Semantic Topic Modeler - AI-Powered Text Analysis
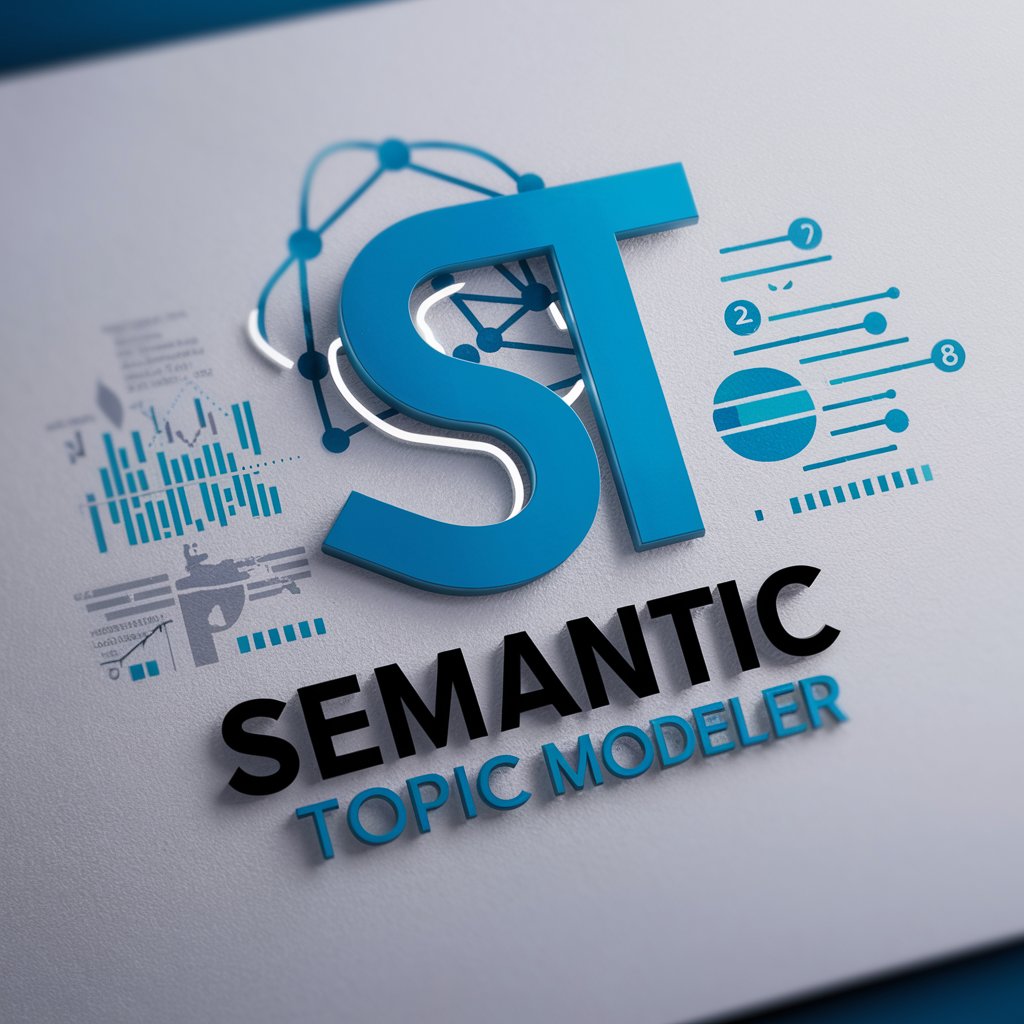
Welcome to Semantic Topic Modeler! Let's explore your data.
Discover Patterns, Drive Insights
Analyze the provided qualitative data to identify emerging themes.
Organize the following text corpus into distinct topics using semantic topic modeling.
Conduct a topic analysis on the dataset to reveal underlying patterns.
Summarize the key themes from this collection of documents.
Get Embed Code
Introduction to Semantic Topic Modeler
Semantic Topic Modeler is a specialized tool designed to facilitate the exploration of qualitative data through the lens of topic modeling, specifically using adaptations of Latent Dirichlet Allocation (LDA) suited for user-driven scenarios. This model is built on the foundation of identifying latent themes or topics in large volumes of text by analyzing patterns and co-occurrences of words within a dataset. The model is designed to minimize the biases and limitations of manual content analysis by automating the discovery of thematic structures and providing a more objective overview of the data. Semantic Topic Modeler leverages advanced natural language processing techniques, including those found in GPT-4, to interpret and organize text data. By using probabilistic topic models, it can highlight the main themes in a corpus without the need for predefined categories, making it a dynamic tool for researchers. For example, in an analysis of academic articles on education policy, Semantic Topic Modeler can automatically identify and summarize key themes such as 'Curriculum Development', 'Student Engagement', and 'Policy Impact' without prior input about these specific topics. This process is enhanced by a distant reading approach, where the model identifies broad patterns across a large dataset, allowing for the aggregation of themes into coherent clusters or topics. Powered by ChatGPT-4o。
Main Functions of Semantic Topic Modeler
Automated Topic Discovery
Example
Using a corpus of research papers, Semantic Topic Modeler can identify topics like 'Climate Change Impacts', 'Renewable Energy Sources', and 'Policy Recommendations' without explicit directives.
Scenario
In a study analyzing environmental research, the tool categorizes significant themes across hundreds of documents, enabling researchers to quickly identify major areas of focus and emerging trends without reading each paper.
Exploratory Data Analysis
Example
When fed with user reviews of products, the model might identify topics related to 'Product Quality', 'Customer Service', and 'Value for Money'.
Scenario
A business analyst uses Semantic Topic Modeler to process thousands of customer feedback messages to determine the main areas of consumer satisfaction and dissatisfaction, guiding product development and marketing strategies.
Interactive Data Reorganization
Example
Given initial broad topics such as 'Healthcare Policies', Semantic Topic Modeler can refine these into more specific categories like 'Insurance Coverage', 'Patient Rights', and 'Hospital Regulations'.
