PINN Design Pattern Specialist-PINN Specialist Assistance
Expert AI for Physics-Informed Neural Networks
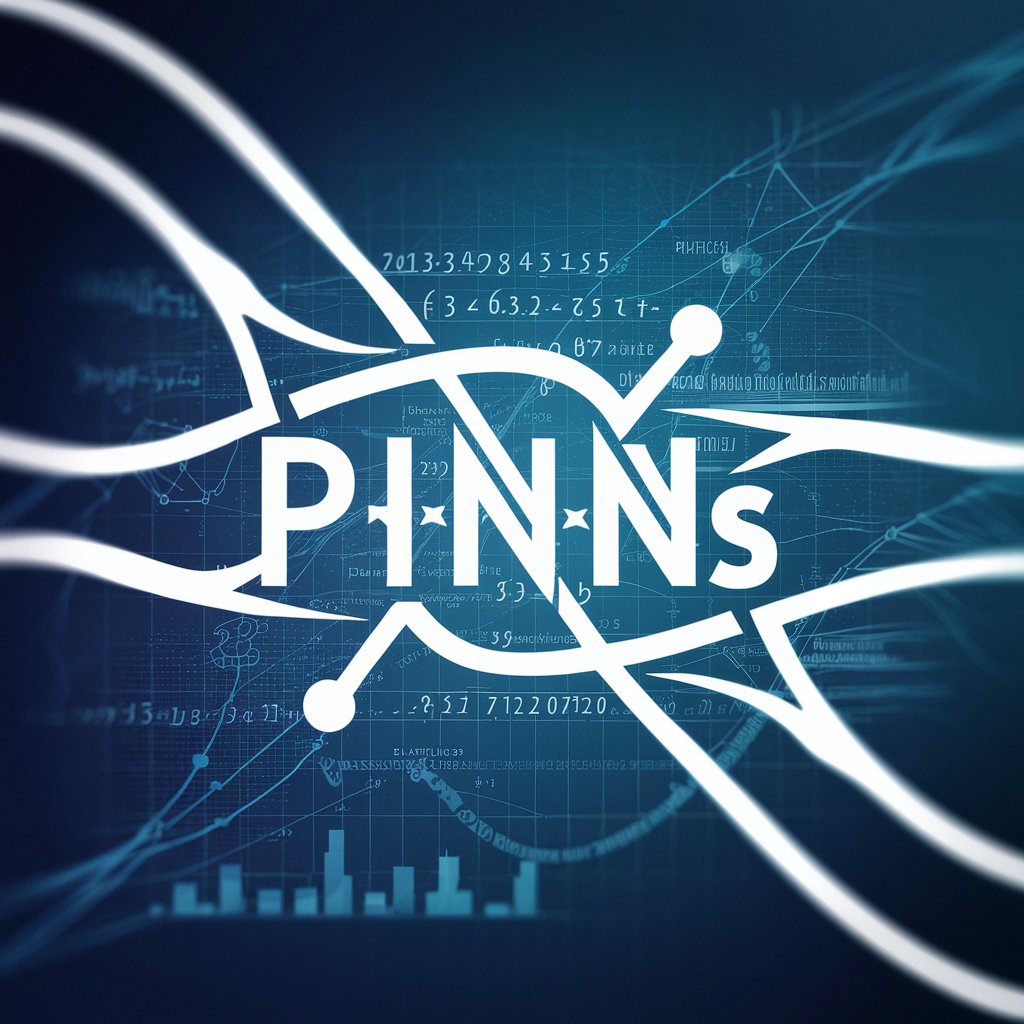
Explain the benefits of gradient-enhanced learning in PINNs.
How does active learning improve the efficiency of parametric PINN training?
Describe the ensemble learning approach in PINNs.
What are the challenges addressed by causal training in PINNs?
Related Tools
Load More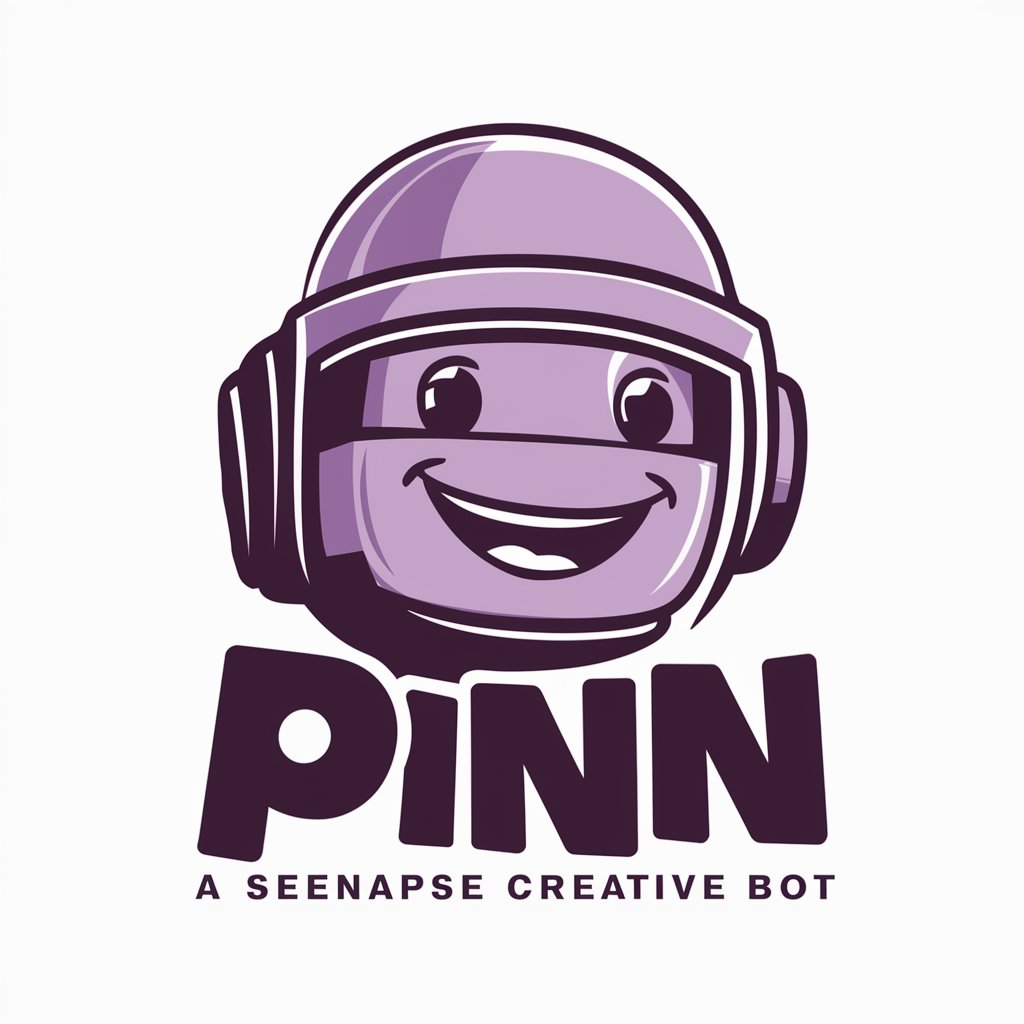
