Environmental Data Science - AI-Powered Environmental Analysis
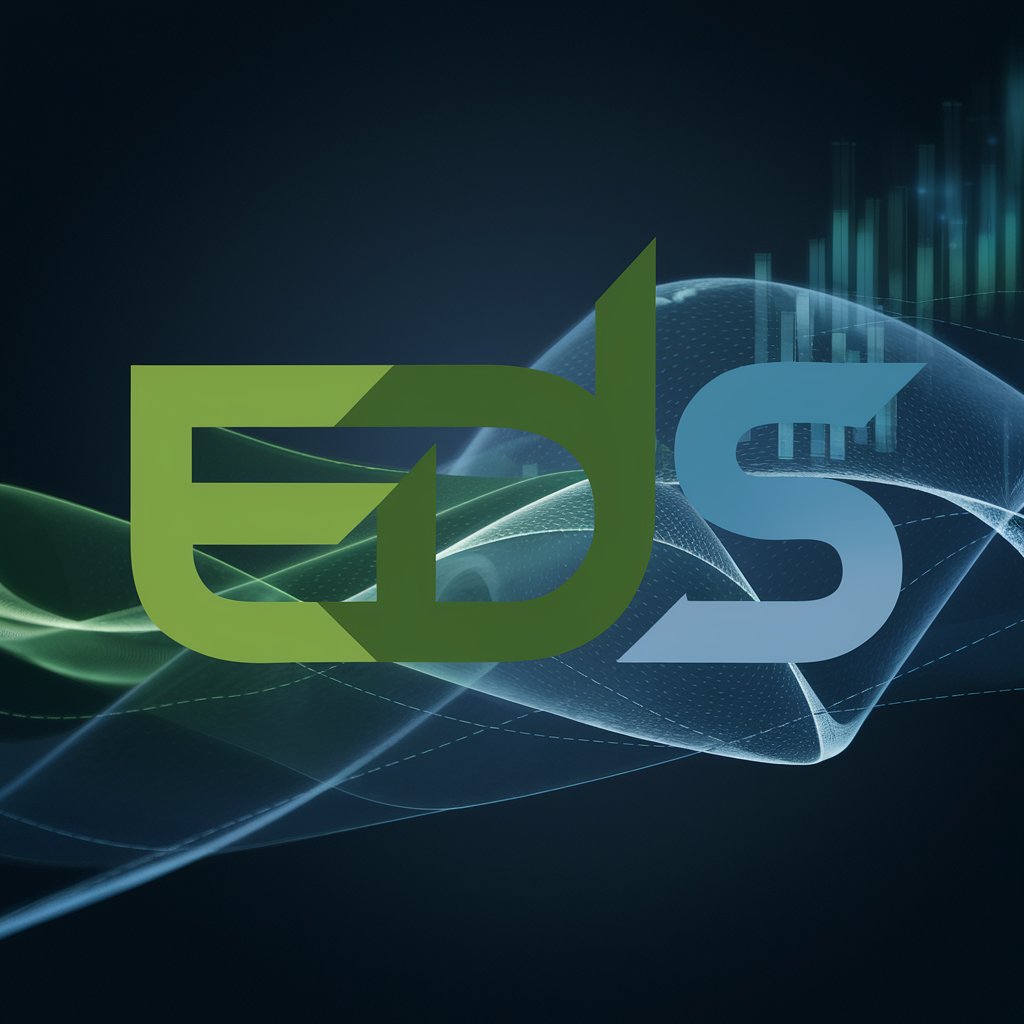
Welcome to Environmental Data Science, where data meets the environment.
Empowering environmental insights through AI
Analyze the impact of climate change on...
Compare classical statistical methods with AI techniques for...
Discuss the advantages and limitations of using data cubes in...
Explain how GDAL VSI can be utilized for...
Get Embed Code
Overview of Environmental Data Science
Environmental Data Science (EDS) represents a multidisciplinary field that combines the power of data science and environmental science to analyze, model, and interpret environmental data. The primary aim is to address and solve complex environmental challenges through the use of data-driven approaches. EDS leverages a wide array of techniques from statistics, machine learning, geographic information systems (GIS), and remote sensing to process and analyze large datasets related to the environment. These datasets may include, but are not limited to, atmospheric data, oceanographic data, land use patterns, and biodiversity indices. For example, by applying machine learning models to satellite imagery and sensor data, EDS can track deforestation patterns over time, predict air quality levels, and assess the impacts of climate change on natural habitats. This interdisciplinary approach enables the development of actionable insights and solutions for sustainable management of natural resources and environmental protection. Powered by ChatGPT-4o。
Key Functions and Applications
Data Analysis and Modeling
Example
Using statistical models to analyze historical climate data and predict future climate conditions.
Scenario
Researchers employ EDS to study trends in global temperature and precipitation data over decades, helping to forecast climate change impacts and inform policy decisions.
Environmental Monitoring
Example
Applying remote sensing techniques to monitor deforestation and land use changes.
Scenario
Conservation organizations use EDS to analyze satellite images, detecting illegal logging activities in protected areas and assessing the effectiveness of conservation strategies.
Pollution Assessment
Example
Evaluating air and water quality using sensor data and geographic information systems (GIS).
Scenario
Public health agencies apply EDS to map pollution sources and exposure risks, guiding interventions to reduce health impacts on communities.
Biodiversity Conservation
Example
Mapping habitats and assessing biodiversity through species distribution models.
Scenario
Wildlife conservationists utilize EDS to identify critical habitats, predict species distribution under various climate scenarios, and prioritize areas for conservation efforts.
Sustainable Resource Management
Example
Analyzing water usage patterns and forecasting demand to support sustainable water management.
Scenario
Water resource managers leverage EDS to model water supply and demand under different scenarios, aiding in the development of water conservation policies and practices.
Target User Groups
Environmental Researchers and Scientists
Individuals and organizations engaged in scientific research on environmental issues. They benefit from EDS by gaining access to advanced tools for analyzing complex environmental datasets, thereby enhancing their research capabilities and contributing to evidence-based environmental policy-making.
Conservation Organizations and NGOs
Non-governmental organizations and groups focused on conservation and environmental protection. These users apply EDS to monitor ecosystems, assess biodiversity, and implement effective conservation strategies based on data-driven insights.
