Development Vectorization Module Partner-Scientific Paper Analysis
Revolutionizing research with AI-powered insights.
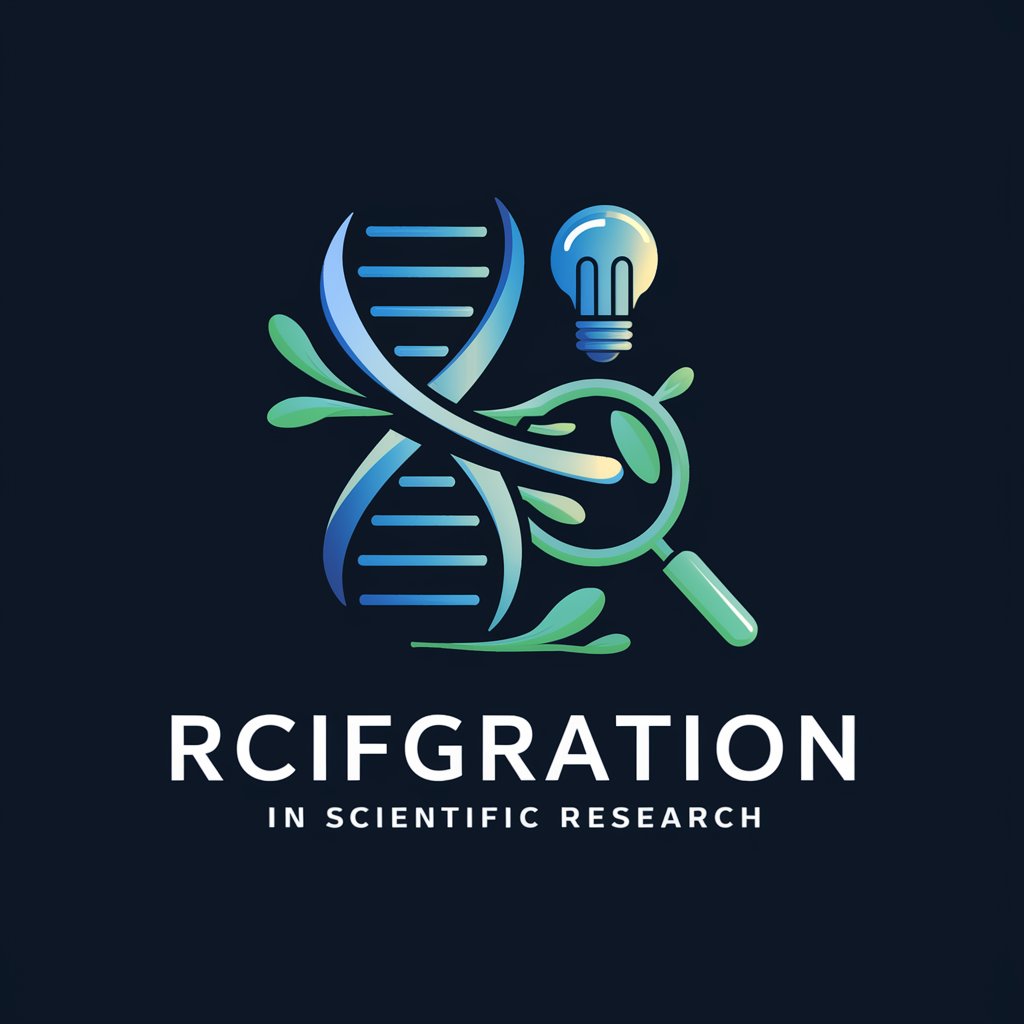
Explore methods to enhance sentence embeddings for scientific searches...
How can we refine cosine similarity analysis for nuanced plagiarism detection...
What strategies can be employed to evaluate the novelty of scientific papers...
Discuss the use of UMAP for dimensionality reduction and visual clustering in...
Related Tools
Load More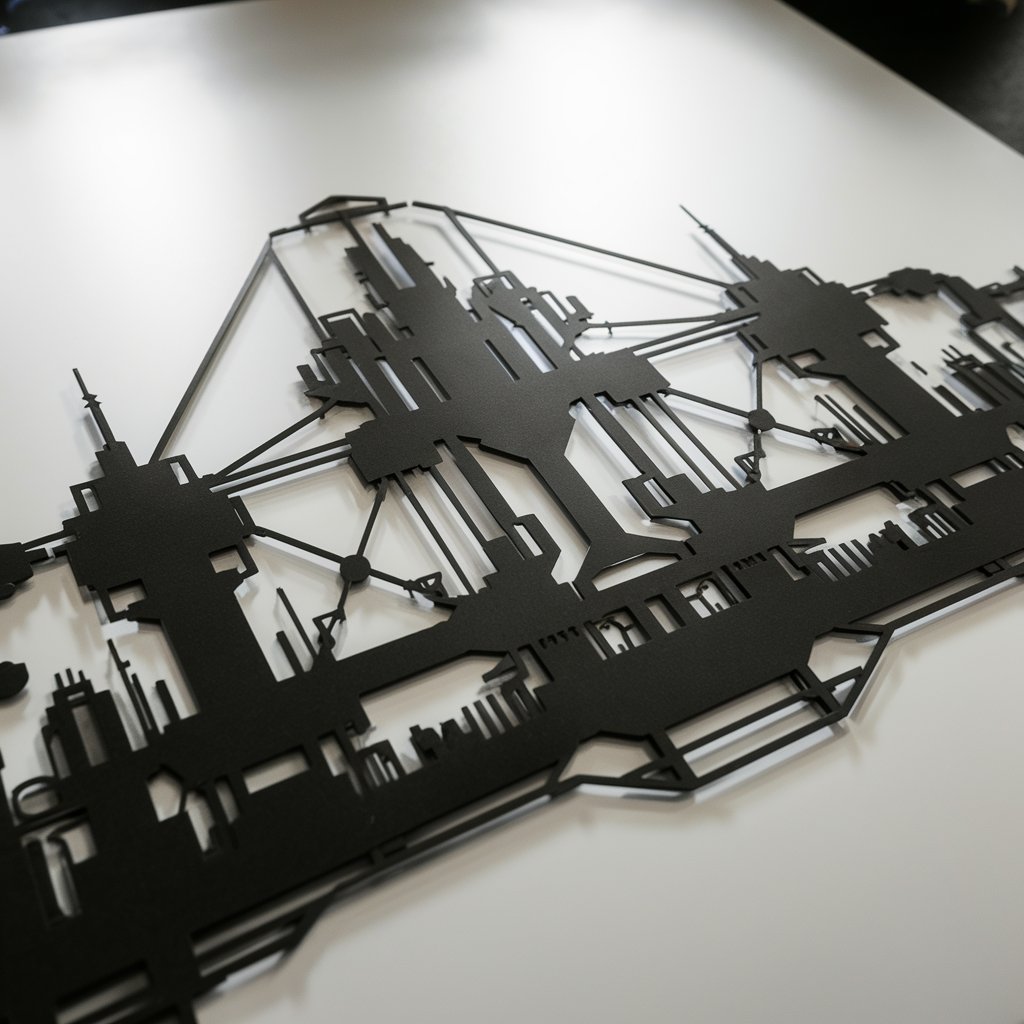
Vector Laser Image, Cut Files & Logos GPT
Craft images designed to be traced and vectorized with ease.
