Data Processing-Efficient Data Analysis
Empowering insights with AI-driven data processing
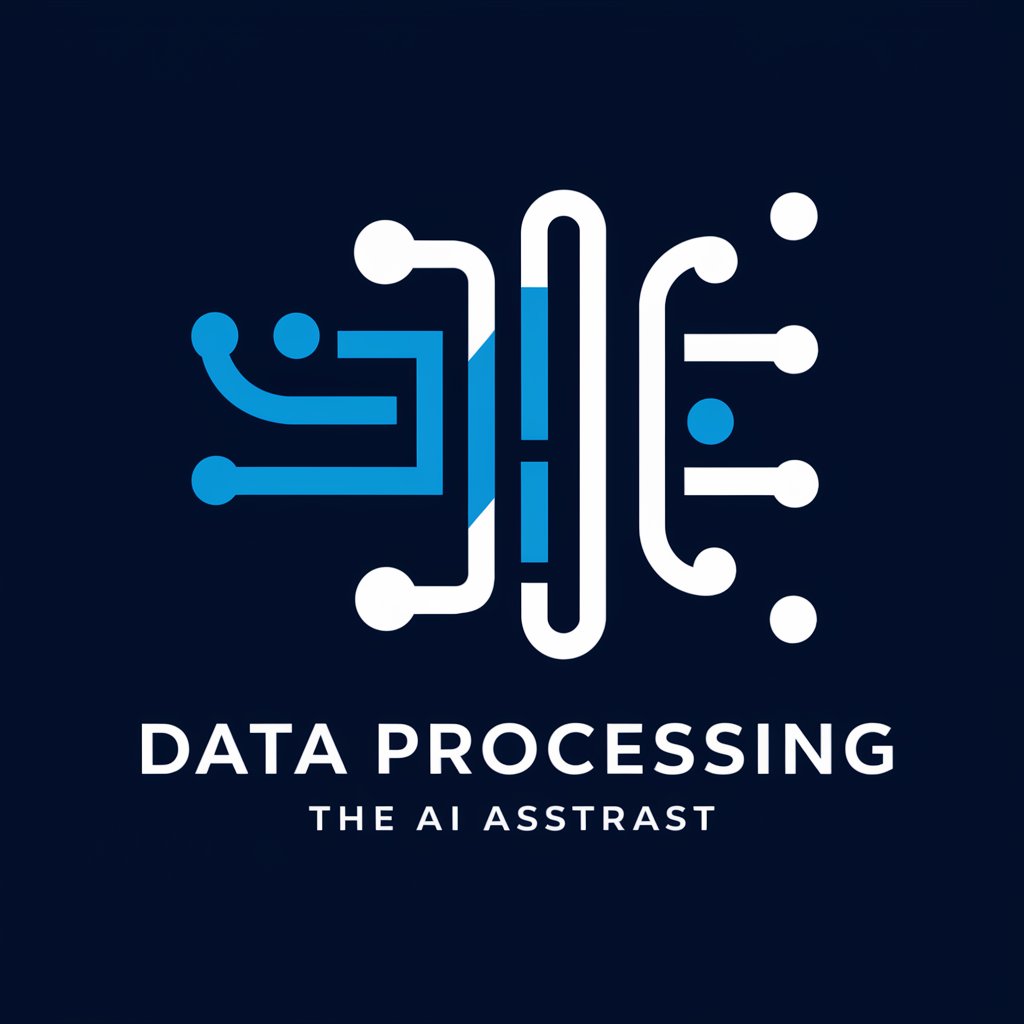
Explain the concept of data normalization in databases.
Describe the latest advancements in machine learning algorithms for data processing.
How does data encryption work in secure data transmission?
What are the best practices for data cleansing and preprocessing?
Related Tools
Load More
Dr. Data
I clean, transform, and analyze CSV data.
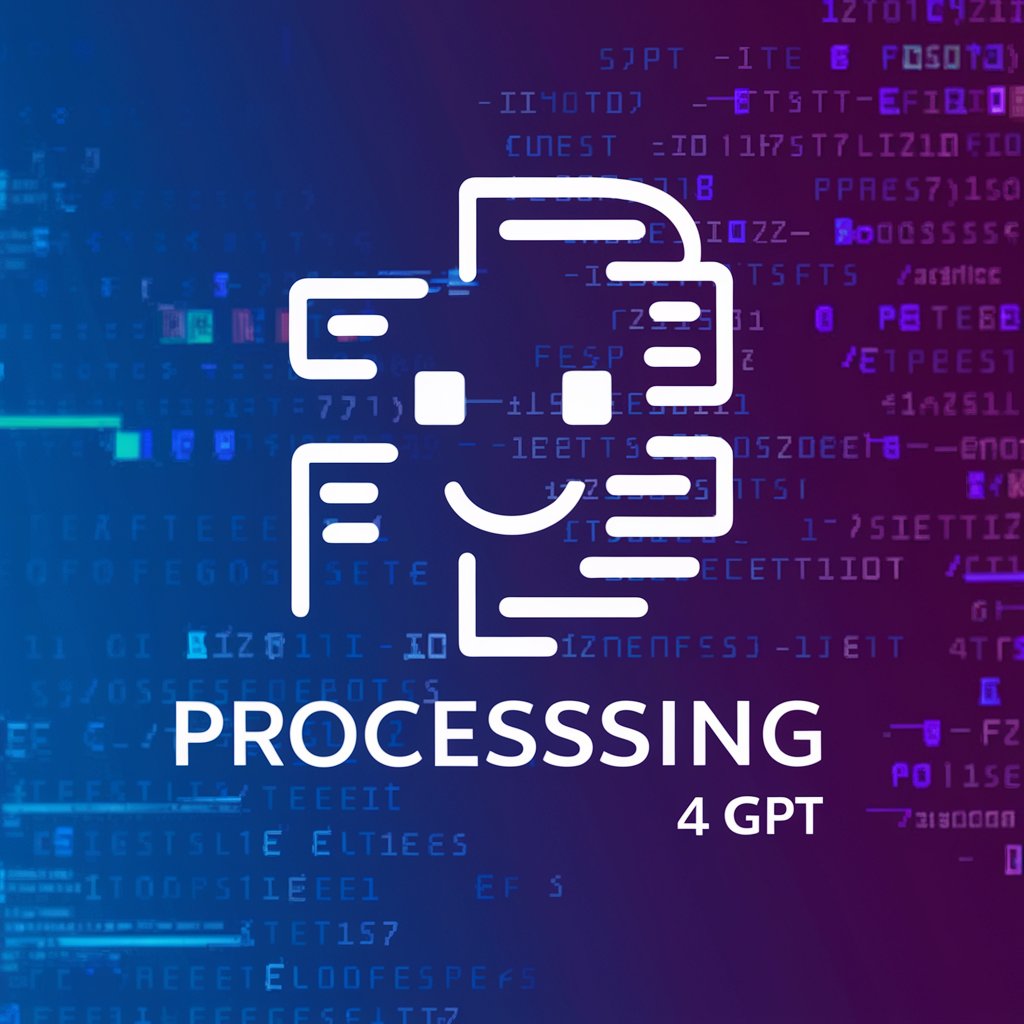
Processing 4 GPT
This GPT will help you create apps and utilities in Processing 4.
