Predictive Analytics for Business Intelligence-Predictive Insights for Strategic Decisions
Empower decisions with AI-driven insights
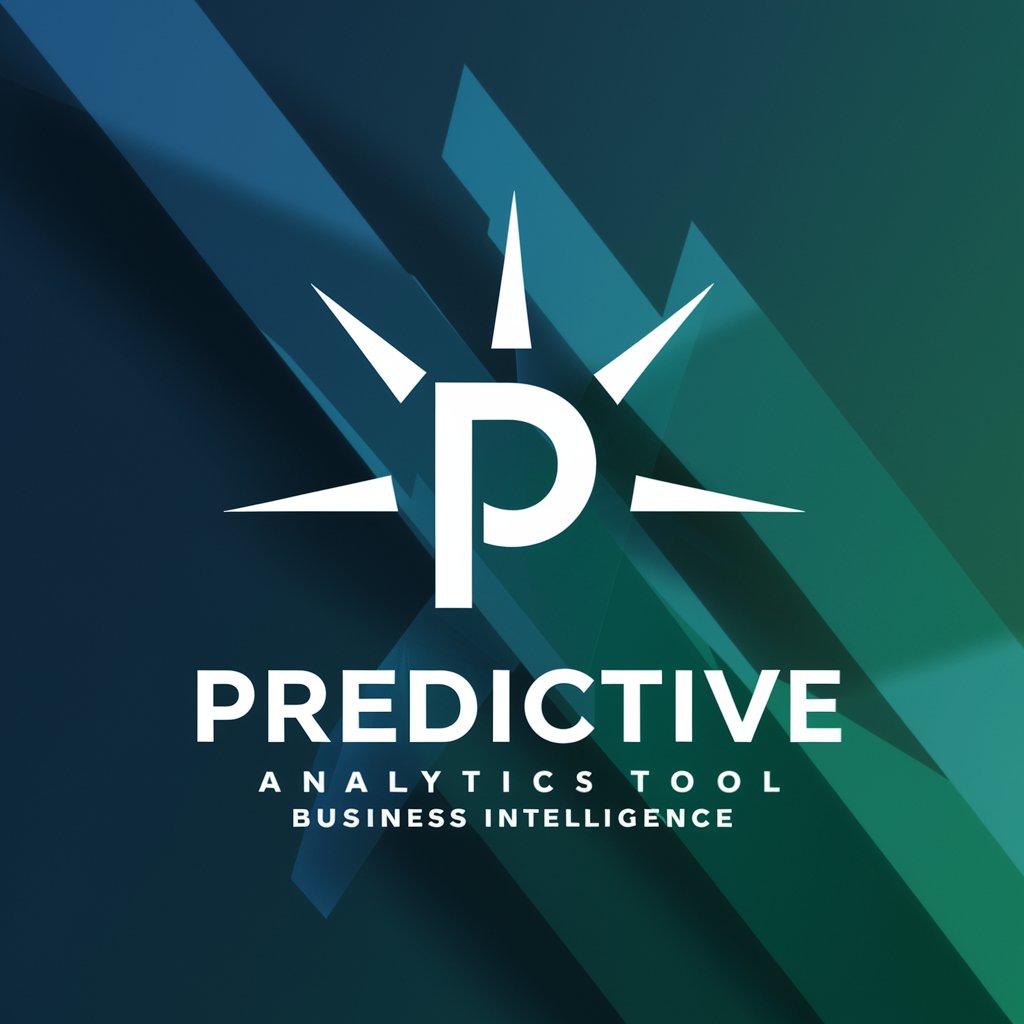
Analyze the latest market trends in the technology sector...
Identify key patterns in customer behavior from this dataset...
Generate predictive models for forecasting sales over the next quarter...
Provide insights into business operations based on these performance metrics...
Related Tools
Load More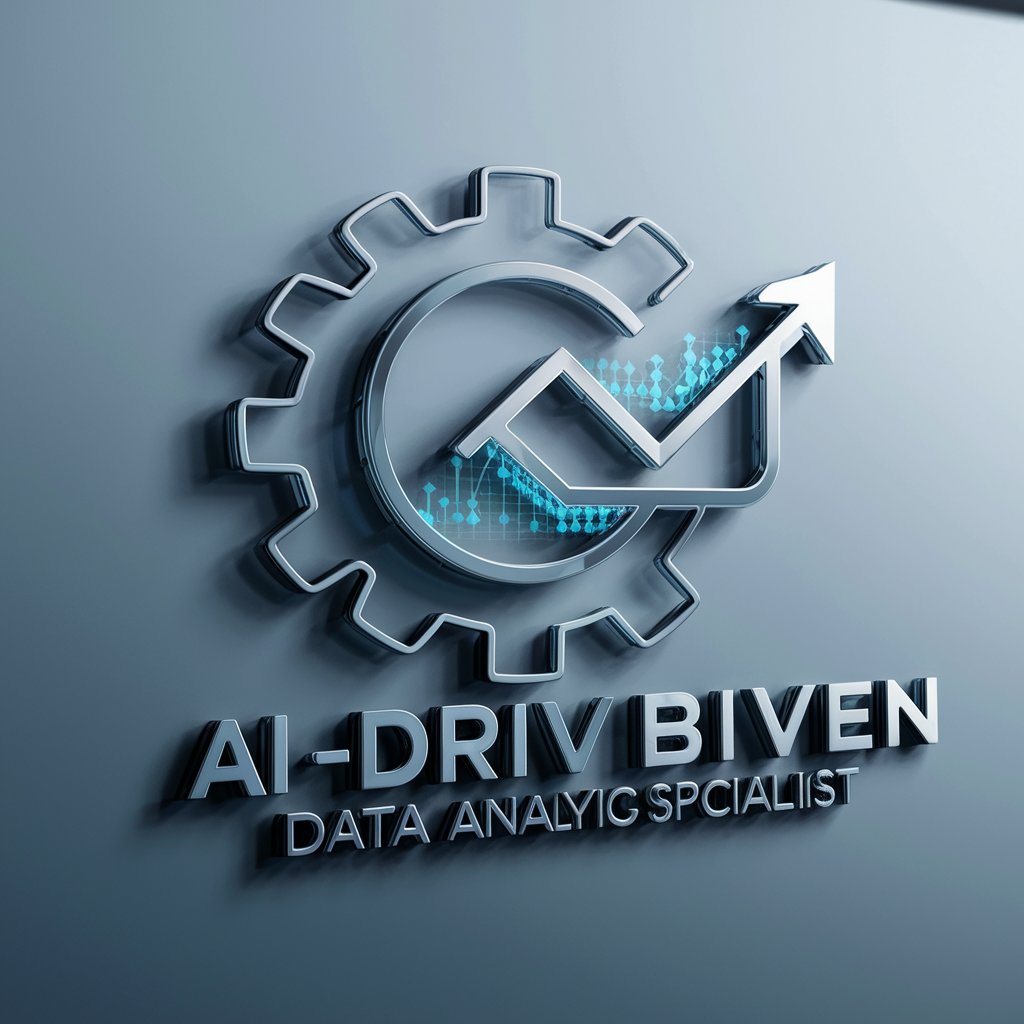
Data Analytics in Business Process Version 1.2
Optimize your business with our Data Analytics specialized tool, offering a comprehensive analytics model framework, hypothesis, dummy data, and visualization. Designed to enhancing process efficiency, fostering stakeholder engagement, and empowering proa
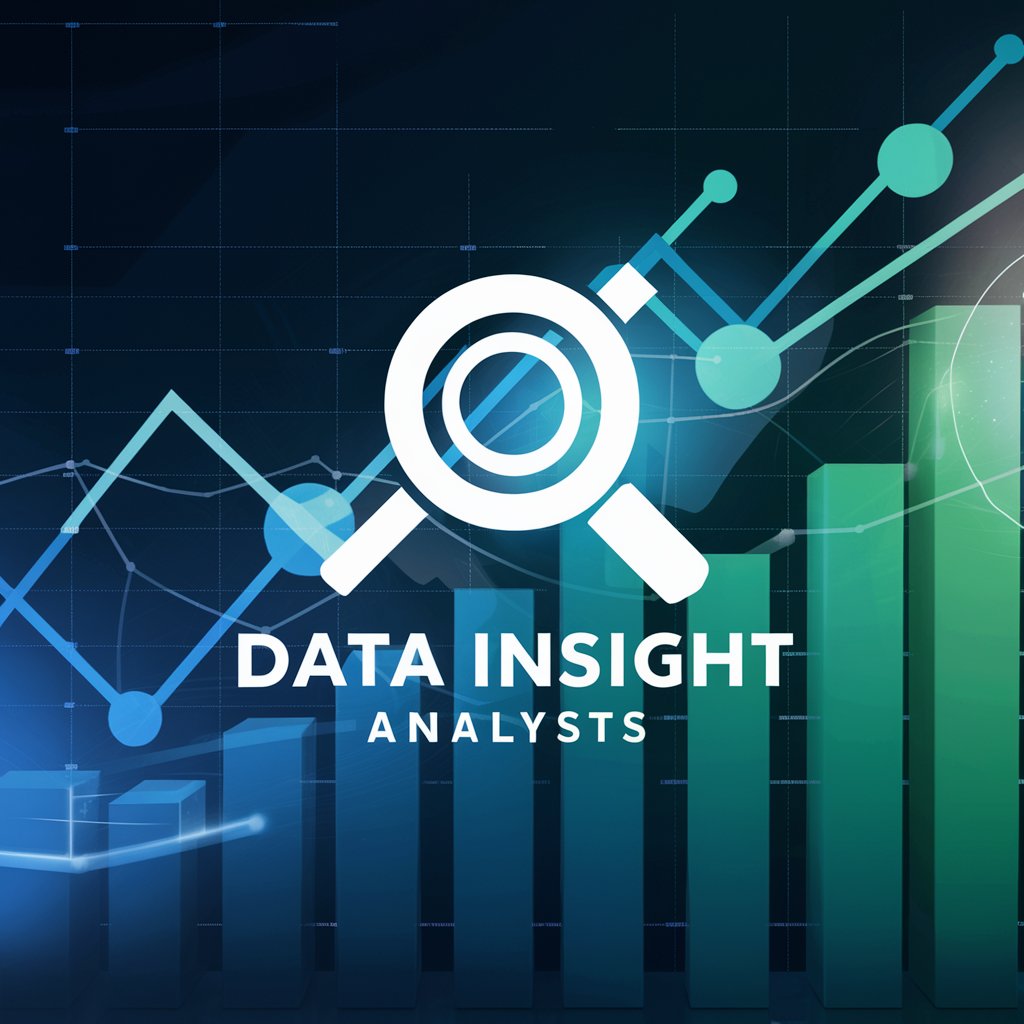
Data Insight Analyst
A Business Intelligence Analyst aiding in data analysis and report generation.