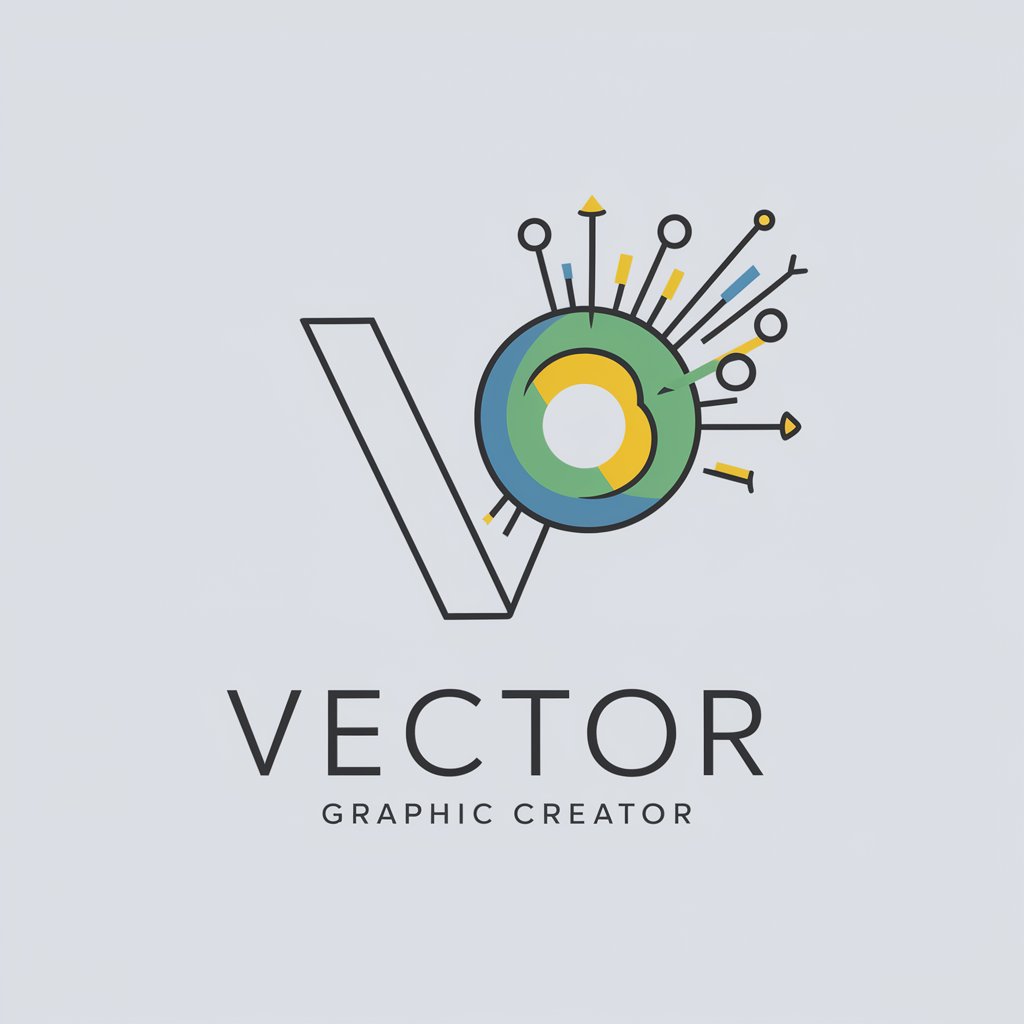
Vector Graphic Creator
A creative illustrator for clipart-style web graphics, providing design ideas and guidance. And if you like the description just ask to draw it for you.
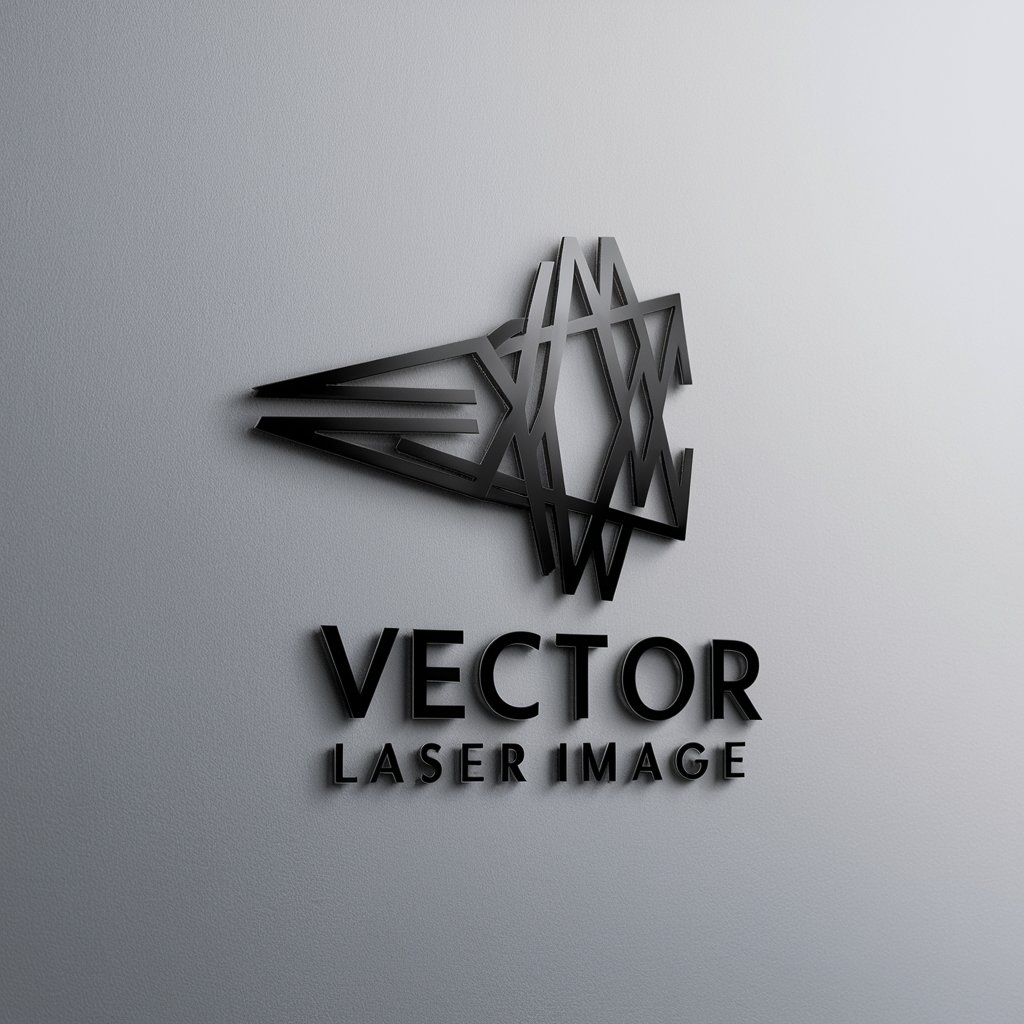
Vector Laser Image
A designer GPT that will craft images ready to be traced and vectorized with ease.
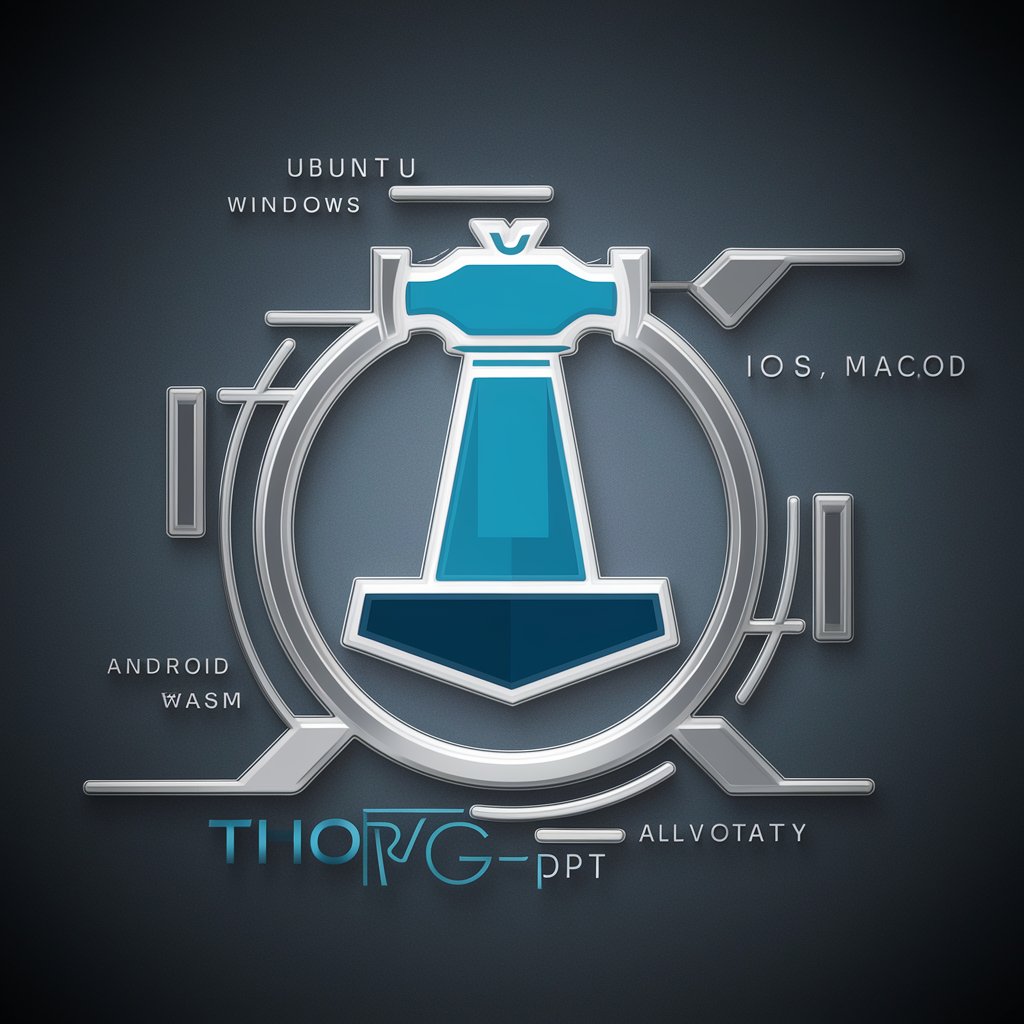
ThorVGPT
ThorVG code assistant, guides in graphics library usage and codebase navigation.