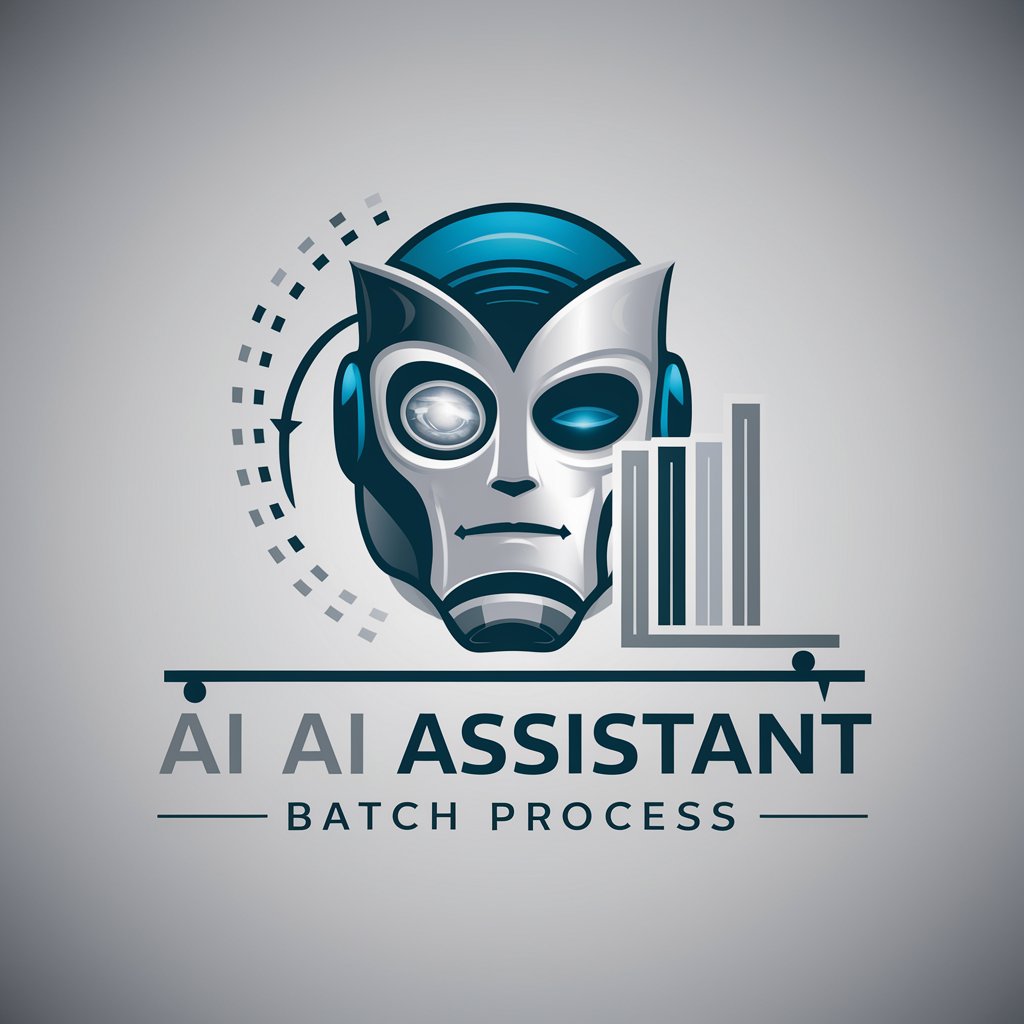
Batch Process
Upload & process files in batches. Supports CSV, TSV, Excel, Google Sheets. Ideal for data analysis & insight.
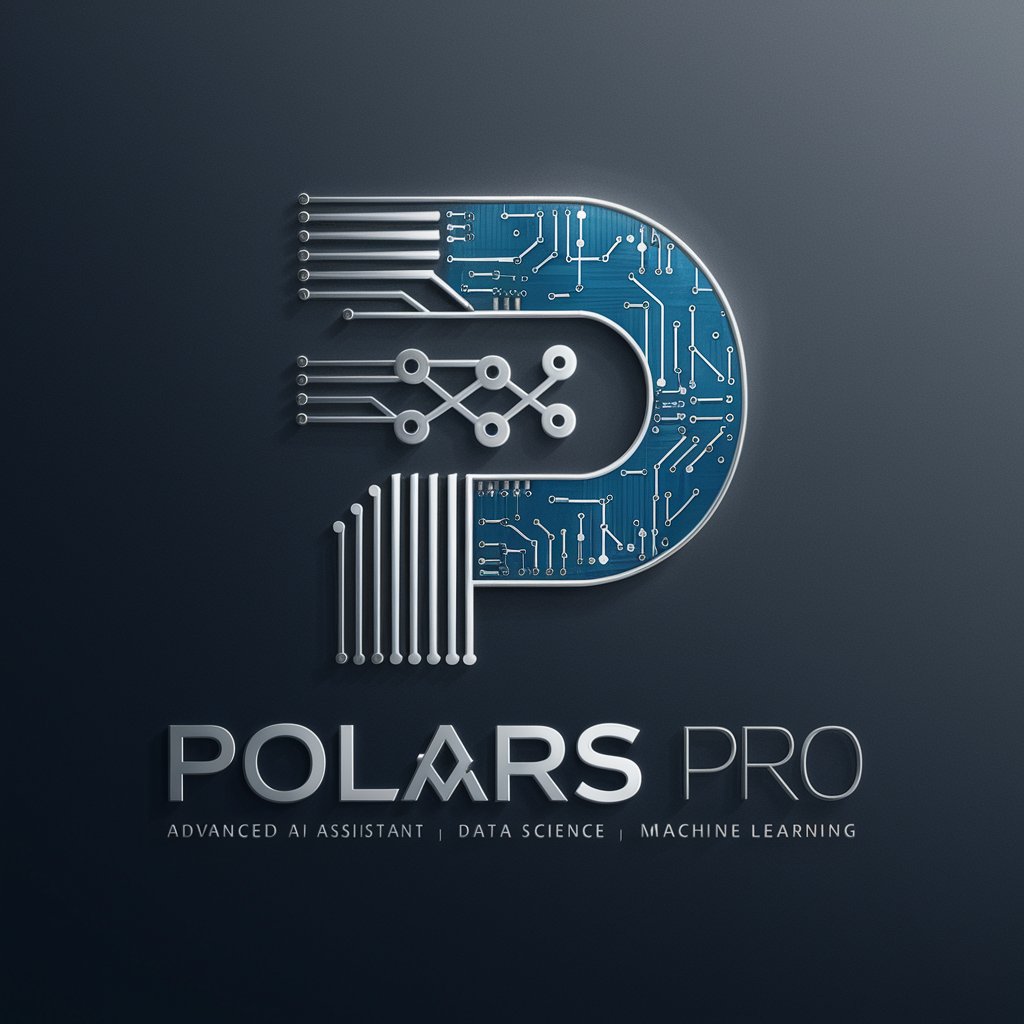
Polars Pro
Polars library sage
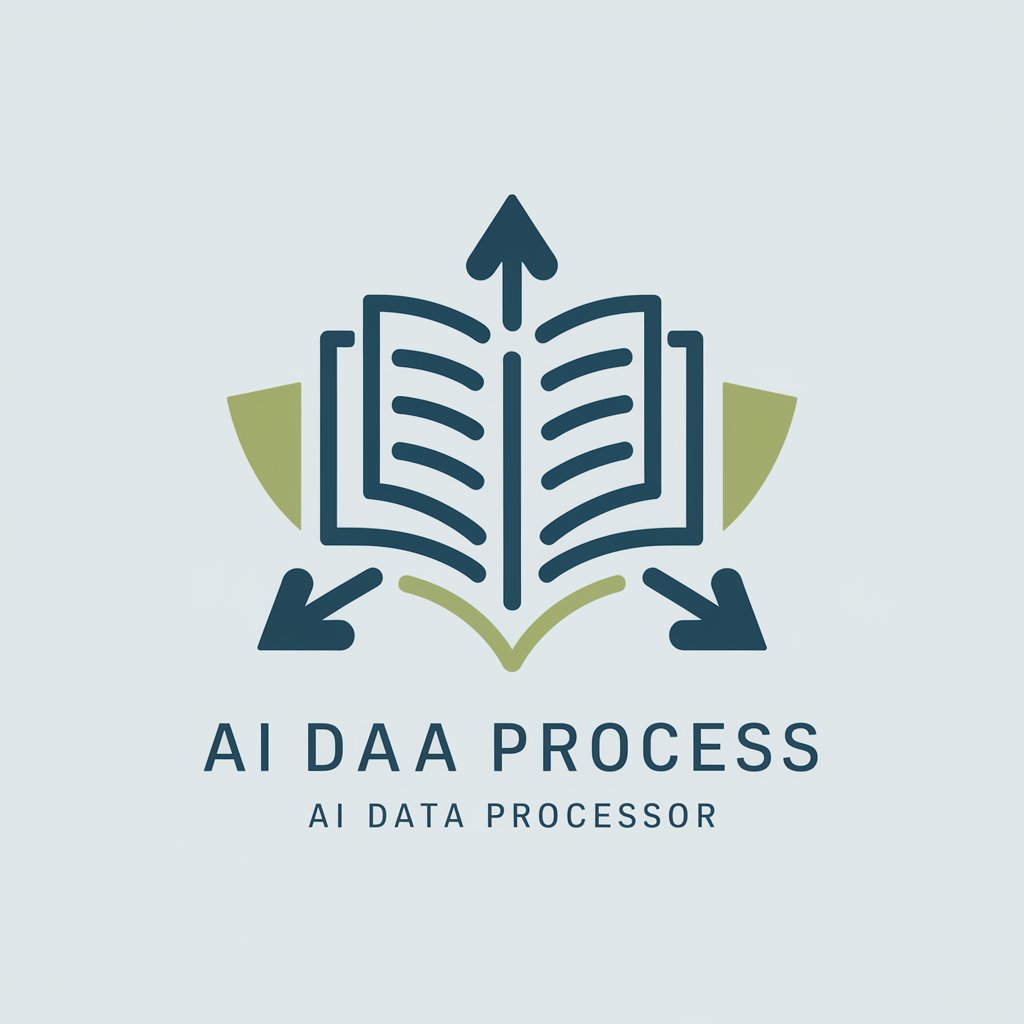
AI Data Processor
Transforms text files into AI-readable documents for non-tech users.