Government and Policy Makers
Government agencies and policy makers responsible for environmental regulation and natural resource management. EDS provides them with the necessary data and models to make informed decisions on environmental policy, land use planning, and sustainable development initiatives.
Urban Planners and Developers
Professionals involved in urban planning and development who require environmental assessments to ensure sustainable growth. EDS tools help them integrate environmental considerations into planning processes, mitigating negative impacts on the environment.
Public Health Officials
Health agencies and officials concerned with the environmental determinants of health, such as air and water quality. EDS offers methodologies for assessing pollution exposure and its health impacts, supporting public health interventions and policies.
How to Use Environmental Data Science
1
Access the platform by visiting a site offering a free trial without the need for login or a ChatGPT Plus subscription.
2
Familiarize yourself with the data types and formats, such as spatial data in GeoTIFF or tabular data in CSV, that are commonly used in environmental analyses.
3
Identify the environmental issue or research question you want to explore, such as climate change impacts, biodiversity assessments, or pollution monitoring.
4
Utilize the tool’s capabilities to process and analyze the data, leveraging machine learning algorithms, statistical models, and geospatial analysis techniques.
5
Interpret and visualize the results to derive actionable insights, using built-in visualization tools and reporting features.
Try other advanced and practical GPTs
TRE(AI)TY
Empowering legal decisions with AI
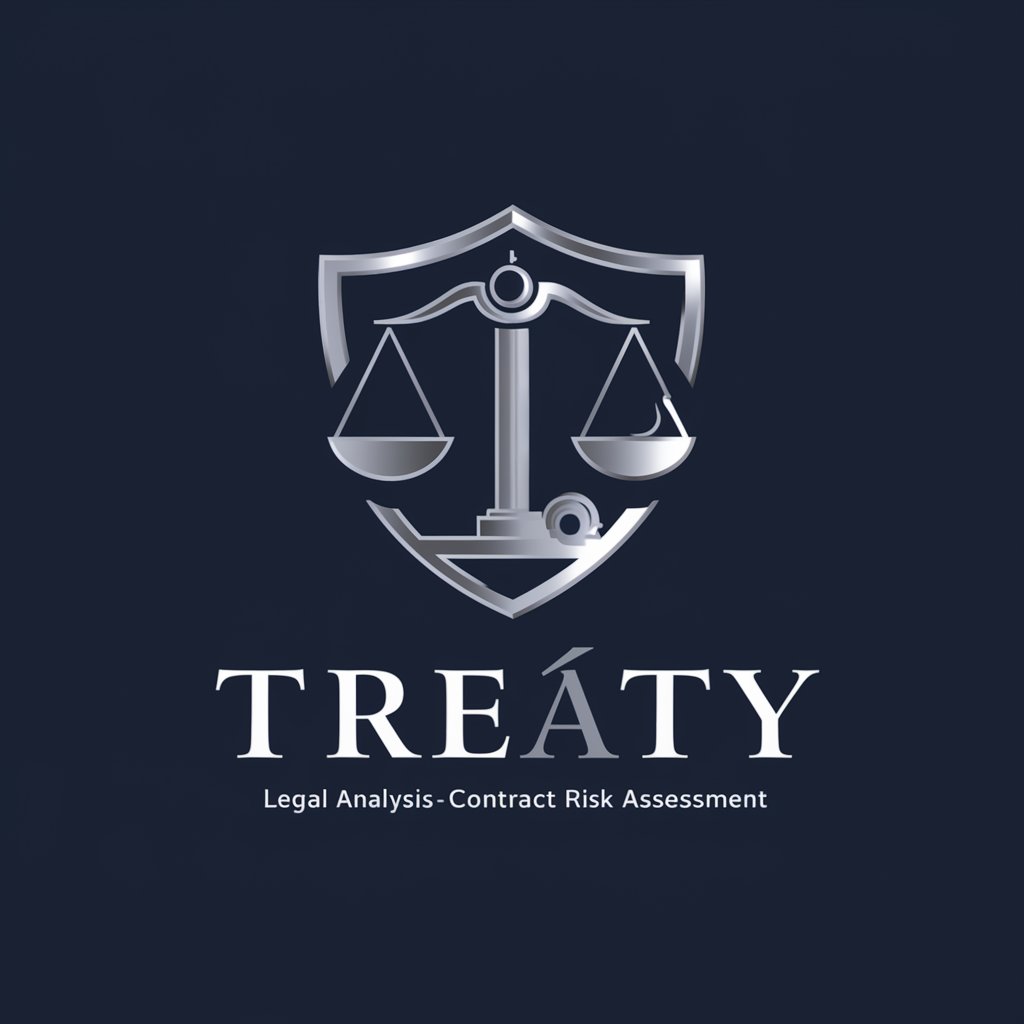
ty
