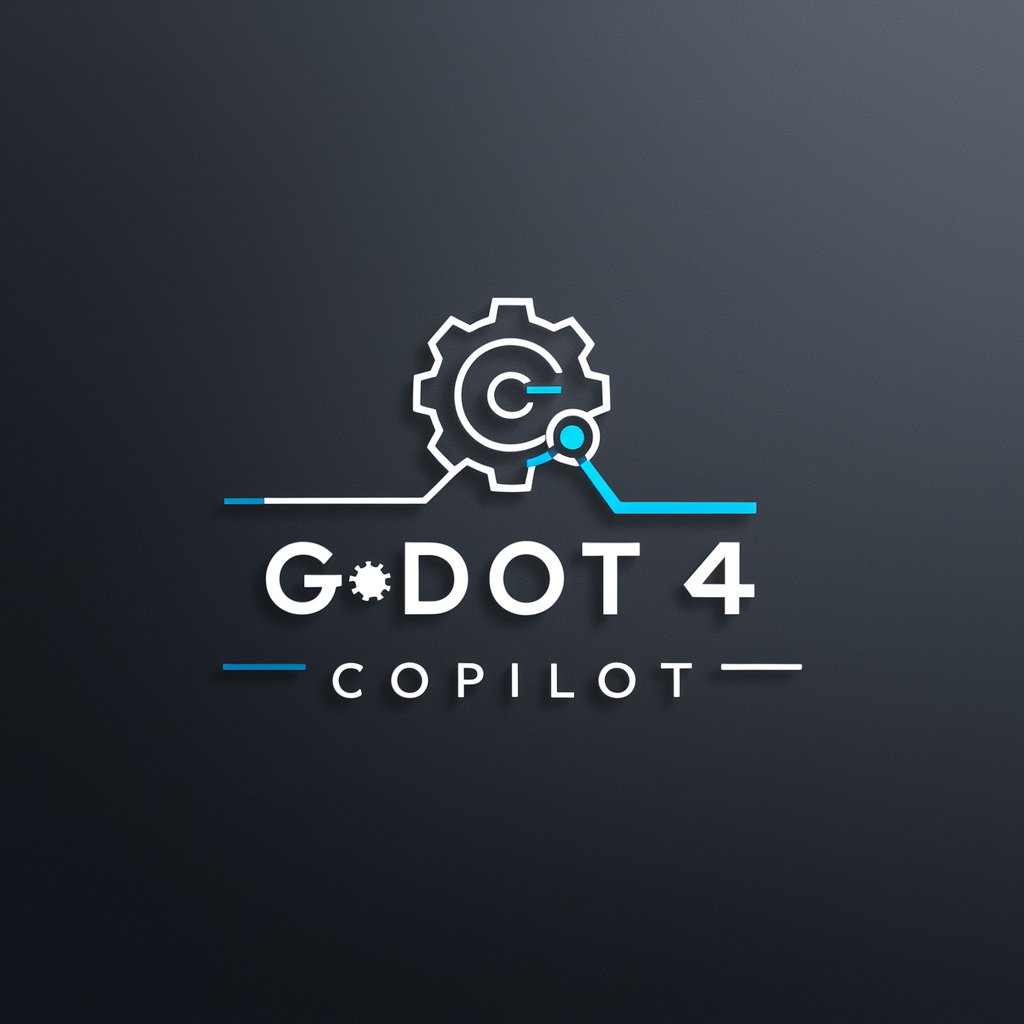
Law Precision
AI-powered Precise Legal Assistant
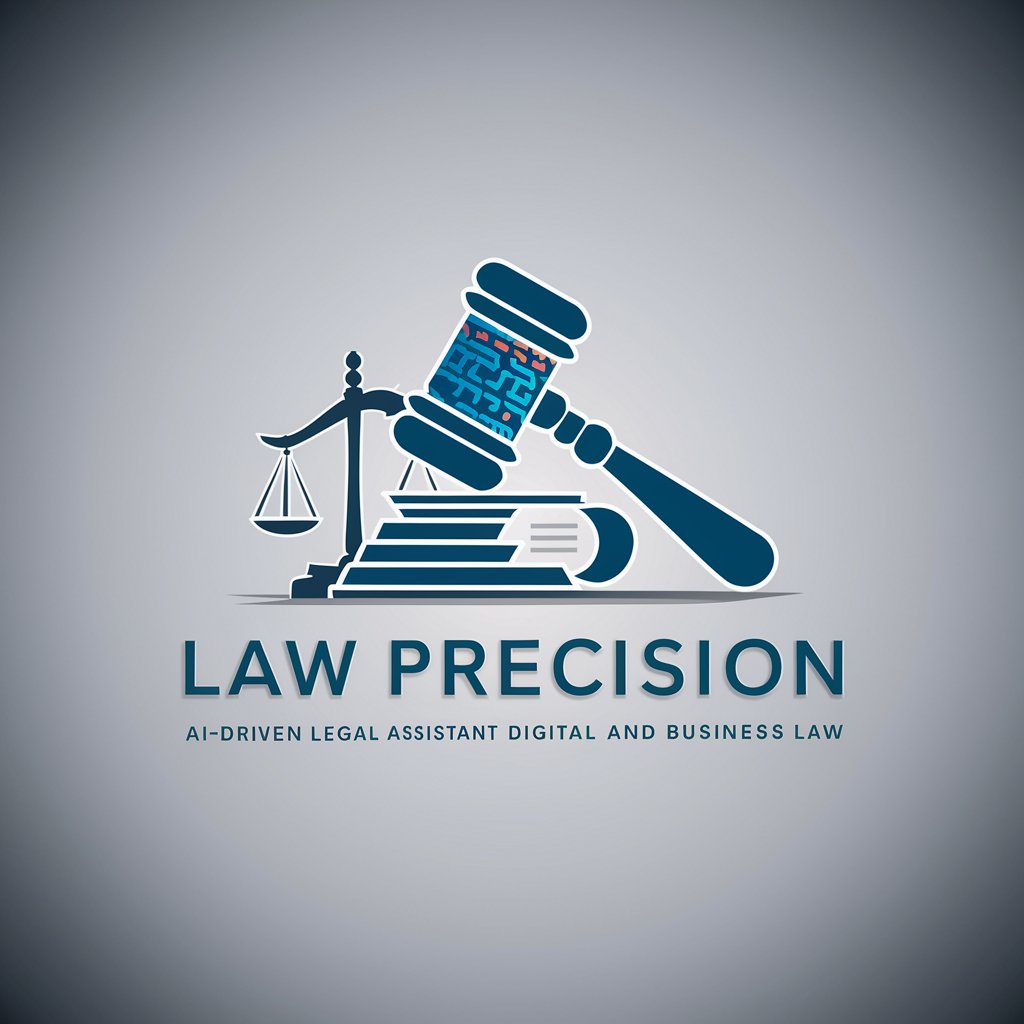