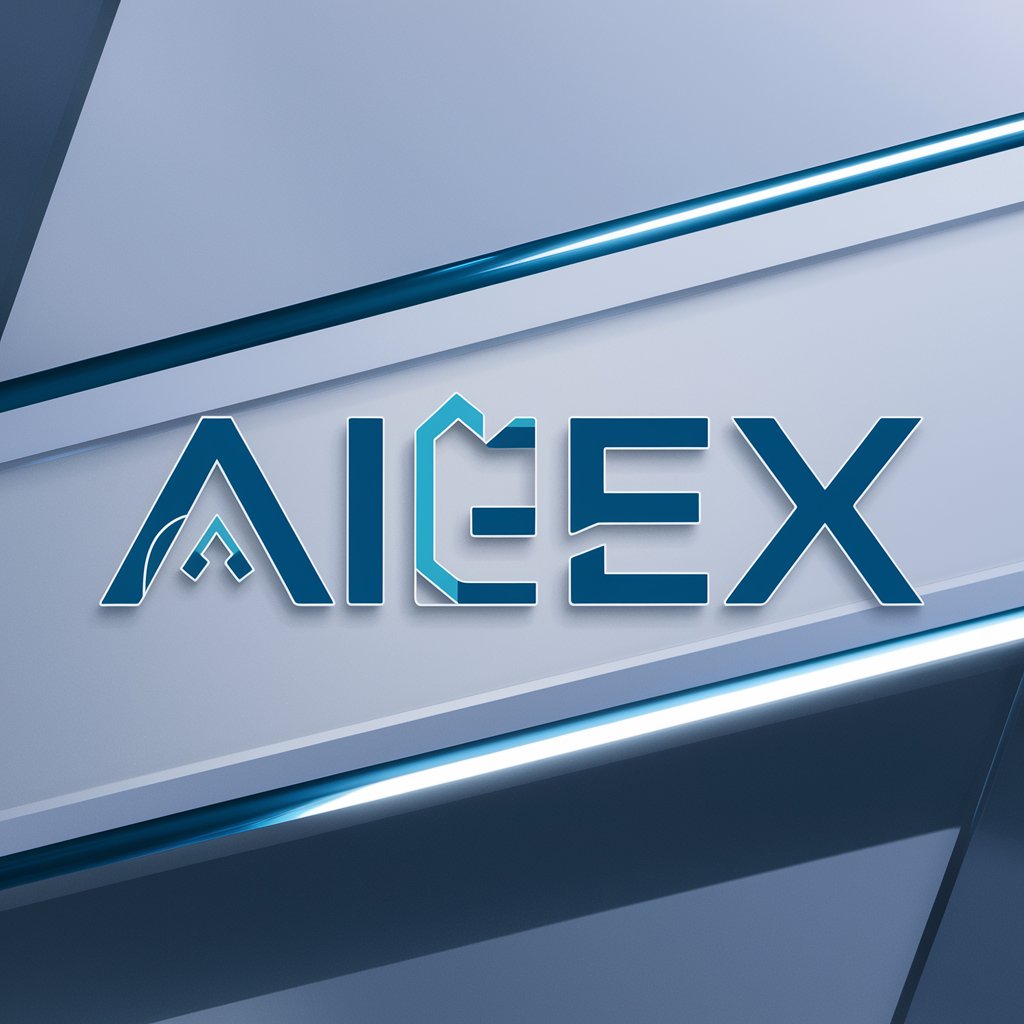
AI.EX: File Processor Pro
Your ally in converting, editing, and merging files seamlessly
Introduction to Data Processing
Data Processing refers to the collection, manipulation, and management of data to generate meaningful information suitable for decision-making, analysis, and dissemination. It encompasses a range of operations that can be performed on data, including its acquisition, validation, storage, processing, and analysis, ultimately leading to the generation of insightful outputs. The design purpose behind data processing systems is to streamline and enhance the efficiency of operations by converting raw data into more usable formats. This process is fundamental in various fields such as business intelligence, data analytics, scientific research, and more. For example, in e-commerce, data processing is used to analyze customer behavior, manage inventory, and personalize shopping experiences. In scientific research, it helps in the analysis of experimental data to draw conclusions or develop new hypotheses. Powered by ChatGPT-4o。
Main Functions of Data Processing
Data Collection
Example
Gathering user feedback through surveys
Scenario
In market research, data collection helps in understanding consumer preferences, which aids in tailoring products to meet customer needs.
Data Cleaning
Example
Removing duplicates and correcting errors in a dataset
Scenario
Ensuring the accuracy of data before analysis in healthcare research, leading to reliable study outcomes.
Data Analysis
Example
Applying statistical methods to analyze sales data
Scenario
Businesses use data analysis to identify sales trends and make informed decisions about product development and marketing strategies.
Data Storage
Example
Using databases to store customer information securely
Scenario
Companies maintain databases of customer information to enhance customer service and manage contacts efficiently.
Data Visualization
Example
Creating charts and graphs to represent financial performance
Scenario
Financial analysts use data visualization to present complex financial data in an understandable format to stakeholders.
Ideal Users of Data Processing Services
Business Analysts
Business Analysts rely on data processing for analyzing market trends, customer behavior, and operational efficiency. The insights gained assist in strategic planning and decision-making.
Data Scientists
Data Scientists use advanced data processing techniques, including machine learning and statistical analysis, to extract insights from data. This is crucial for predictive modeling, algorithm development, and data-driven decision-making.
IT Professionals
IT Professionals utilize data processing for managing databases, ensuring data security, and optimizing data storage and retrieval processes. This supports the infrastructure for data analytics and business intelligence.
Research Scientists
Research Scientists apply data processing in analyzing experimental or observational data to validate hypotheses and publish findings. It is essential for advancing knowledge in various scientific fields.
How to Use Data Processing
1
Initiate your data processing journey by accessing a free trial at yeschat.ai, with no requirement for login or a ChatGPT Plus subscription.
2
Identify your data processing needs by considering the volume, variety, and velocity of the data you aim to process. This will help in selecting the appropriate tools and methods.
3
Choose the right data processing model (batch, real-time, or hybrid) based on your project's requirements. Consider latency, throughput, and data integrity needs.
4
Leverage available templates and tutorials on yeschat.ai to familiarize yourself with the platform's capabilities and best practices in data processing.
