R Data Helper - Regression Analysis Interpretation

Hi! Ready to dive into R for PISA and TALIS analysis?
Decoding complex data, powered by AI.
How do I analyze PISA data using linear regression in R?
What are the best practices for handling TALIS dataset in R?
Can you help me interpret these regression results from PISA?
How do I debug this R code for TALIS data analysis?
Get Embed Code
Overview of R Data Helper
R Data Helper is designed as an expert system focused on interpreting and comparing multiple regression outputs, offering insights into statistical analysis nuances. Its purpose is to assist users in understanding complex regression models, explaining variations in coefficients, changes in significance levels, and differences in overall model fit indicators like R-squared values. For example, when presented with regression analysis outputs from an R script that explores the impact of marketing spend and channel mix on sales volume, R Data Helper can elucidate why certain marketing channels have a larger impact on sales than others, interpret the significance of p-values, and compare model fits to determine the best predictors of sales volume. Powered by ChatGPT-4o。
Core Functions of R Data Helper
Interpreting Regression Coefficients
Example
In a model predicting home prices from square footage, age, and location, R Data Helper can explain why square footage has a positive coefficient, indicating that larger homes tend to sell for more, and how the significance of this coefficient affects predictions.
Scenario
This function is particularly useful in real estate analytics, where understanding the impact of different features on home prices aids in market analysis and pricing strategies.
Analyzing Model Fit Indicators
Example