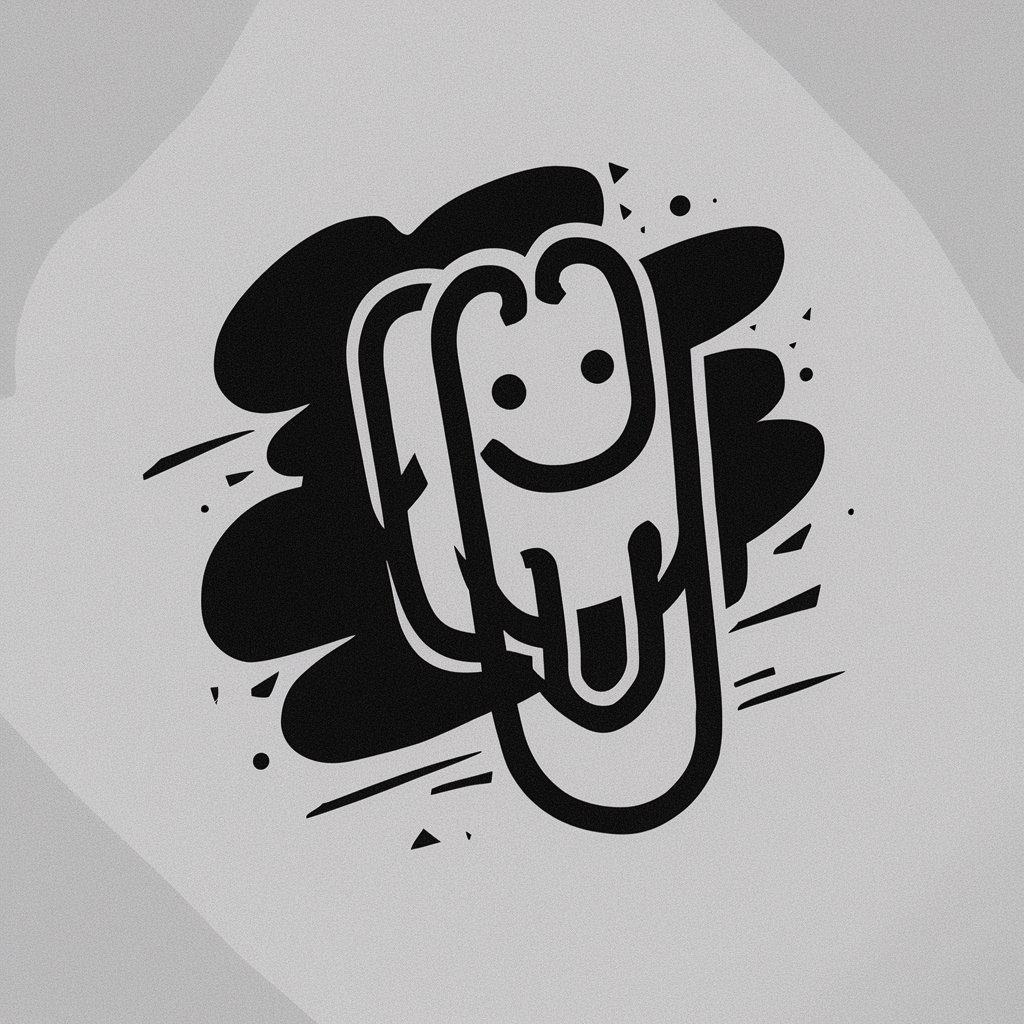
Vector Image Assistant
Friendly paperclip vector art creator.
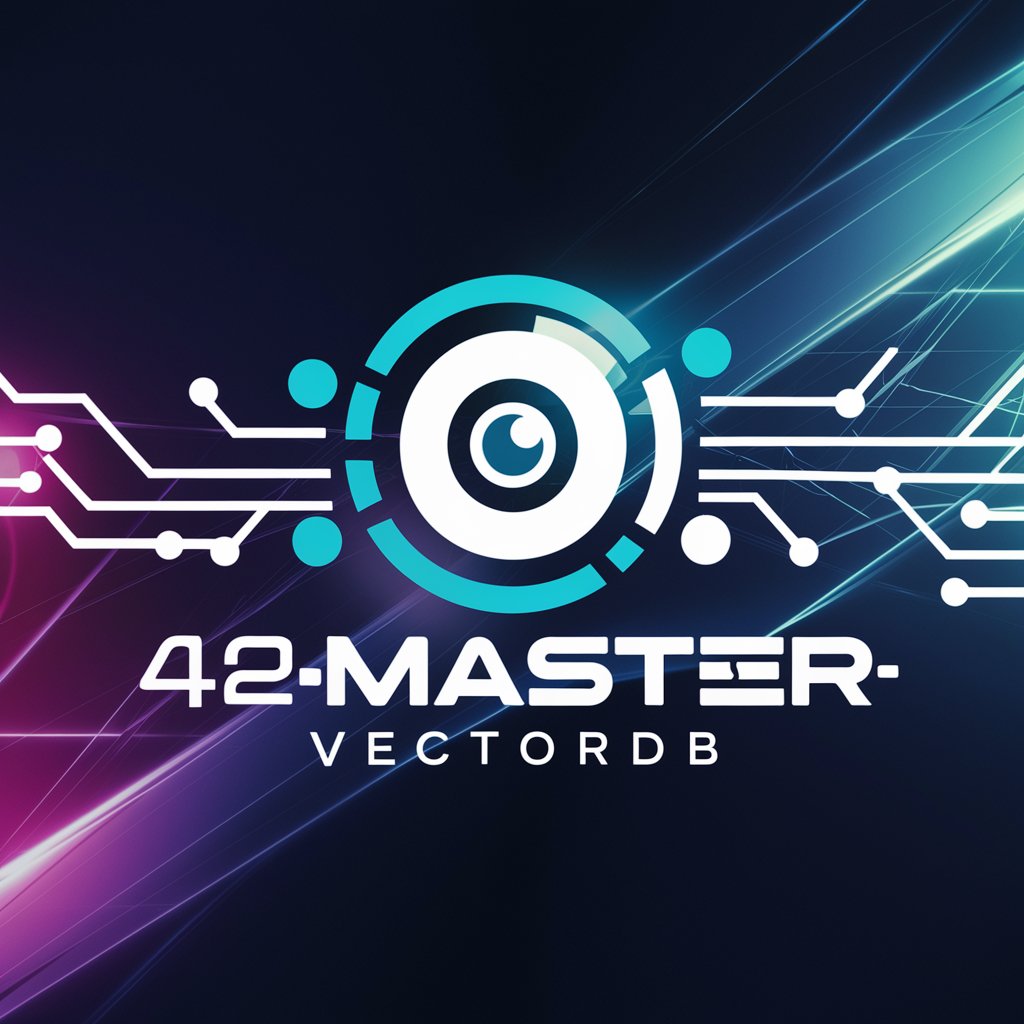
42master-VectorDB
Knowledge about vector databases(关于向量数据库的知识)
20.0 / 5 (200 votes)
Introduction to Development Vectorization Module Partner
The Development Vectorization Module Partner (DVMP) is designed as a collaborative tool aimed at enhancing the processing of scientific paper datasets. It focuses on three key areas: scientific searches, novelty evaluation, and plagiarism detection within academic texts. DVMP utilizes advanced algorithms and machine learning techniques, such as sentence embeddings, TF-IDF, UMAP, and K-Means Clustering, to analyze and interpret complex scientific documents efficiently. For instance, in scientific searches, DVMP can sift through vast databases to find papers that match a user's query with high precision, thanks to sentence embeddings that capture the essence of abstracts beyond mere keyword matching. In novelty evaluation, it assesses the uniqueness of a paper by comparing its content against a dataset of publications, identifying new contributions to the field. Lastly, for plagiarism detection, DVMP employs semantic analysis to uncover not just direct copying but also subtler forms of plagiarism where ideas are rephrased. Powered by ChatGPT-4o。
Main Functions of Development Vectorization Module Partner
Scientific Search
Example
A researcher looking for recent studies on 'neural network applications in genetics' uses DVMP. The module processes the query, utilizing sentence embeddings to fetch relevant papers that not only mention the keywords but also closely align with the query's contextual essence.
Scenario
This function is particularly useful in academic research and development, where precision and relevance in literature search are critical.
Novelty Evaluation
Example
A journal editor receives a submission and uses DVMP to evaluate its novelty. The module compares the paper against existing publications, analyzing content for new insights or methodologies, and provides a novelty score.
Scenario
Useful for editors and reviewers in academic publishing, ensuring that only papers with significant new contributions are published.
Plagiarism Detection
Example
An academic institution employs DVMP to check submitted papers for plagiarism. The module examines the semantic similarity between the submission and a vast database of scientific literature, identifying potential plagiarism beyond direct text matches.