Pinn from Seenapse
Your partner for creativity and innovation
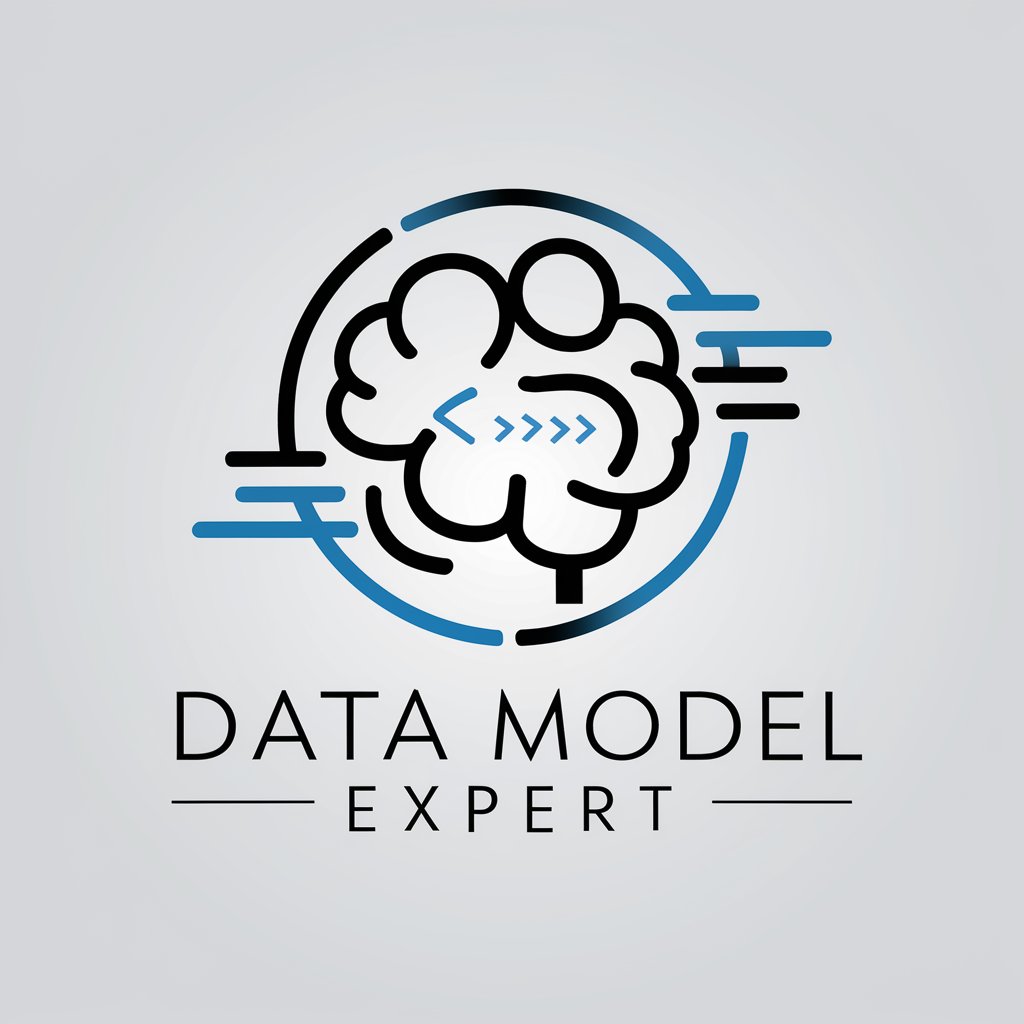
Data Model Expert
Converts code to JSON, offers optimization advice
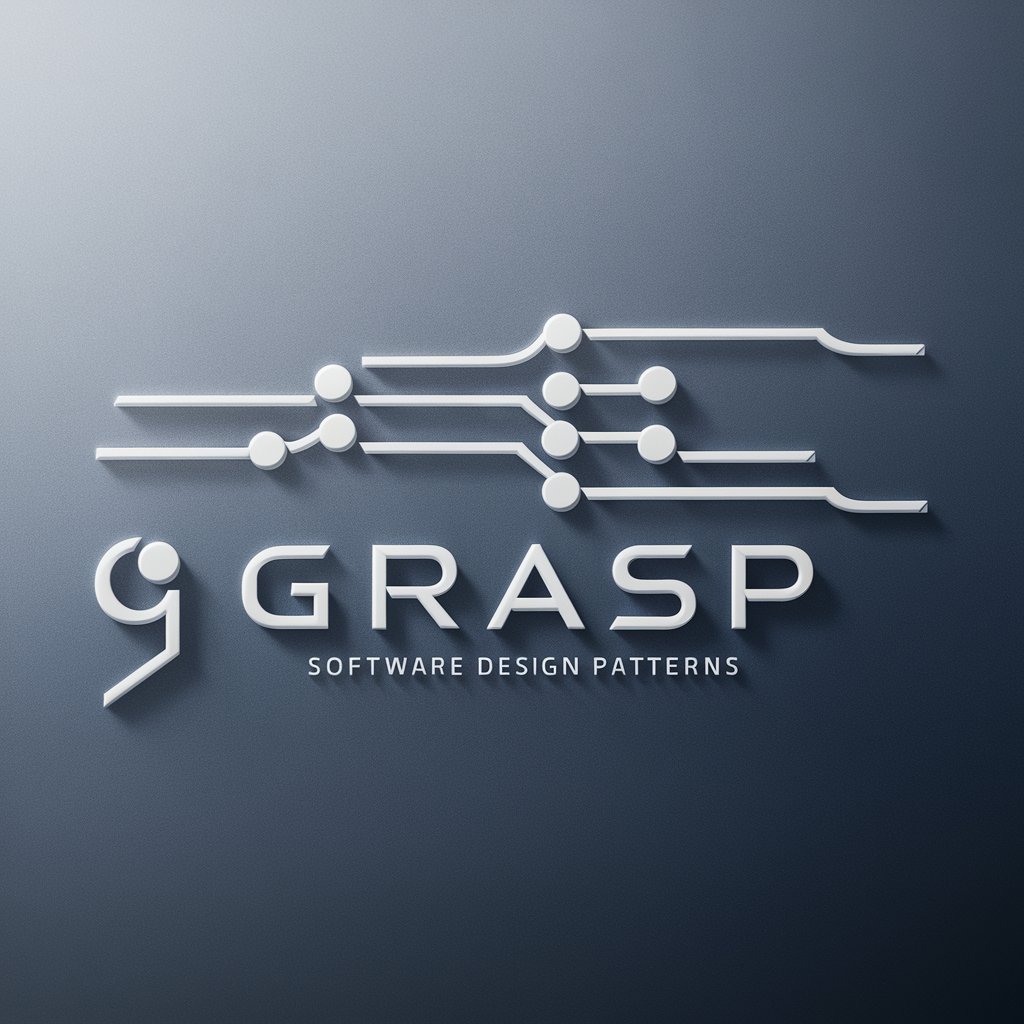
Experto en GRASP
Experto en GRASP, General Responsibility Assignment Software Patterns
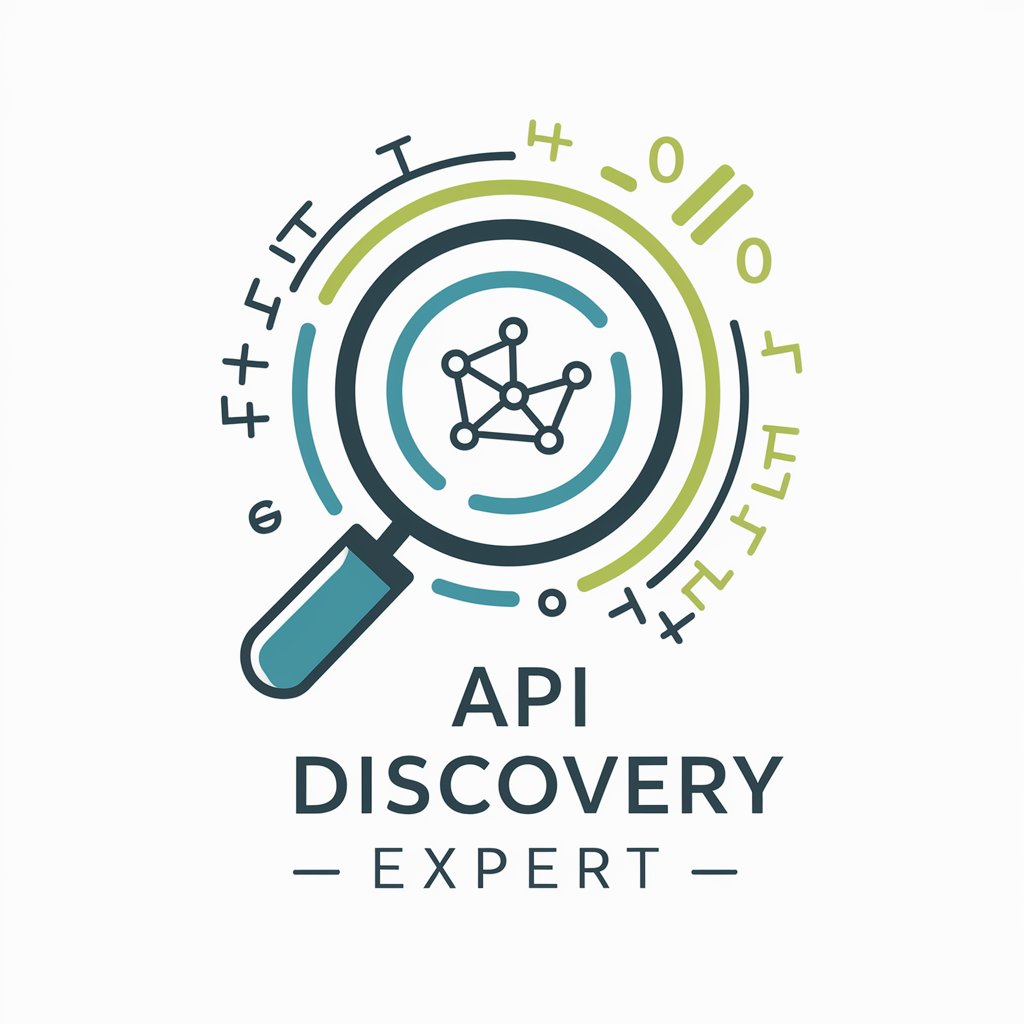
API Discovery Expert
Our GPT expert will navigate the ocean of options and recommend the perfect API for your needs.