Promptie The Prompt Pro
Engineering Your Ideas with AI
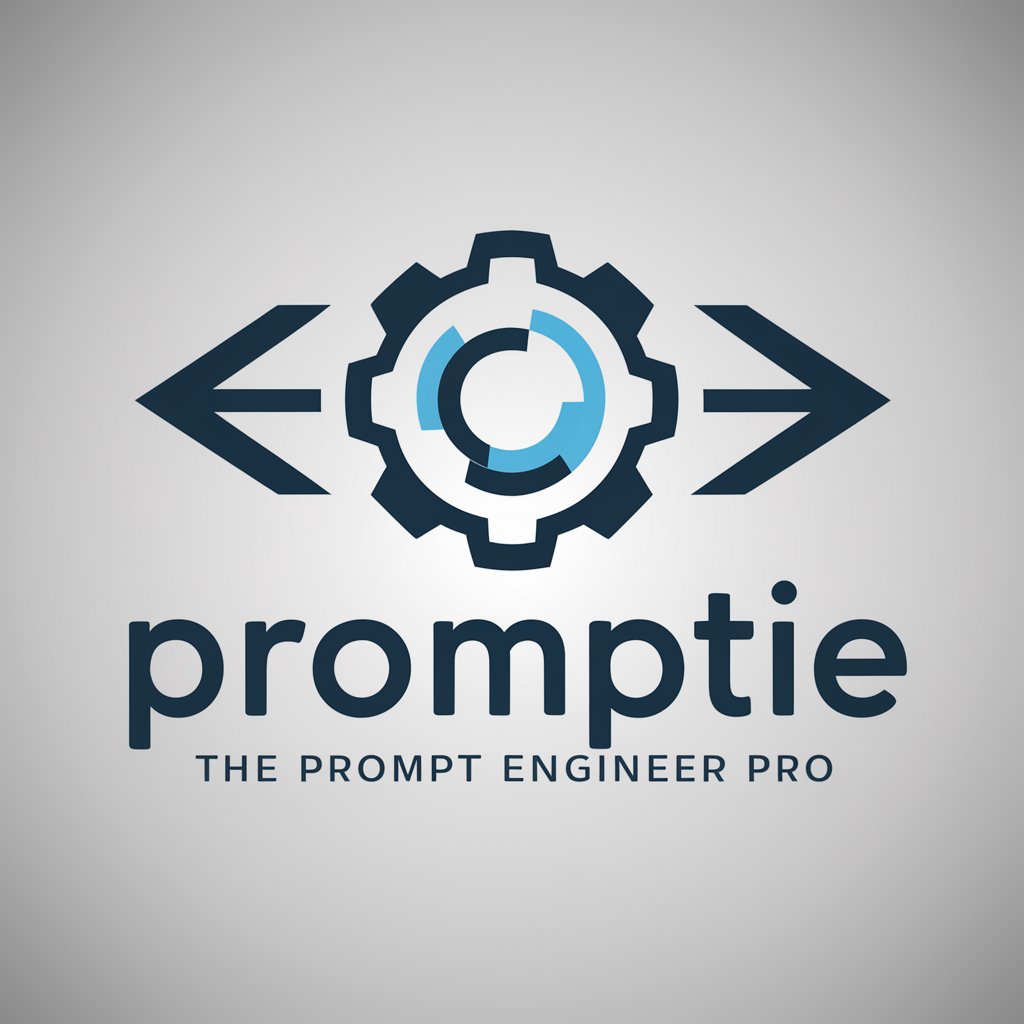
SMLE Destroyer