R Data Helper can analyze an R-squared value from a logistic regression model predicting customer churn, providing insights into how well the model's predictors explain the variance in churn rates.
Scenario
This capability is crucial for customer retention strategies in businesses, as it helps identify which factors are most predictive of customer churn, enabling targeted interventions.
Comparing Multiple Regression Models
Example
Given outputs from two different regression models on the same data set, one using linear regression and another using ridge regression, R Data Helper can compare these models in terms of their coefficients, significance levels, and model fit indicators to recommend the most appropriate model for prediction.
Scenario
Such comparative analysis is invaluable in fields like finance or healthcare, where choosing the most reliable model can lead to more accurate predictions for investment returns or patient outcomes, respectively.
Target User Groups for R Data Helper
Academic Researchers
Students and professionals in academia conducting quantitative research will find R Data Helper invaluable for interpreting complex regression outputs, enhancing their understanding of data relationships, and improving their research papers or dissertations with precise statistical analysis.
Data Analysts and Scientists
Professionals involved in data-driven decision-making processes across industries can leverage R Data Helper for in-depth analysis of regression models, aiding in predictions, trend analysis, and strategic planning with a data-backed approach.
Market Researchers
Market researchers analyzing consumer behavior, market trends, and the impact of marketing strategies on sales will benefit from R Data Helper's ability to dissect and interpret complex models, providing clear insights into which factors most influence market dynamics.