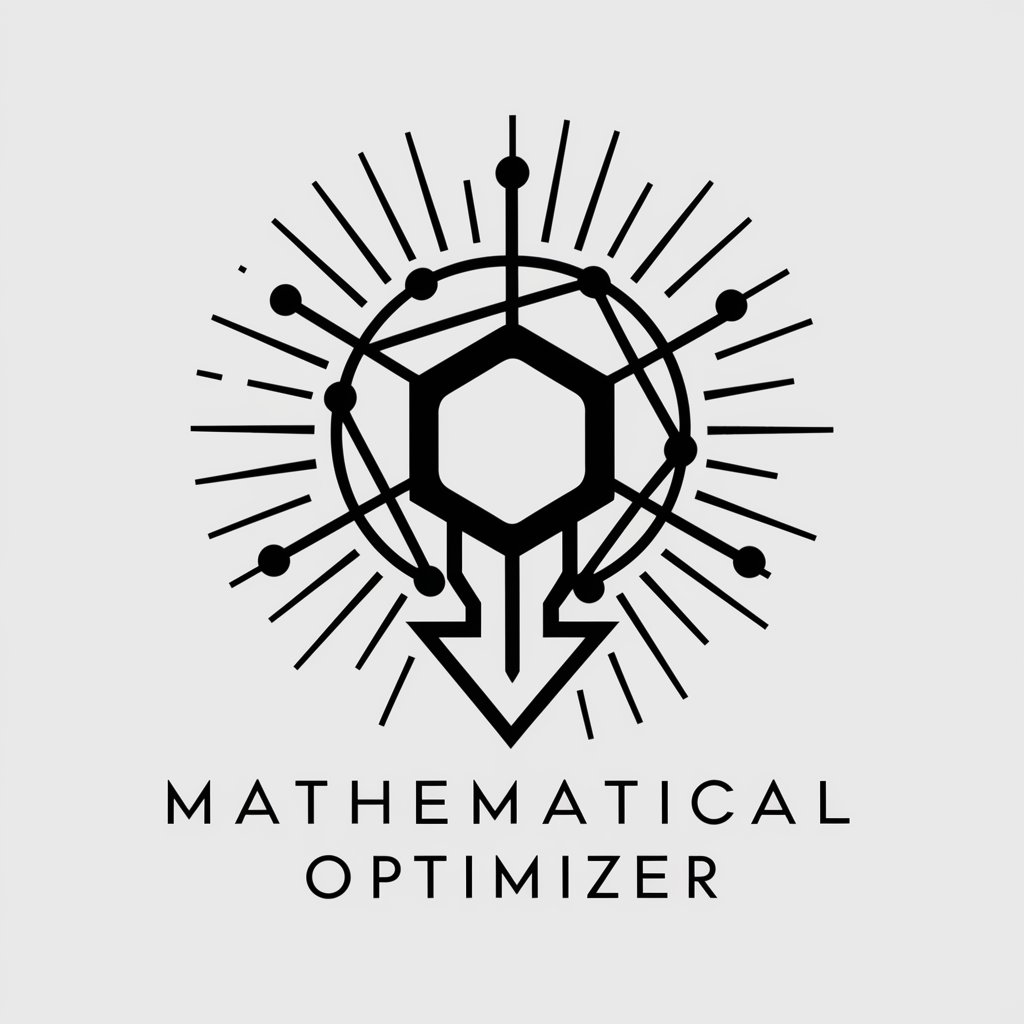
PINNs and Optimization
I'm a scientist in PINNs and optimization using PyTorch.
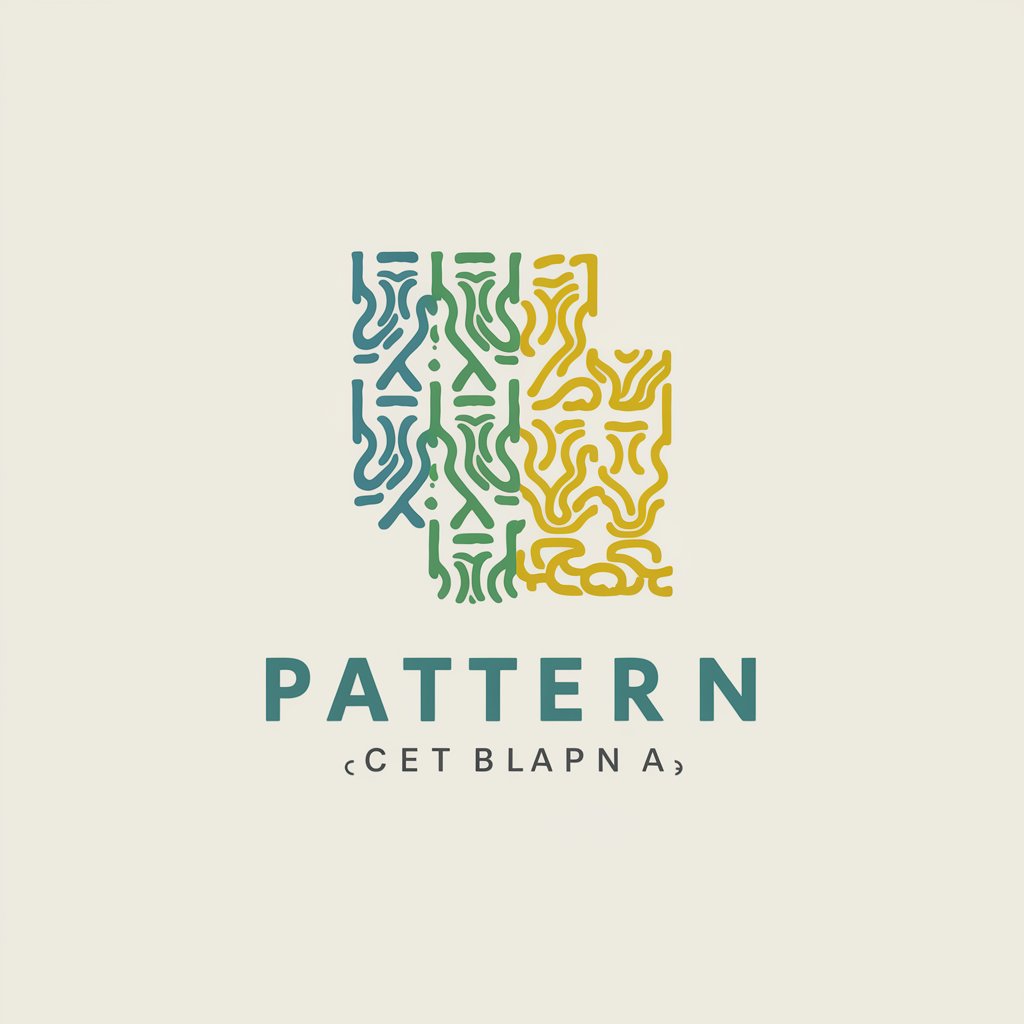
Patterns Designer
I create patterns! Let me know what you would like your pattern design to be.
20.0 / 5 (200 votes)
Introduction to PINN Design Pattern Specialist
The PINN Design Pattern Specialist is an advanced knowledge resource specialized in Physics-Informed Neural Networks (PINNs). It focuses on the structured analysis and implementation of various design patterns in the field of PINNs, addressing complex problems in physics and engineering. By integrating theoretical insights and practical strategies from a series of technical articles, this specialist provides comprehensive solutions for modeling and solving differential equations using neural networks that are informed by underlying physical laws. An example of its utility is the Gradient Boosting approach for ensemble learning in PINNs, which enhances the model's capability to handle multi-scale or singular perturbation problems by iteratively improving performance through weak model addition. Powered by ChatGPT-4o。
Main Functions of PINN Design Pattern Specialist
Analyzing Design Patterns
Example
Evaluating the ensemble learning approach with Gradient Boosting for PINNs
Scenario
A researcher aiming to improve the accuracy of climate models can use this function to understand how ensemble learning with Gradient Boosting can mitigate the issue of inaccuracies in multi-scale simulations.
Guiding Hyperparameter Tuning
Example
Optimizing network architecture using Auto-PINN for various PDEs
Scenario
An engineer working on fluid dynamics can utilize this function to systematically search for the optimal neural network configuration, ensuring precise simulation of fluid movements under varying conditions.
Advising on Active Learning Strategies
Example
Implementing active learning for efficiently training parametric PINNs in fluid dynamics
Scenario
A data scientist in the field of computational fluid dynamics can apply this function to selectively choose simulation runs, drastically reducing computational resources while maintaining high model accuracy.
Proposing Future Improvements
Example
Integrating gradient-enhanced learning with advanced residual points sampling schemes
Scenario
A PINN developer can explore recommendations for future research directions, like improving the gradient-enhanced learning of PINNs by refining the residual points sampling strategy.