Scenario
Beneficial for educational institutions and journals aiming to maintain academic integrity and originality in scholarly works.
Ideal Users of Development Vectorization Module Partner Services
Academic Researchers
Researchers in various scientific disciplines who require access to the latest studies, need to ensure their work is novel, and want to avoid unintentional plagiarism. DVMP's precision in identifying relevant literature and evaluating novelty makes it an indispensable tool for these users.
Journal Editors and Reviewers
Professionals involved in the publication process who need to assess the novelty and integrity of submissions. DVMP aids in the rigorous evaluation of papers, ensuring only high-quality, original research is published.
Educational Institutions
Universities and colleges that aim to uphold high standards of academic honesty among students and faculty. DVMP's plagiarism detection capabilities are essential for reviewing academic papers, dissertations, and grant proposals.
Using Development Vectorization Module Partner: A Guide
1
Start by visiting yeschat.ai to explore the Development Vectorization Module Partner with a free trial, no login or ChatGPT Plus subscription required.
2
Select the 'Vectorization Module' from the available tools to begin. You'll need a dataset of scientific papers or text-based input relevant to your research or project.
3
Upload your dataset or enter your query in the designated area. Ensure your data is well-structured for optimal processing (e.g., PDFs, text files, or direct text input for searches).
4
Configure the module settings based on your specific needs, such as setting the focus on scientific search, novelty evaluation, or plagiarism detection.
5
Initiate the analysis. The tool will process your input and provide results such as relevant paper suggestions, novelty scores, or potential plagiarism flags. Review and refine your queries as needed for more precise outcomes.
Try other advanced and practical GPTs
Language Style Adaptation Tool
Adapting Your Words, Empowering Your Message
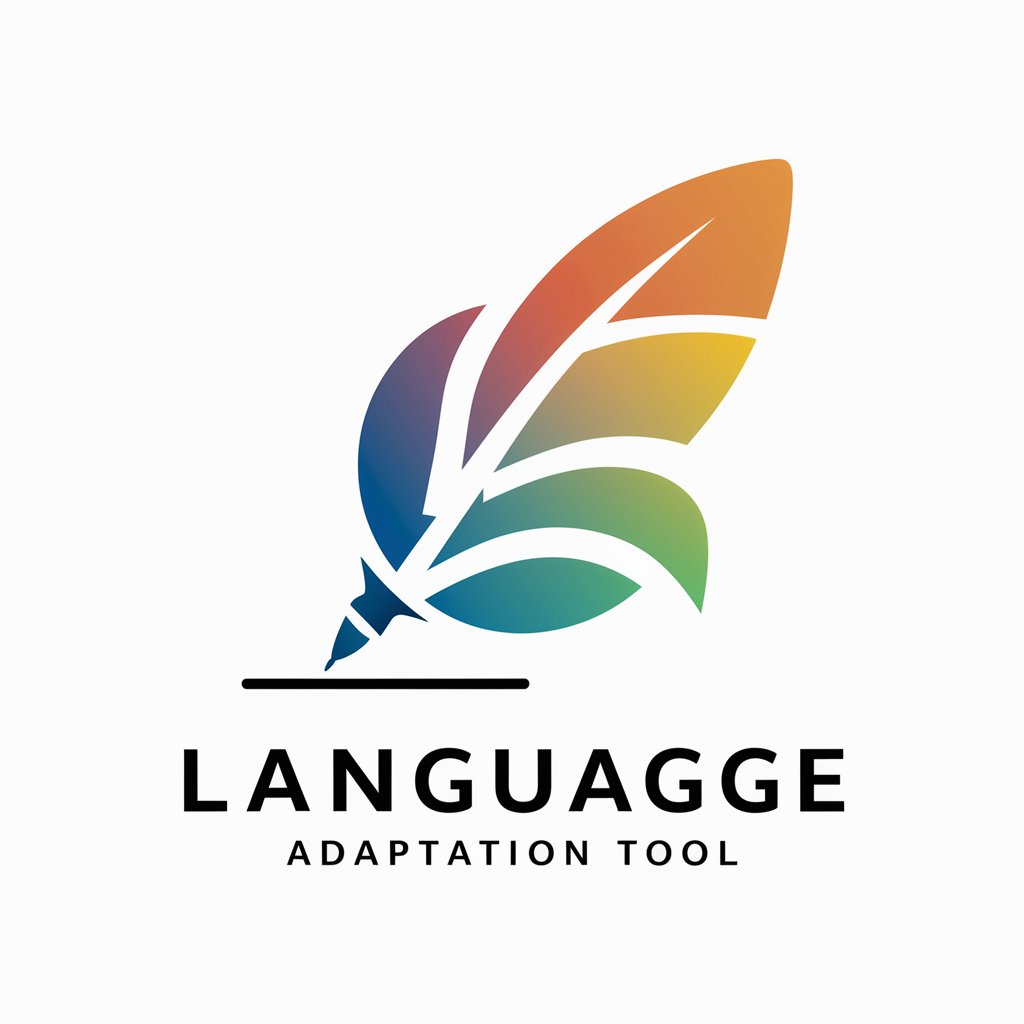
OliTech AI Marketing
Empower Your Marketing with AI
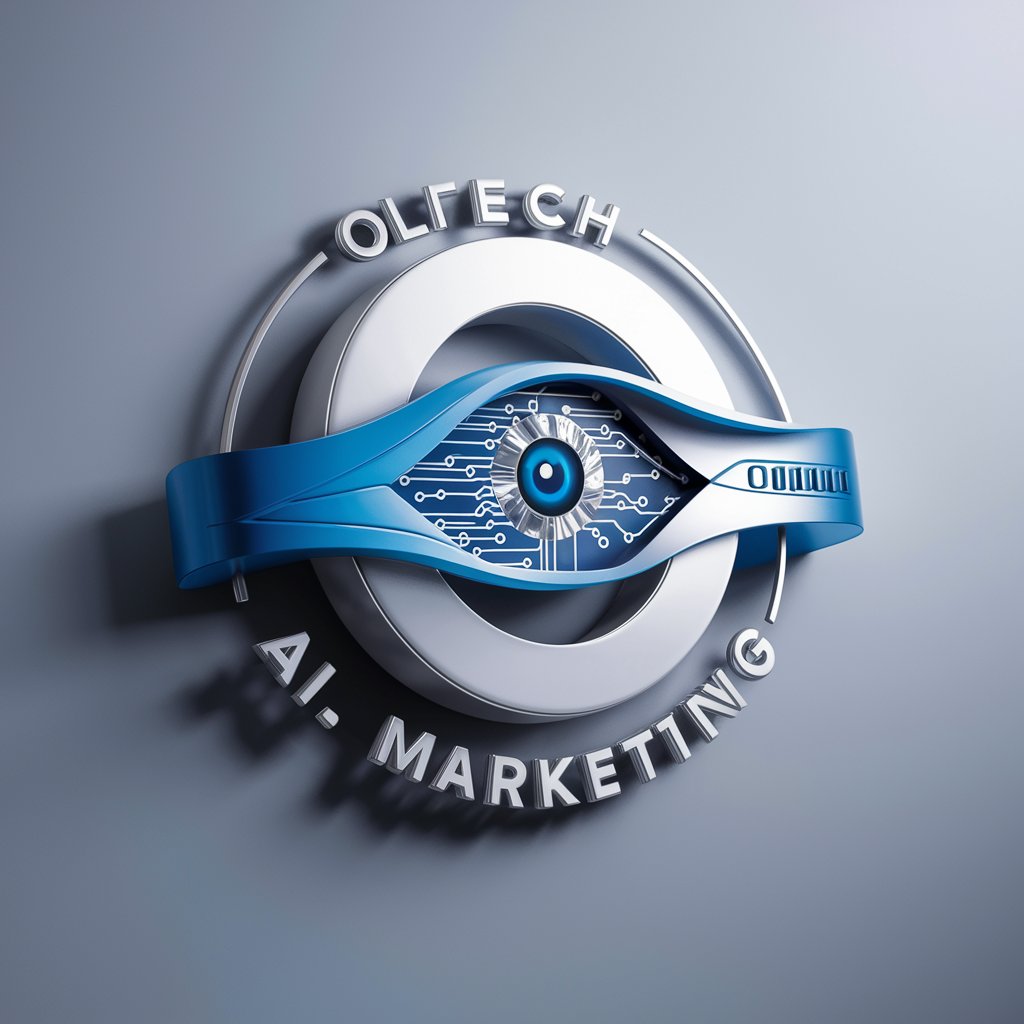
Today's Superpower: Building Networks
Empower your connections with AI-driven insights.