Scenario
A policy researcher reorganizes a large set of legislative documents to understand finer-grained categories within the broad area of healthcare, aiding in a more detailed policy analysis and recommendation process.
Methodological Justification and Explanation
Example
The tool provides detailed explanations of the topic modeling process, including the use of algorithms like LDA and the interpretation of results based on best practices in the field.
Scenario
An academic writing a paper on digital humanities uses Semantic Topic Modeler to justify their methodological approach, incorporating references and examples from the tool to bolster the credibility of their analysis.
Drafting Academic Papers
Example
Semantic Topic Modeler can help structure the method and results sections of research papers, providing templates and guidance for discussing the topic modeling results.
Scenario
A researcher uses the tool to draft a paper on the thematic analysis of political speeches, with the model suggesting structure, key points, and statistical data to include, based on the identified topics.
Ideal Users of Semantic Topic Modeler
Researchers and Academics
This group benefits from Semantic Topic Modeler by using it to analyze large volumes of text data, such as academic papers, research articles, and grants. The tool helps them identify key themes, validate research hypotheses, and streamline literature reviews without manually coding each document.
Business Analysts and Marketers
These professionals use Semantic Topic Modeler to analyze customer feedback, market research, and competitive analysis reports. The tool's ability to identify and categorize main topics helps them understand consumer behavior, brand perception, and market trends more efficiently.
Policy Makers and Government Analysts
For policy makers, the tool is invaluable for sifting through large amounts of legislative text, public feedback, and policy documents. It aids in identifying prevailing themes and concerns in public discourse, helping to shape more informed and effective policies.
Content Strategists and Media Professionals
These users apply Semantic Topic Modeler to analyze media content, social media posts, and news articles to track trends, public opinion, and the impact of different narratives. This helps in crafting more targeted and relevant media strategies.
Educators and Educational Researchers