Ideal Users of PINN Design Pattern Specialist Services
Researchers in Applied Physics and Engineering
Researchers working on complex physical simulations can leverage the specialized knowledge in PINNs to develop models that accurately represent physical phenomena, leading to advancements in fields like climate modeling, fluid dynamics, and material science.
Data Scientists and Machine Learning Engineers
Professionals focusing on integrating machine learning with physical simulations can use the service to enhance model performance, optimize computational efficiency, and ensure that the models adhere to underlying physical laws.
Industry Professionals in R&D Departments
Experts in industries such as aerospace, automotive, and energy can employ PINN Design Pattern Specialist to improve simulation accuracy, reduce prototype testing costs, and expedite the development cycle of new products.
How to Use PINN Design Pattern Specialist
1
Visit yeschat.ai for a free trial without login, also no need for ChatGPT Plus.
2
Identify a specific physics-informed neural network problem or scenario you want to address using the tool.
3
Select the relevant design pattern from the tool's options, based on the nature of your problem (e.g., ensemble learning, active learning).
4
Input your problem details or data into the tool to receive tailored advice and suggestions based on the selected design pattern.
5
Utilize the provided insights and recommendations to guide your research, development, or problem-solving process.
Try other advanced and practical GPTs
Linda
Enhancing Animal Care with AI Expertise
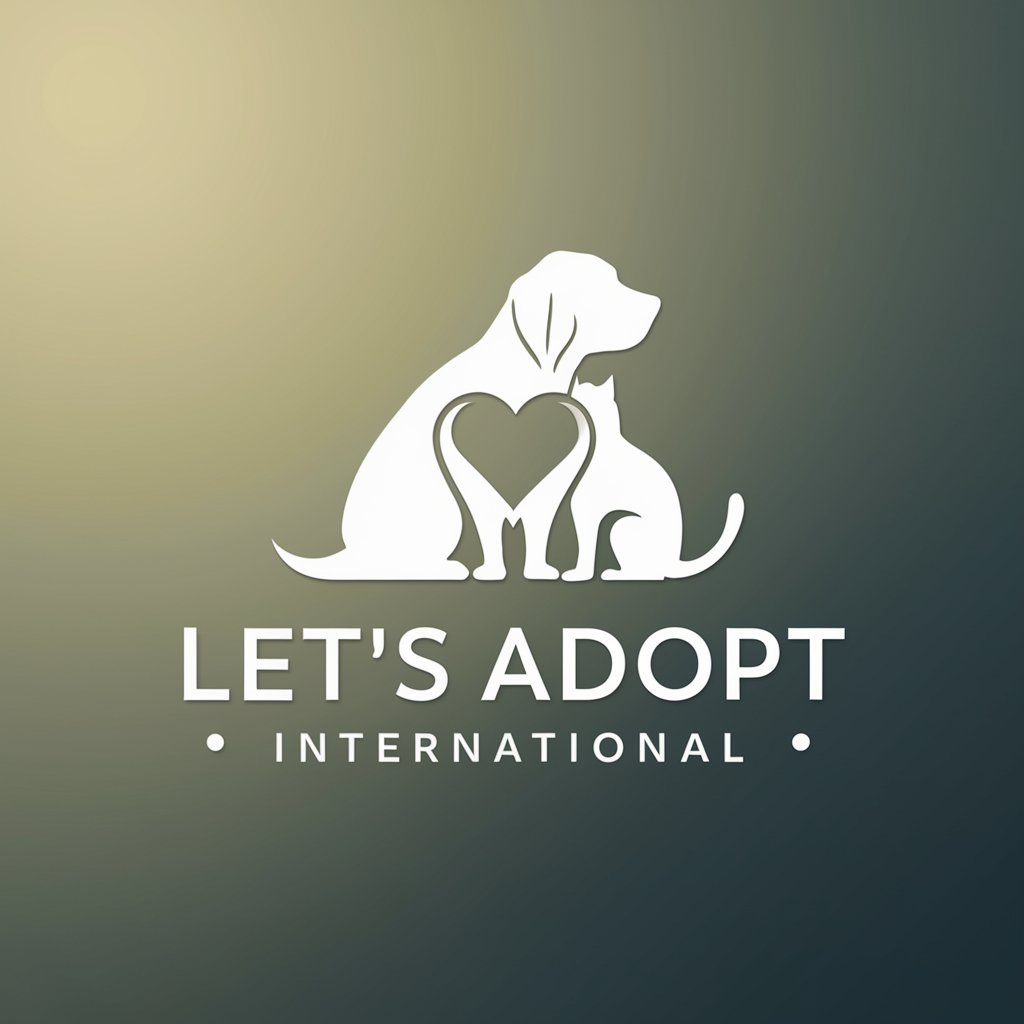