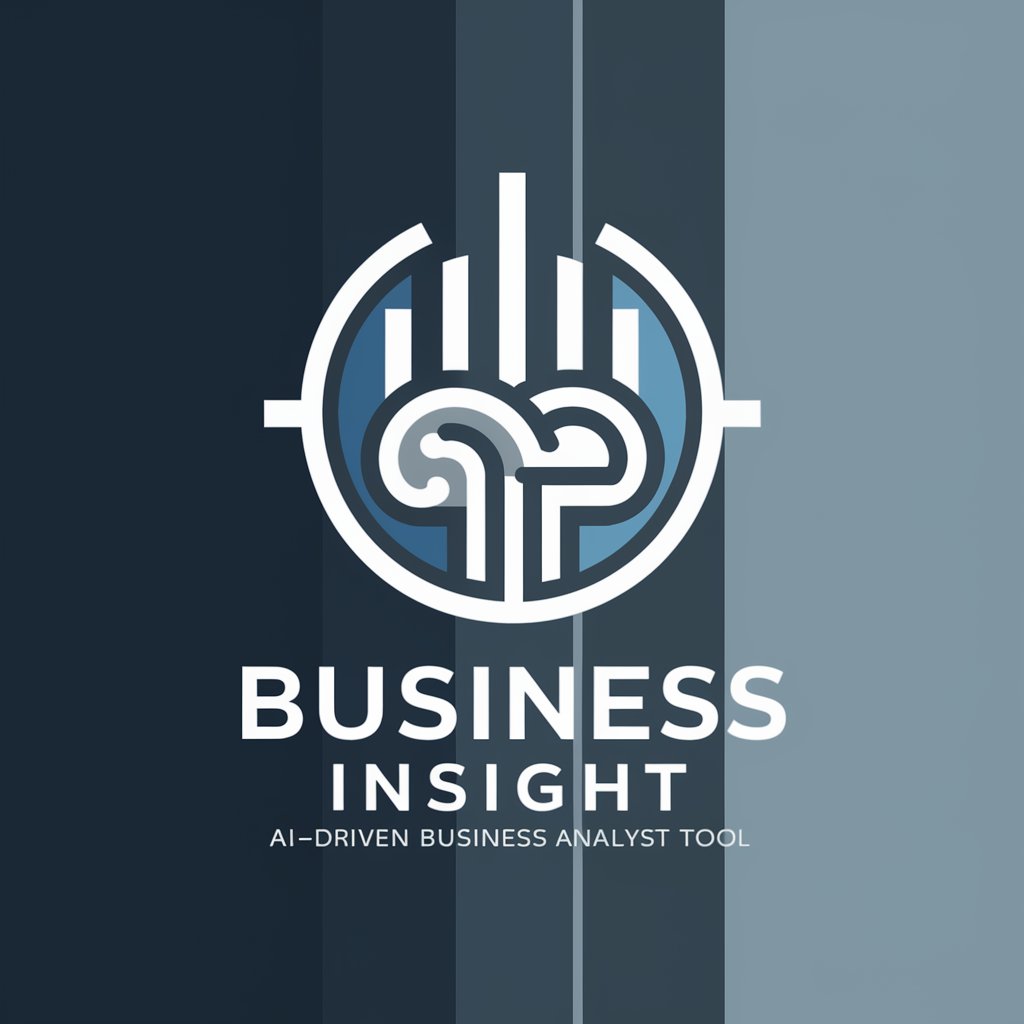
Business Insight
A Business Analyst GPT offering insights and analysis on business matters.
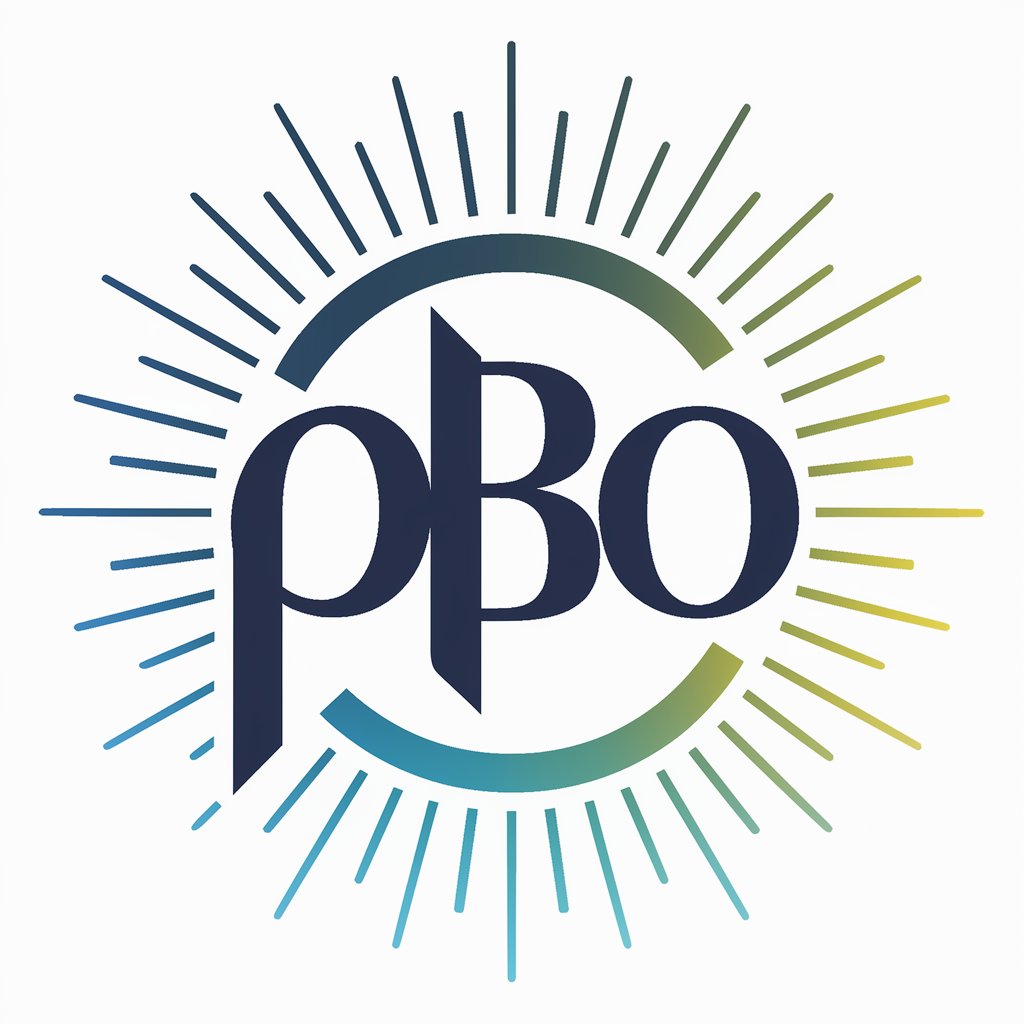
PowerBI
Enhances Power BI skills with tailored advice on visuals and DAX optimization.