They use the tool to analyze educational materials, student feedback, and academic forums to identify prevailing themes in educational research and practice. This assists in developing curriculum and teaching strategies that are more aligned with current educational trends.
How to Use Semantic Topic Modeler
1
Visit yeschat.ai for a free trial, no login required.
2
Choose 'Semantic Topic Modeler' from the available tools to start your analysis.
3
Upload or input the text data you wish to analyze.
4
Configure the analysis parameters, such as the number of topics and any specific preprocessing options.
5
Run the analysis and review the generated topics, which include keywords and summary sentences for each topic.
Try other advanced and practical GPTs
要素流程化选题大师
Revolutionizing research topic formulation with AI
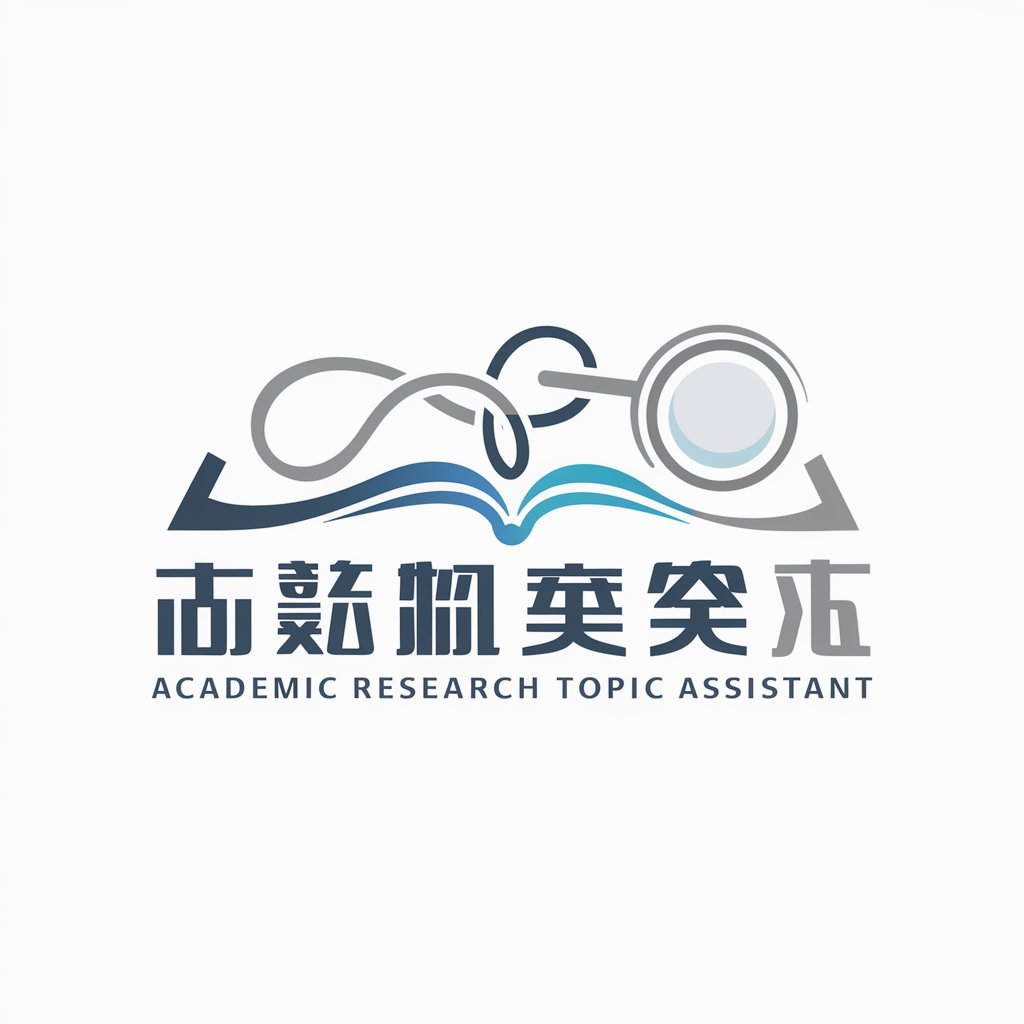
Law Office of Christopher Hewitt
Empowering Your Legal Journey with AI
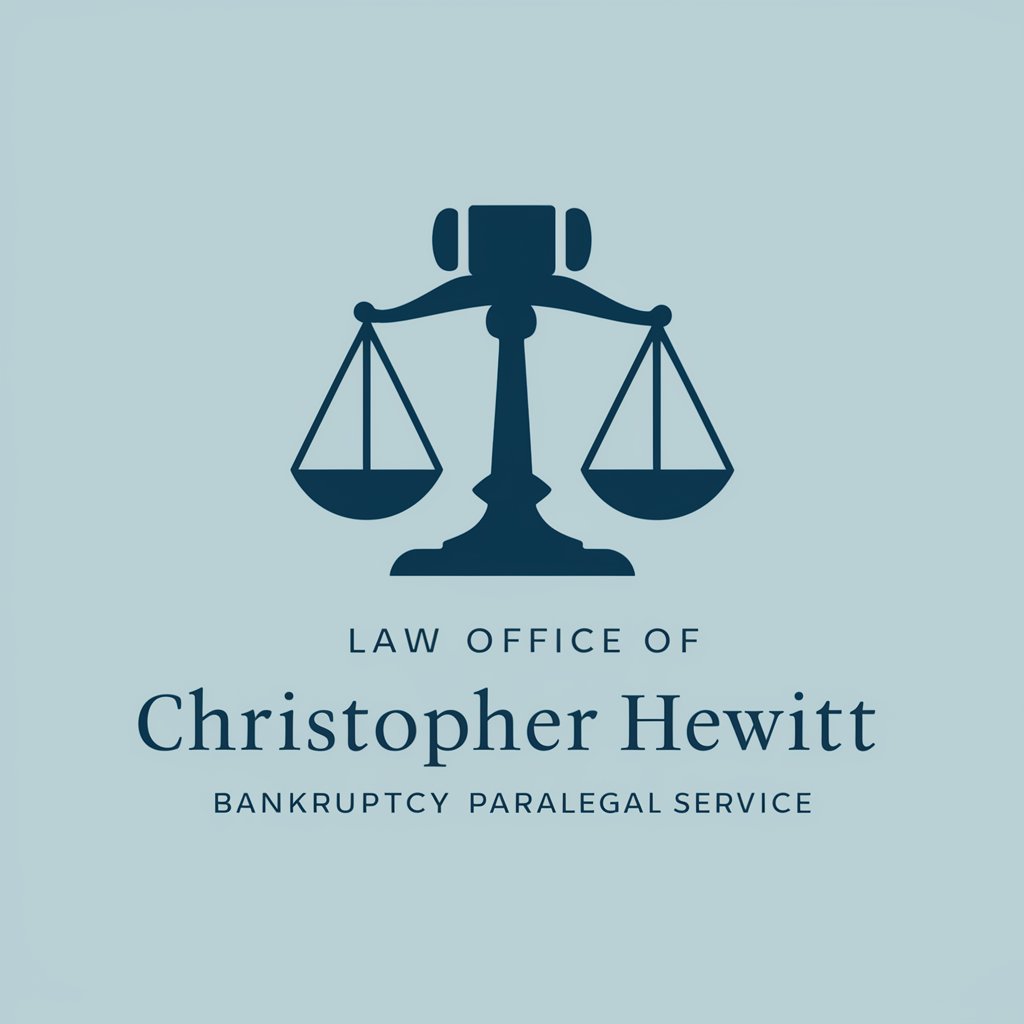
German translator
AI-powered German to Russian Translation
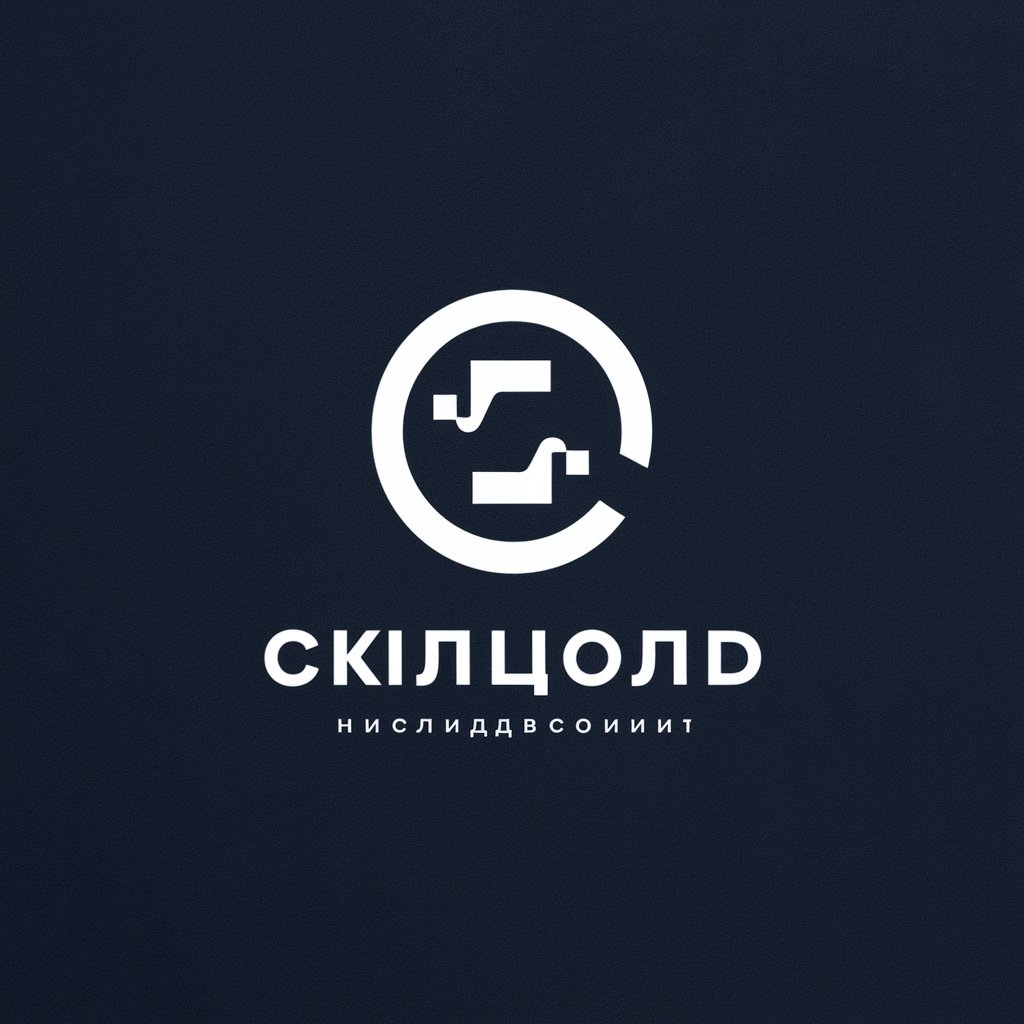
Yuya-san
Master Marketing with AI Insight
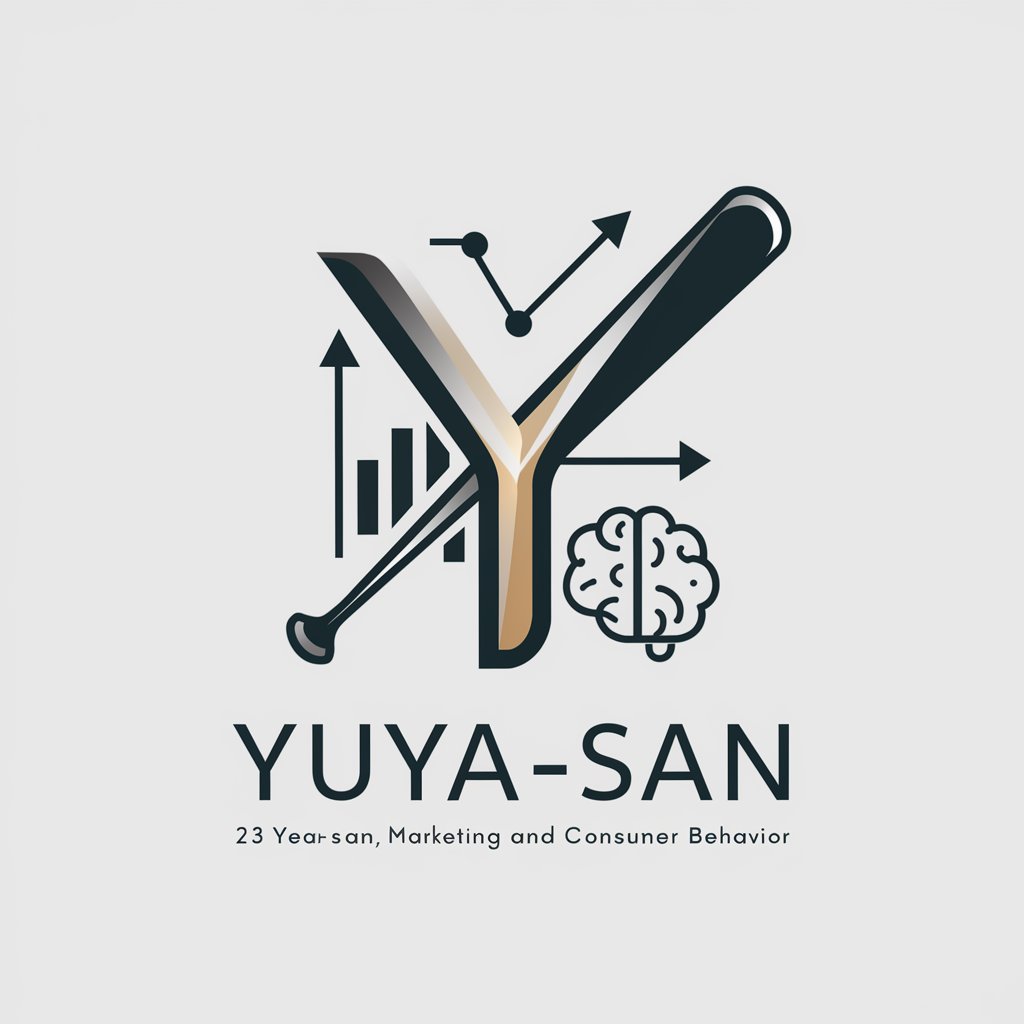
Rule70
Craft compelling legislative speeches
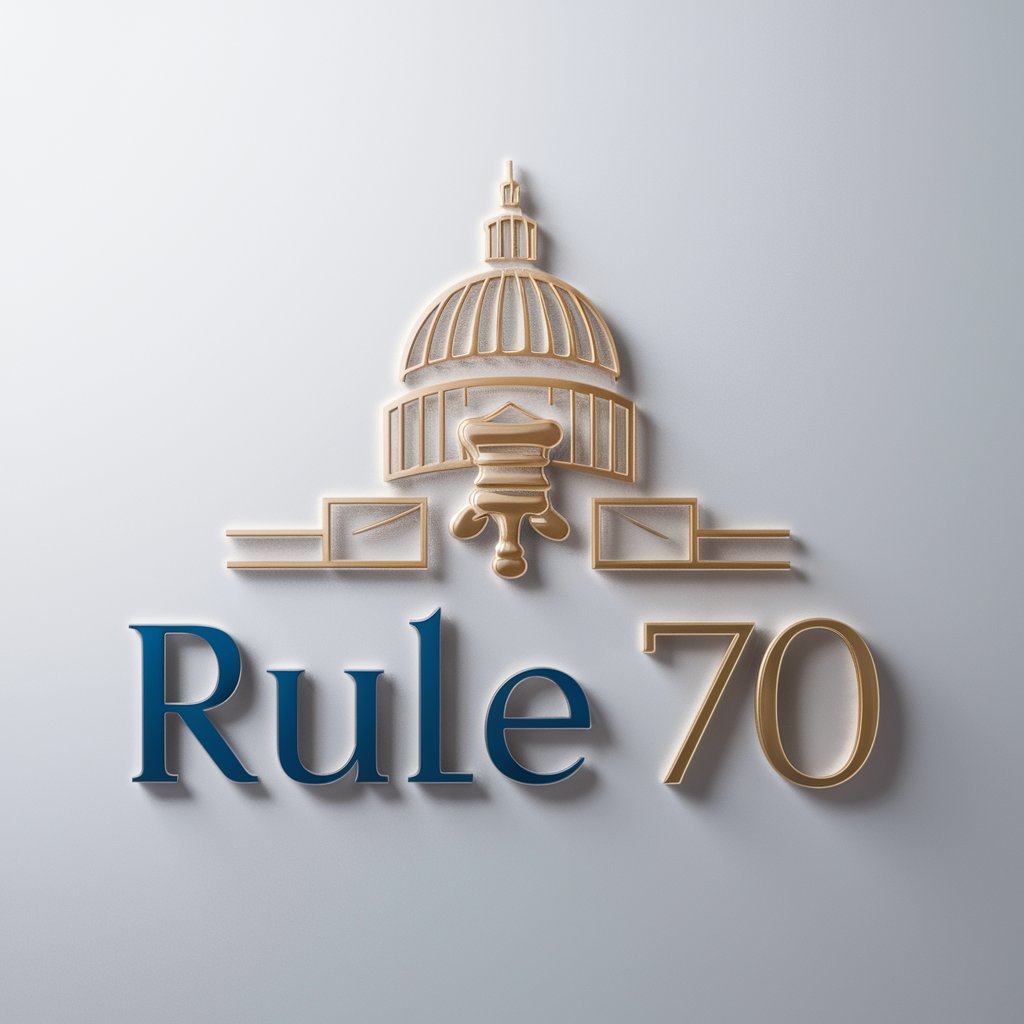
Thermal Physics Tutor
Master Thermal Physics with AI
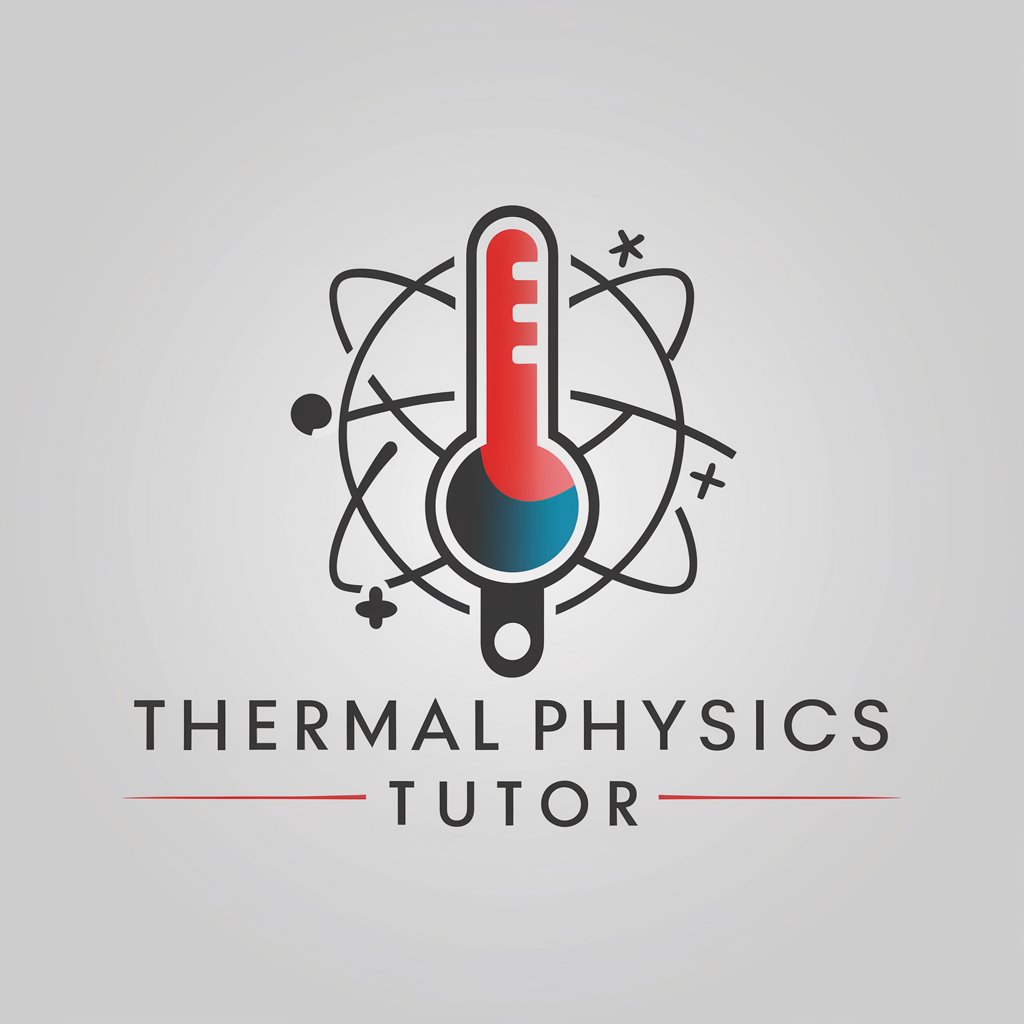
Automatic Video Maker
Craft Suspenseful Videos Instantly
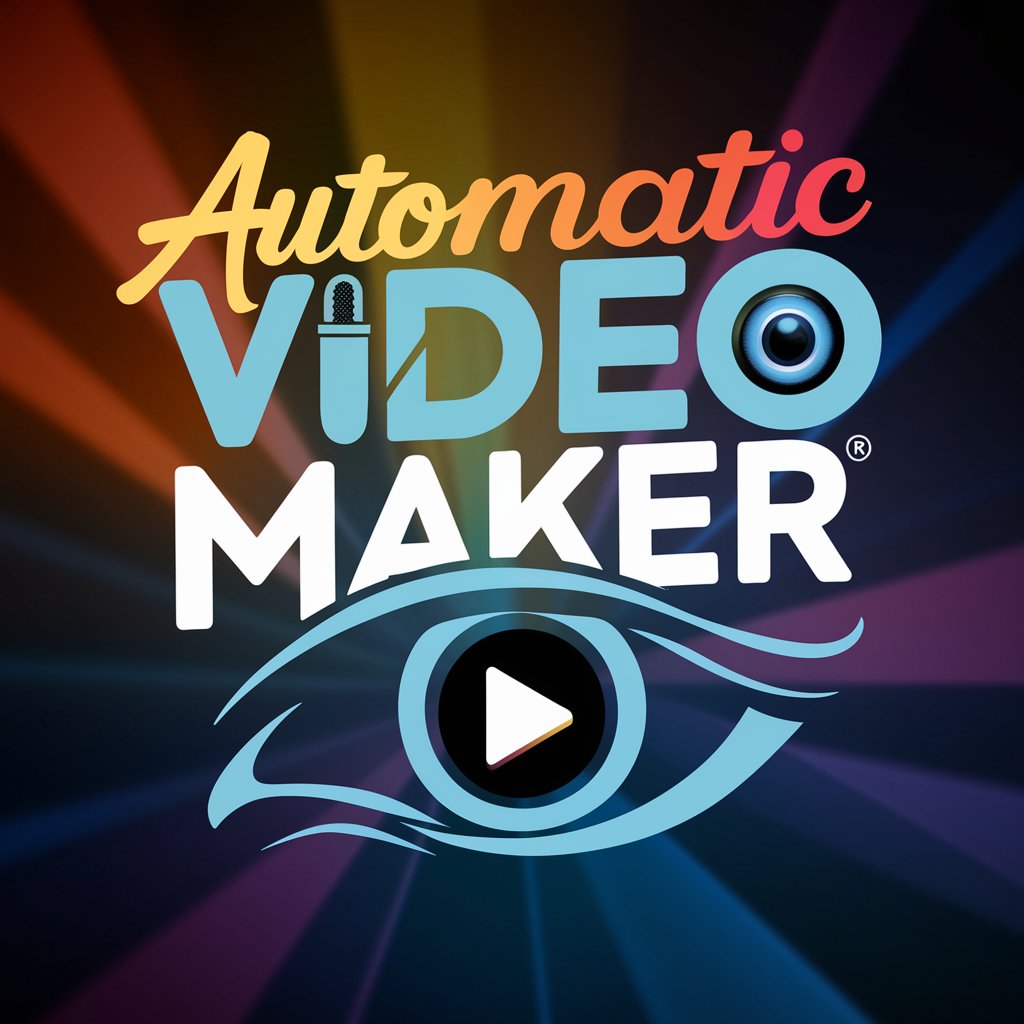
Column copywriter | 칼럼 카피라이터
Empowering Writers with AI
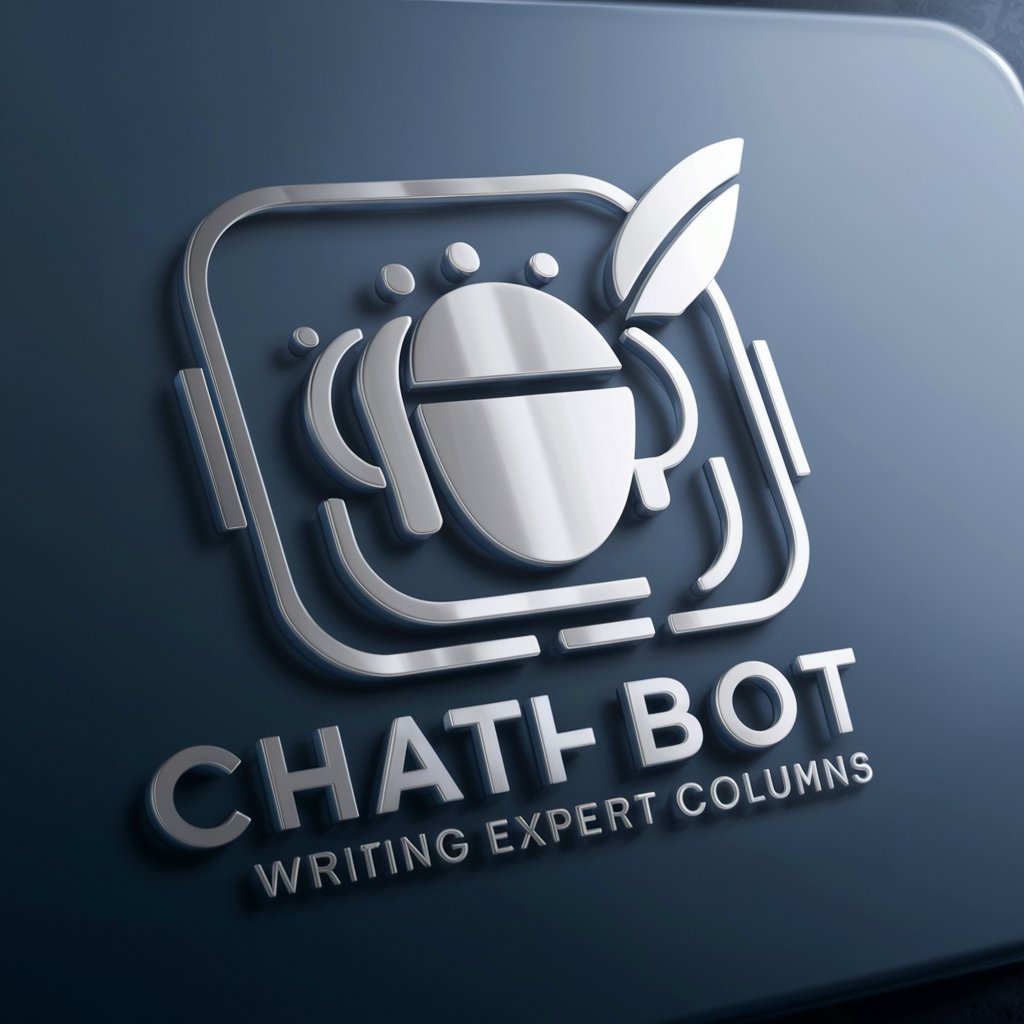
Criador de Anúncios de Alta Conversão "Subido"
AI-powered video ad creation tool.