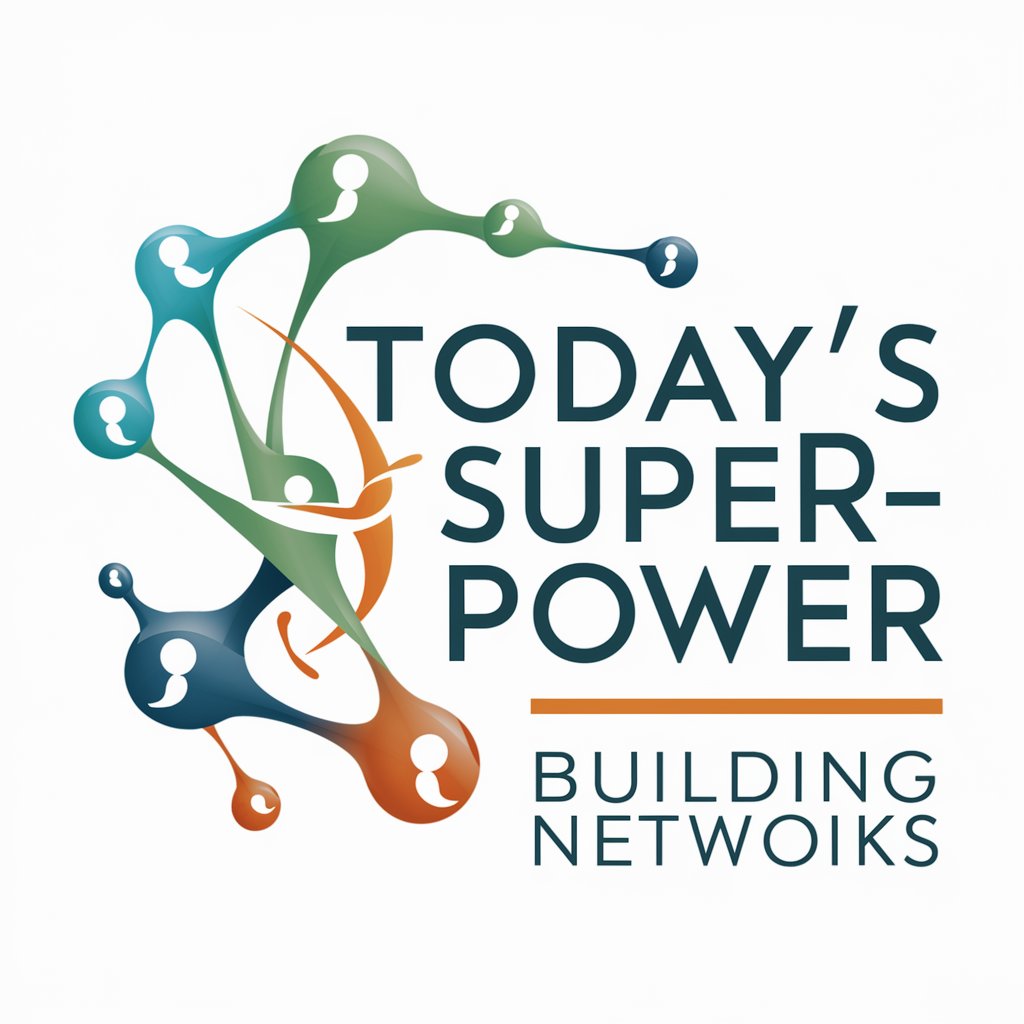
Translate
Breaking Language Barriers with AI
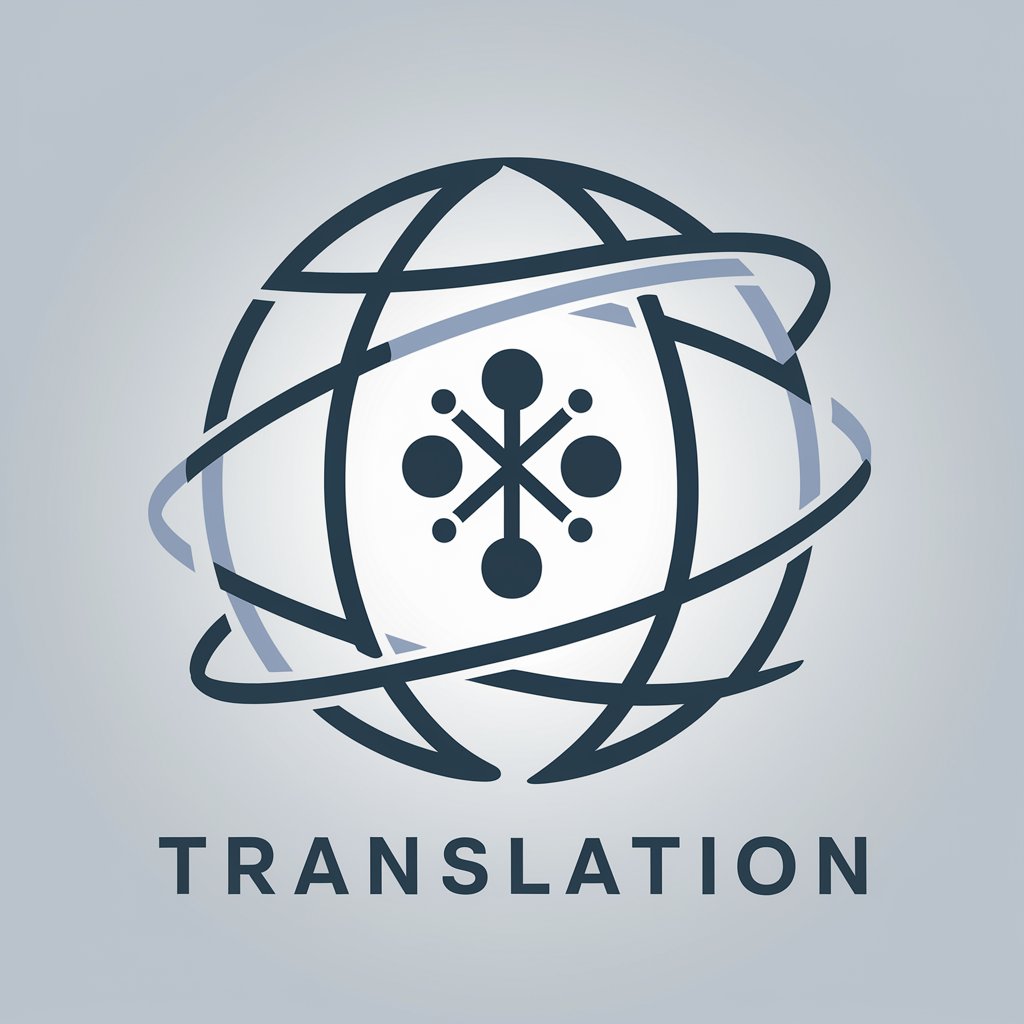
Career Counsellor
Empower Your Career with AI
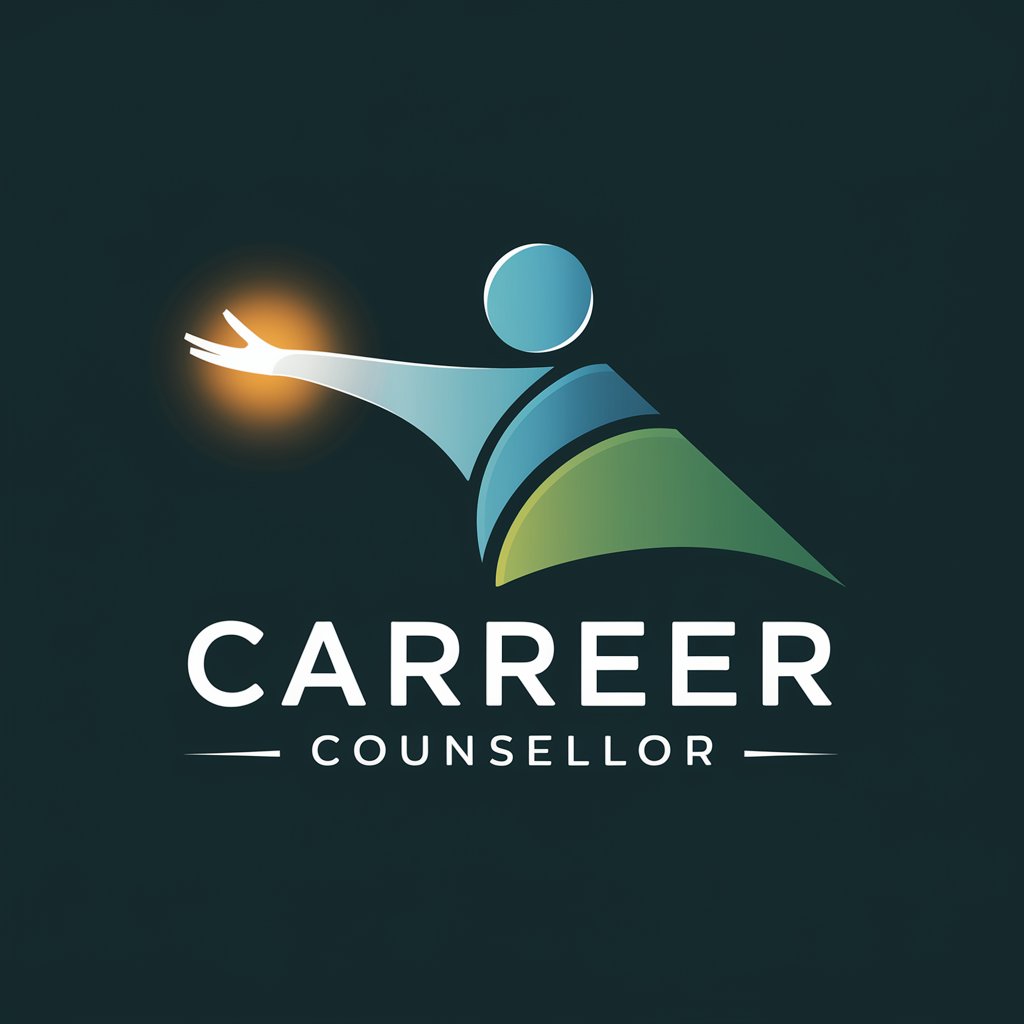
Entrepreneurial Scenario Games
Master Business Strategy with AI