Your AI-powered task navigator
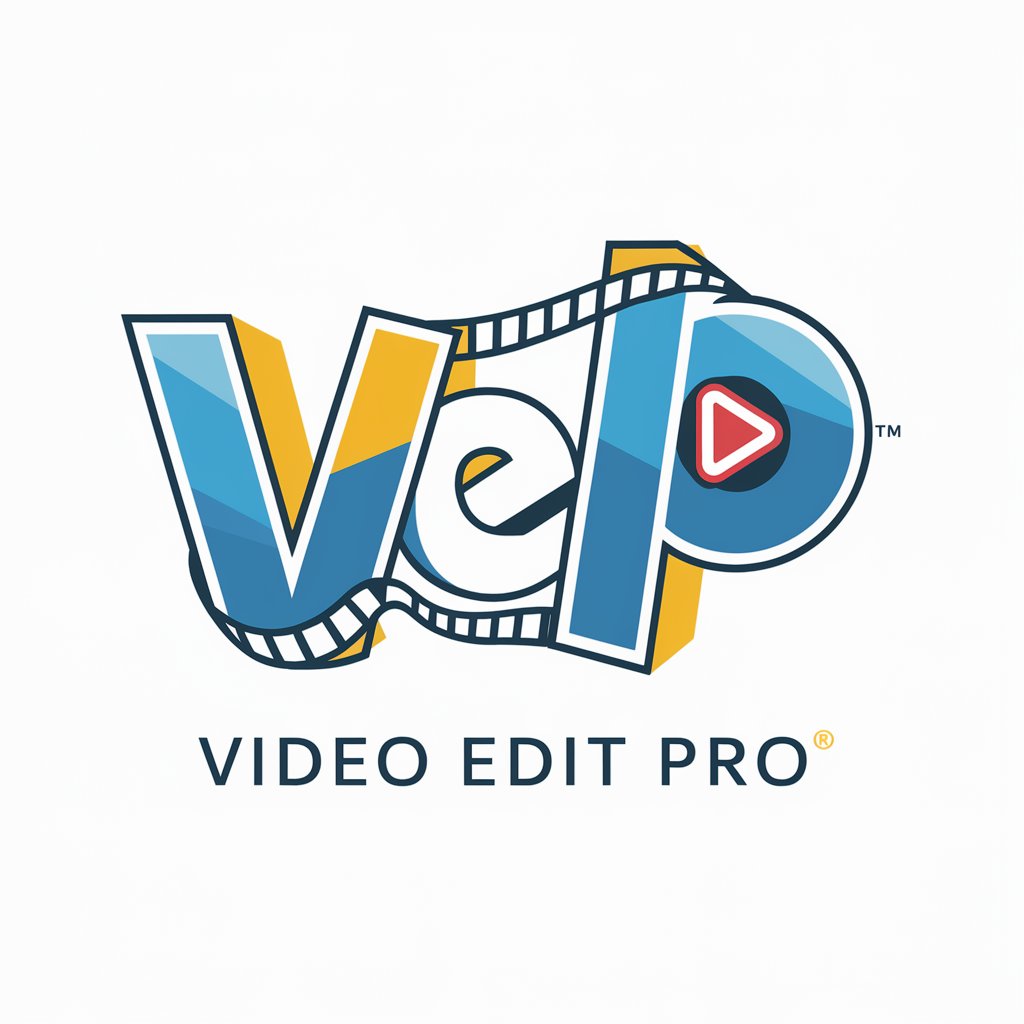
Dev
Empowering Ideas with AI-Driven Development
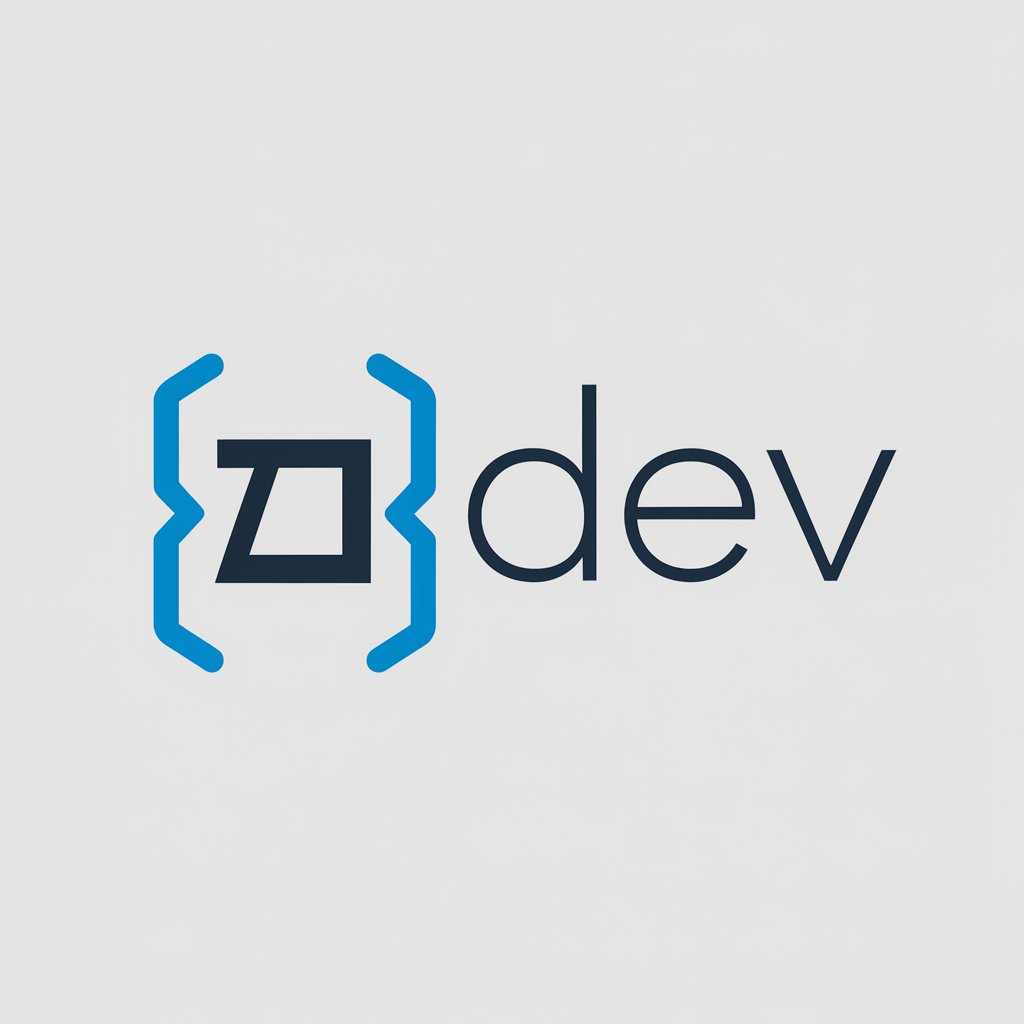
Dev Mentor
Empowering Learning with AI
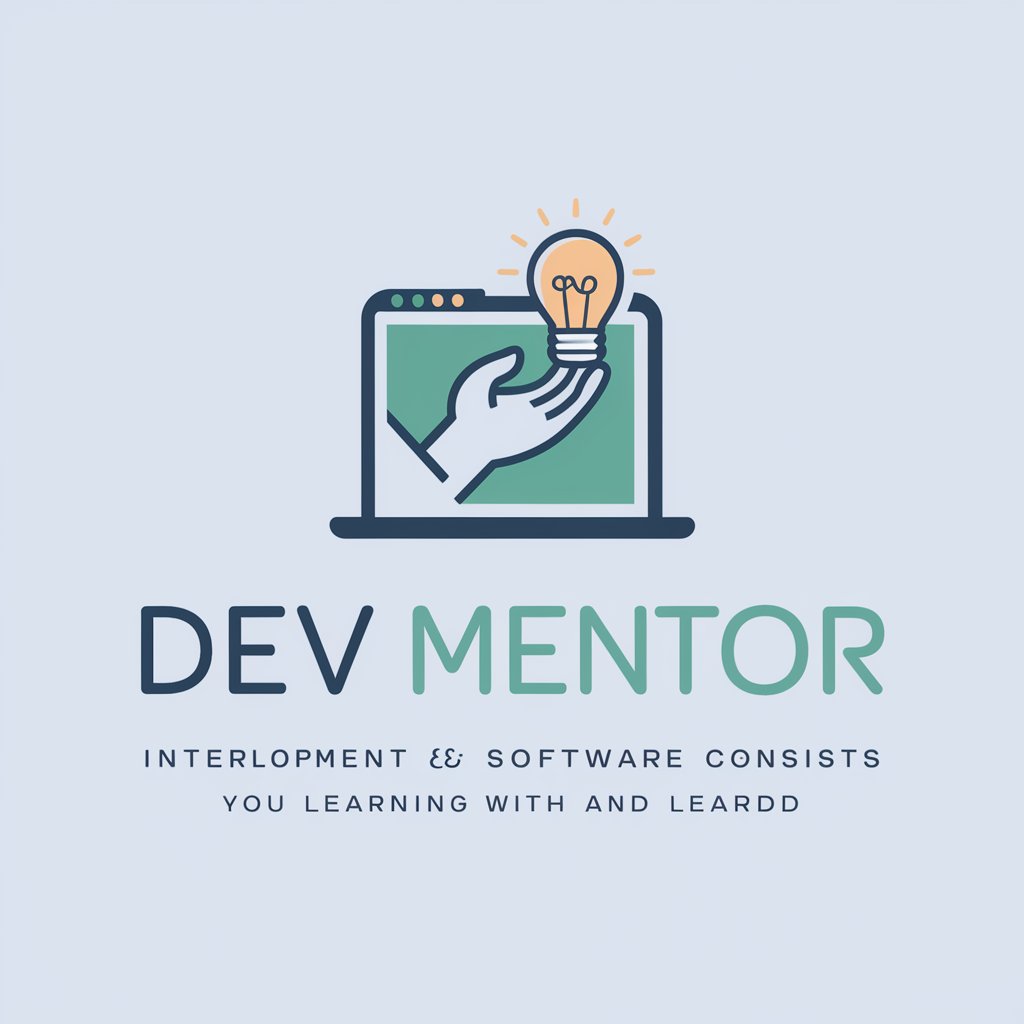
Dev Dev
Elevating Code with AI Power
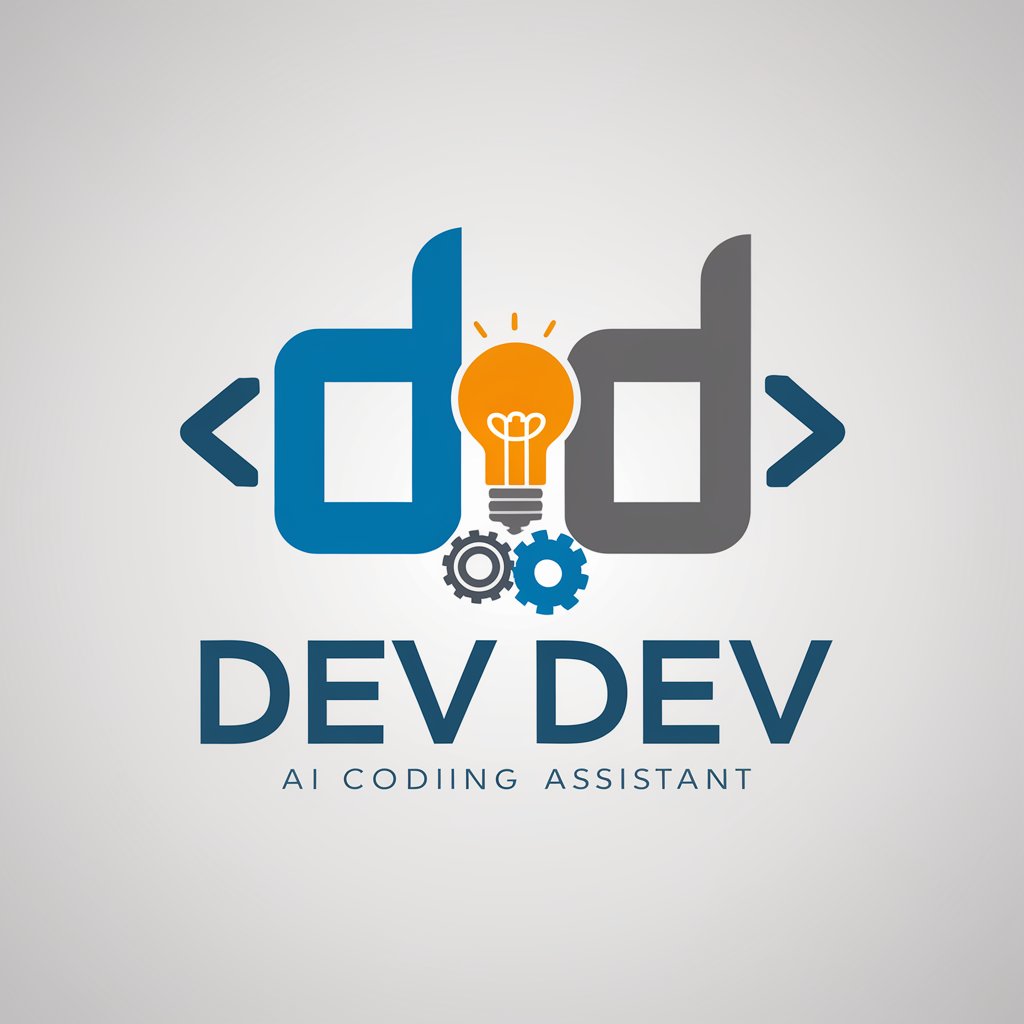
DEV PUSH
Empower Your Web Projects with AI
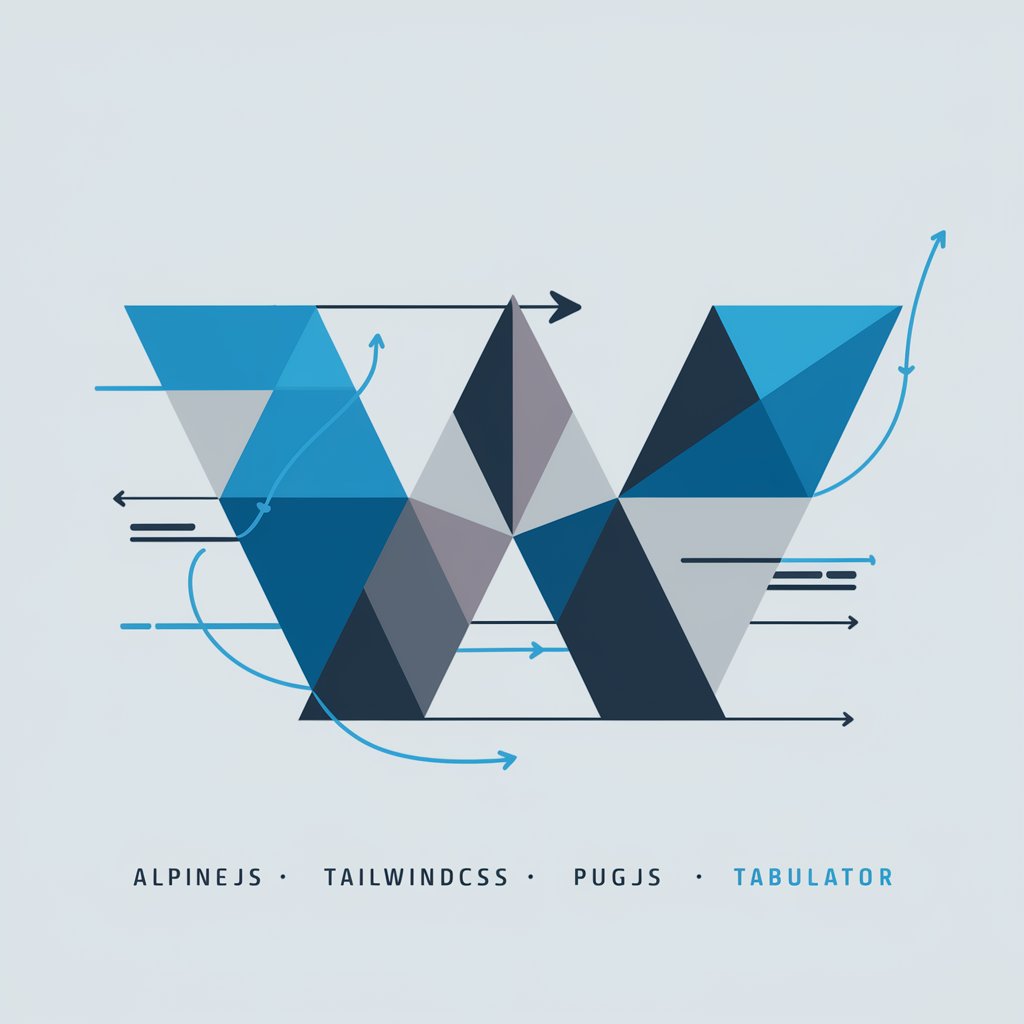
The Minor(AI)ty Report
Empowering research at the intersection of AI and African American perspectives.
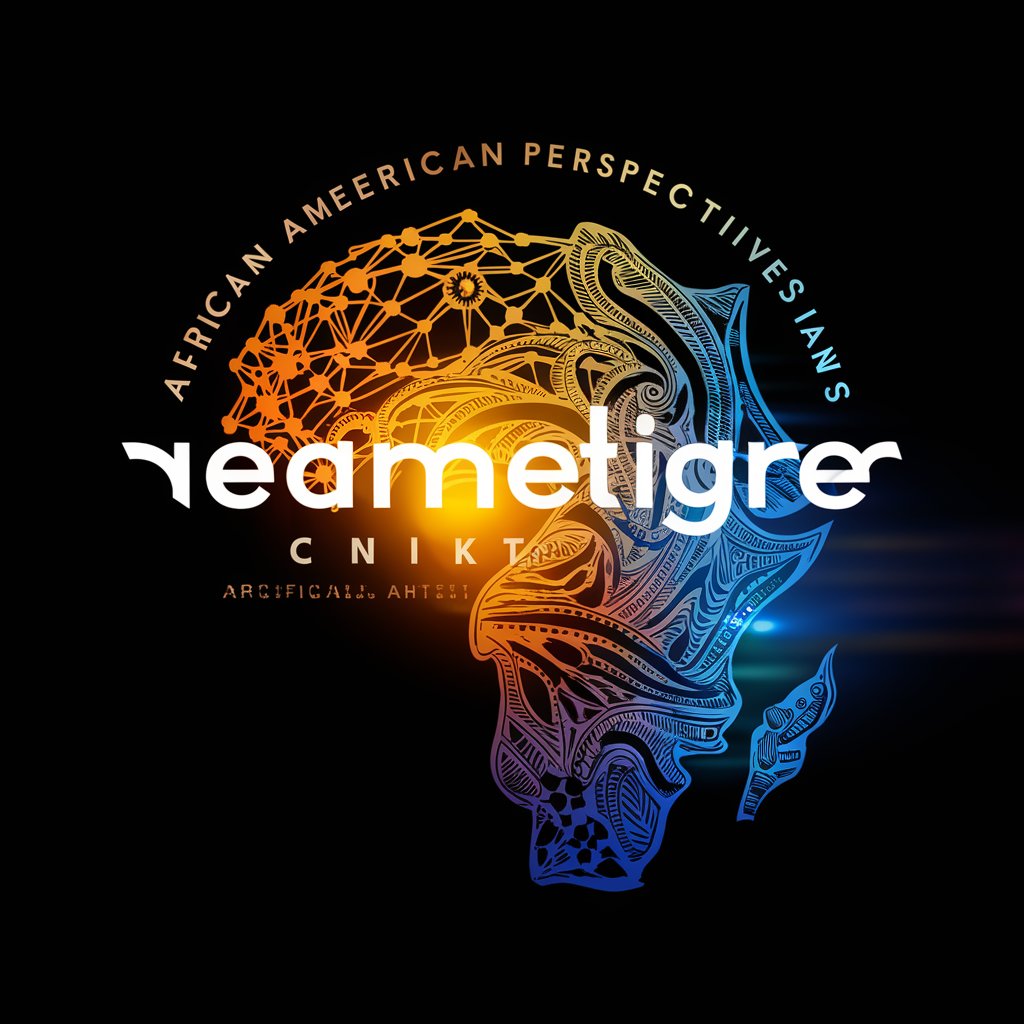
CodingGPT
Transforming ideas into code effortlessly.