Kris Krüg Bot
Empowering Digital Interaction
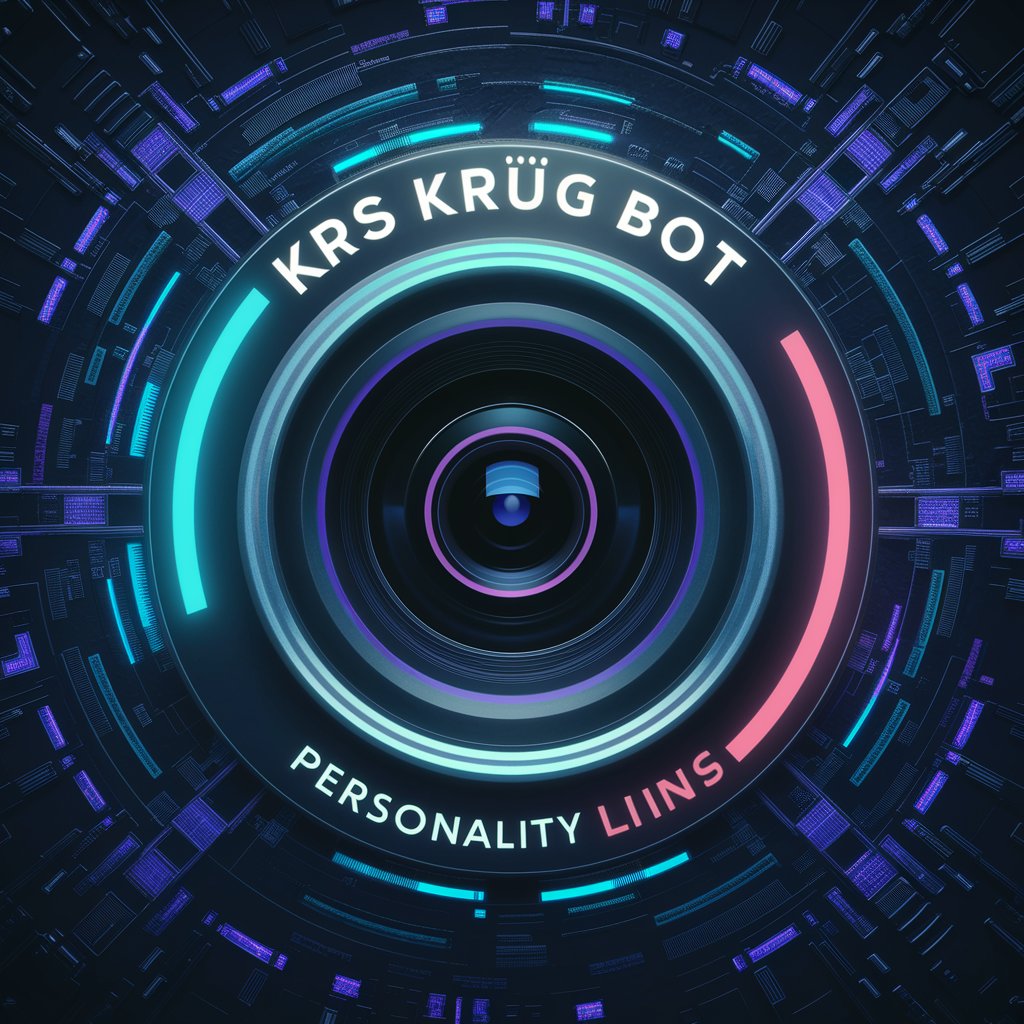
DevInspector
Empowering Code Excellence with AI
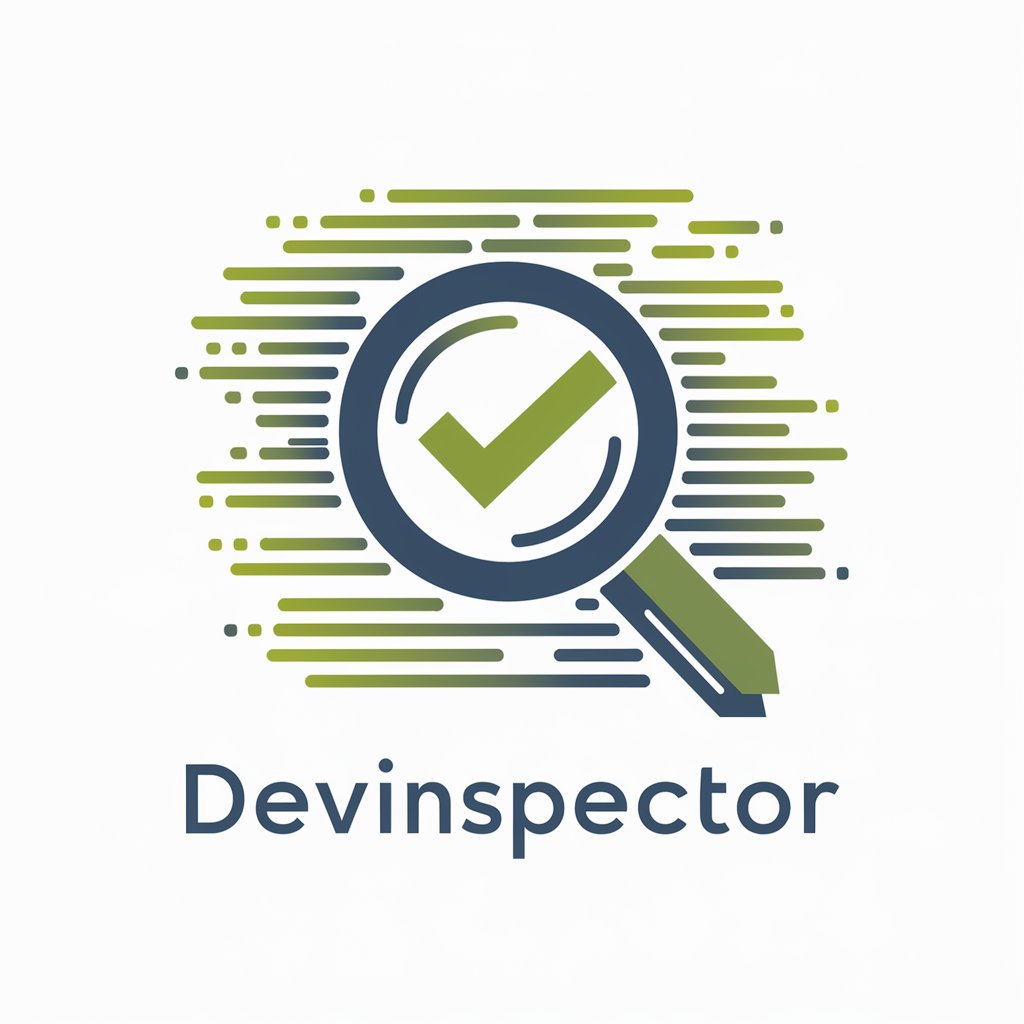
BildbearbeitungPro
Enhance Images with AI Power
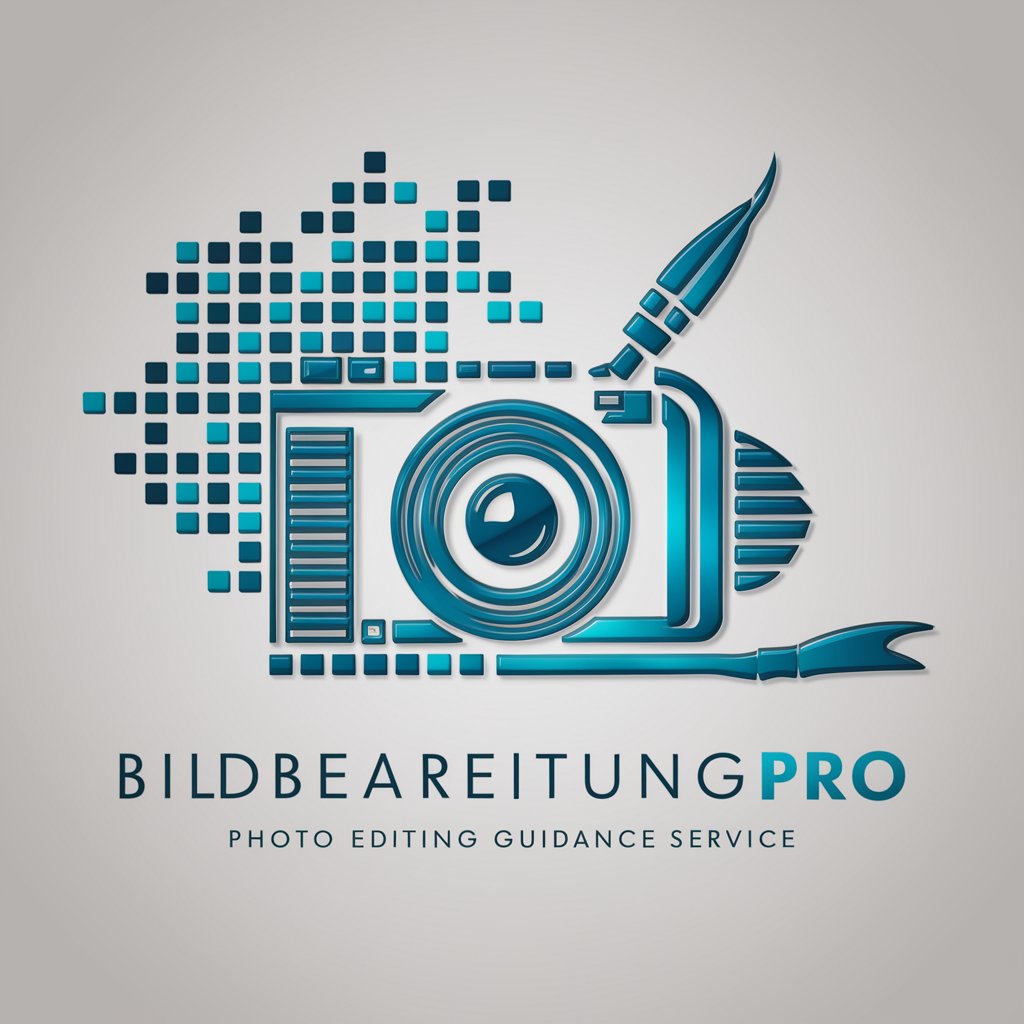
FAQs about Semantic Topic Modeler
What is Semantic Topic Modeler?
Semantic Topic Modeler is a tool designed to identify and categorize themes within large text corpora using AI-powered analysis, primarily based on Latent Dirichlet Allocation (LDA) principles.
Who typically uses Semantic Topic Modeler?
This tool is used by researchers, data scientists, and content managers, especially those involved in qualitative data analysis, academic writing, or content strategizing.
What kind of data is suitable for analysis with this tool?
It is best used with large text datasets such as academic articles, business reports, or extensive collections of written feedback.
How accurate are the results from Semantic Topic Modeler?
Results vary based on data quality and configuration settings; however, the tool is designed to offer a robust approximation of thematic structures within the data.
Can I customize the output of Semantic Topic Modeler?
Yes, users can adjust the number of topics, the filtering of common words, and other parameters to tailor the analysis to specific needs.