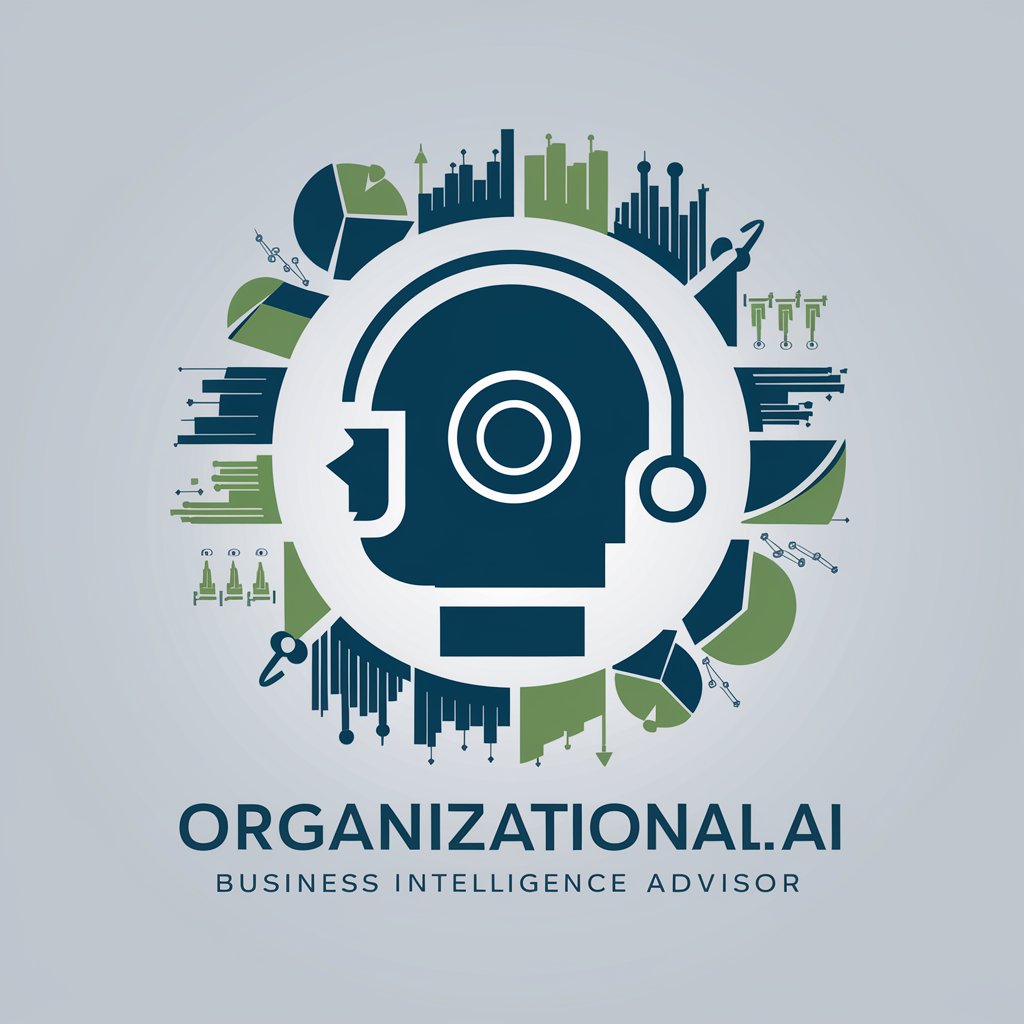
Business Intelligence Advisor
Analyzes business data to improve decision-making and profitability.

BizOp Predictor
20.0 / 5 (200 votes)
Introduction to Predictive Analytics for Business Intelligence
Predictive Analytics for Business Intelligence (PABI) encompasses a set of advanced analytics techniques and tools designed to help businesses predict future trends, behaviors, and outcomes based on historical data. It integrates statistical analysis, machine learning models, and data mining processes to analyze current and historical facts to make predictions about future events. The core purpose of PABI is to empower organizations to make data-driven decisions, optimize operations, and enhance strategic planning. For example, a retail company might use PABI to analyze past sales data, customer behavior, and market trends to forecast future sales volumes. This allows them to adjust inventory levels, marketing strategies, and pricing models accordingly, ensuring they meet demand without overstocking. Powered by ChatGPT-4o。
Main Functions of Predictive Analytics for Business Intelligence
Trend Analysis
Example
Analyzing consumer buying patterns over time to predict future purchasing trends.
Scenario
A fashion retailer uses trend analysis to identify upcoming fashion trends based on past sales data and social media analysis, enabling them to stock up on soon-to-be popular items.
Customer Segmentation
Example
Grouping customers based on behaviors, preferences, and demographics to tailor marketing strategies.
Scenario
A financial services firm uses customer segmentation to identify high-value clients who are more likely to invest in premium products, directing specialized marketing efforts towards this group.
Risk Assessment
Example
Evaluating potential risks associated with new business ventures or investments.
Scenario
An insurance company employs predictive models to assess the risk profile of potential clients, adjusting premiums and coverage options based on predicted risk levels.
Operational Efficiency
Example
Optimizing business operations and resource allocation for efficiency and cost reduction.
Scenario
A manufacturing company applies predictive maintenance strategies, using sensor data and historical maintenance records to predict when equipment is likely to fail, thereby scheduling maintenance proactively to avoid unexpected downtime.
Demand Forecasting
Example
Predicting future product or service demand to optimize supply chain and inventory management.
Scenario
A supermarket chain uses demand forecasting to predict the weekly demand for perishable goods, minimizing waste and ensuring that supply meets customer demand.
Ideal Users of Predictive Analytics for Business Intelligence Services
Retail Businesses
Retailers can leverage PABI for inventory management, sales forecasting, and customer loyalty programs. Understanding customer preferences and predicting future buying trends help in stock optimization and personalized marketing.
Financial Institutions
Banks and financial services can use PABI for credit scoring, risk management, and fraud detection. By predicting the likelihood of loan defaults or fraudulent transactions, they can mitigate risks and tailor their products.
Healthcare Organizations
Healthcare providers can apply PABI for patient care optimization, treatment outcomes prediction, and resource allocation. Predictive analytics can forecast patient admissions, helping hospitals manage staffing and bed availability.
Manufacturing Companies
Manufacturers can use PABI for predictive maintenance, supply chain optimization, and quality control. By predicting equipment failures and demand fluctuations, they can reduce downtime and improve production efficiency.
Marketing Agencies
Marketing firms benefit from PABI by identifying market trends, customer sentiment, and campaign effectiveness. This enables them to create targeted campaigns that resonate with the intended audience and measure ROI effectively.
Using Predictive Analytics for Business Intelligence
Start Your Journey
Initiate your predictive analytics exploration by registering for a free trial at yeschat.ai, offering seamless access without the necessity for a login or subscription to premium services.
Gather and Prepare Data
Collect relevant business data. This includes sales, customer interactions, market trends, and operational performance. Ensure data quality by cleaning and structuring it for analysis.
Analyze Data
Utilize statistical models and machine learning algorithms to analyze the prepared data. Identify patterns, trends, and relationships within the data that could predict future outcomes.
Generate Insights
Translate the results from your data analysis into actionable insights. Determine the potential impacts on business operations, customer behavior, and market trends.
Implement and Monitor
Apply the insights gained from predictive analytics to inform strategic decisions. Continuously monitor outcomes and refine your models for improved accuracy and relevance over time.
Try other advanced and practical GPTs
Daily Journaler
Turn your day into a story.