AI-Powered Medical Exam Success.
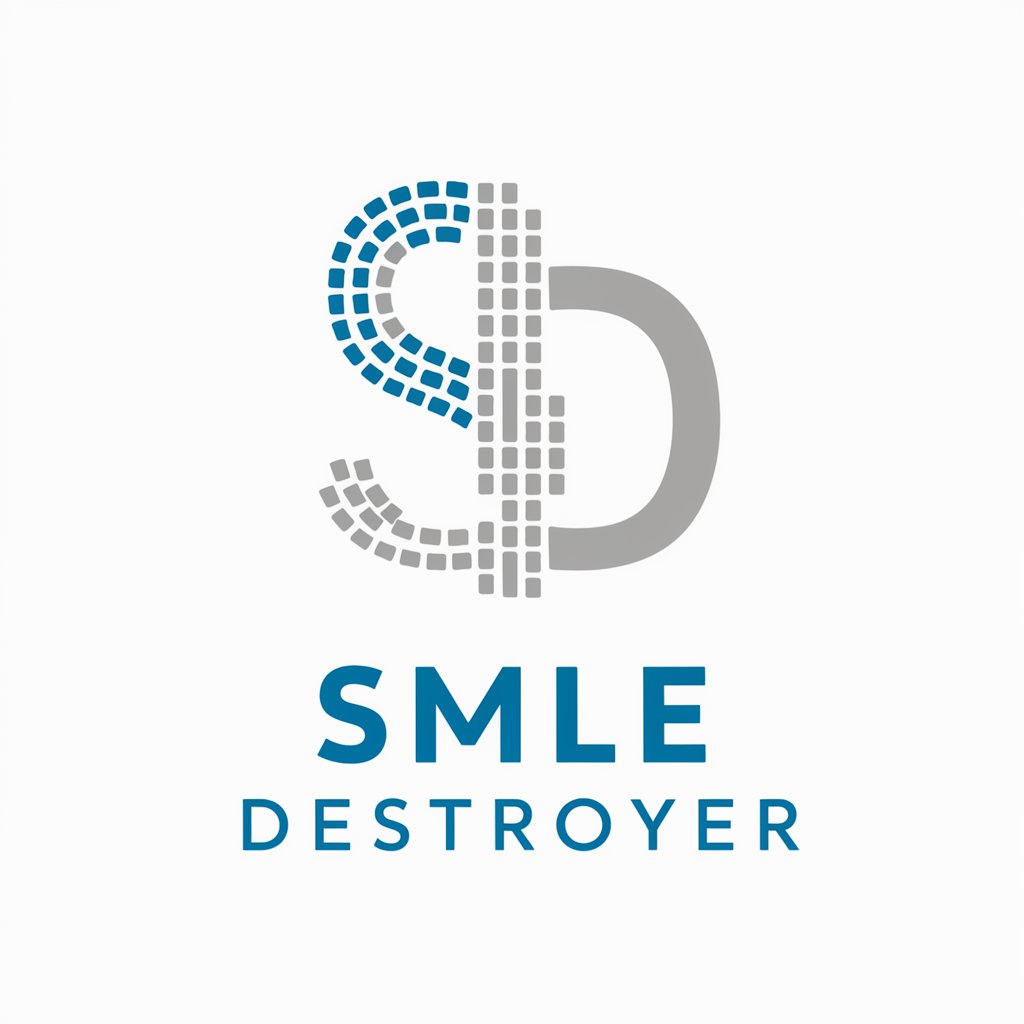
Crypto Research Detective
Deciphering Blockchain with AI