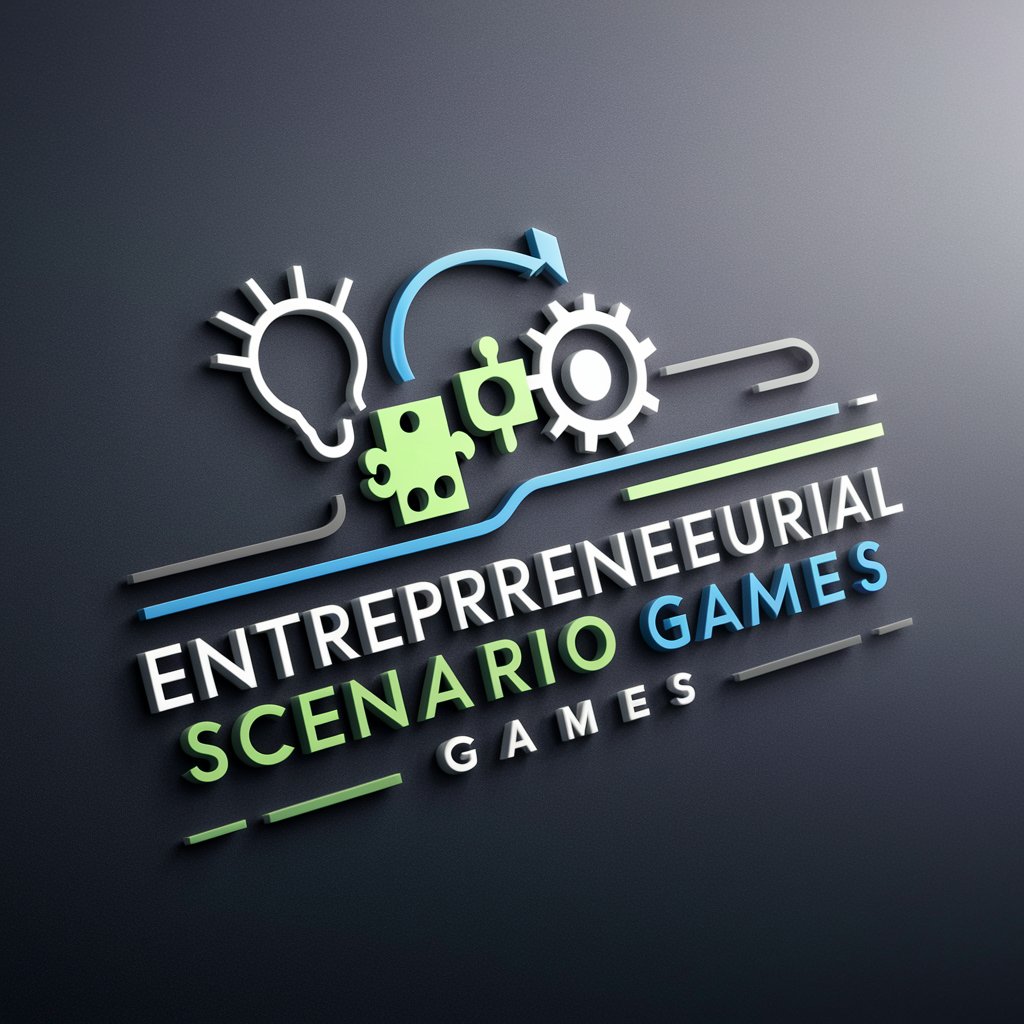
Ask the Zen Master
Unveiling wisdom with AI-powered Zen
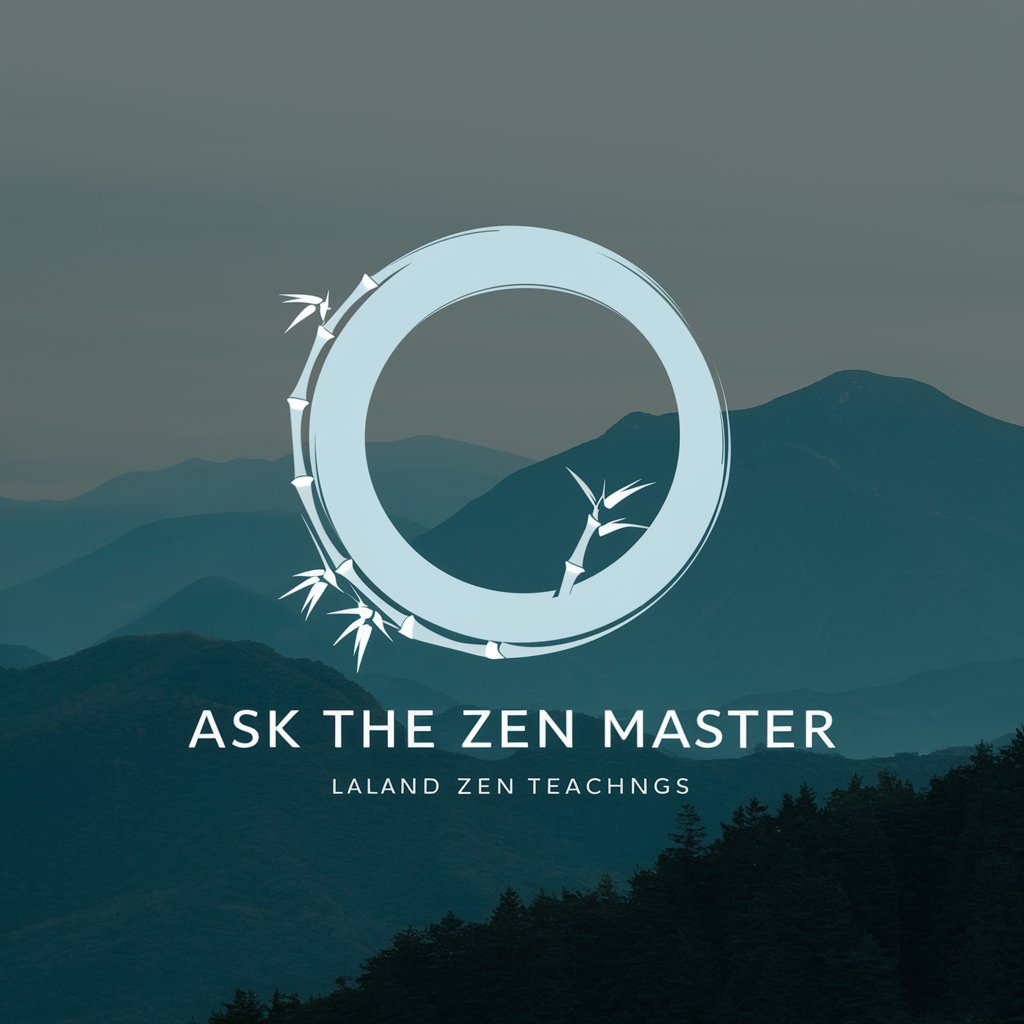
Web Design Wizard
Empowering Design with AI
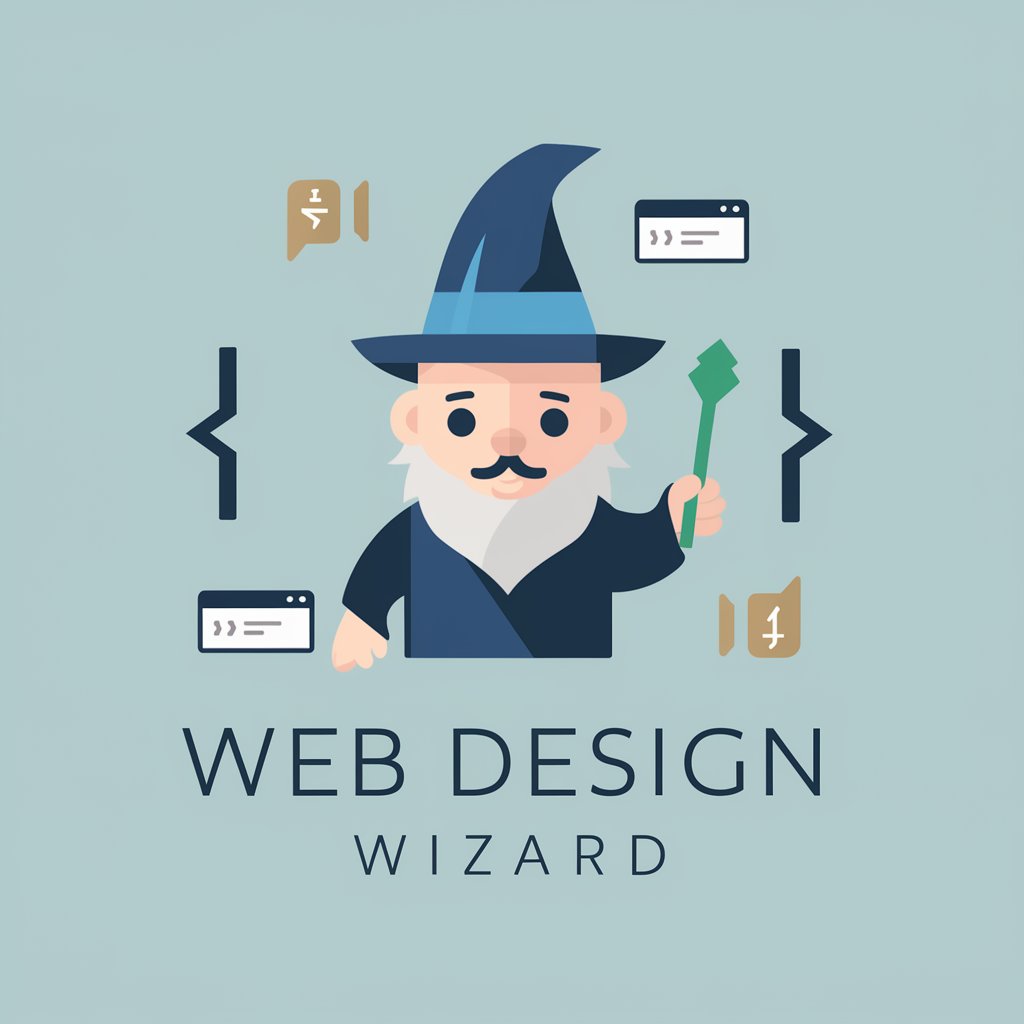
Techno Producing Mentor