5
Iteratively refine your data processing workflows by incorporating feedback and monitoring performance metrics to ensure optimal results.
Try other advanced and practical GPTs
Land Investing
Empowering your land investment journey with AI.
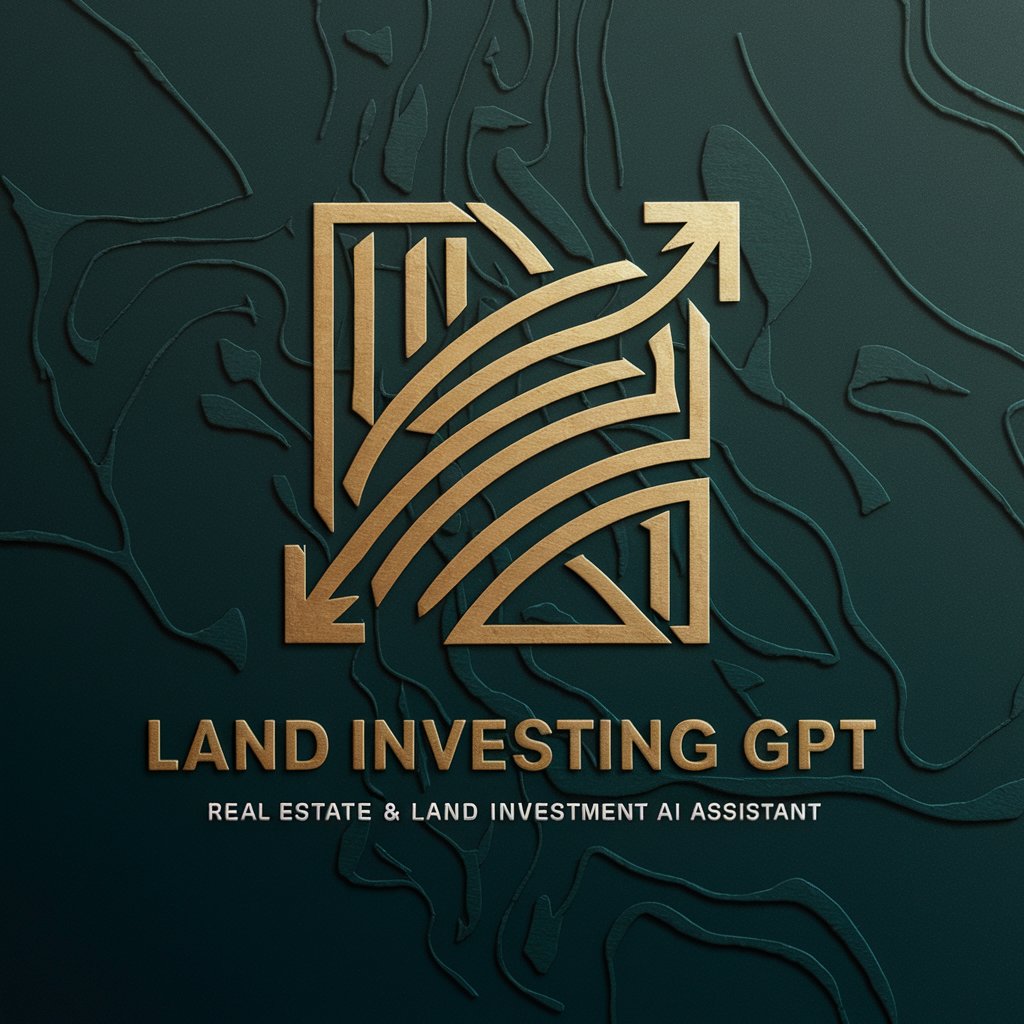
Portugal Real Estate