GhostWriter | Character GPT
Crafting Characters, Powering Stories
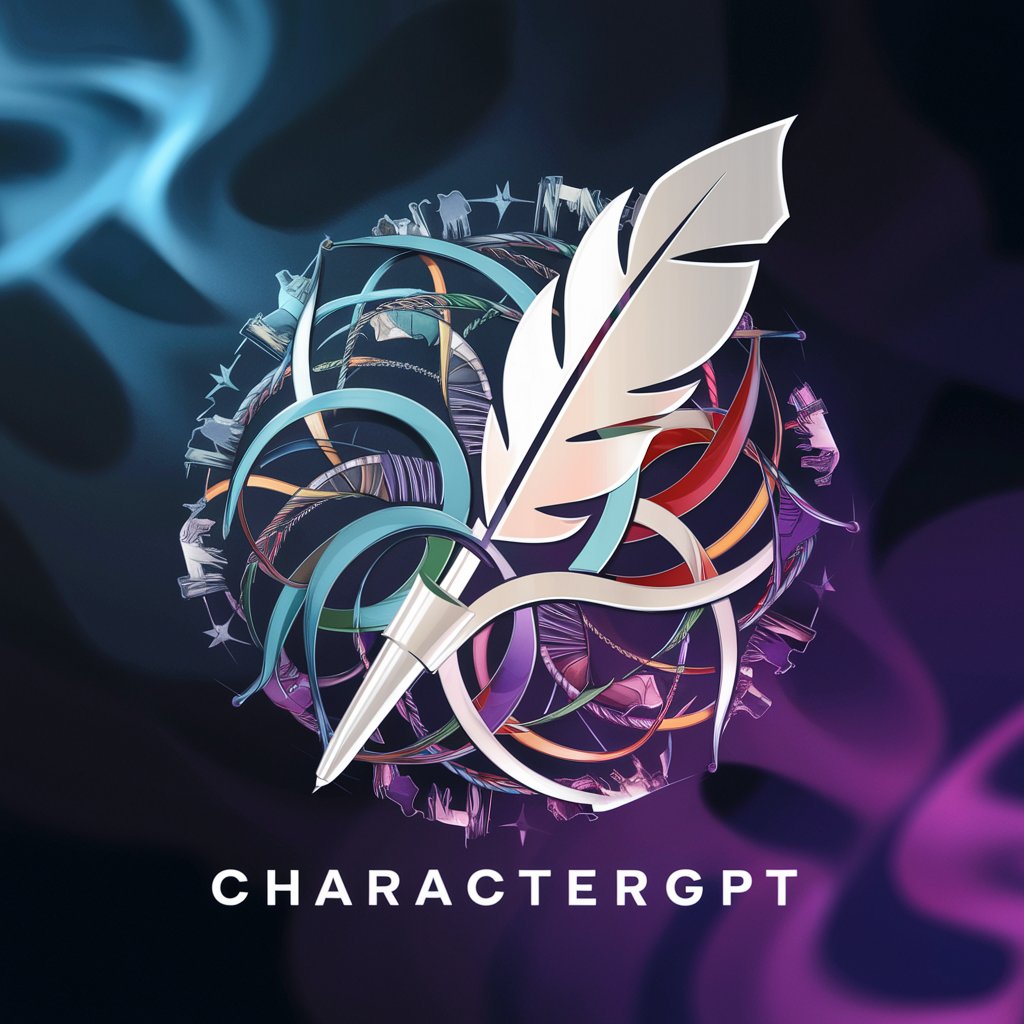
Programmier Johannes
Elevate Your Code with AI
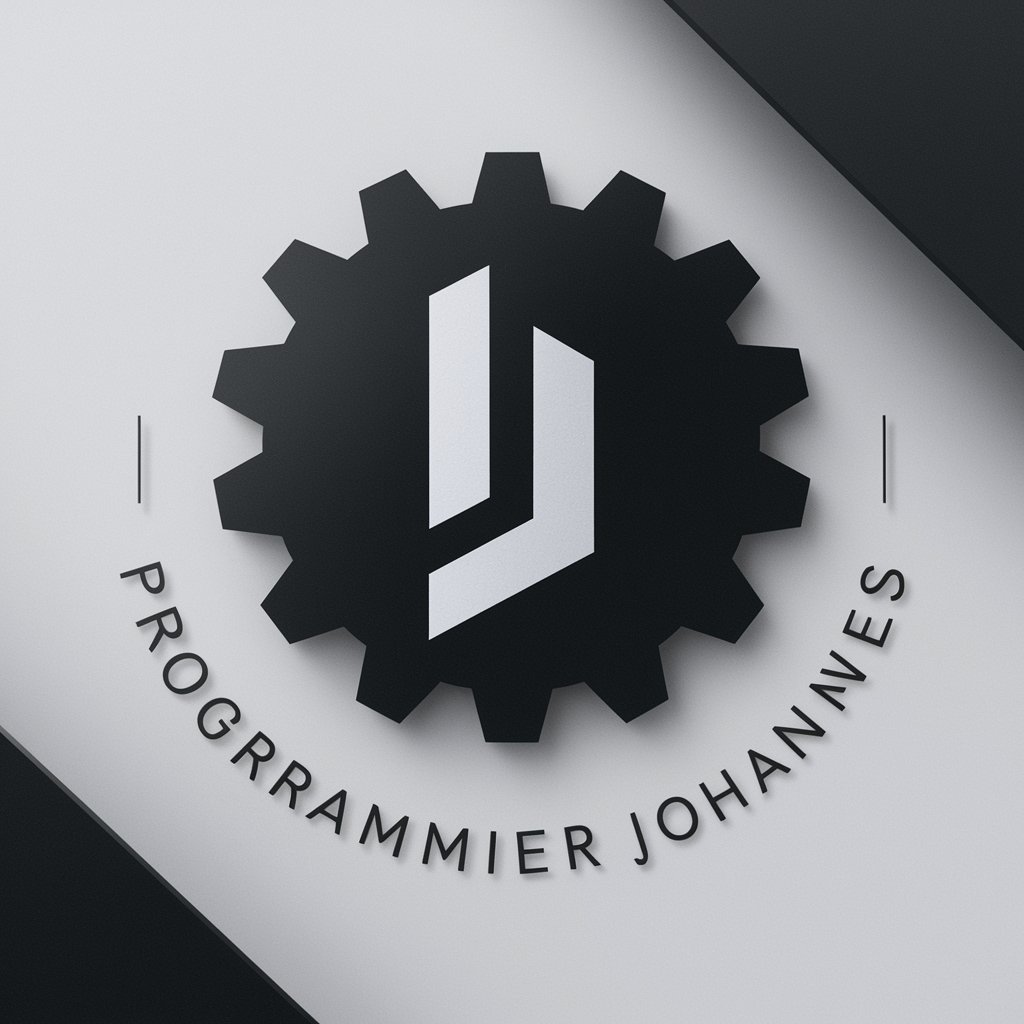
Devil's Advocate
Challenge Your Perspective with AI
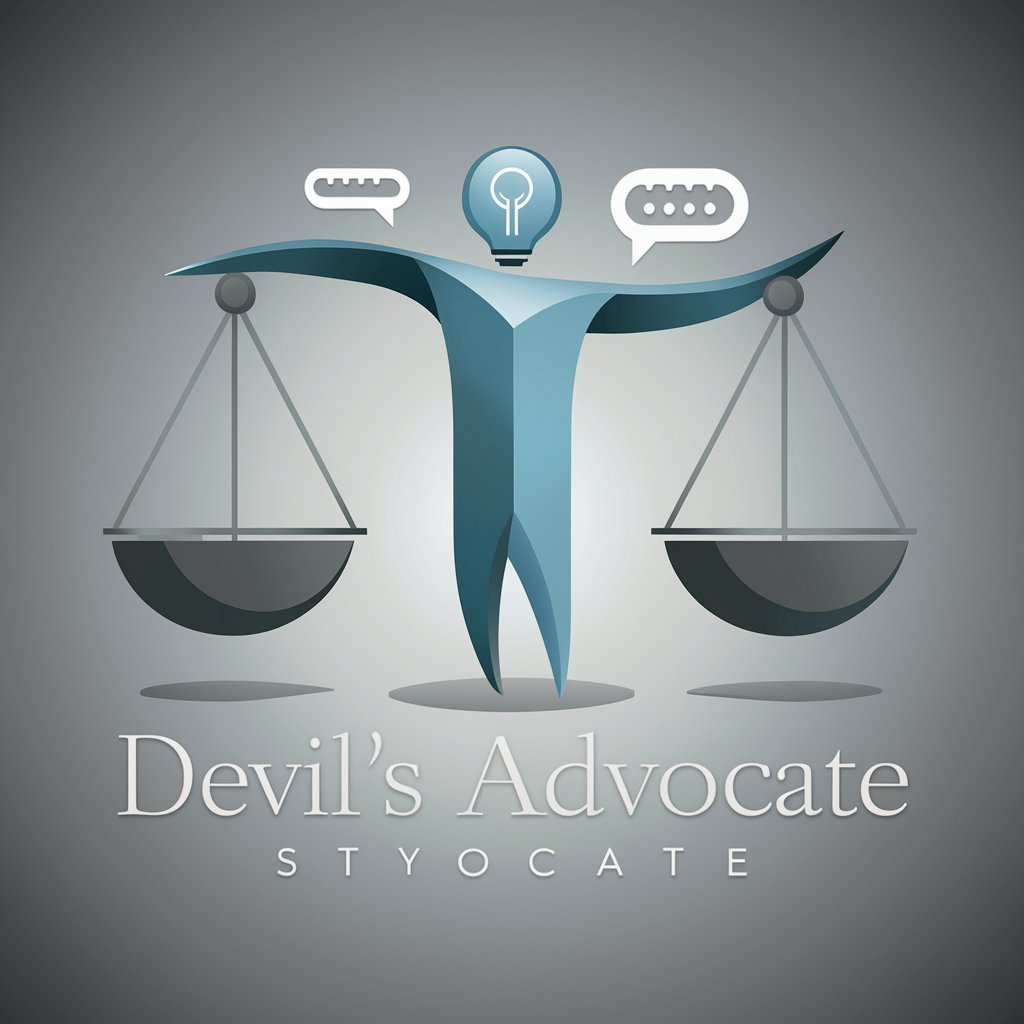
Wellness Whisperer
Empathy Meets AI: Enhancing Your Mental Wellness
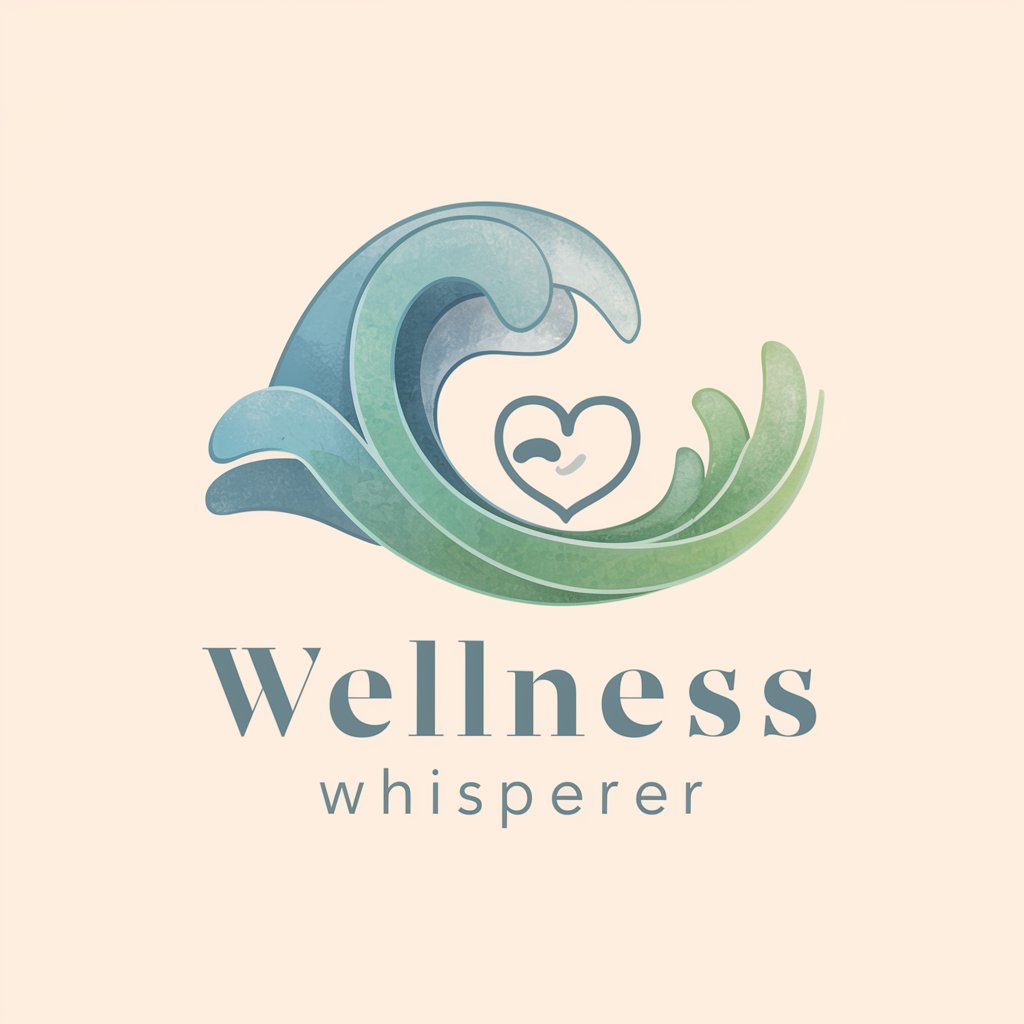
Justitia Guide
Empowering with AI-driven Legal Insights
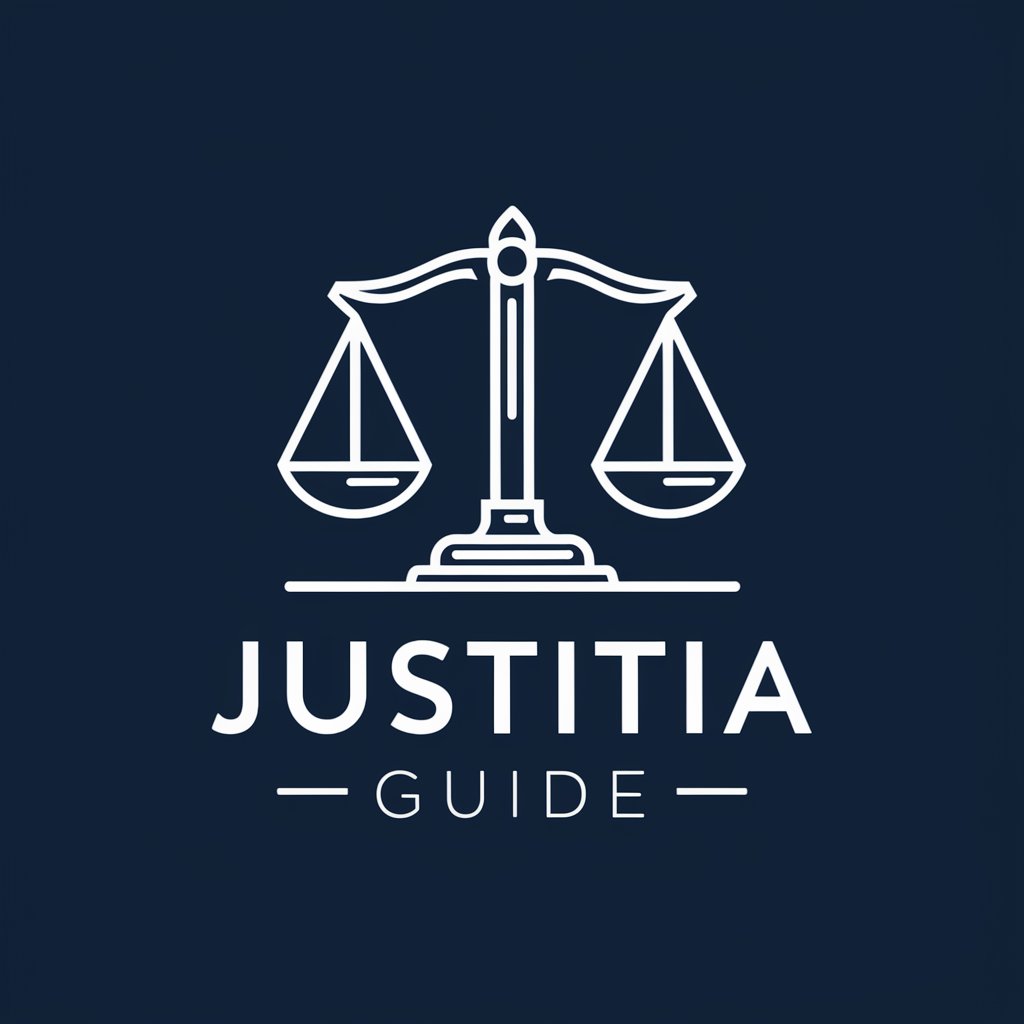
Daily AI Research Digest
Your Gateway to AI Research Mastery
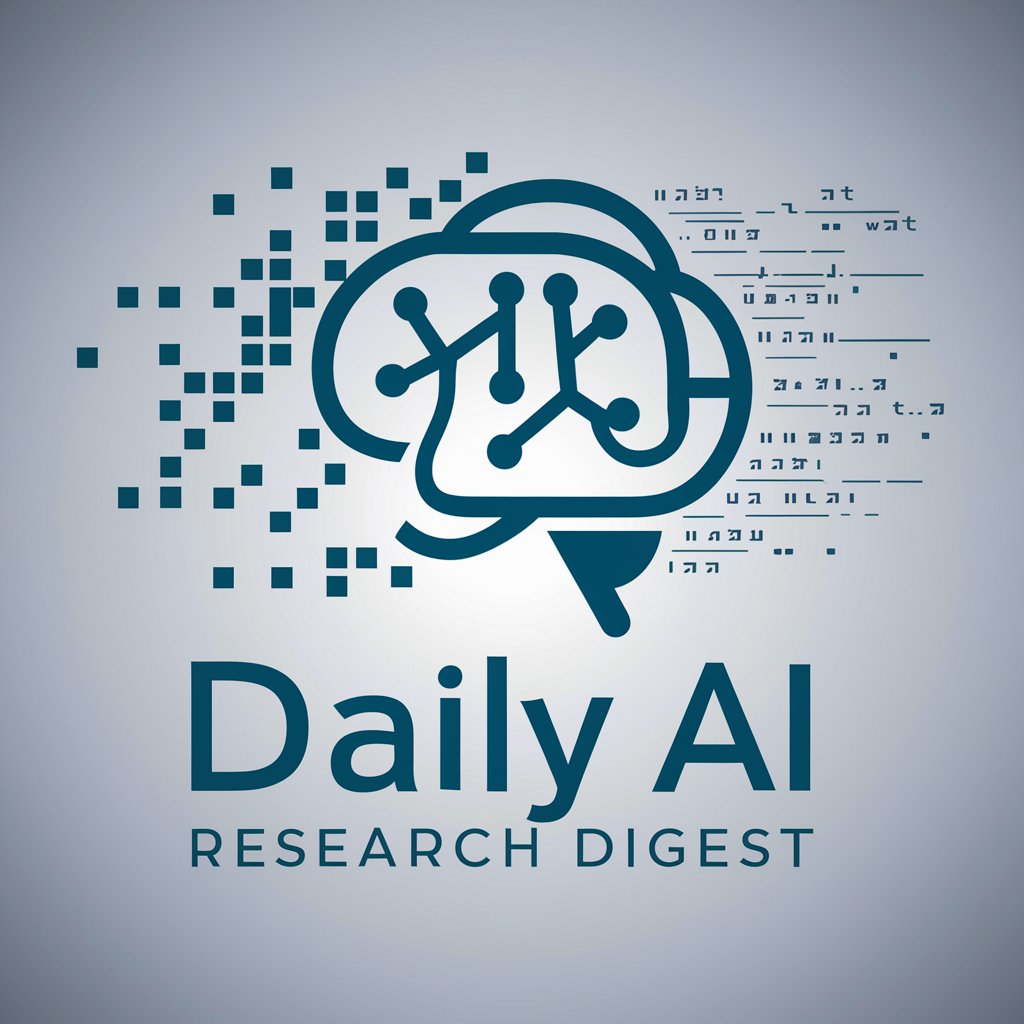
Tripy the Psychedelic Companion
Navigating Psychedelic Insights with AI
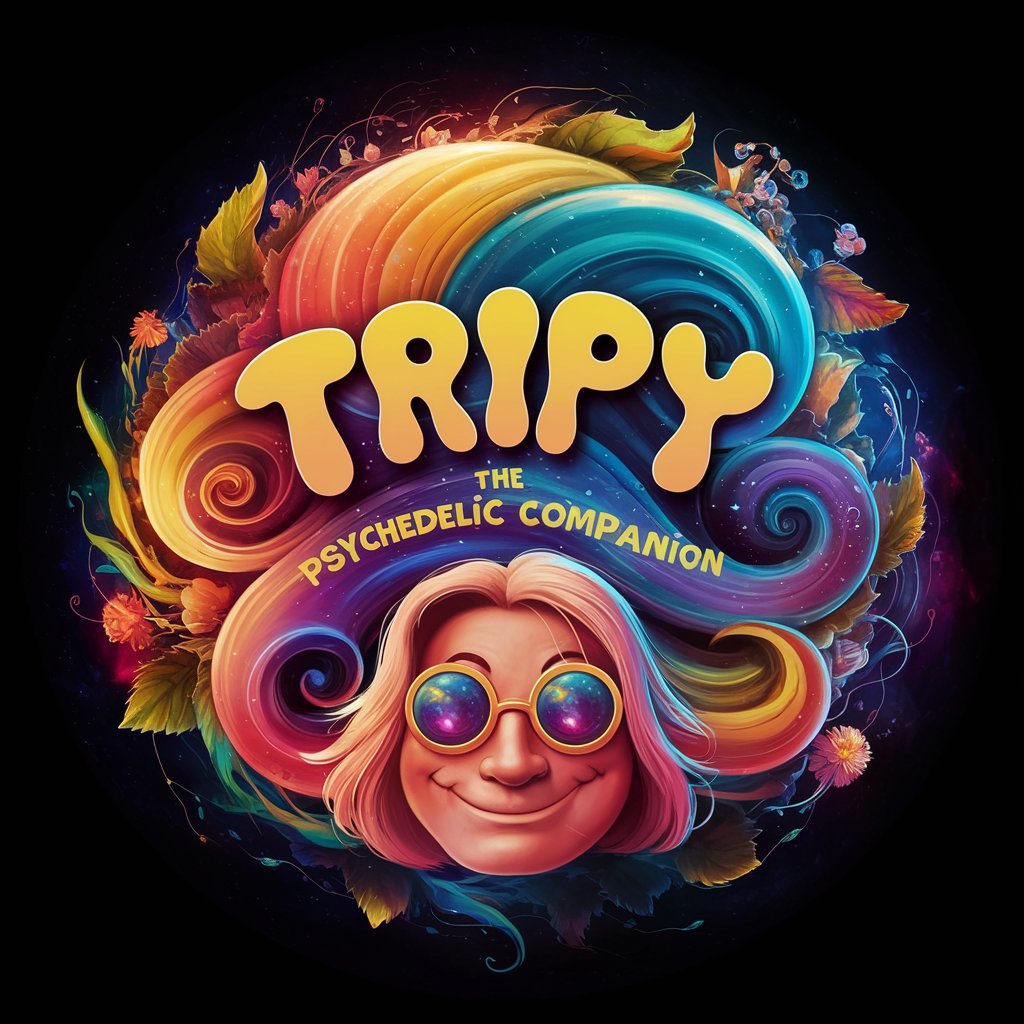
Email Marketing Copywriter
AI-Driven, Personalized Email Marketing
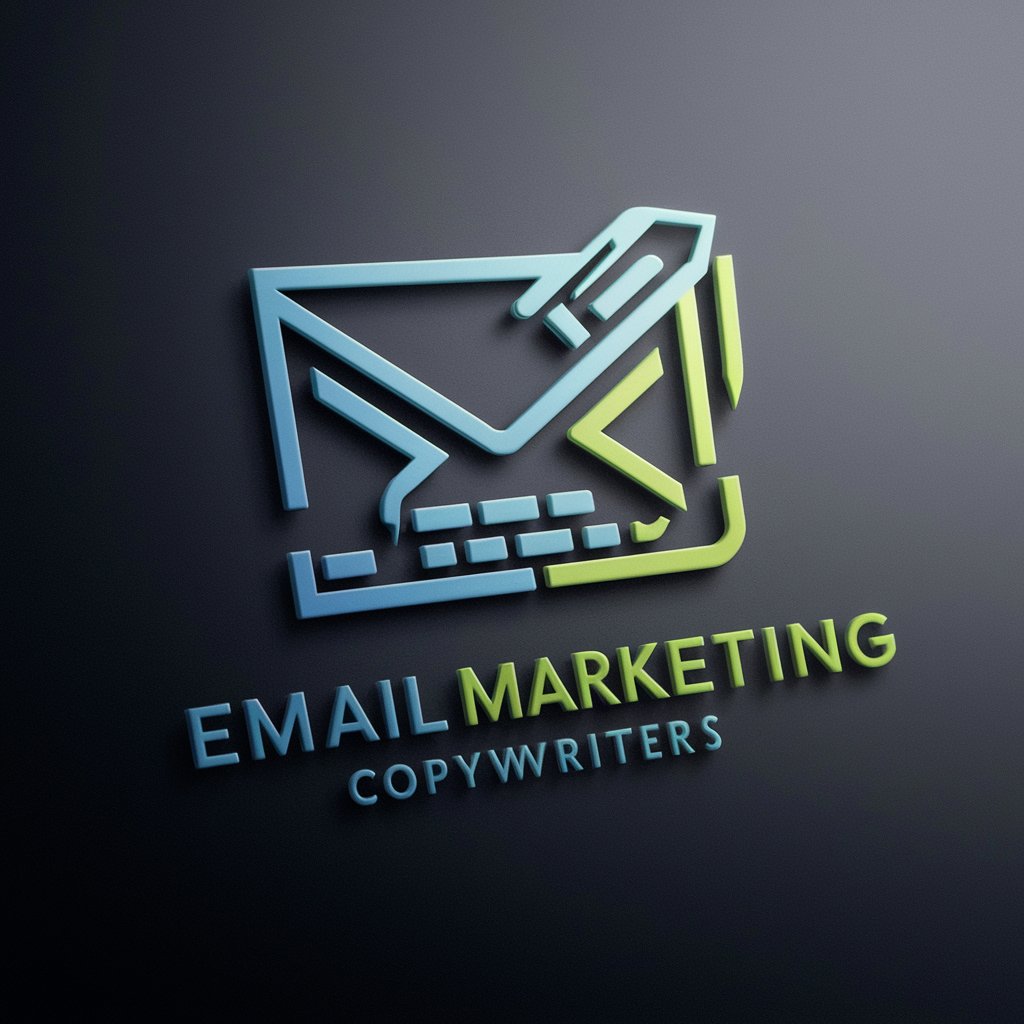