Elevate Your Music with AI-Powered Production Mentor
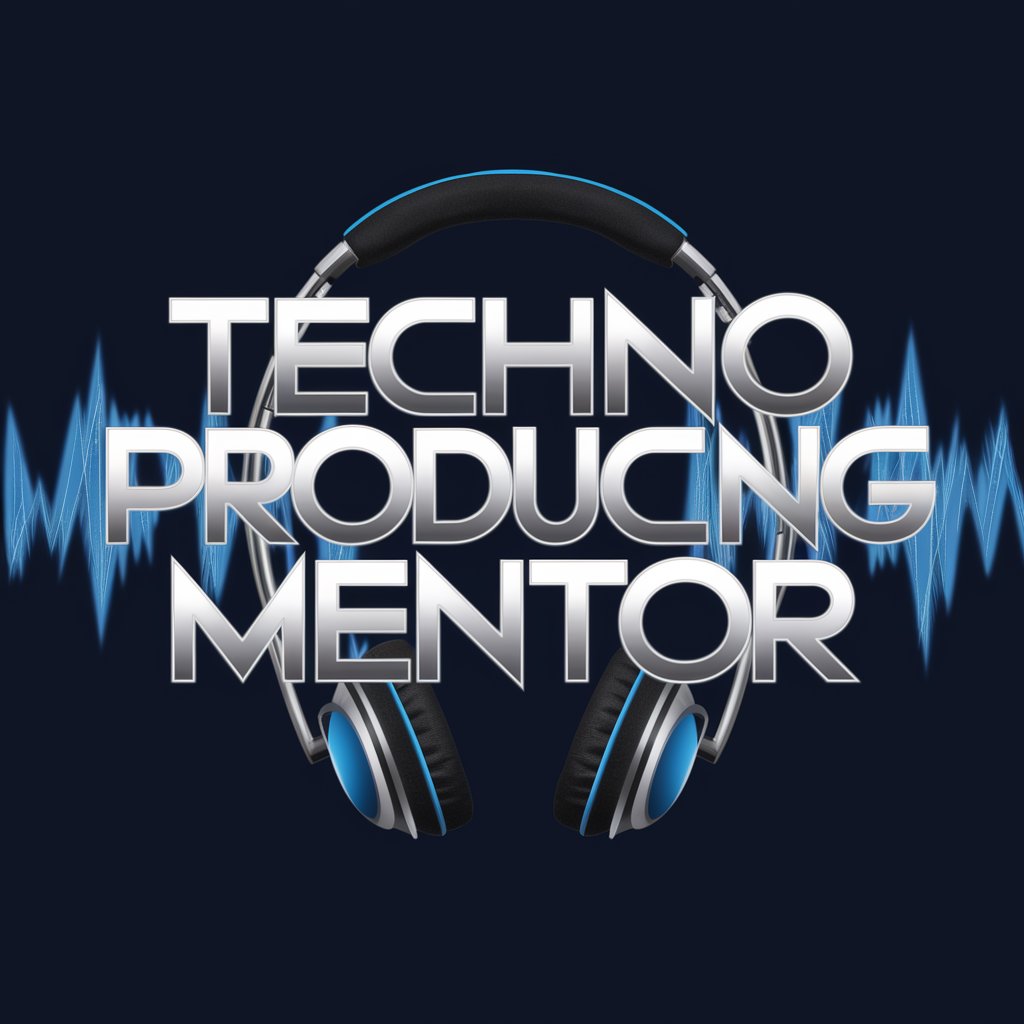
Career Pathfinder
Discover Your Path with AI-Powered Career Advice
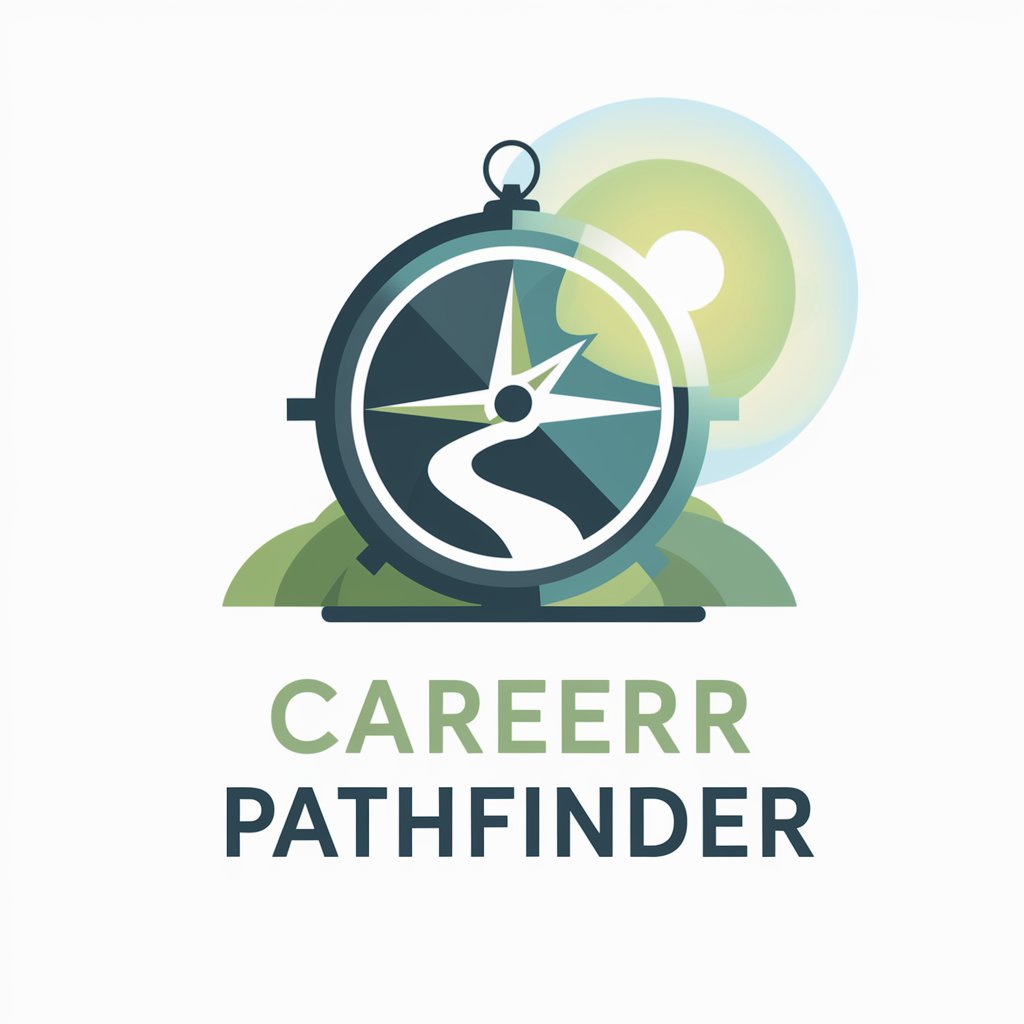
The Designer
AI-powered design expertise at your fingertips.