Navigating Portuguese Real Estate with AI
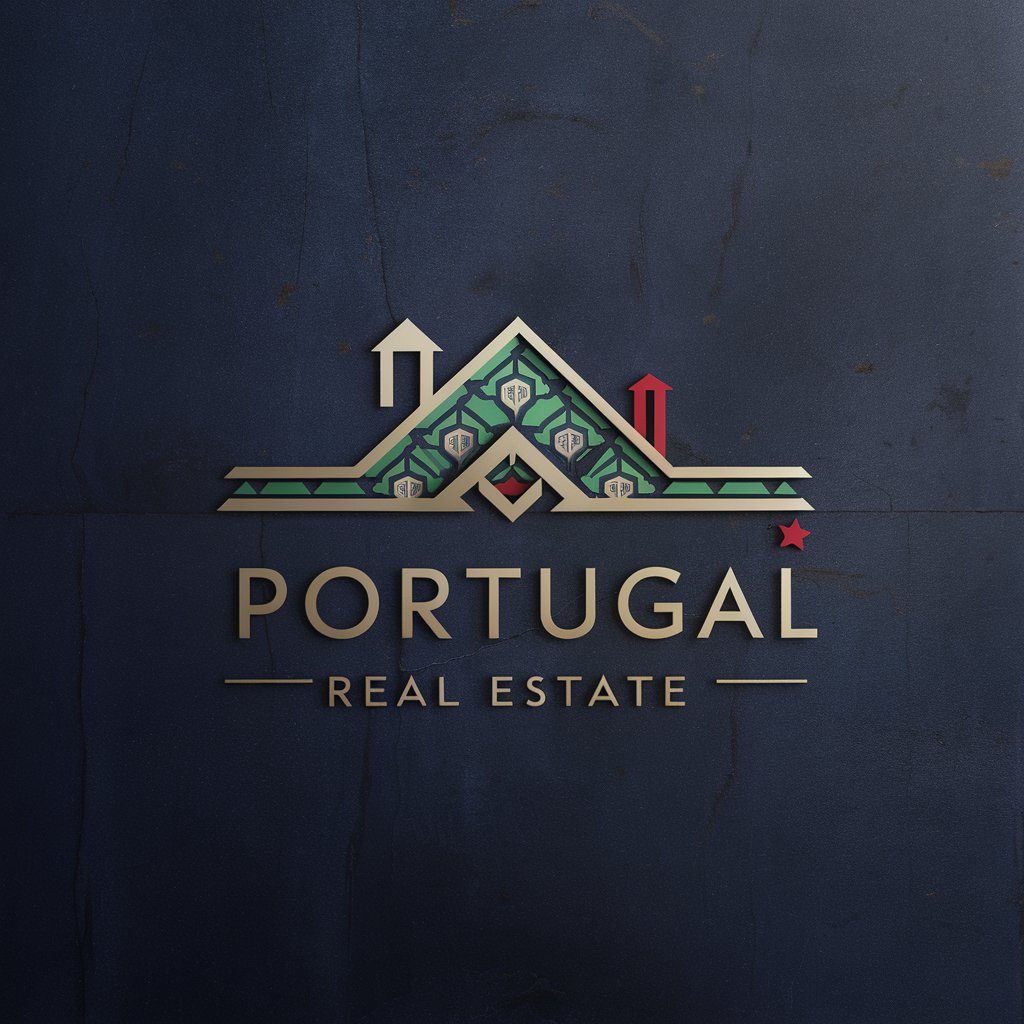
Realty Ai
Empowering Real Estate Decisions with AI
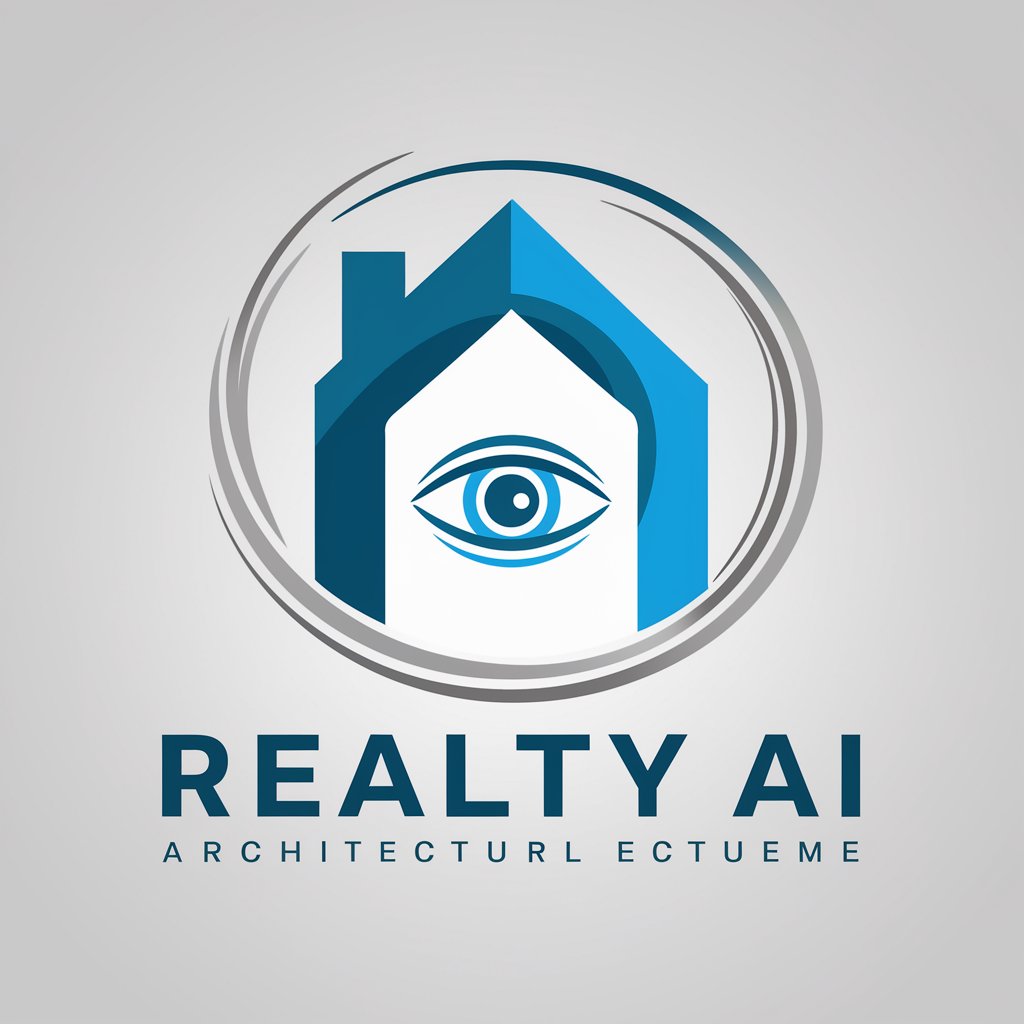
Virtual Real Estate Assistant
Empowering Your Real Estate Decisions with AI
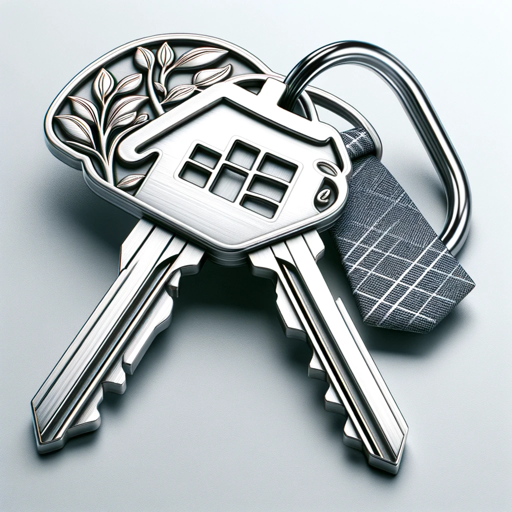
Land for Sale