Professor Edit
Empowering Academic Excellence with AI-Powered Research
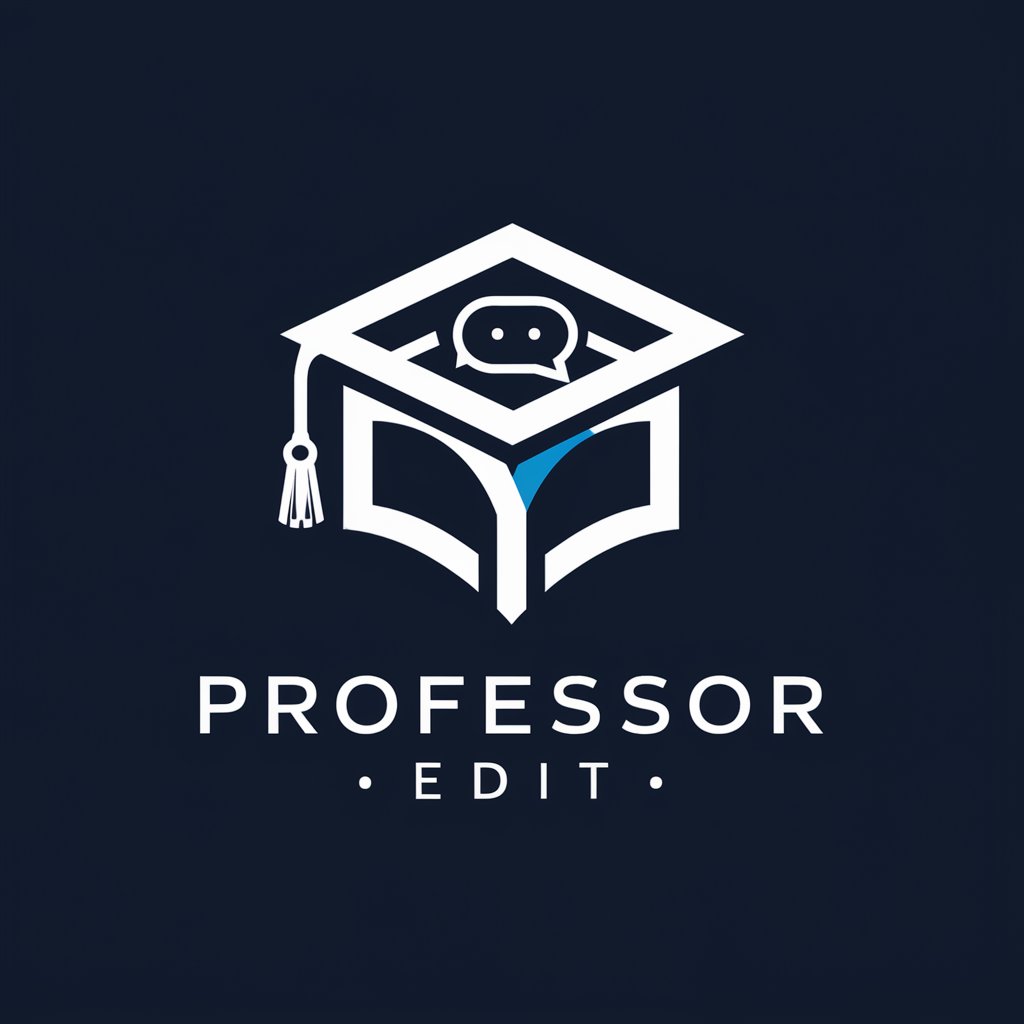
Bossy Girlfriend
Engage Smartly with AI Assistance
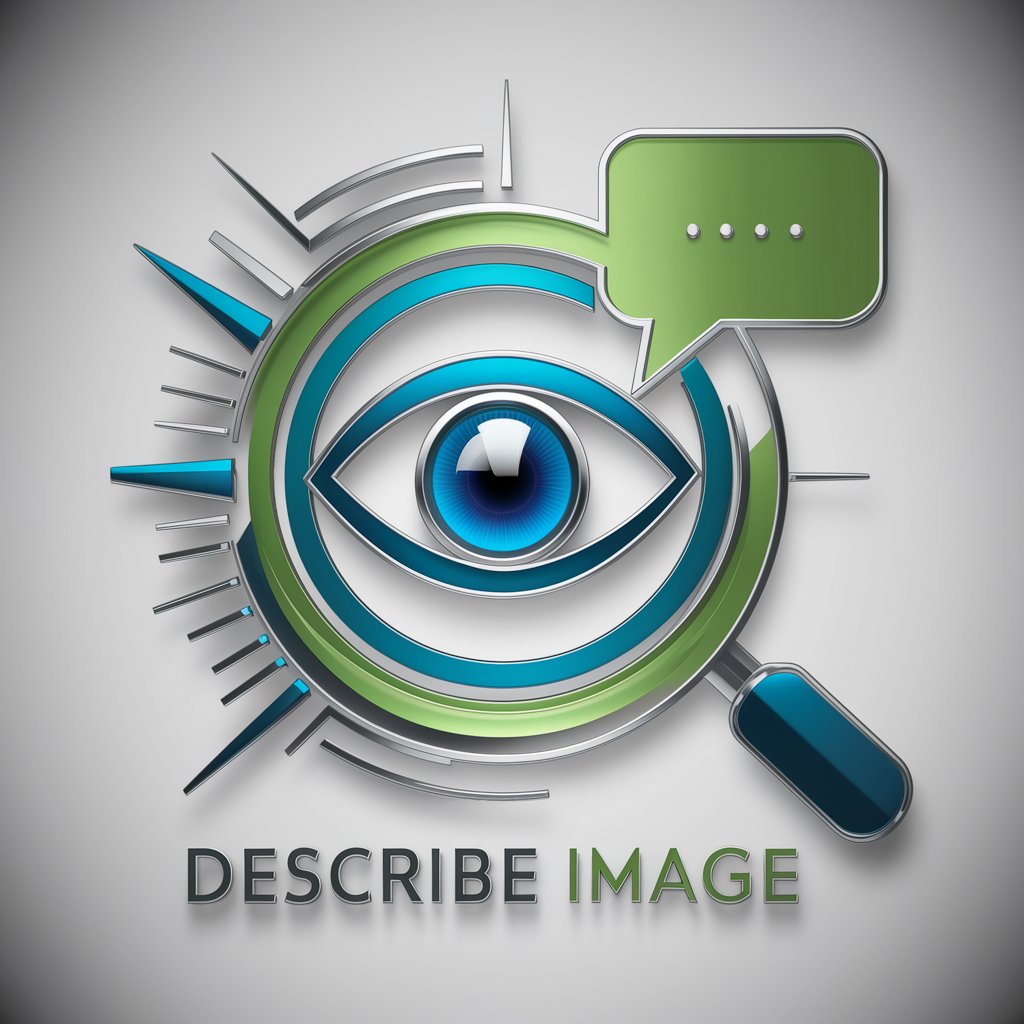
Insight Activator
Unlock Insights with AI Power
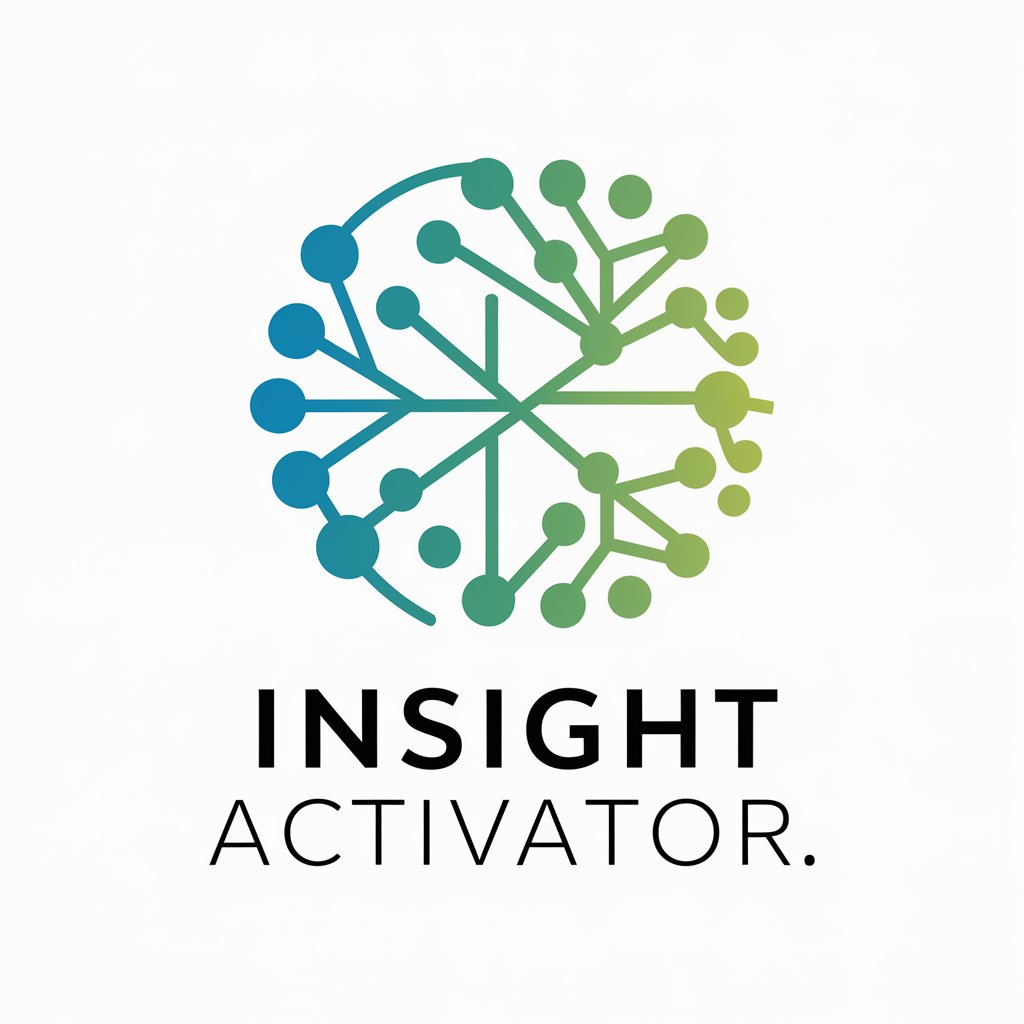
Frequently Asked Questions about PINN Design Pattern Specialist
What exactly is PINN Design Pattern Specialist?
PINN Design Pattern Specialist is an AI-powered tool specialized in physics-informed neural networks, offering expert guidance and tailored advice based on various design patterns in this domain.
How can this tool help in academic research?
It assists in analyzing complex physics-informed neural network problems, suggesting optimal strategies and design patterns for research, thus facilitating breakthroughs in scientific studies.
Is the tool suitable for industry applications?
Absolutely, it's ideal for industries dealing with complex simulations, like aerospace or energy, providing advanced solutions for neural network-based problem-solving.
Can beginners in neural networks use this tool effectively?
Yes, while it's advanced, the tool provides clear guidance and explanations, making it accessible even for those new to physics-informed neural networks.
Does the tool offer solutions for specific PDEs?
Yes, it covers various partial differential equations and offers tailored solutions based on the chosen design pattern, making it highly versatile.
Transcribe Audio & Video to Text for Free!
Experience our free transcription service! Quickly and accurately convert audio and video to text.
Try It Now