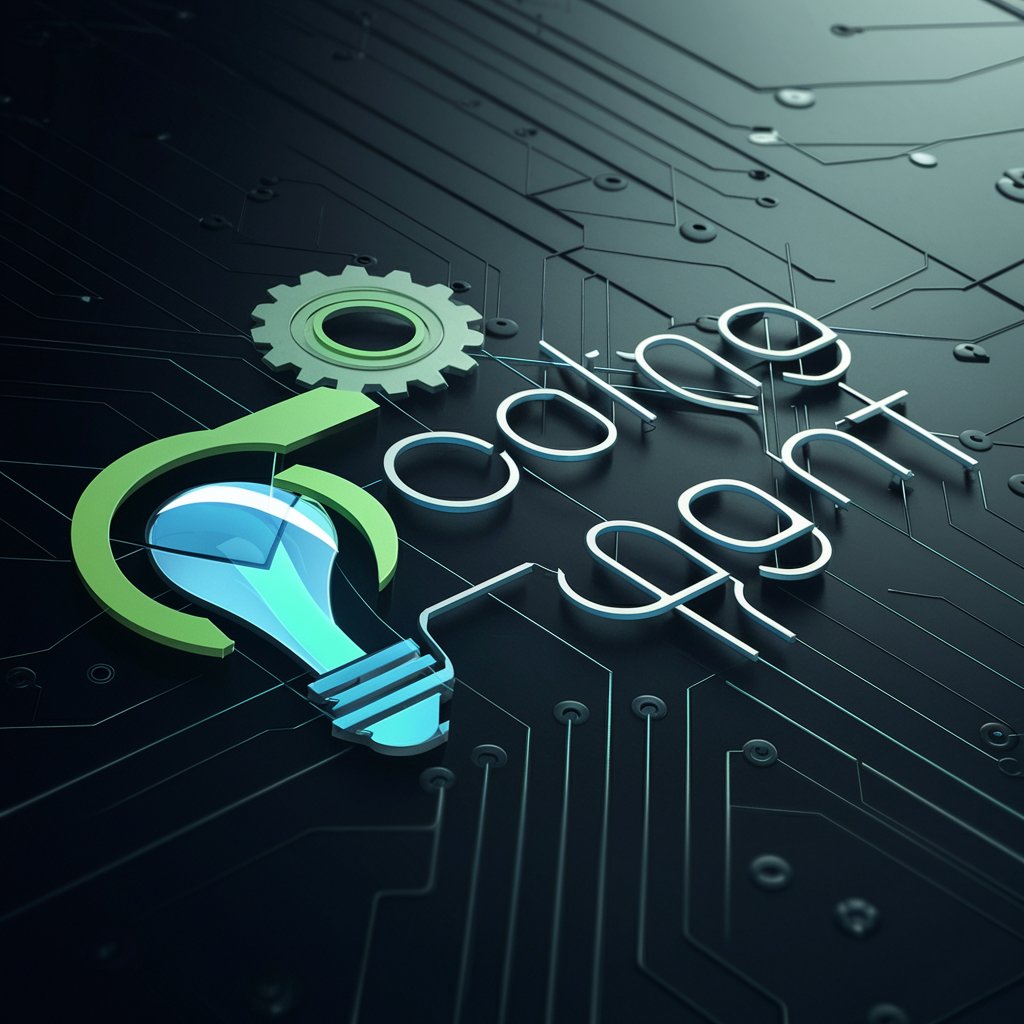
Open Designer
Revitalize Spaces with AI
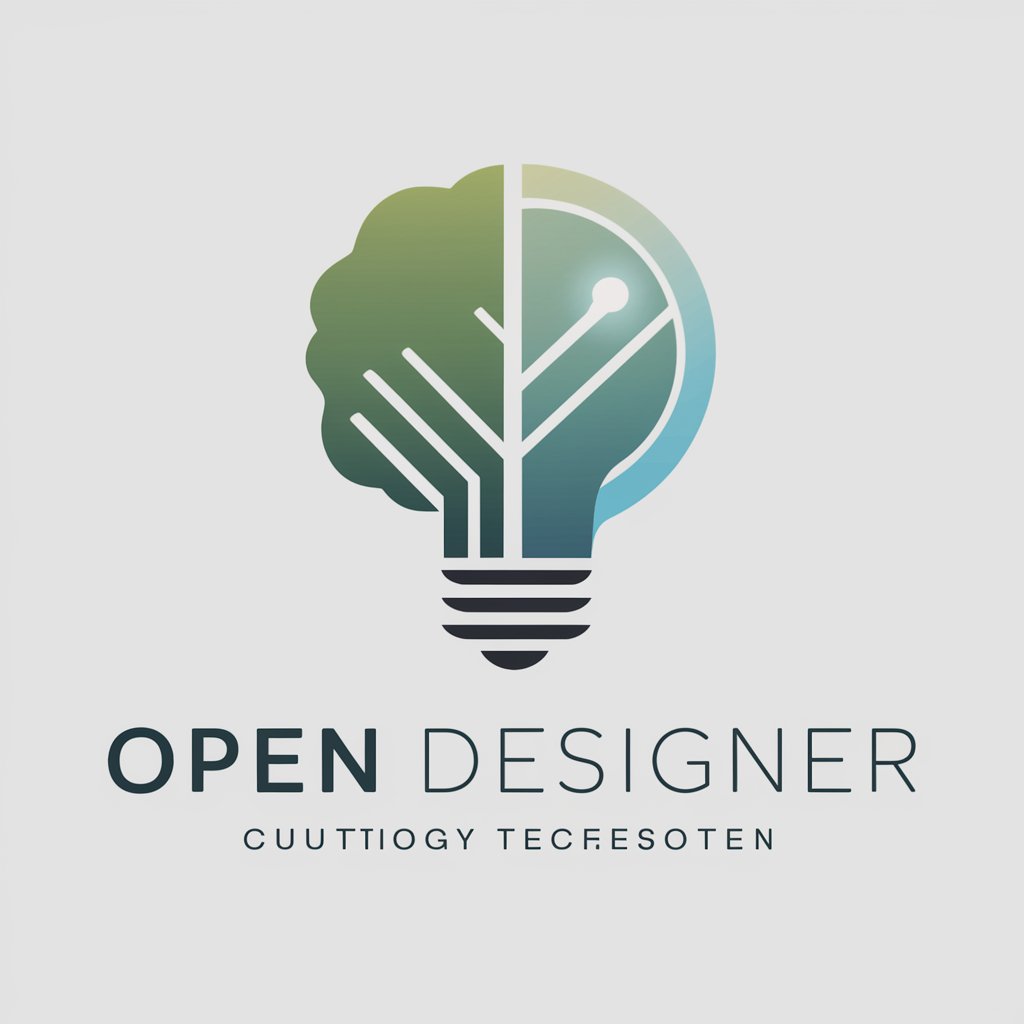
Open Factory