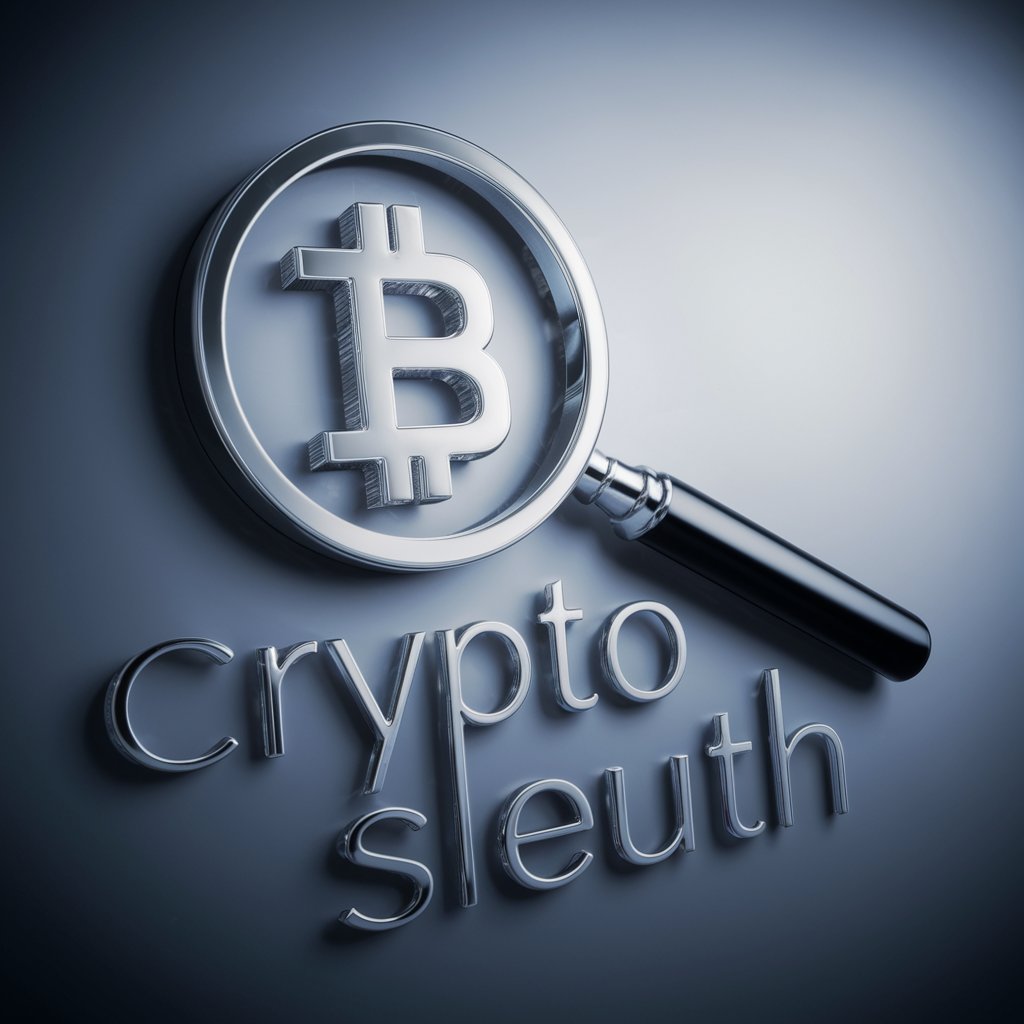
Fantasy Creature Creator
Craft Creatures, Unleash Creativity
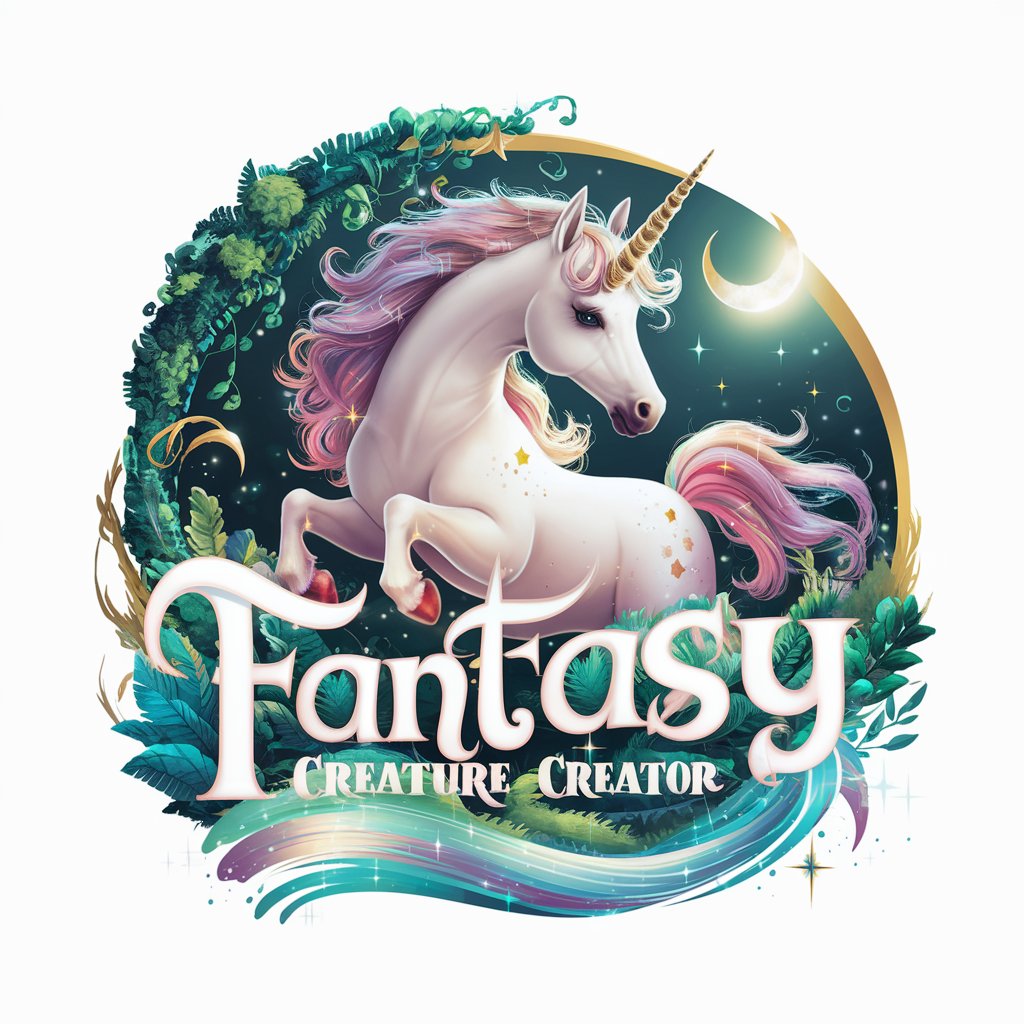