Empowering Land Investment Decisions with AI
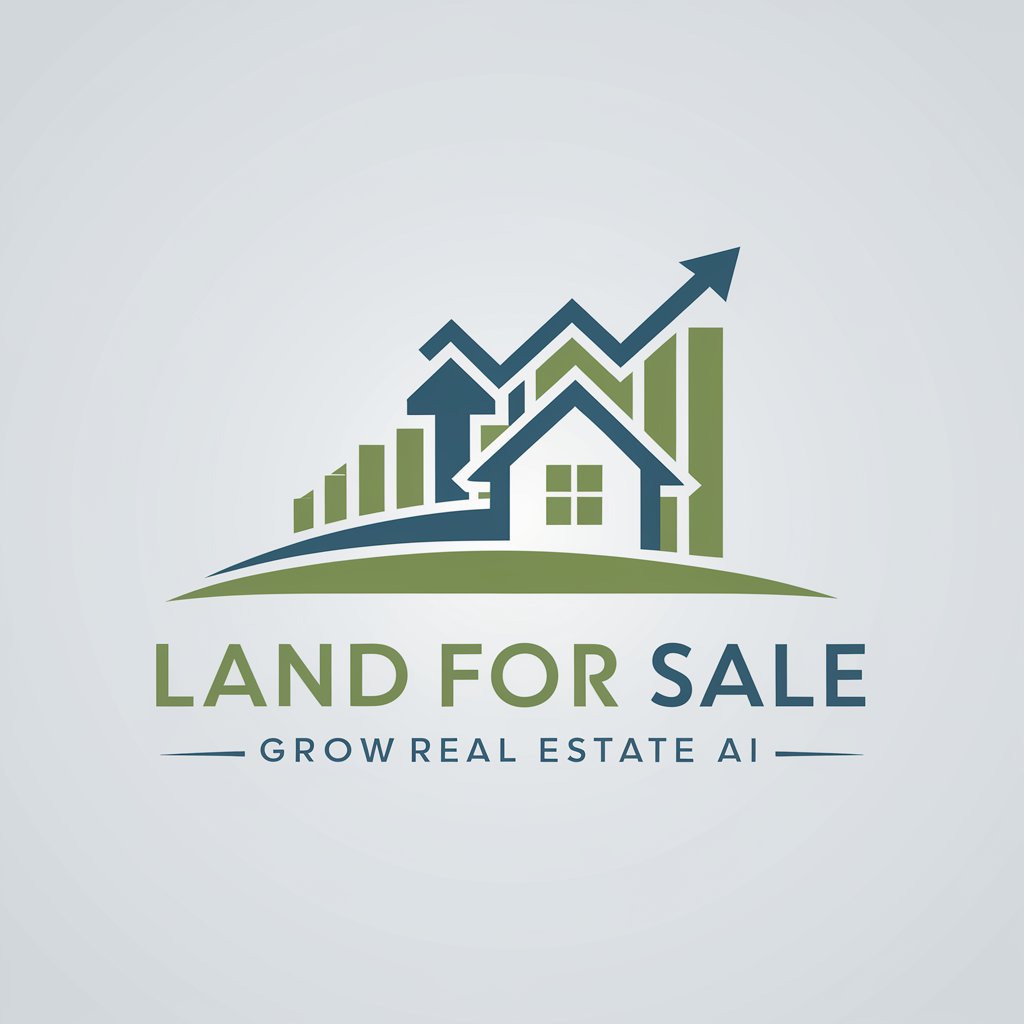
New York Property Advisor
Empowering Your New York Real Estate Decisions
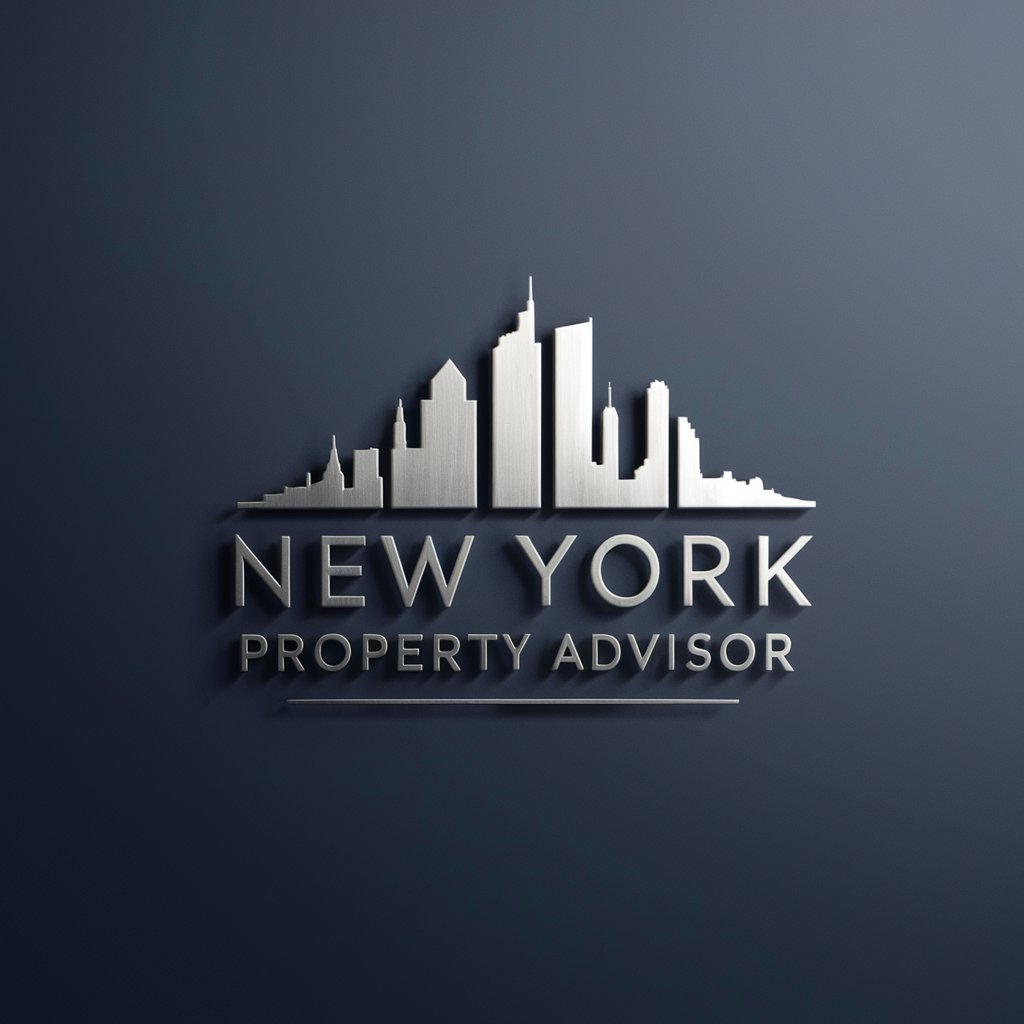
客户流失趋势分析
AI-powered churn trend insights
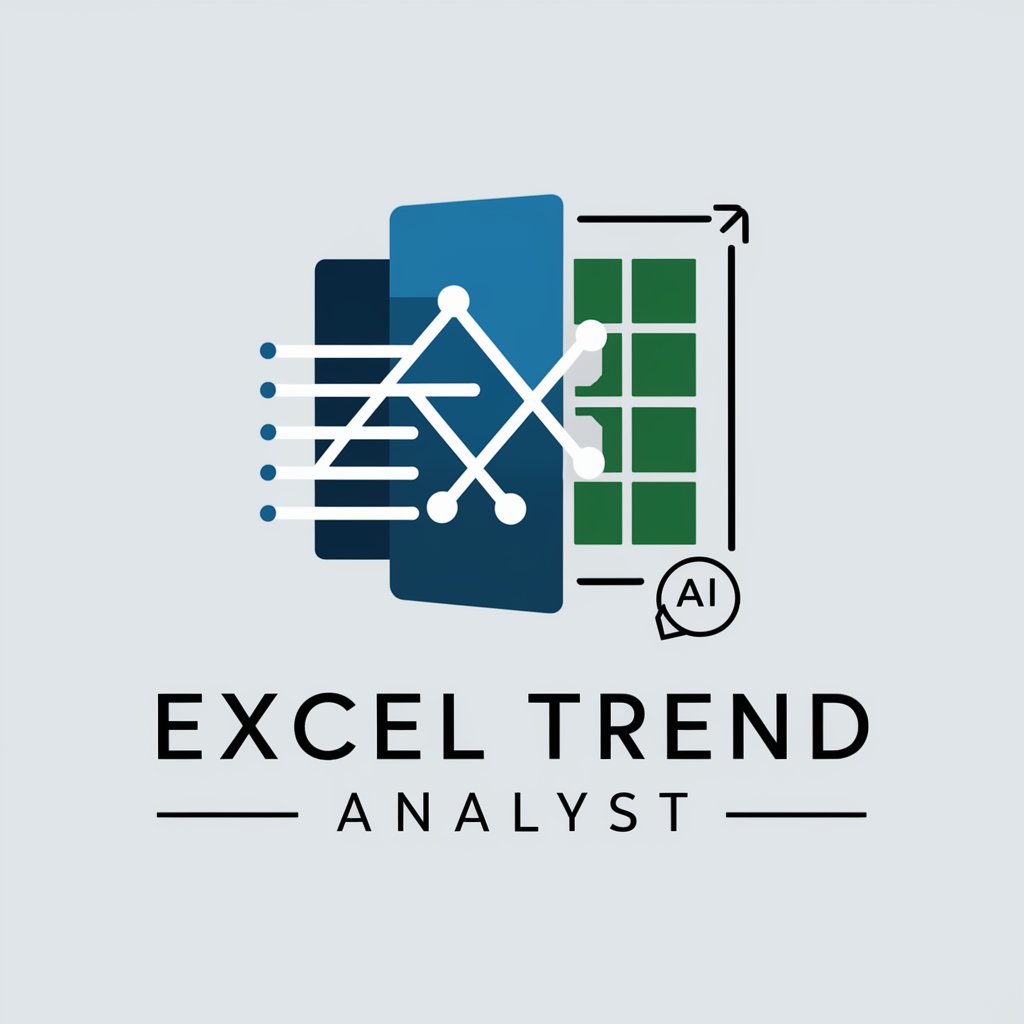
AgriPredict Expert
Empowering Agriculture with AI Insights