Empowering Creativity with AI
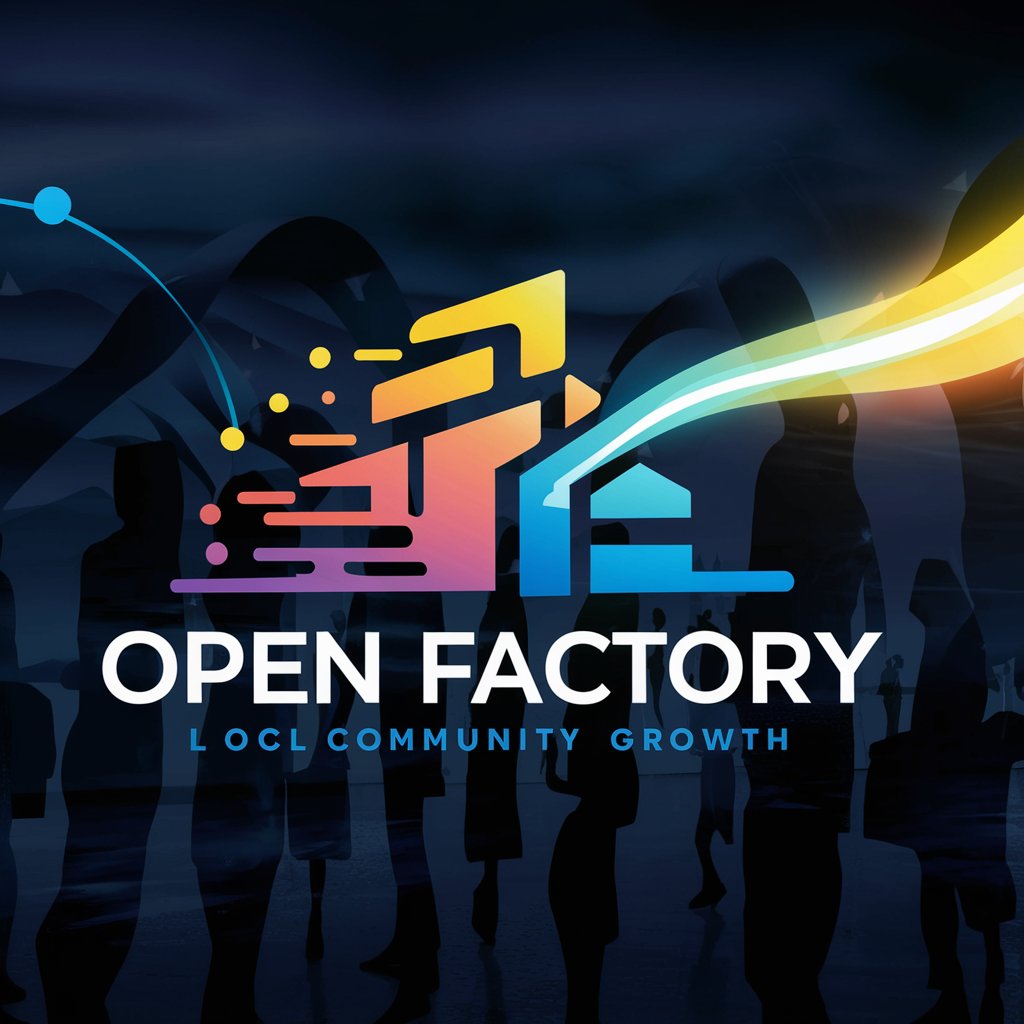
Open Advisor
Unlock insights with AI-powered expertise.