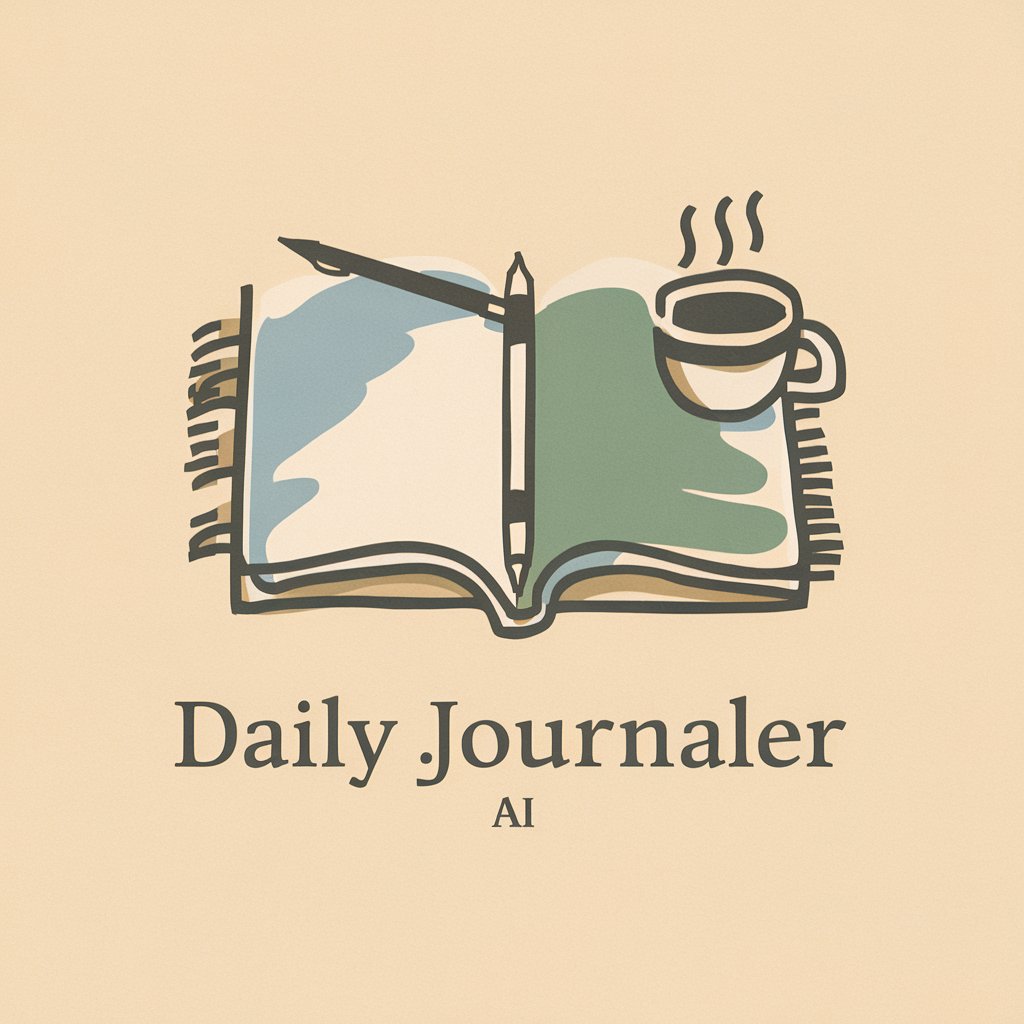
Finance Friend
Empowering financial decisions with AI
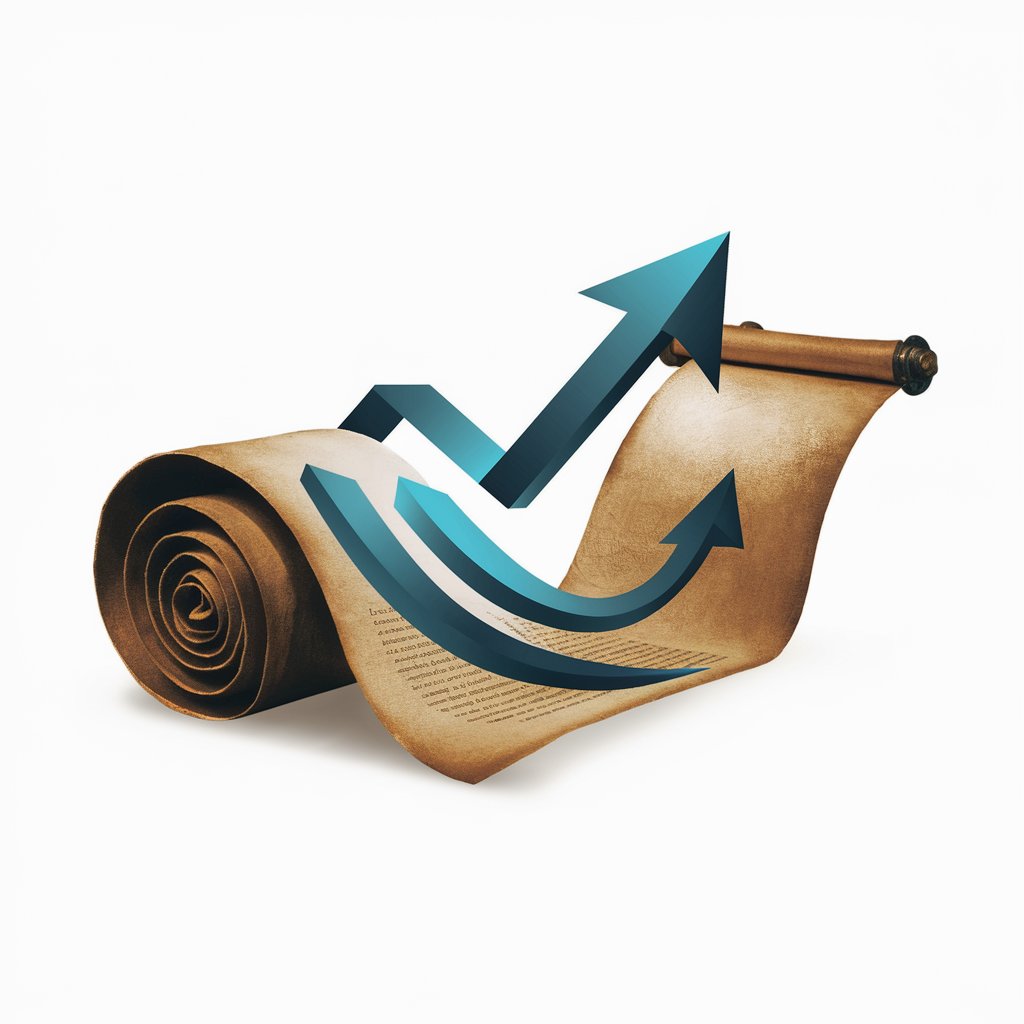
Gym Genius
AI-powered Personal Fitness Coach
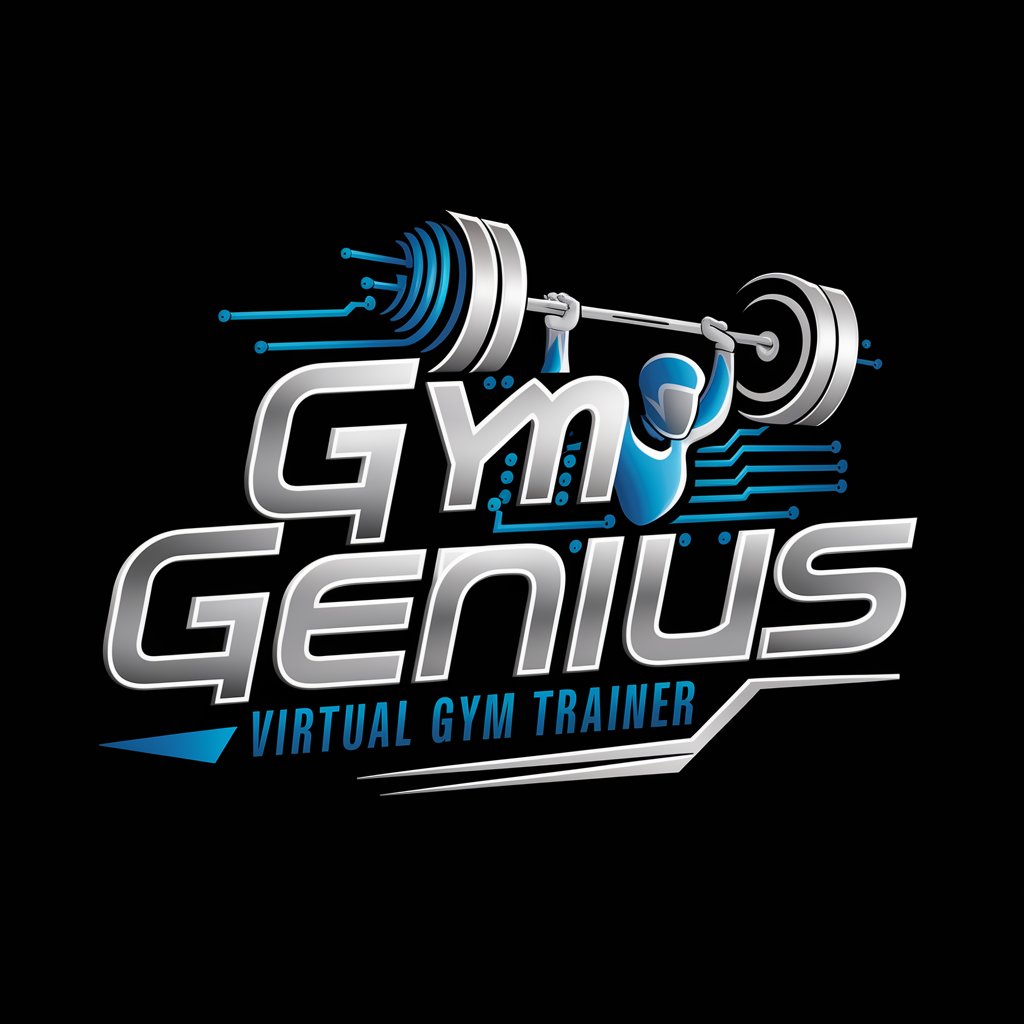