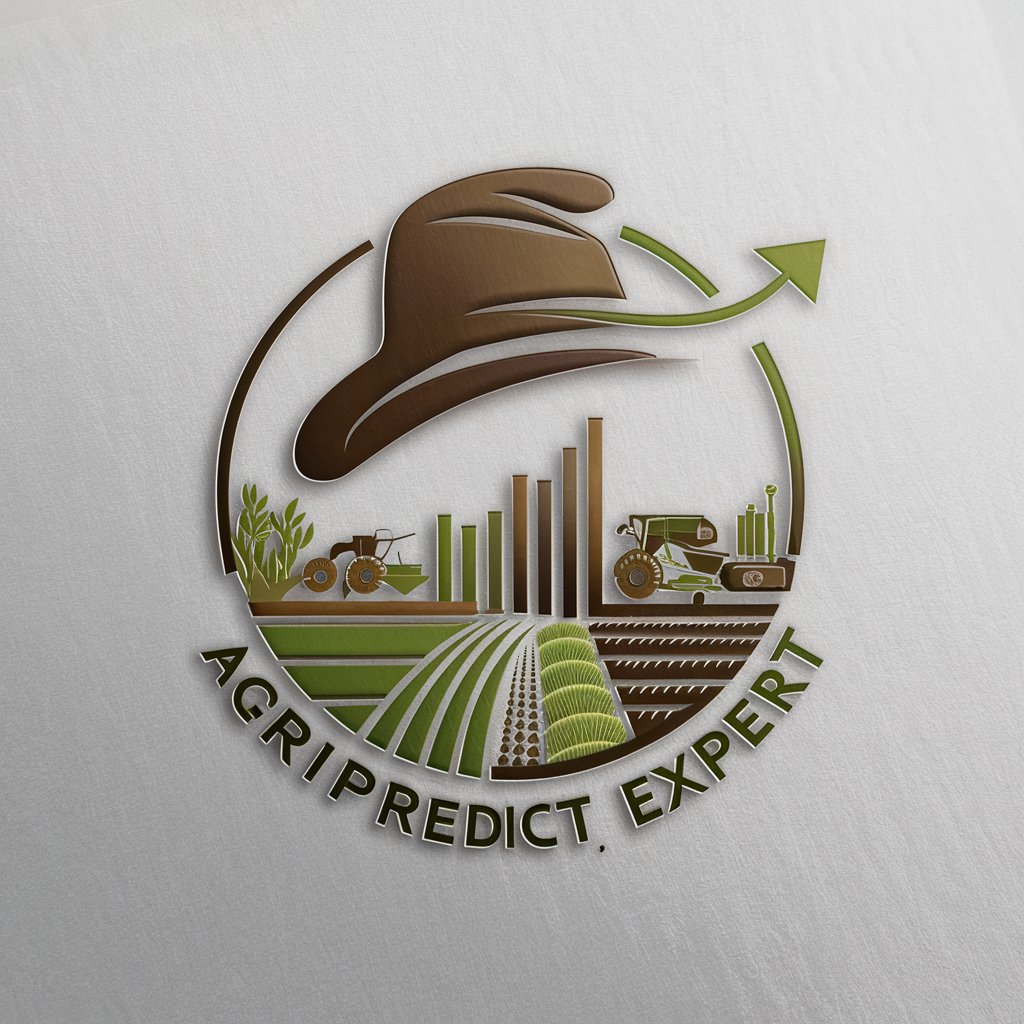
Data Analysis Coach
Empowering Your Data Analysis Journey with AI
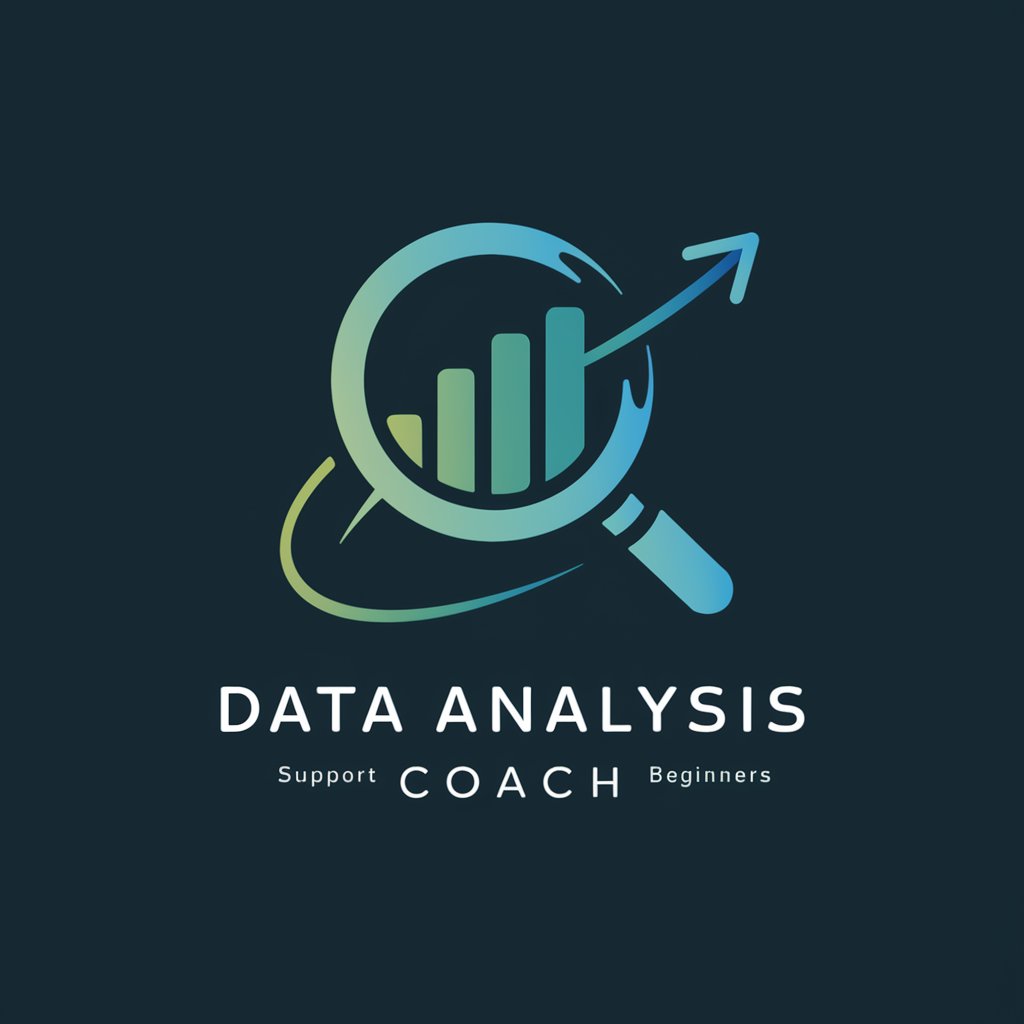
AutoBiz GPT
Empowering decisions with AI-driven insights
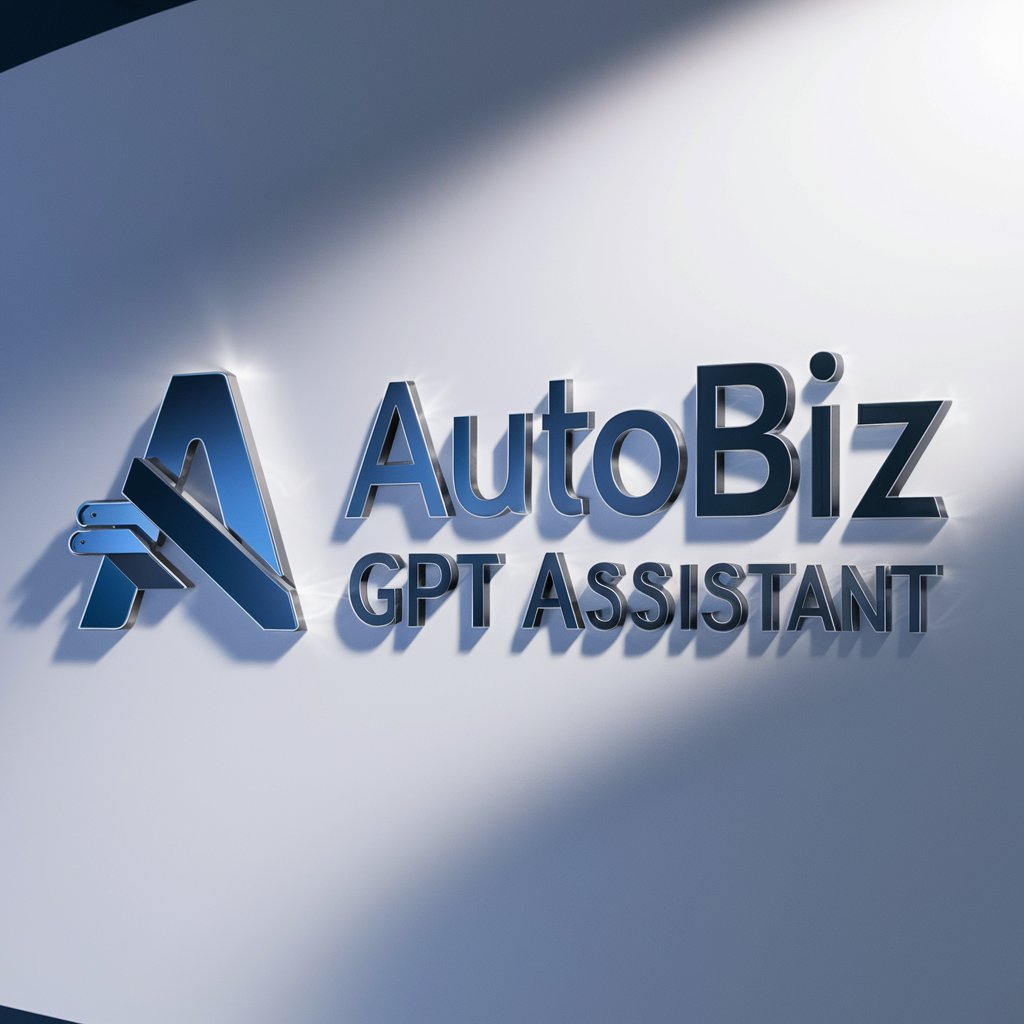
WebCraft Wizard
Crafting the future of web design, AI-powered.