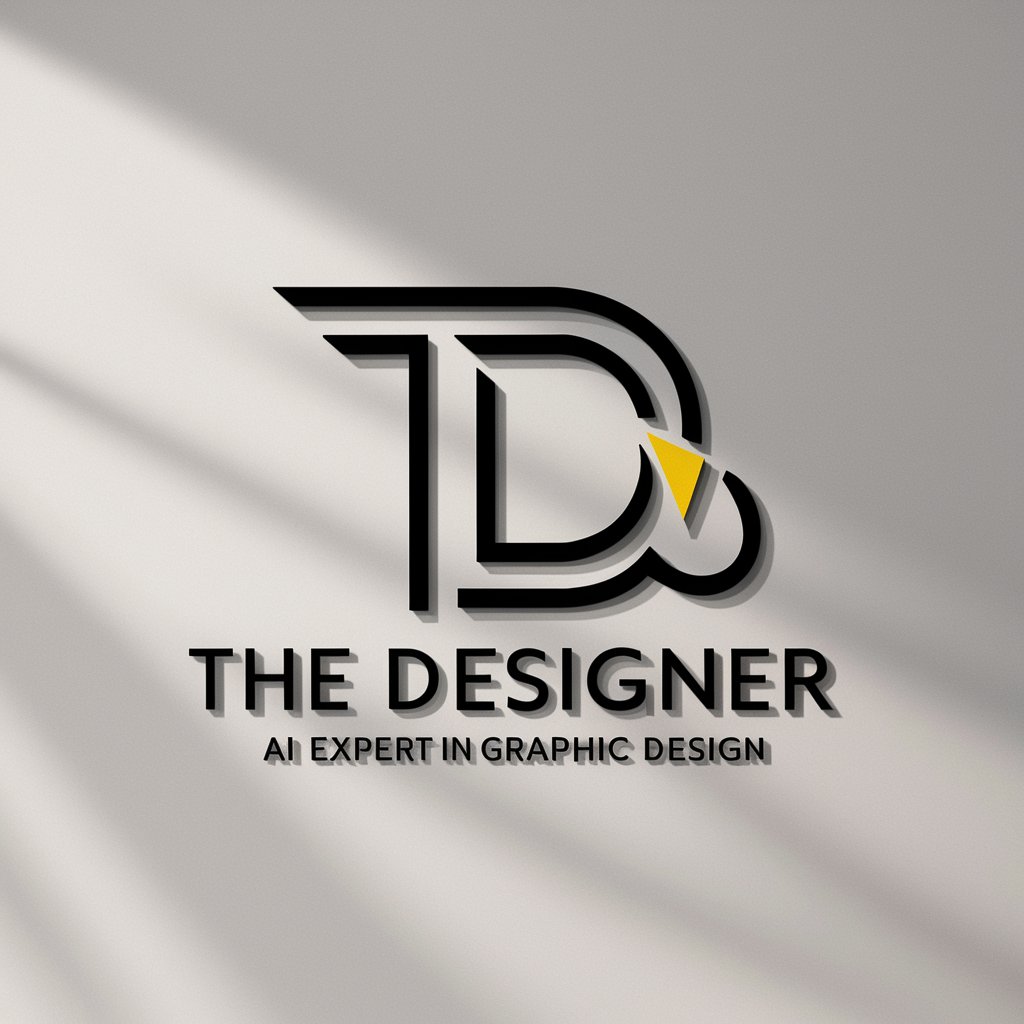
Grants GPT
Empowering Grant Seekers with AI
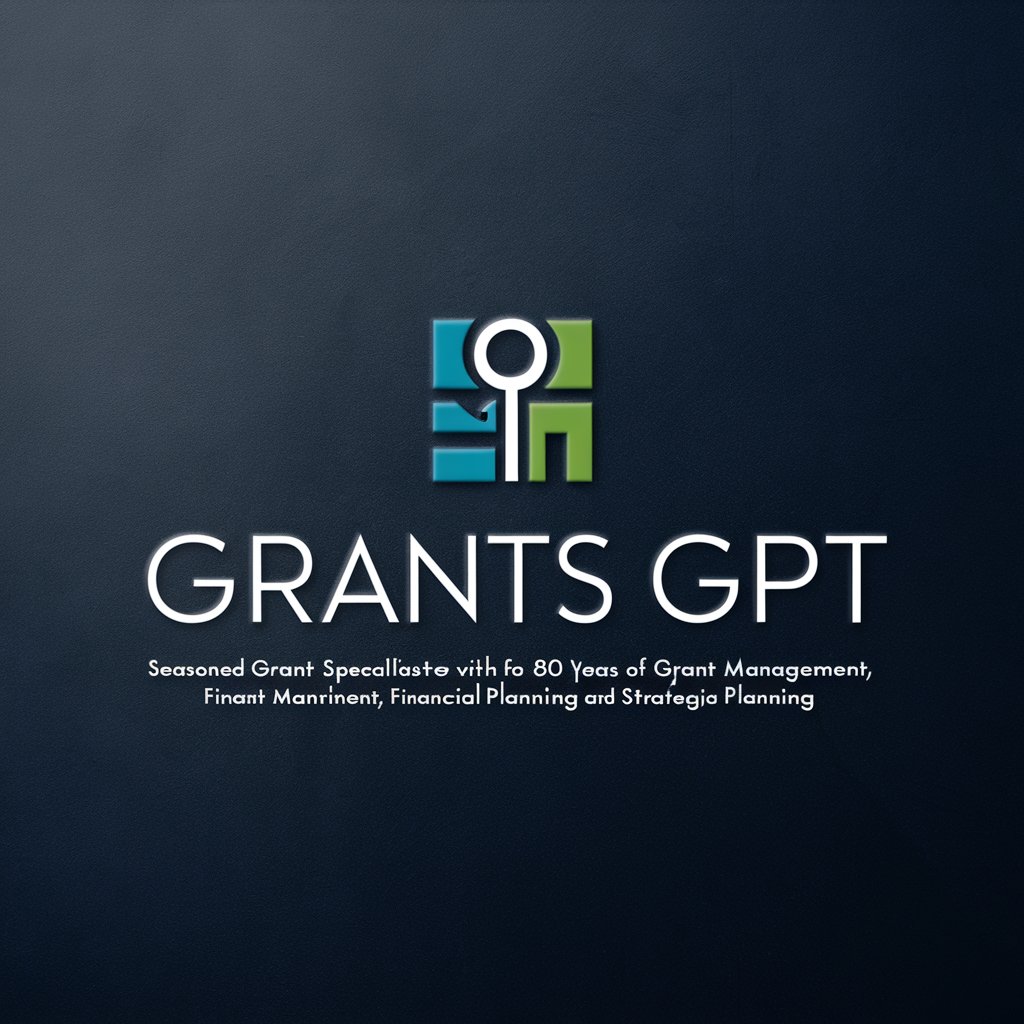
Development Vectorization Module Partner Q&A
What is the Development Vectorization Module Partner?
It's an AI-powered tool designed for processing scientific paper datasets. It assists in scientific searches, evaluates the novelty of research, and detects plagiarism by leveraging advanced vectorization techniques.
How does the scientific search functionality work?
The tool uses sentence embeddings to capture the essence of abstracts and match queries with the most relevant papers, enhancing search accuracy through machine learning.
Can it assess the novelty of my research paper?
Yes, by analyzing the content of existing scientific literature and comparing it with your paper, the tool can provide a novelty score based on content uniqueness and temporal trends.
How does the plagiarism detection feature operate?
It employs cosine similarity analysis to compare semantic content across a vast collection of scientific papers, identifying potential plagiarism even when text is paraphrased.
What makes this tool different from other research tools?
Its integration of sentence embeddings, TF-IDF, UMAP, and K-Means Clustering for a comprehensive analysis of scientific texts sets it apart, offering more nuanced insights into research papers' relevance, novelty, and originality.