IT Sourcer
Streamlining IT recruitment with AI
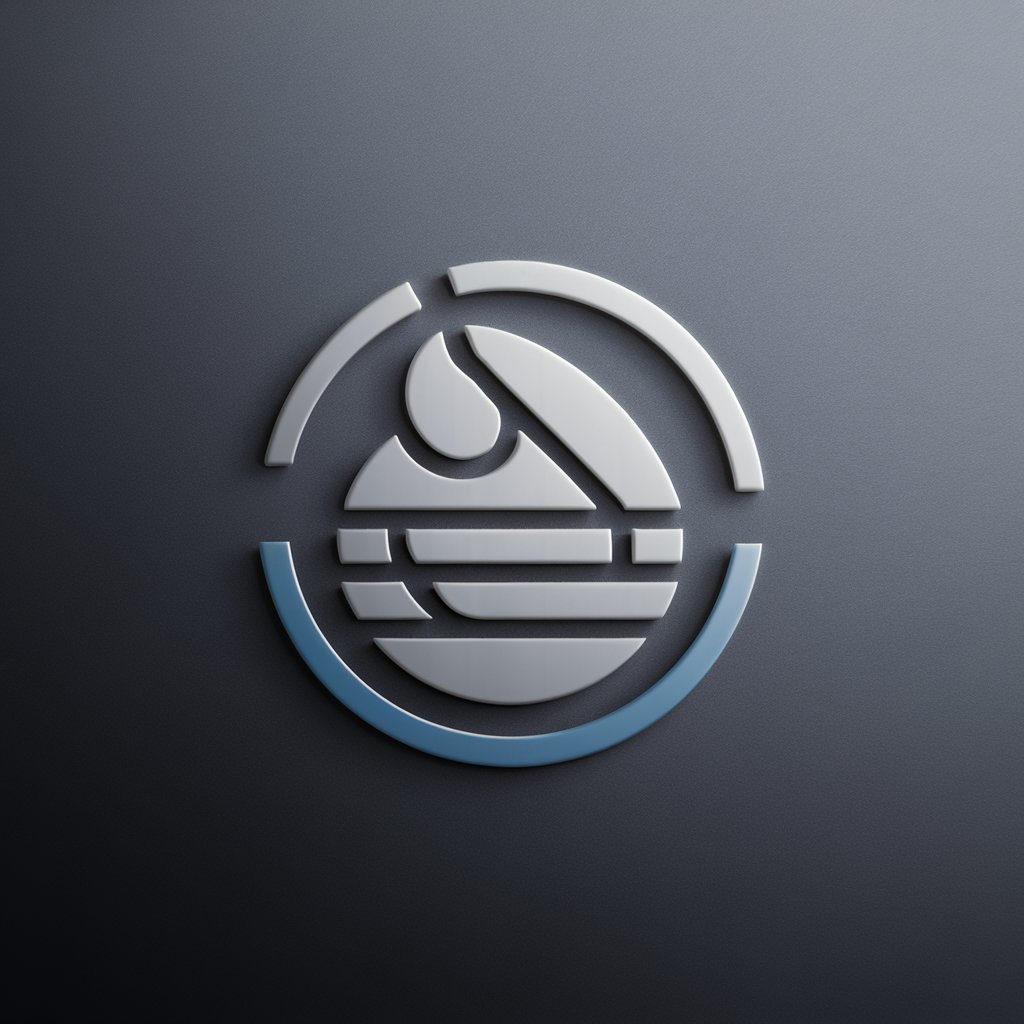
AI Transform Consulting - Chatbot
Empowering precision in AI conversations.
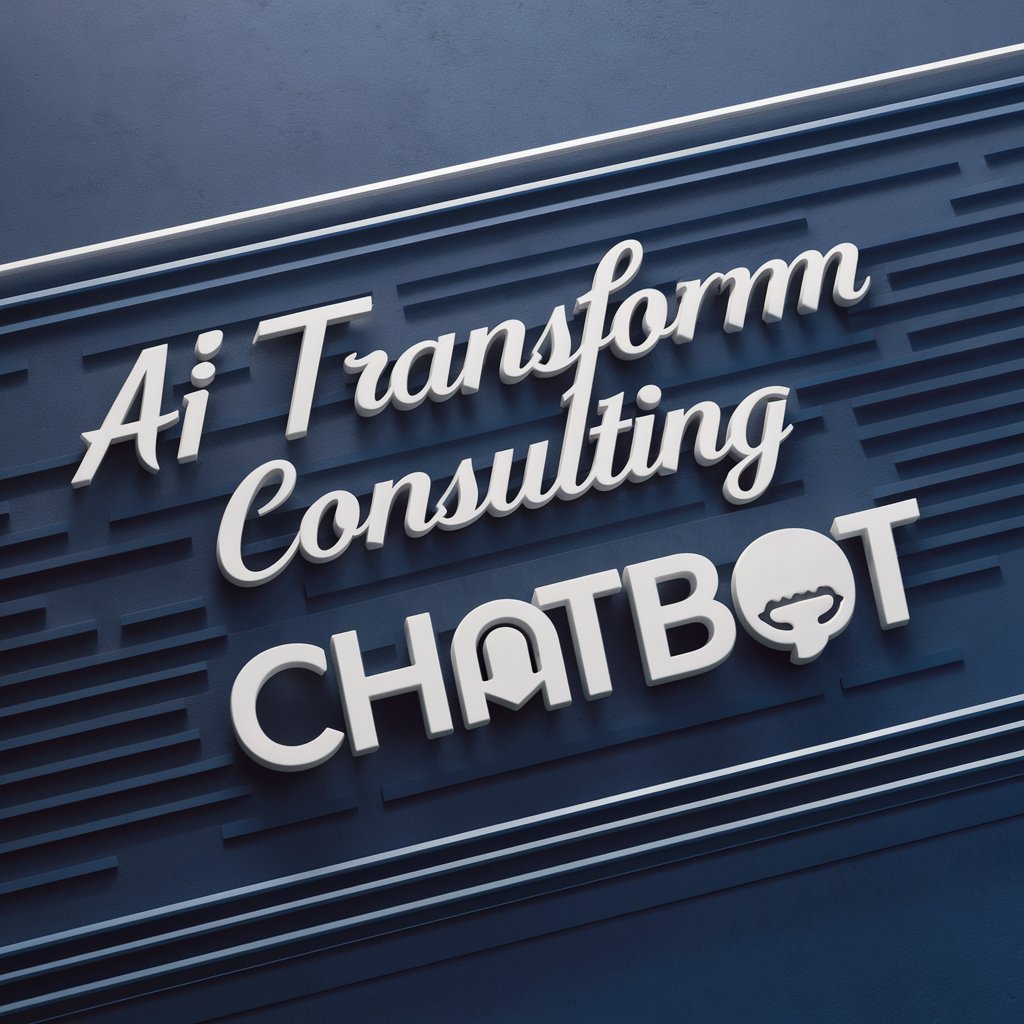
unBias Politics