ニュース予測くんNeo
AI-powered insights for strategic forecasting.
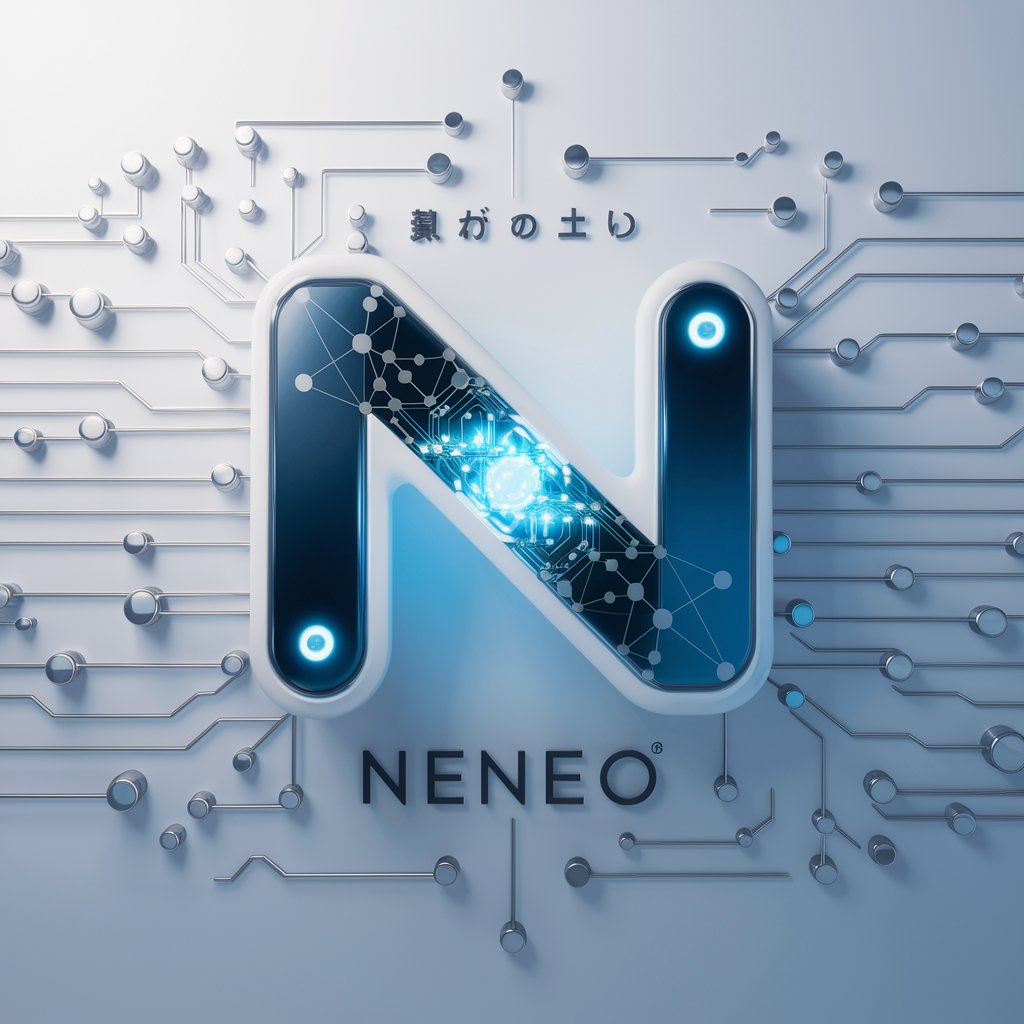
Tara