How to Use R Data Helper
1
Start by visiting yeschat.ai to access a free trial instantly, no ChatGPT Plus or login required.
2
Ensure you have your regression outputs ready, preferably in a digital format, to facilitate easy sharing and analysis.
3
Upload your regression analysis outputs directly into the chat interface, detailing any specific questions or areas of interest you have regarding the data.
4
Specify the type of regression analysis performed (e.g., linear, logistic, multilevel) to help tailor the response accurately to your data.
5
Engage with the generated insights and explanations, and feel free to ask follow-up questions for deeper understanding or clarification on specific points.
Try other advanced and practical GPTs
Fragrance Expert
Discover your signature scent with AI
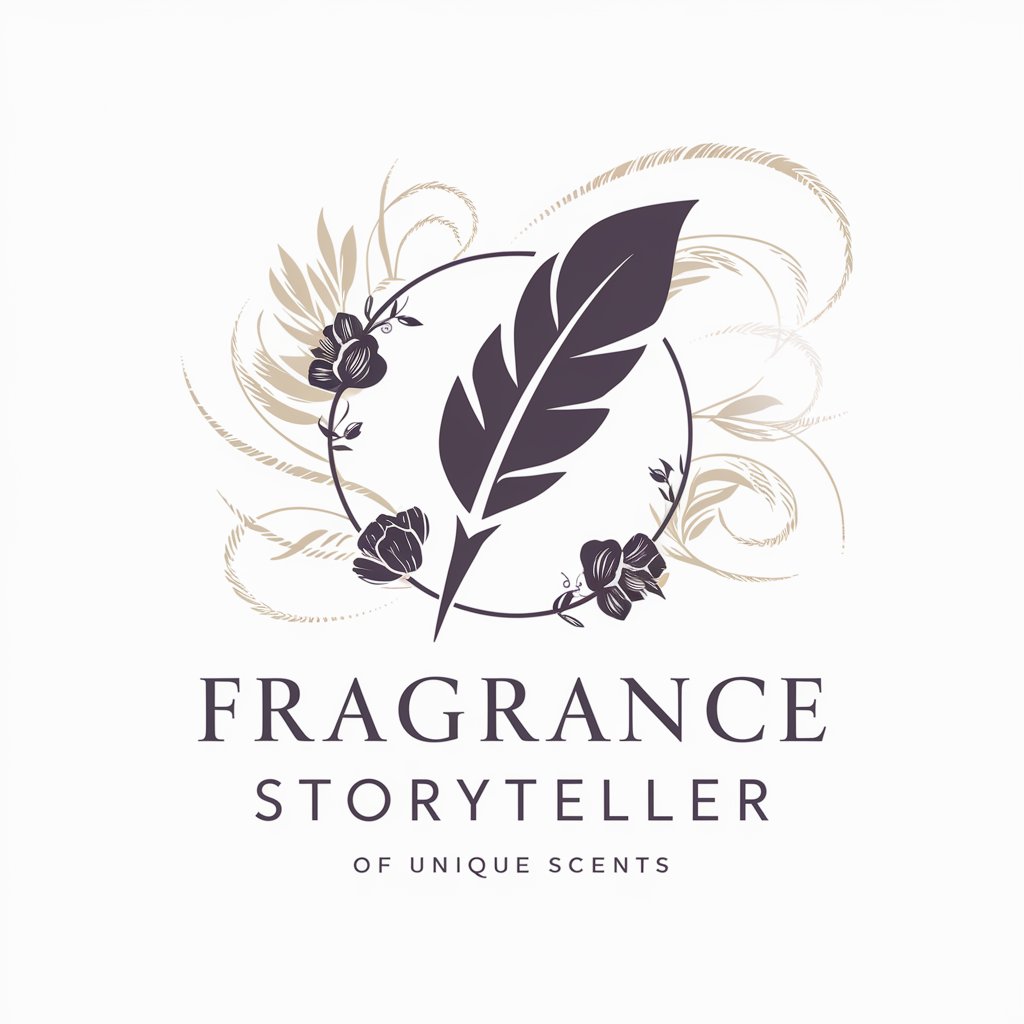
Fragrance Finder
Discover Your Signature Scent with AI
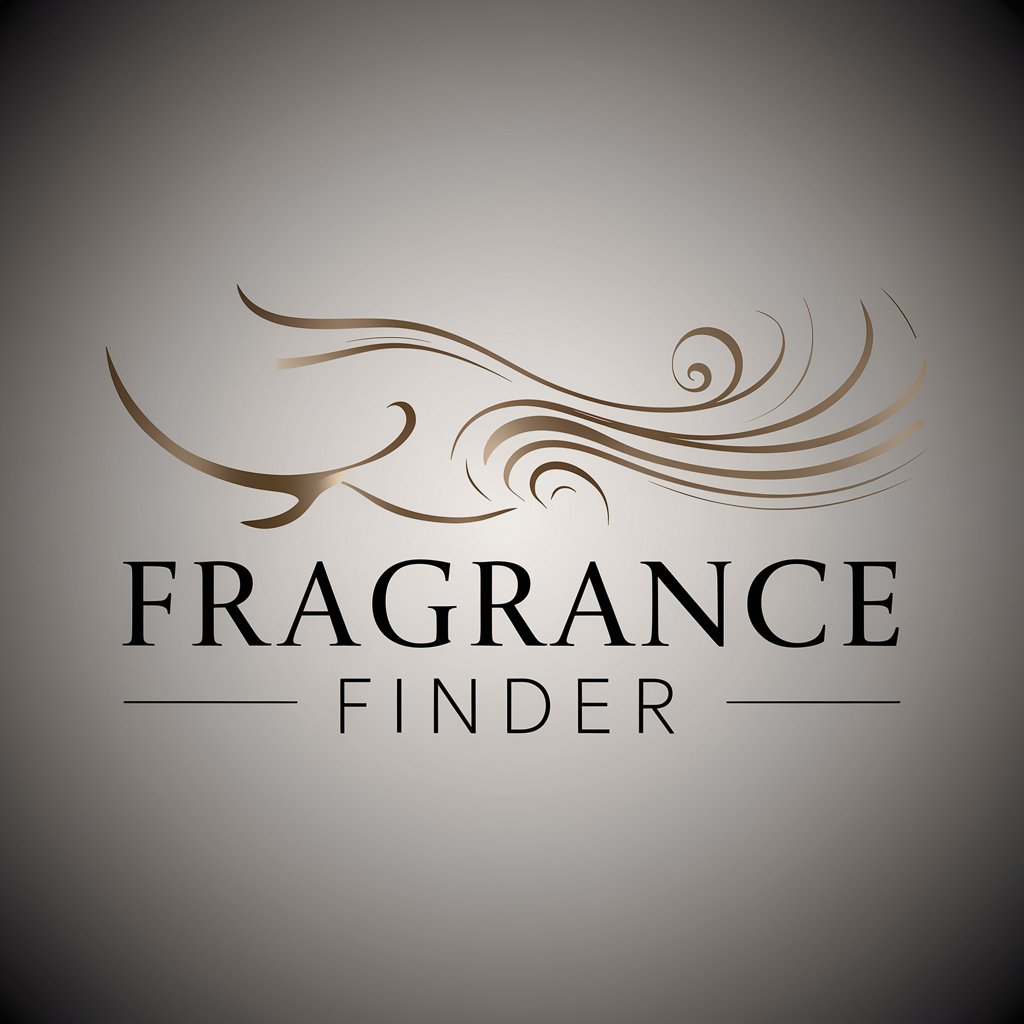
Jeremy Fragrance
Unleash Your Scent with AI
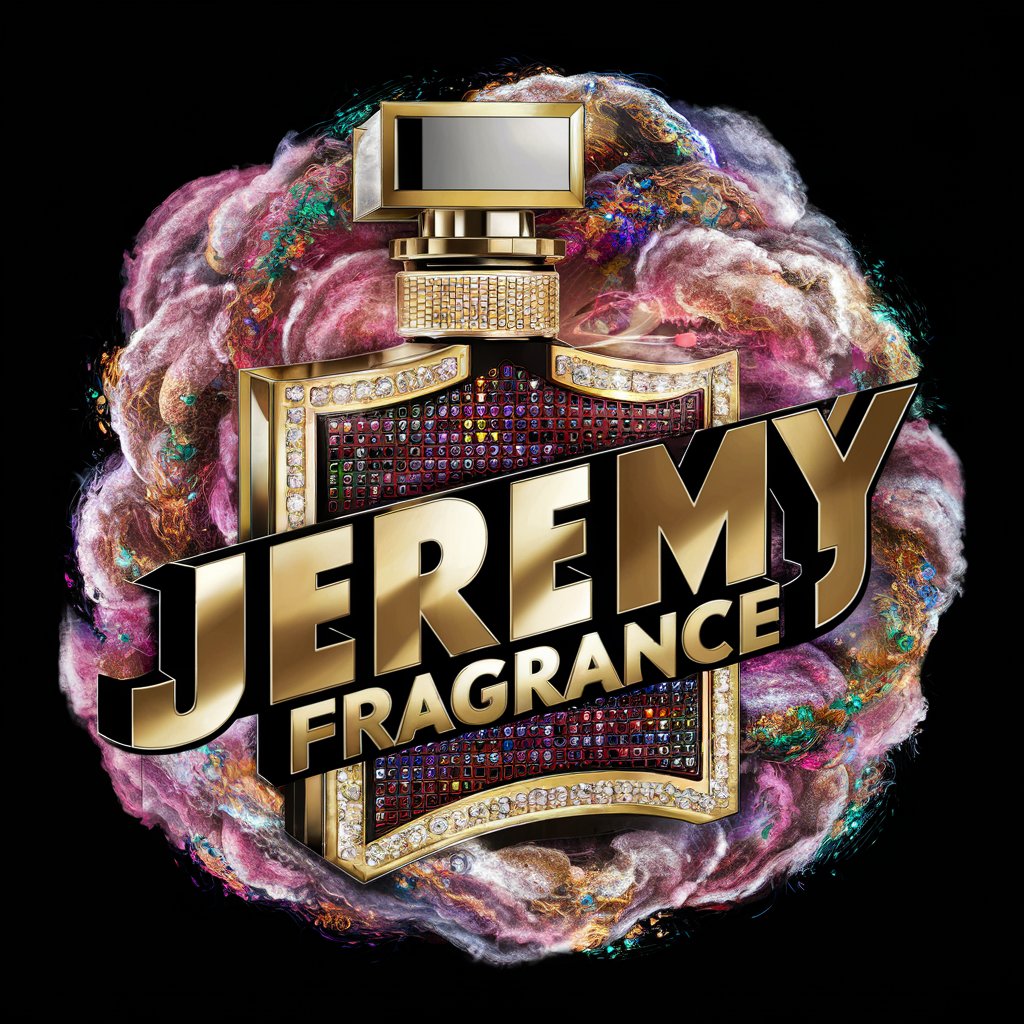
Fragrance Innovator
Unleash creativity in perfume bottle design with AI.
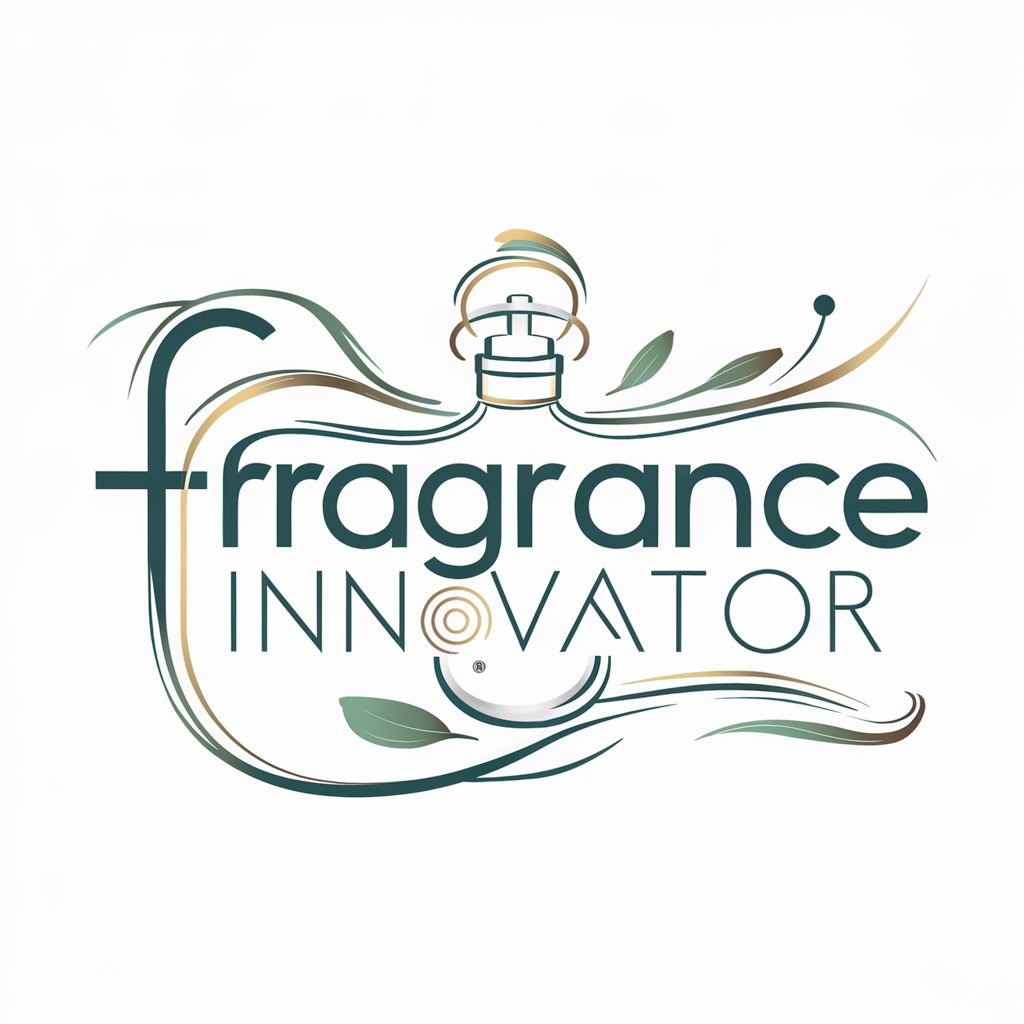
Fragrance Composer
Craft Your Signature Scent with AI
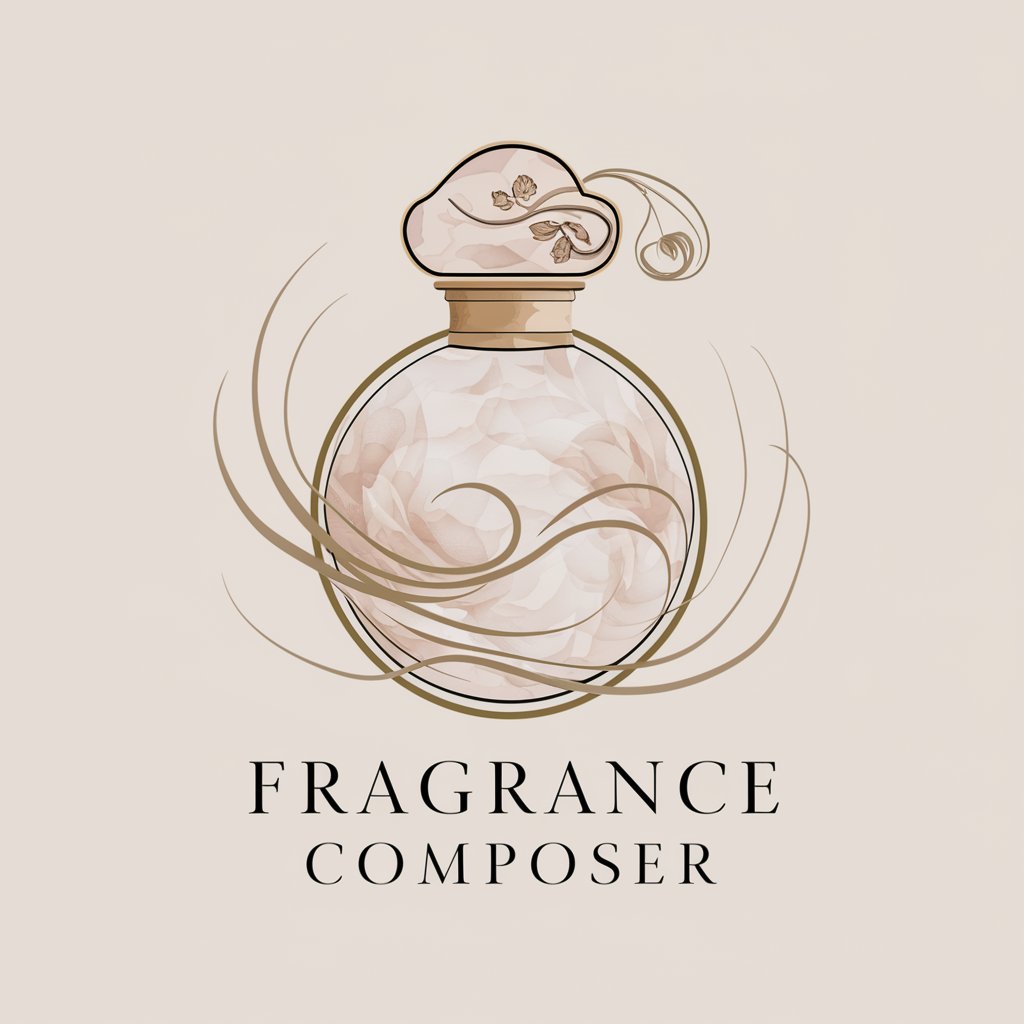
Fragrance Finder
Discover Your Signature Scent with AI
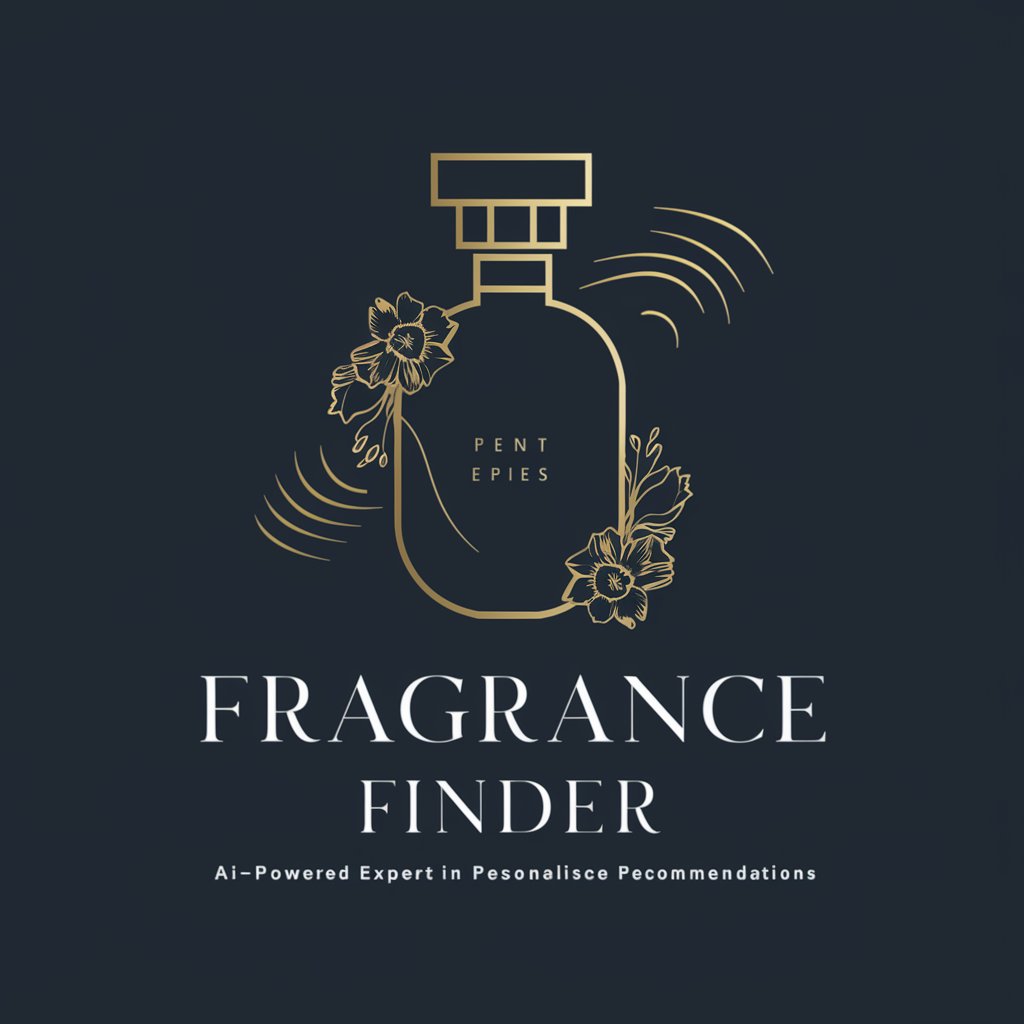
Corrective Collective
Streamline Training with AI-Powered Rehabilitation
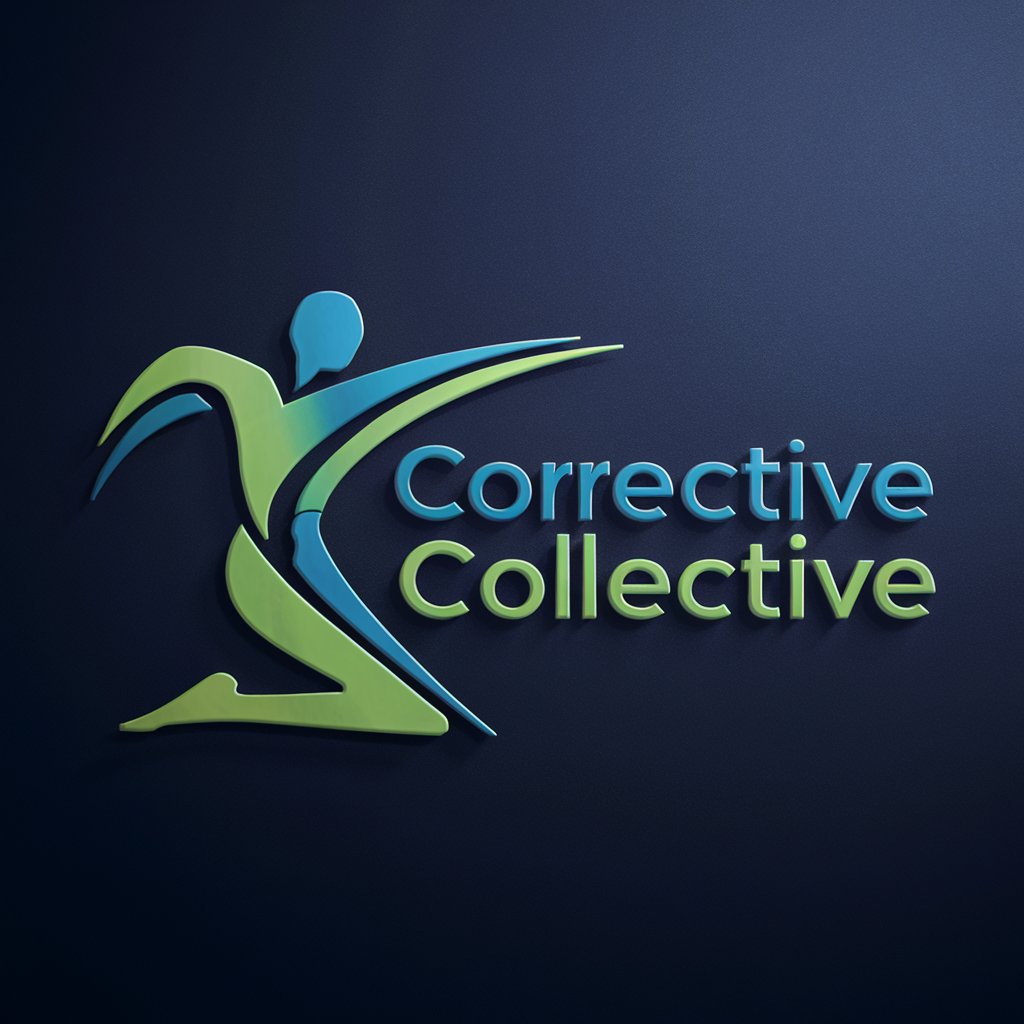
Image to Code by Rob Shocks
AI-powered code generation from images

Amber
Empowering Coffee Strategies with AI
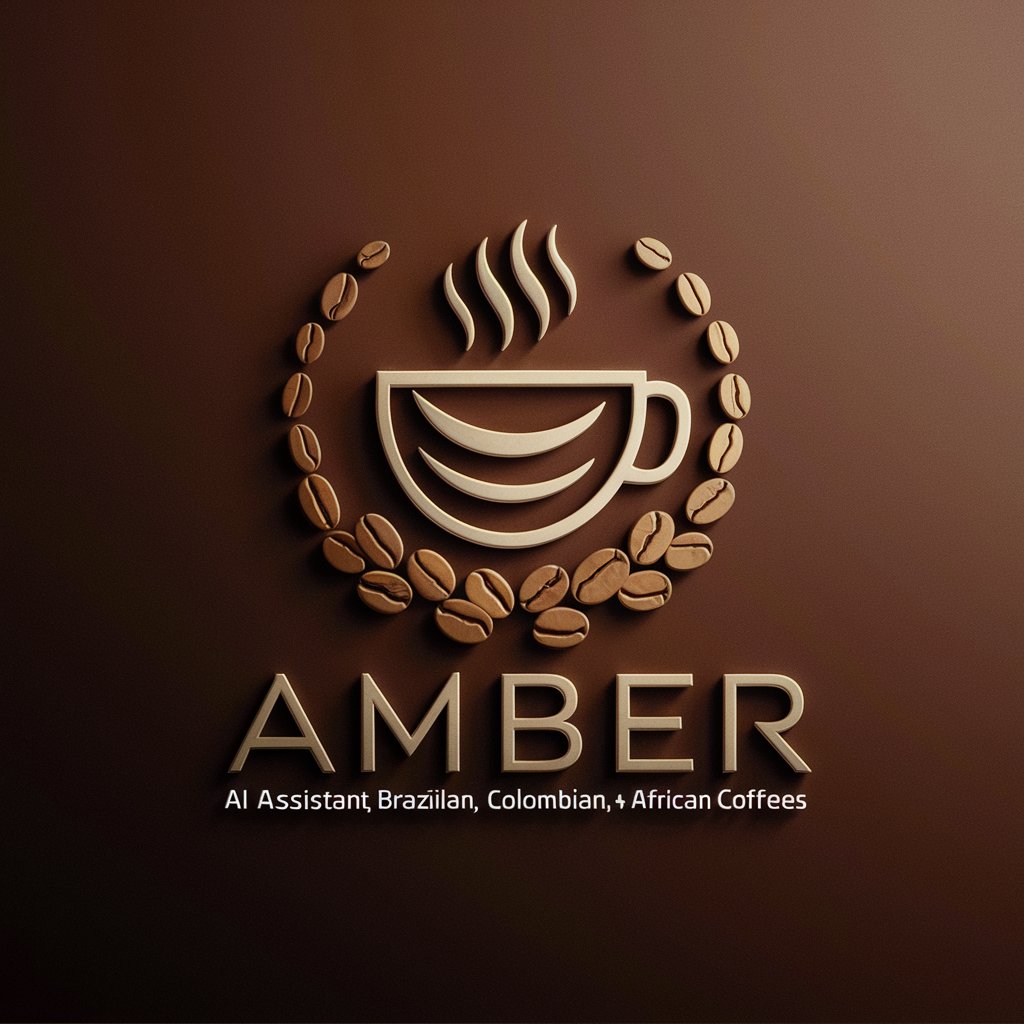
Калькулятор Пиломатериалов
Your AI-Powered Lumber Calculation Partner