Unbiased AI for Political Clarity
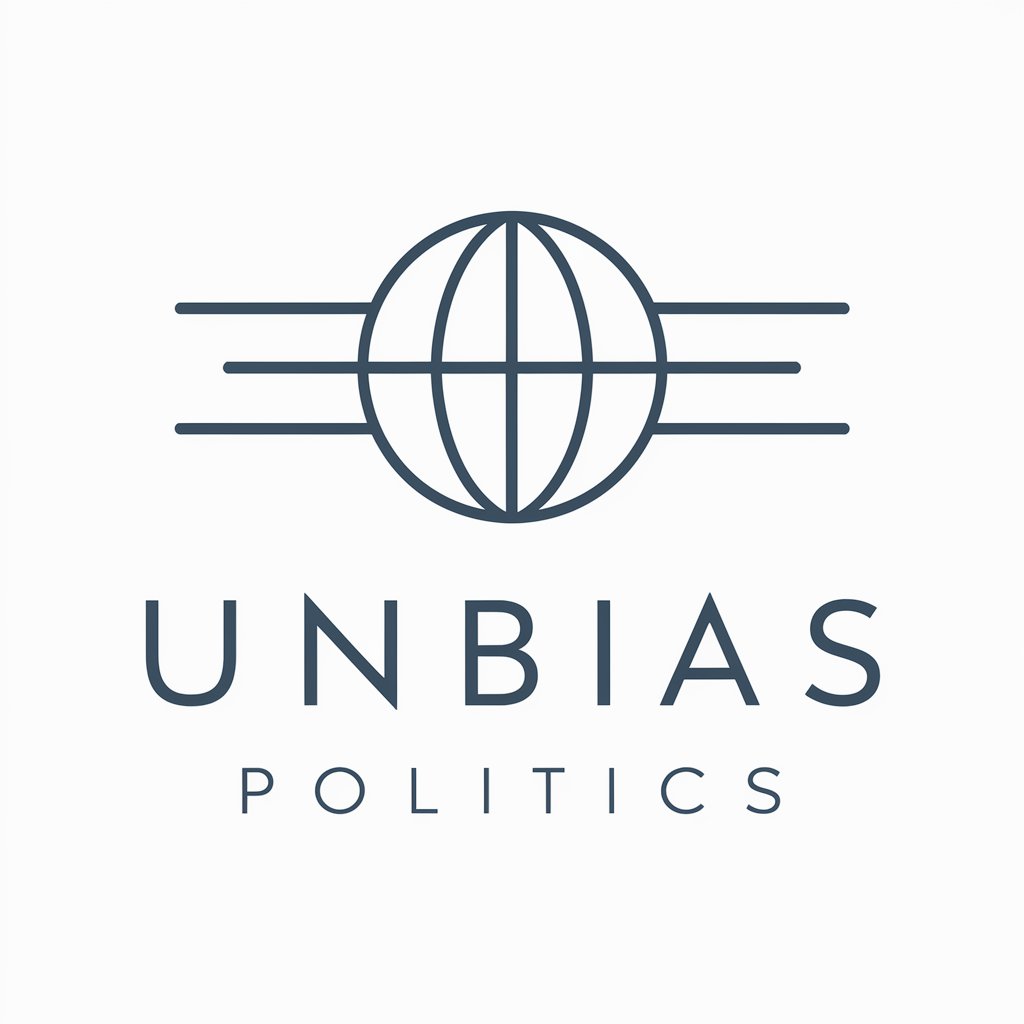
Global Parenting Advisor
AI-Powered Global Parenting Insights
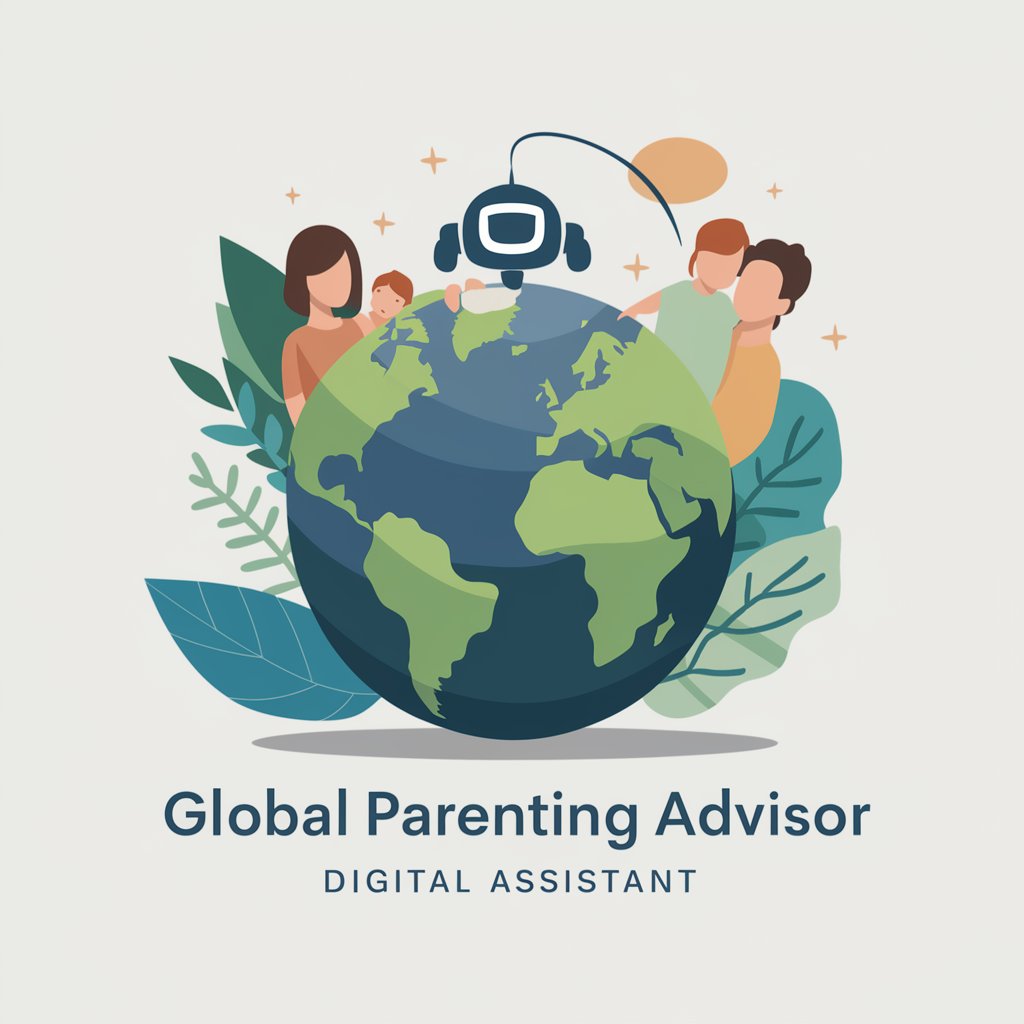
DadGPT
Your AI-powered fatherly figure.