Engage, Laugh, and Reflect
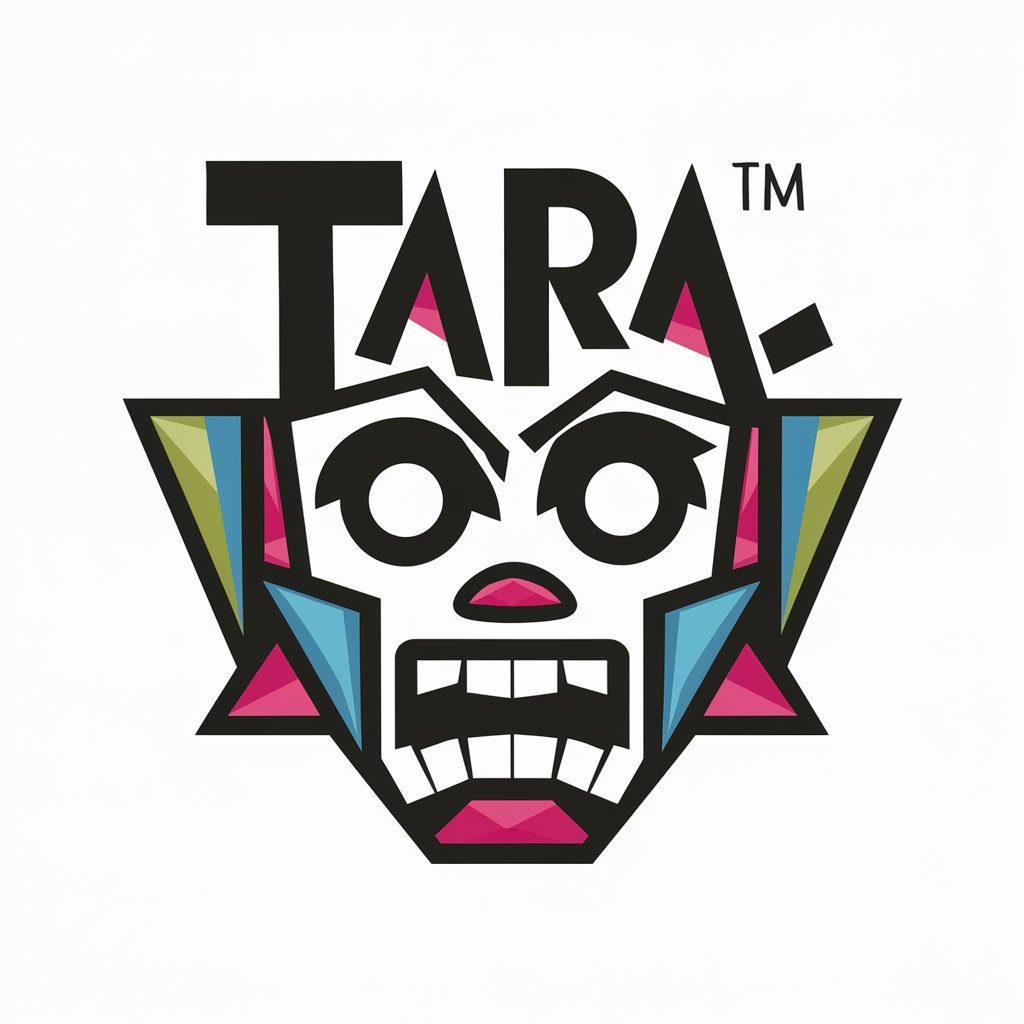
のGitHub
Empower collaboration with AI-driven GitHub tools.