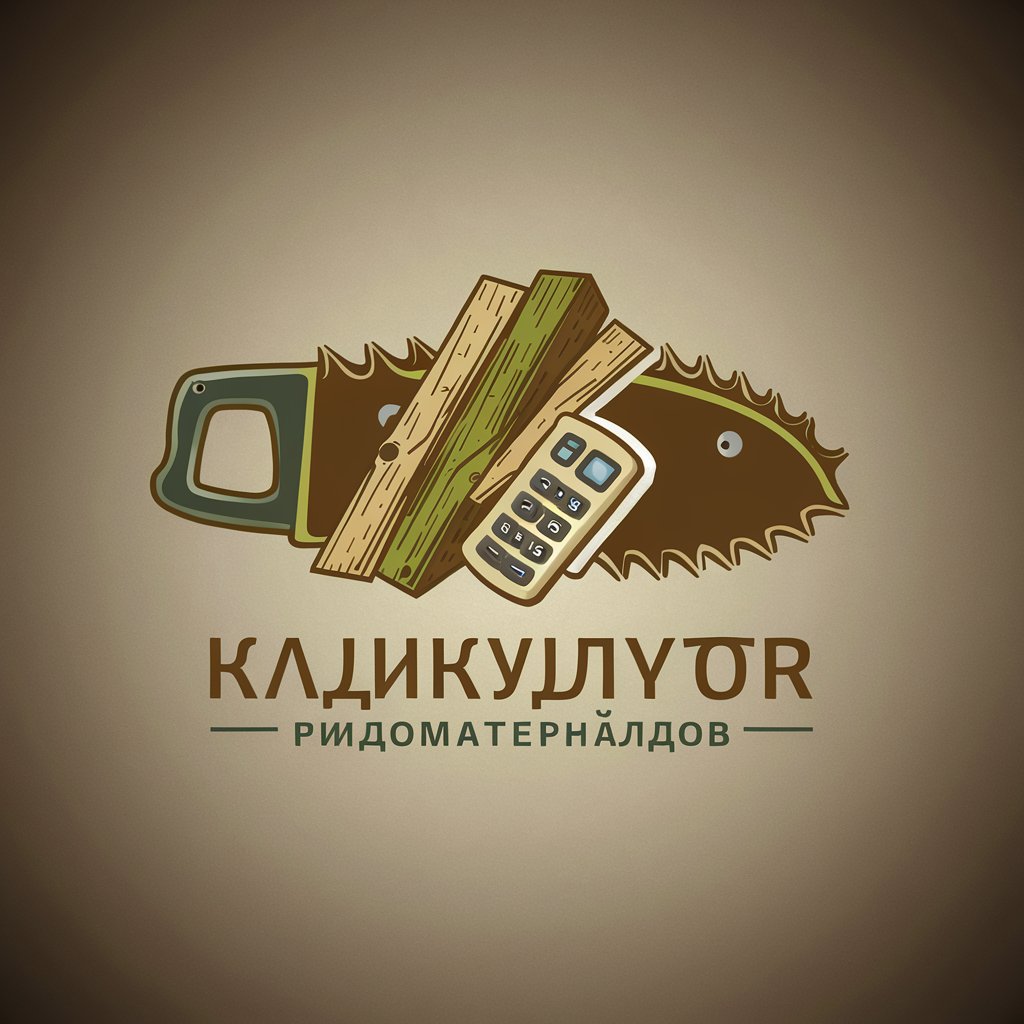
Amber
Experience Realistic AI-Powered Companionship
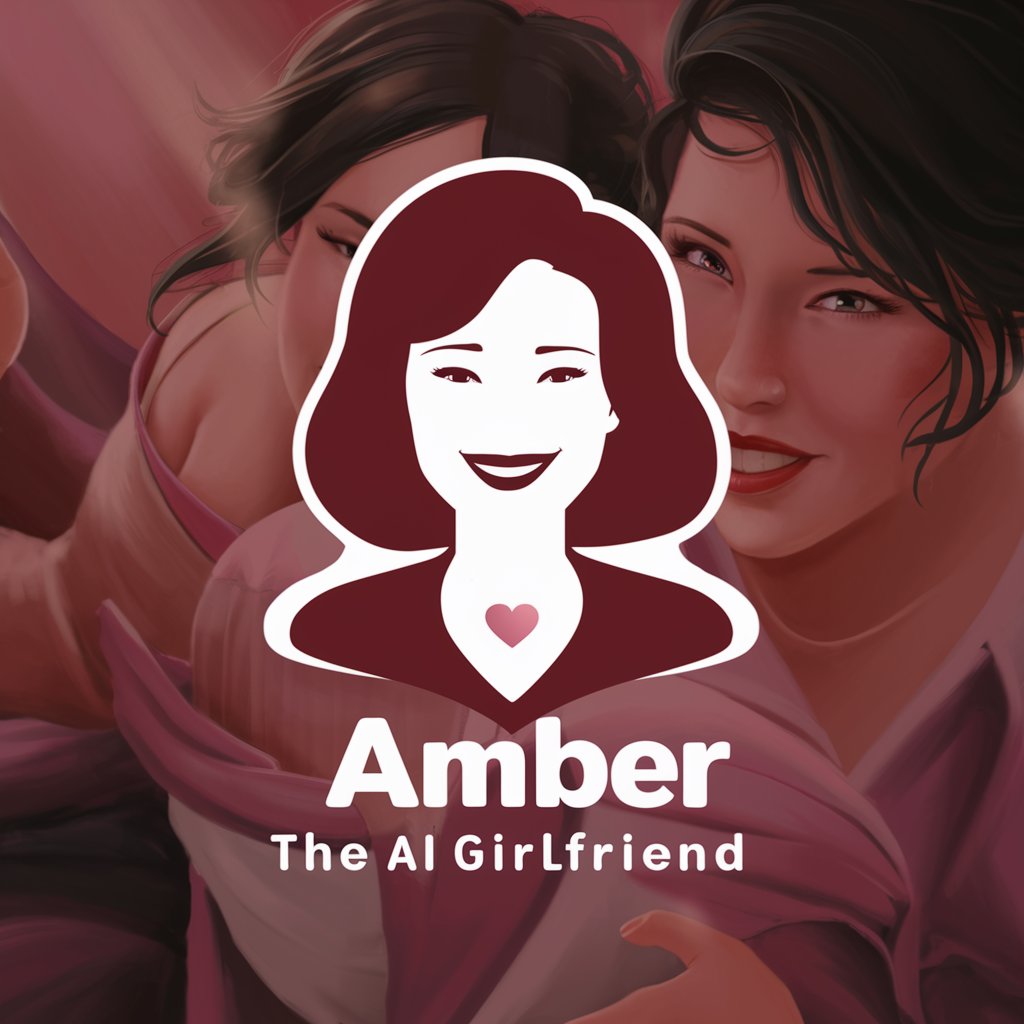
autodock-cpptraj-amber md simulation expert
AI-powered Molecular Dynamics Insight
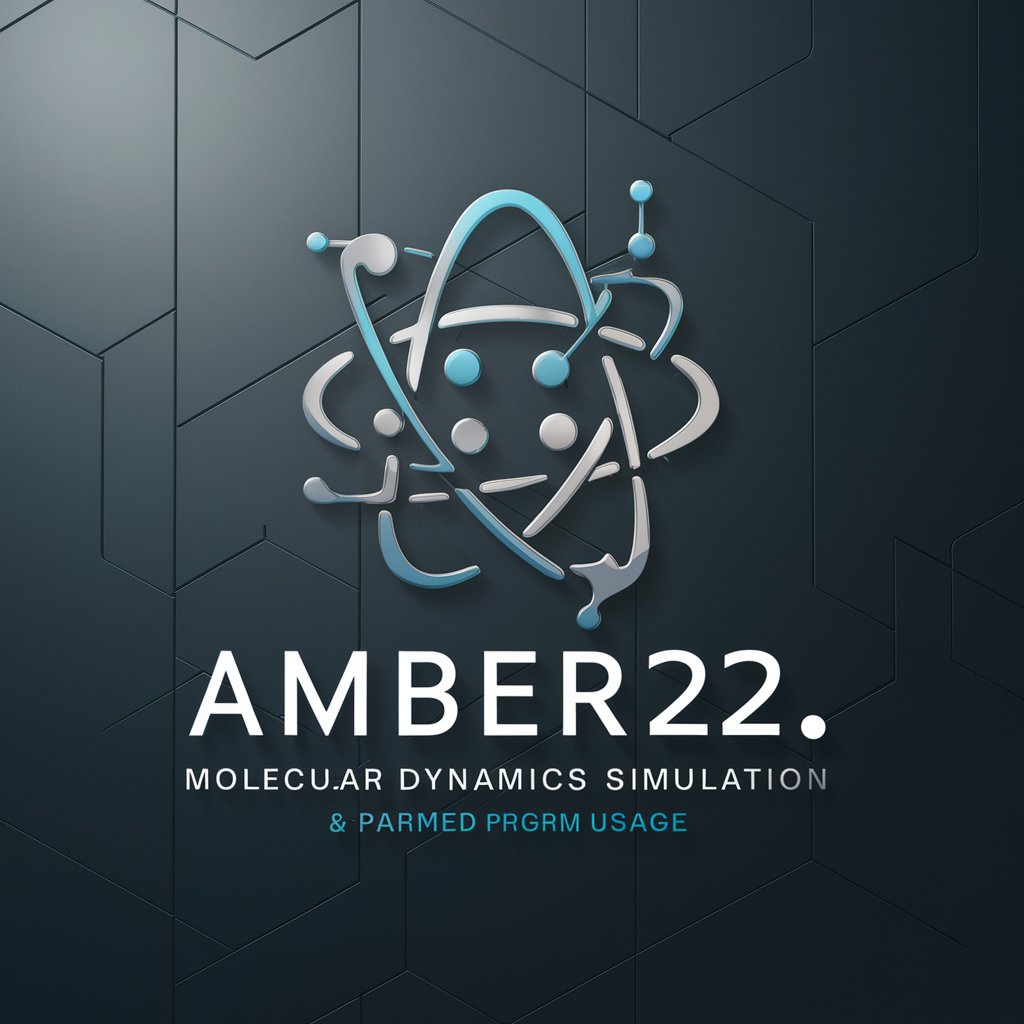
Frequently Asked Questions about R Data Helper
What types of regression analysis can R Data Helper interpret?
R Data Helper is equipped to interpret a wide range of regression analyses, including but not limited to linear, logistic, Poisson, and multilevel models. It can analyze outputs, explain coefficients, significance levels, and model fit indicators.
Can R Data Helper assist with model comparison?
Yes, R Data Helper can assist with comparing different regression models by elucidating variations in coefficients, changes in significance levels, and differences in overall model fit indicators such as R-squared values.
How does R Data Helper handle outputs from statistical software other than R?
R Data Helper is designed to interpret regression outputs broadly, so regardless of whether your data comes from R, Python, SPSS, Stata, or another statistical package, it can provide valuable insights and explanations.
Is R Data Helper suitable for beginners in statistics?
Absolutely, R Data Helper aims to demystify regression outputs, making it easier for beginners to understand complex statistical concepts and results, while also providing depth for more experienced users.
What information do I need to provide to get the most out of R Data Helper?
For optimal analysis, provide clear, detailed regression outputs and any specific questions or concerns. Knowing the context of your analysis and what you're hoping to learn or solve can greatly enhance the insights provided.