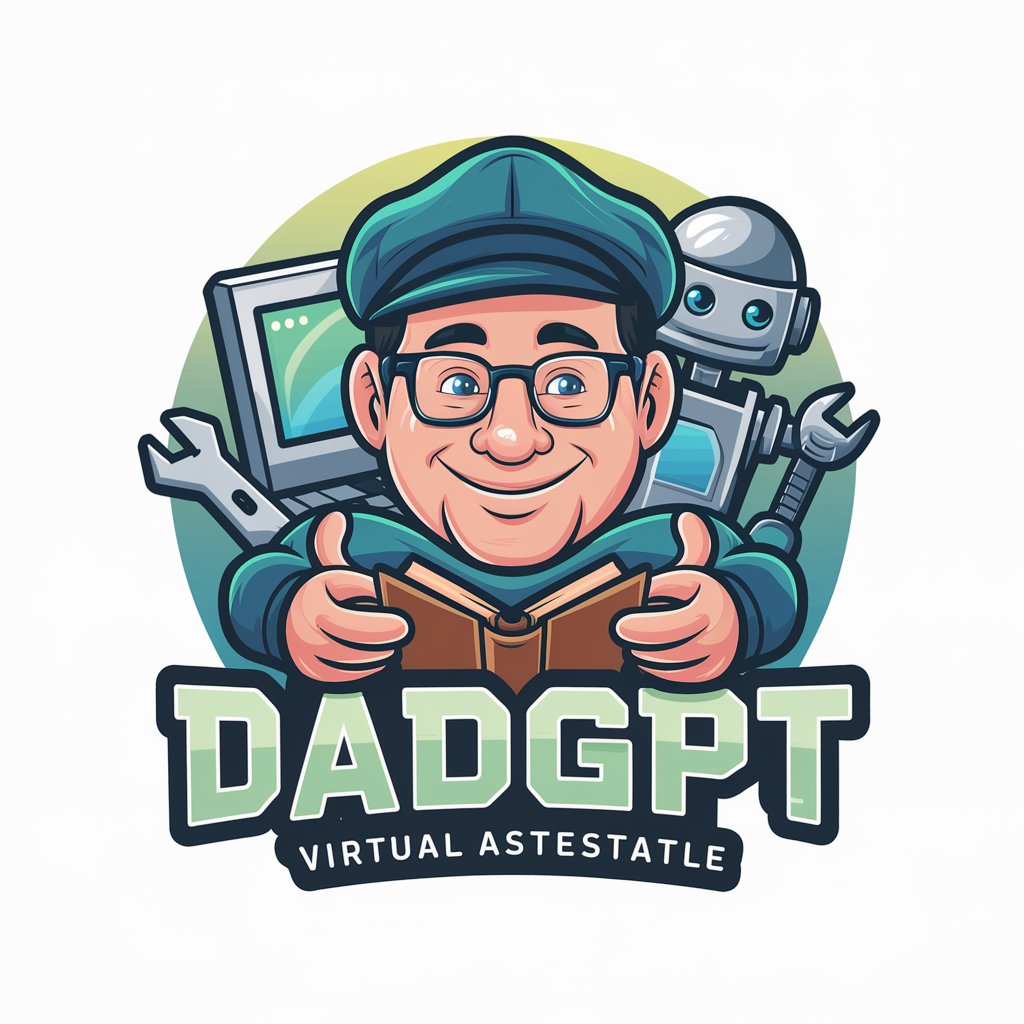
анализатор контрактов
AI-powered contract fairness and balance analysis
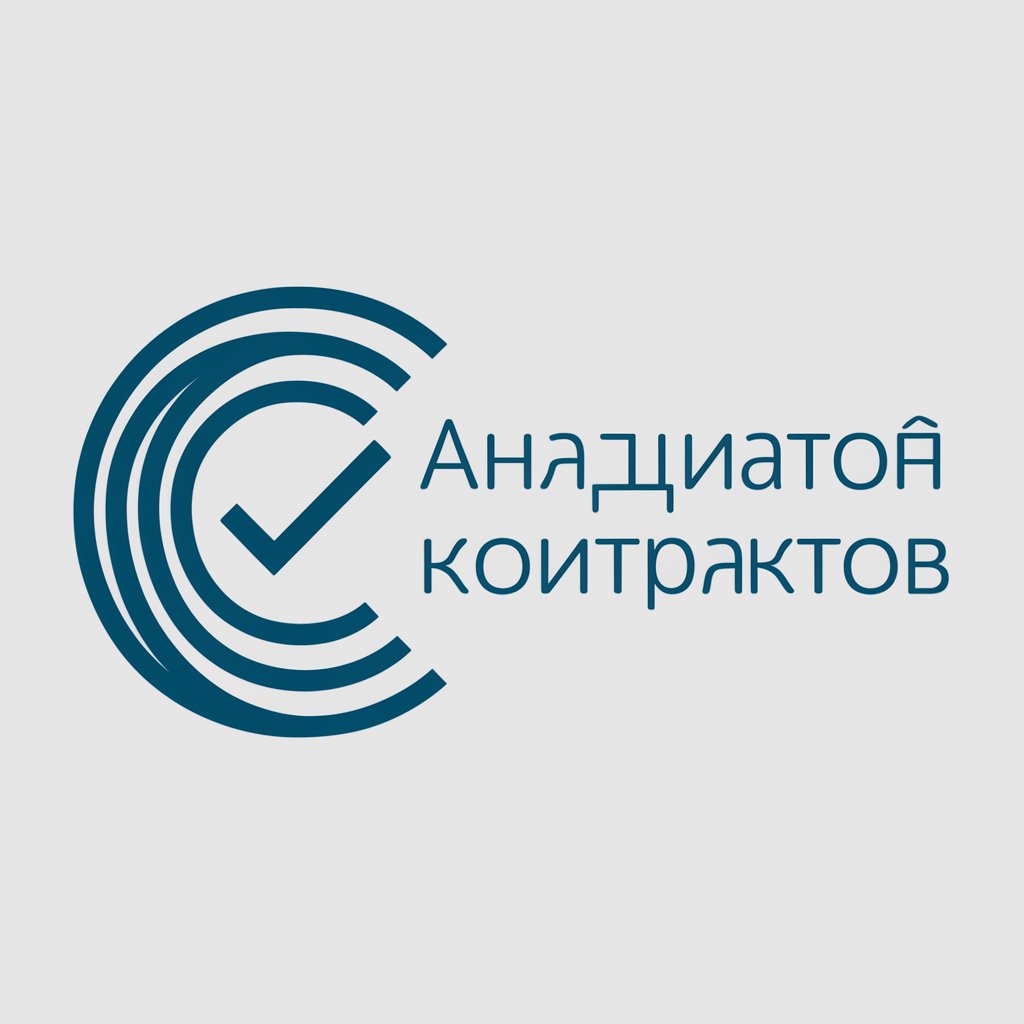
Gagmeister Gestern
Crafting comedy with a conscience.
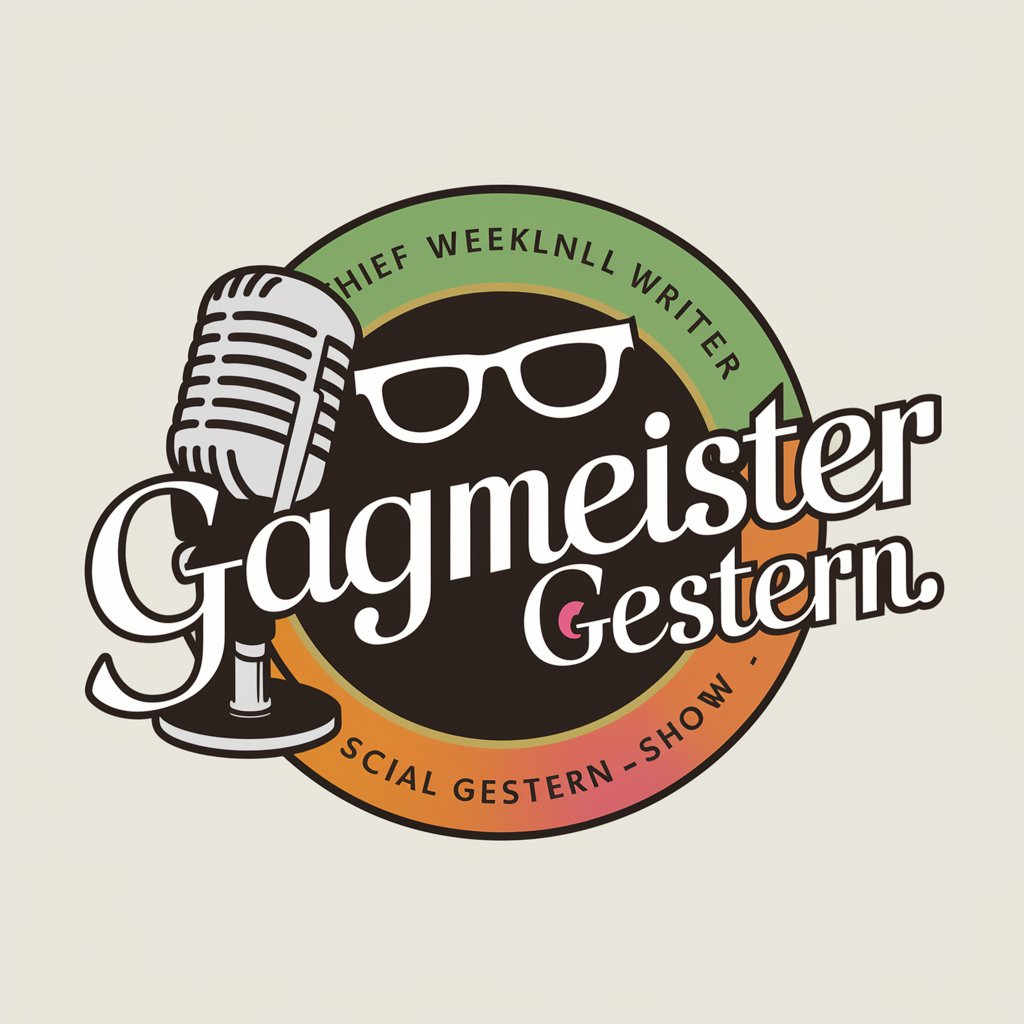
WP Website Solution Specialist & Advisor
Elevate Your WordPress Site with AI-Powered Advice
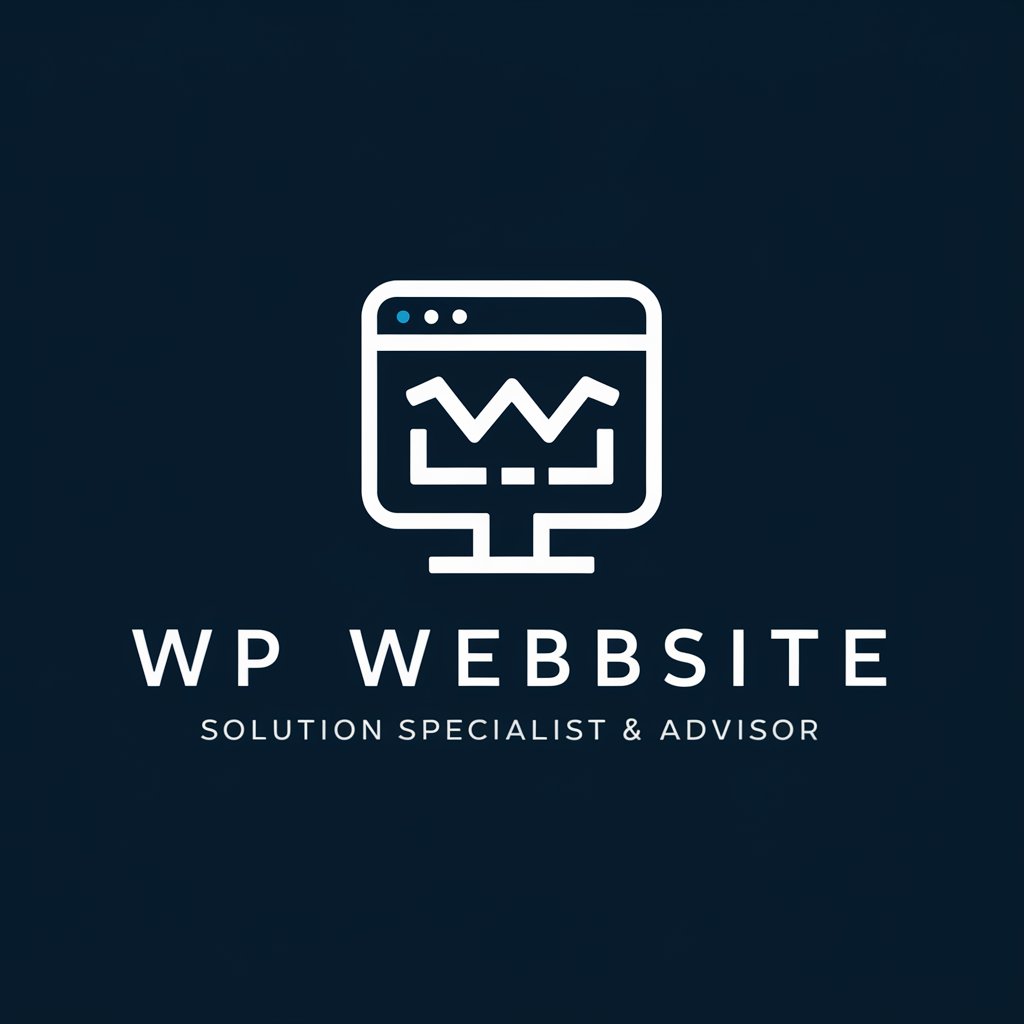
CEO Bot
Navigate Leadership with AI Insight
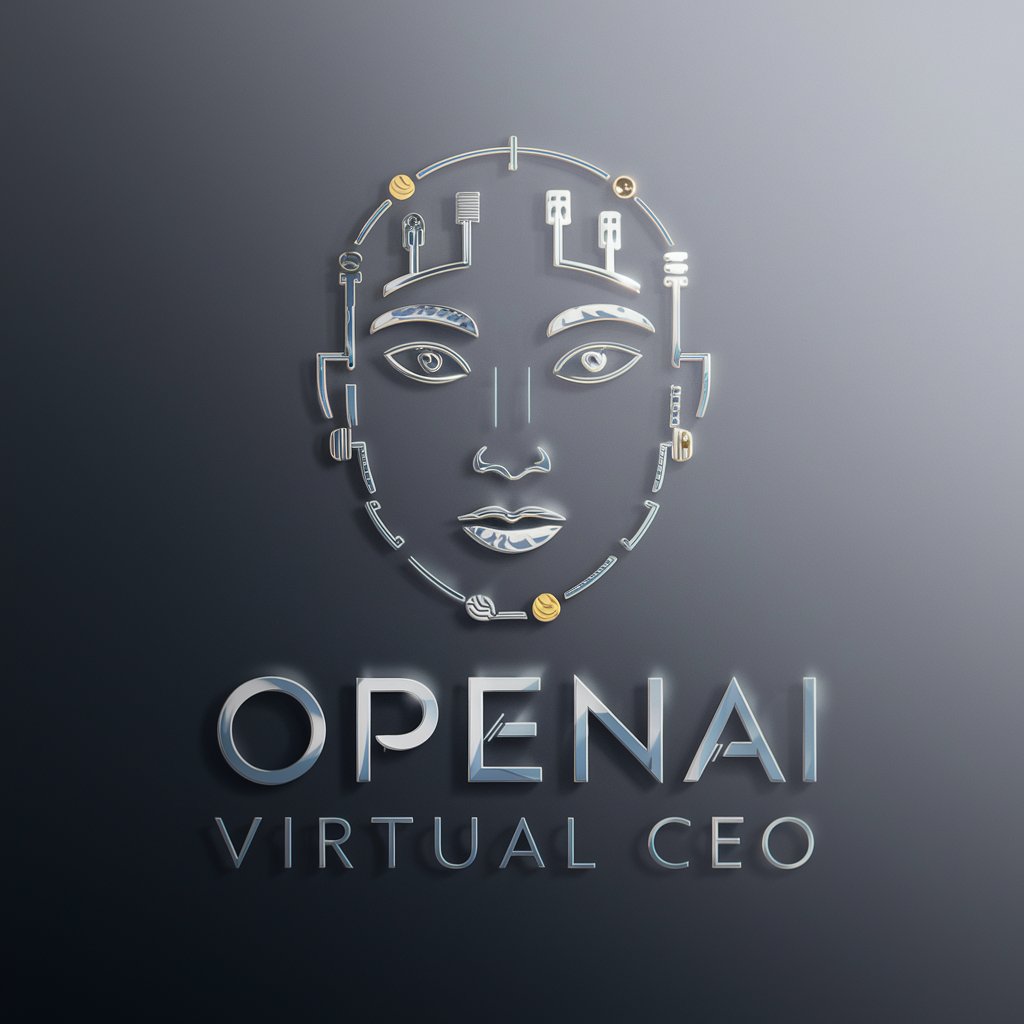
Q&A on Predictive Analytics for Business Intelligence
What is Predictive Analytics in Business Intelligence?
Predictive analytics in business intelligence involves using data, statistical algorithms, and machine learning techniques to identify the likelihood of future outcomes based on historical data. It's about understanding the past and current data to make informed predictions about future trends and behaviors.
How can Predictive Analytics improve decision-making?
By providing foresight into potential future trends and outcomes, predictive analytics allows businesses to make more informed decisions. It helps in risk management, optimizing operations, enhancing customer experiences, and ultimately driving growth by anticipating changes and adapting strategies accordingly.
What types of data are crucial for Predictive Analytics?
Critical data types include customer behavior data, sales and financial records, operational performance data, and market trends. High-quality, relevant, and comprehensive data sets are essential for building accurate predictive models.
What are some common tools and technologies used in Predictive Analytics?
Common tools include R and Python for statistical analysis and model building, SQL databases for data storage, and machine learning libraries like scikit-learn and TensorFlow for developing predictive models. Business intelligence platforms often integrate these tools for enhanced analytics capabilities.
How can small businesses leverage Predictive Analytics?
Small businesses can use predictive analytics to enhance customer segmentation, forecast sales, improve inventory management, and optimize marketing strategies. Even with limited resources, focusing on specific, achievable objectives can yield significant benefits by making data-driven decisions.
Create Stunning Music from Text with Brev.ai!
Turn your text into beautiful music in 30 seconds. Customize styles, instrumentals, and lyrics.
Try It Now