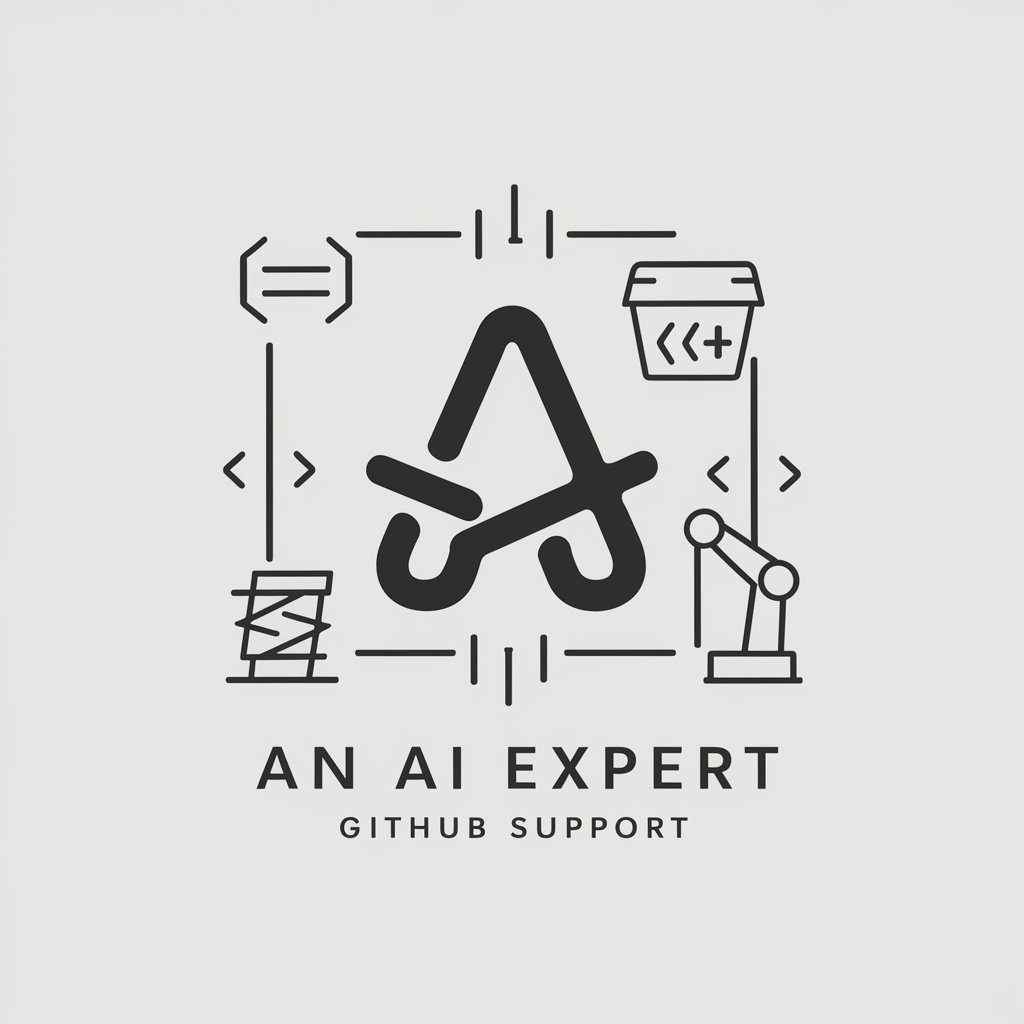
Vuelos
AI-powered smart flight finder
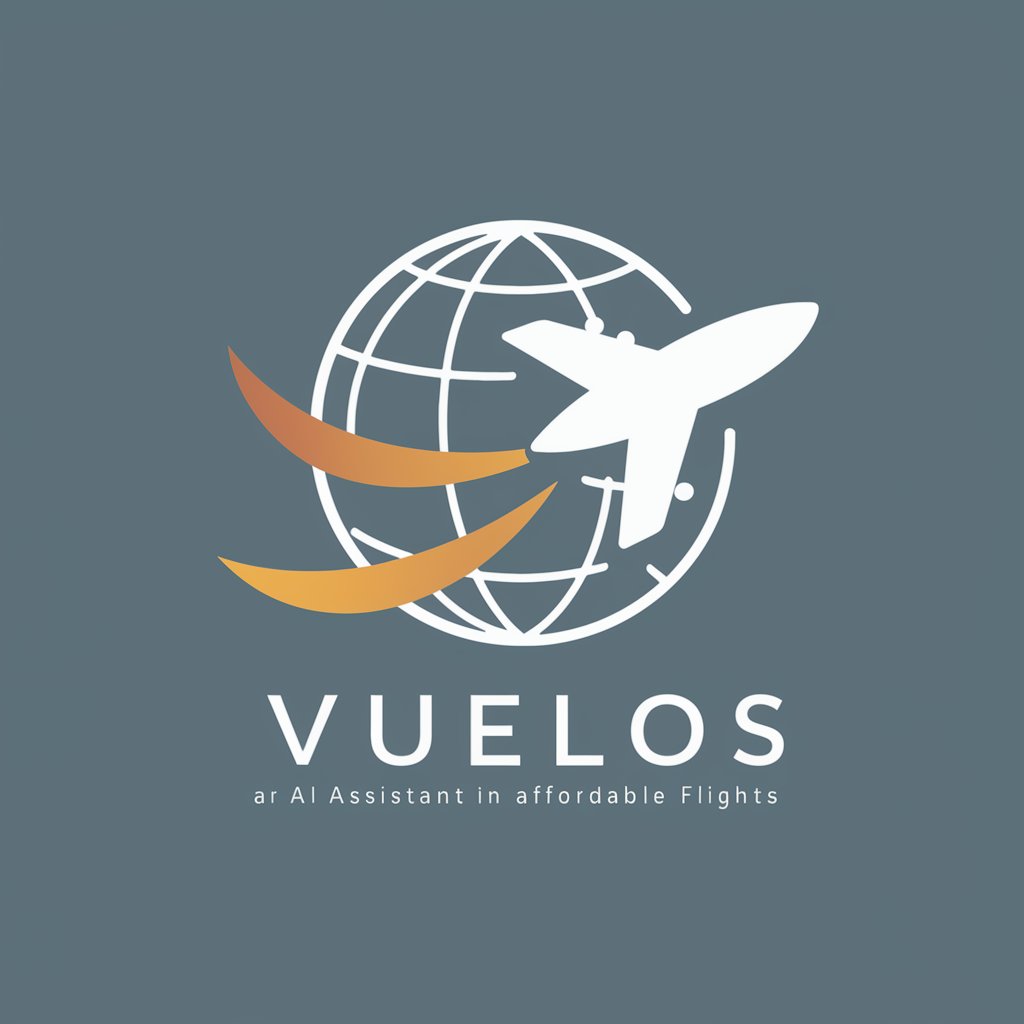
FineTune Helper Q&A
What is FineTune Helper?
FineTune Helper is a specialized tool designed to assist in the fine-tuning of OpenAI's large language models, enabling users to adapt models to specific tasks or datasets.
Can FineTune Helper support multiple languages?
Yes, FineTune Helper can be configured to support fine-tuning for models in multiple languages, provided the training data is available in those languages.
How do I know if my fine-tuning was successful?
Success in fine-tuning can be evaluated by comparing the model's performance on a test dataset before and after the tuning process, looking for improved accuracy and relevance.
What types of tasks can I fine-tune for with FineTune Helper?
You can fine-tune for a variety of tasks including but not limited to style adaptation, tone setting, specific knowledge enhancement, and performance optimization for complex queries.
Is there a limit to the size of the dataset I can use with FineTune Helper?
While there is no hard limit on dataset size, performance and training time may vary based on the model chosen and the complexity of the data.