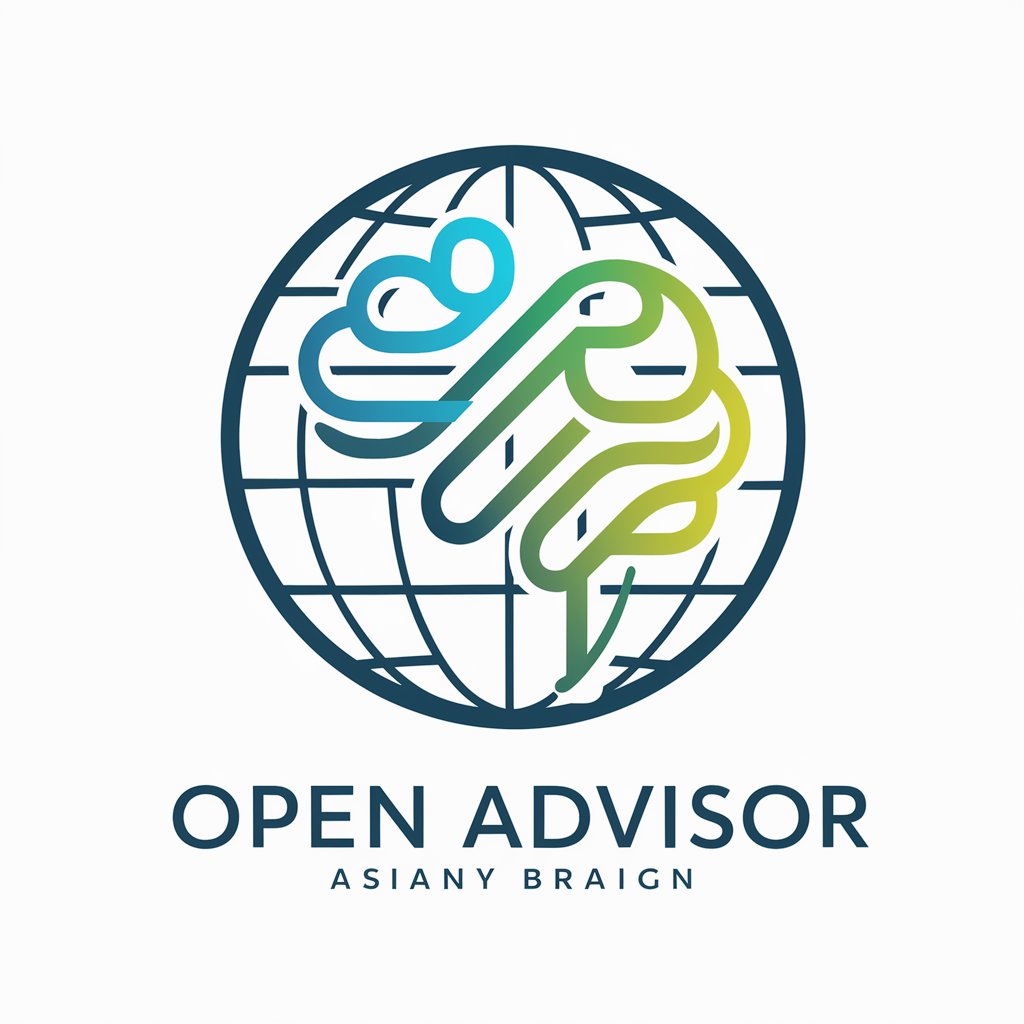
Open Mind
Empowering Immigrants with AI-Powered Insights
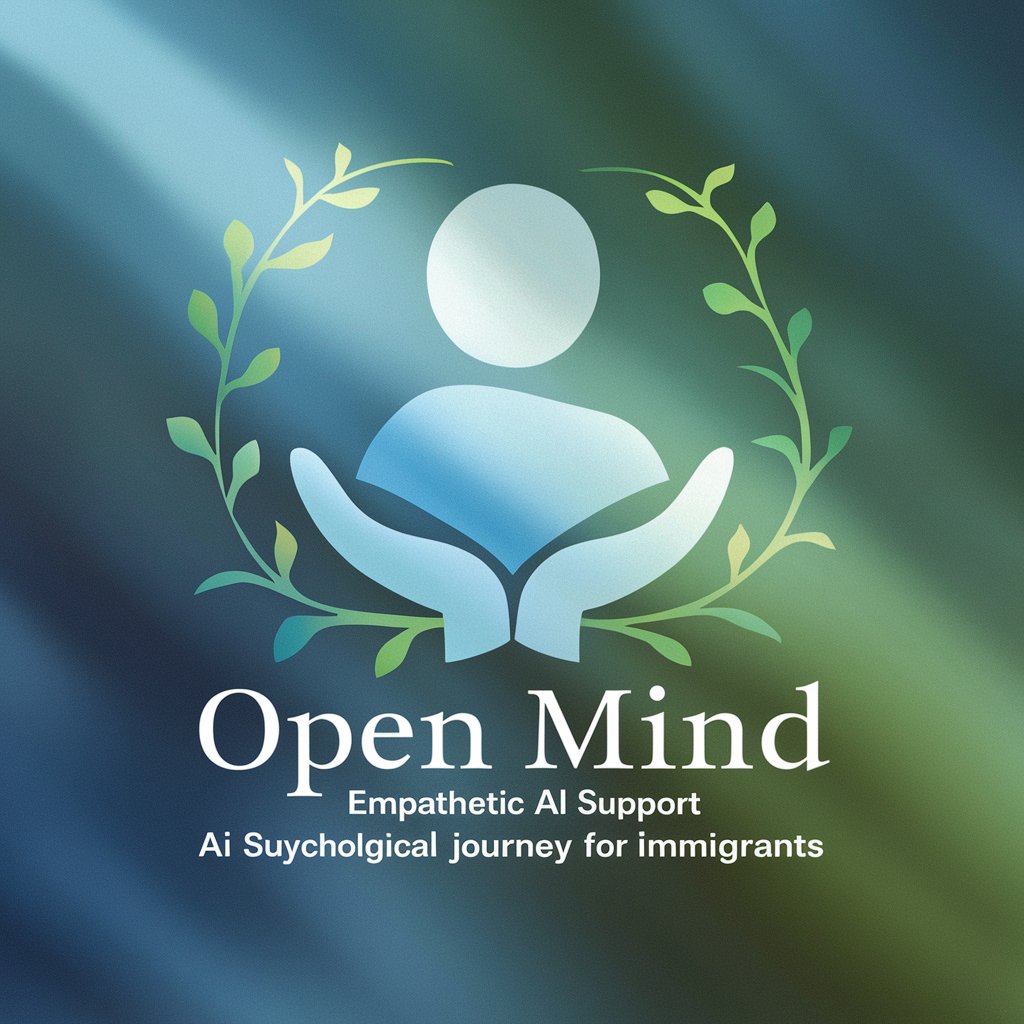
FAQs on Environmental Data Science
What data formats are supported by Environmental Data Science tools?
Supported formats include raster (e.g., GeoTIFF), vector (e.g., Shapefile, GeoJSON), and tabular data (e.g., CSV, Parquet).
How does Environmental Data Science integrate with AI?
It integrates through machine learning models for predicting environmental trends, deep learning for image analysis, and data mining techniques for uncovering patterns.
Can Environmental Data Science handle large datasets?
Yes, it can manage large datasets through distributed computing platforms and efficient data storage formats like Parquet.
What are common use cases for Environmental Data Science?
Common use cases include climate change analysis, environmental impact assessment, resource management, and pollution tracking.
How do you ensure the accuracy of environmental data analysis?
Accuracy is ensured through data validation, calibration of models, rigorous testing against known datasets, and continuous updating of data sources and algorithms.