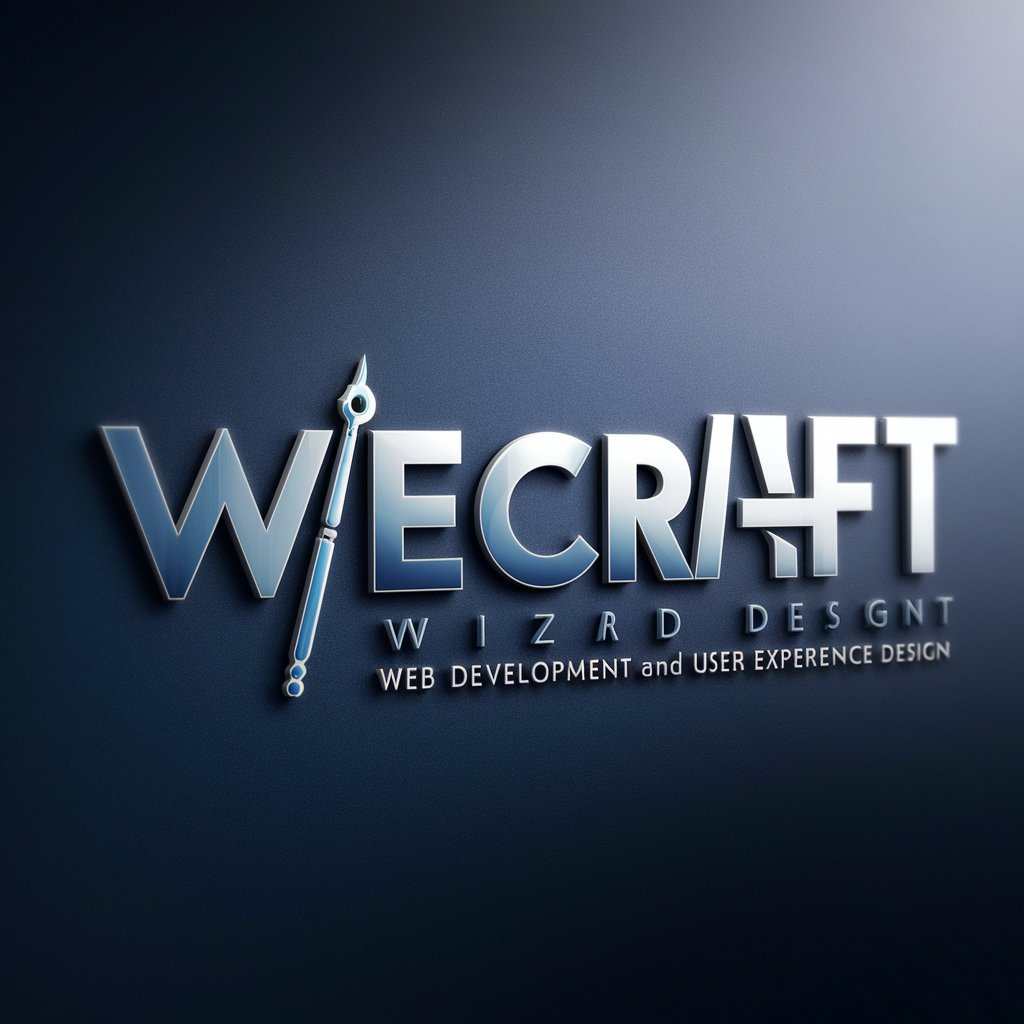
Advanced Case Study Expert
Empowering Design Decisions with AI
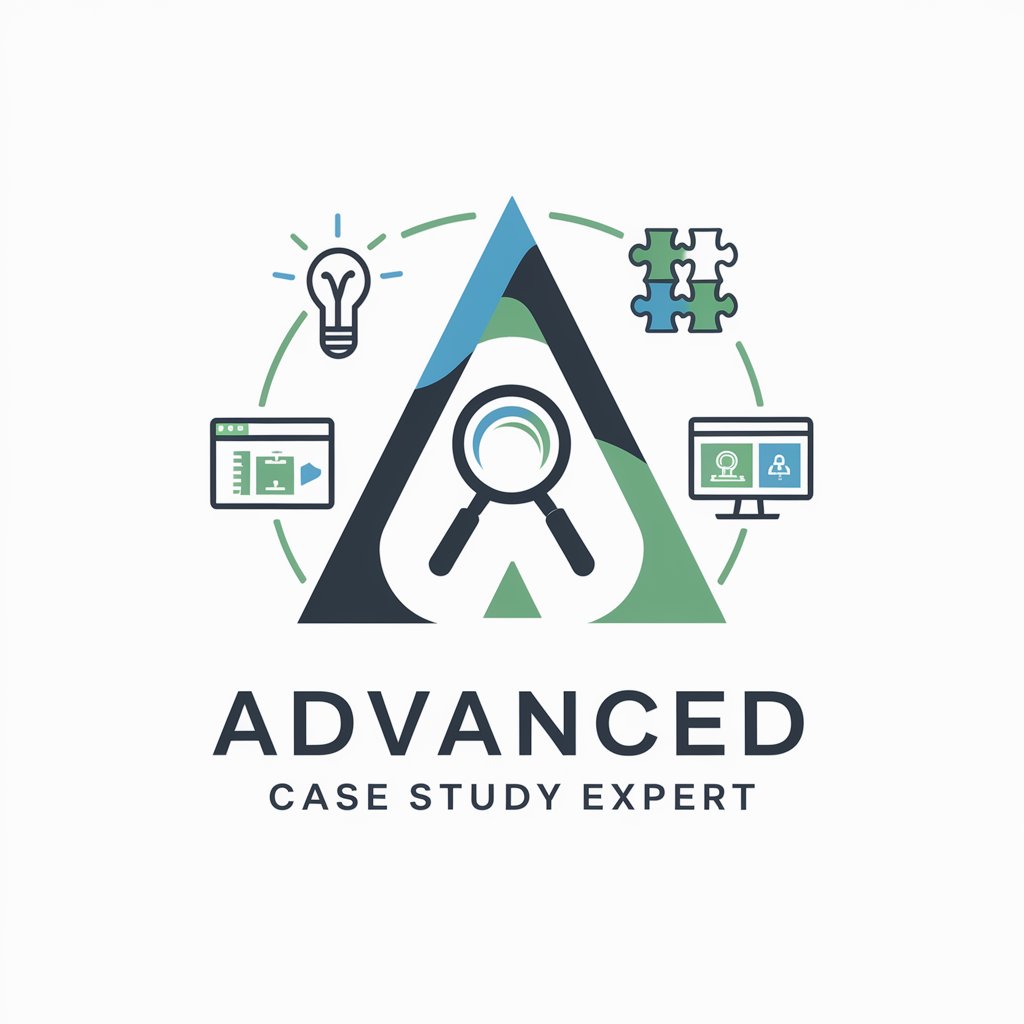
Data Processing Q&A
What is Data Processing?
Data Processing refers to the collection, transformation, and management of data to extract useful information, support decision-making, and enable automated processes.
Can Data Processing handle large volumes of data?
Yes, Data Processing is designed to efficiently manage and process large datasets, utilizing scalable architectures and distributed computing techniques.
How does Data Processing ensure data quality?
Data Processing employs various techniques such as data validation, cleansing, deduplication, and normalization to improve data quality and reliability.
What types of data can be processed?
Data Processing can handle diverse data types, including structured, semi-structured, and unstructured data, enabling wide applicability across different domains.
How does Data Processing support real-time analytics?
By utilizing stream processing technologies and in-memory computing, Data Processing enables the analysis of data in real-time, providing immediate insights and enabling timely decision-making.